一、概述
Janus-Pro是DeepSeek最新开源的多模态模型,是一种新颖的自回归框架,统一了多模态理解和生成。通过将视觉编码解耦为独立的路径,同时仍然使用单一的、统一的变压器架构进行处理,该框架解决了先前方法的局限性。这种解耦不仅缓解了视觉编码器在理解和生成中的角色冲突,还增强了框架的灵活性。Janus-Pro 超过了以前的统一模型,并且匹配或超过了特定任务模型的性能。
代码链接:https://github.com/deepseek-ai/Janus
模型链接:https://modelscope.cn/collections/Janus-Pro-0f5e48f6b96047
体验页面:https://modelscope.cn/studios/AI-ModelScope/Janus-Pro-7B
二、虚拟环境
环境说明
本文使用WSL2运行的ubuntu系统来进行演示,参考链接:https://www.cnblogs.com/xiao987334176/p/18864140
创建虚拟环境
conda create --name vll-Janus-Pro-7B python=3.12.7
激活虚拟环境,执行命令:
conda activate vll-Janus-Pro-7B
查看CUDA版本,执行命令:
# nvcc -V
nvcc: NVIDIA (R) Cuda compiler driver
Copyright (c) 2005-2025 NVIDIA Corporation
Built on Wed_Jan_15_19:20:09_PST_2025
Cuda compilation tools, release 12.8, V12.8.61
Build cuda_12.8.r12.8/compiler.35404655_0
三、安装Janus-Pro
创建项目目录
mkdir vllm
cd vllm
克隆代码
git clone https://github.com/deepseek-ai/Janus
安装依赖包,注意:这里要手动安装pytorch,指定版本。
pip3 install torch torchvision torchaudio --index-url https://download.pytorch.org/whl/cu128
安装其他依赖组件
pip3 install transformers attrdict einops timm
下载模型
可以用modelscope下载,安装modelscope,命令如下:
pip install modelscope
modelscope download --model deepseek-ai/Janus-Pro-7B
效果如下:
# modelscope download --model deepseek-ai/Janus-Pro-7B
Downloading Model from https://www.modelscope.cn to directory: /root/.cache/modelscope/hub/models/deepseek-ai/Janus-Pro-7B
Downloading [config.json]: 100%|███████████████████████████████████████████████████| 1.42k/1.42k [00:00<00:00, 5.29kB/s]
Downloading [configuration.json]: 100%|████████████████████████████████████████████████| 68.0/68.0 [00:00<00:00, 221B/s]
Downloading [README.md]: 100%|█████████████████████████████████████████████████████| 2.49k/2.49k [00:00<00:00, 7.20kB/s]
Downloading [processor_config.json]: 100%|███████████████████████████████████████████████| 210/210 [00:00<00:00, 590B/s]
Downloading [janus_pro_teaser1.png]: 100%|██████████████████████████████████████████| 95.7k/95.7k [00:00<00:00, 267kB/s]
Downloading [preprocessor_config.json]: 100%|████████████████████████████████████████████| 346/346 [00:00<00:00, 867B/s]
Downloading [janus_pro_teaser2.png]: 100%|███████████████████████████████████████████| 518k/518k [00:00<00:00, 1.18MB/s]
Downloading [special_tokens_map.json]: 100%|███████████████████████████████████████████| 344/344 [00:00<00:00, 1.50kB/s]
Downloading [tokenizer_config.json]: 100%|███████████████████████████████████████████████| 285/285 [00:00<00:00, 926B/s]
Downloading [pytorch_model.bin]: 0%|▏ | 16.0M/3.89G [00:00<03:55, 17.7MB/s]
Downloading [tokenizer.json]: 100%|████████████████████████████████████████████████| 4.50M/4.50M [00:00<00:00, 6.55MB/s]
Processing 11 items: 91%|█████████████████████████████████████████████████████▋ | 10.0/11.0 [00:19<00:00, 14.1it/s]
Downloading [pytorch_model.bin]: 100%|█████████████████████████████████████████████| 3.89G/3.89G [09:18<00:00, 7.48MB/s]
Processing 11 items: 100%|███████████████████████████████████████████████████████████| 11.0/11.0 [09:24<00:00, 51.3s/it]
可以看到下载目录为/root/.cache/modelscope/hub/models/deepseek-ai/Janus-Pro-1B
把下载的模型移动到vllm目录里面
mv /root/.cache/modelscope/hub/models/deepseek-ai /home/xiao/vllm
四、测试图片理解
vllm目录有2个文件夹,结构如下:
# ll
total 20
drwxr-xr-x 4 root root 4096 May 8 18:59 ./
drwxr-x--- 5 xiao xiao 4096 May 8 14:50 ../
drwxr-xr-x 8 root root 4096 May 8 18:59 Janus/
drwxr-xr-x 4 root root 4096 May 8 16:01 deepseek-ai/
进入deepseek-ai目录,会看到一个文件夹Janus-Pro-7B
# ll
total 16
drwxr-xr-x 4 root root 4096 May 8 16:01 ./
drwxr-xr-x 4 root root 4096 May 8 18:59 ../
drwxr-xr-x 2 root root 4096 May 7 18:32 Janus-Pro-7B/
返回上一级,在Janus目录,创建image_understanding.py文件,代码如下:
import torch
from transformers import AutoModelForCausalLM
from janus.models import MultiModalityCausalLM, VLChatProcessor
from janus.utils.io import load_pil_images
model_path = "../deepseek-ai/Janus-Pro-7B"
image='aa.jpeg' question='请说明一下这张图片' vl_chat_processor: VLChatProcessor = VLChatProcessor.from_pretrained(model_path) tokenizer = vl_chat_processor.tokenizer vl_gpt: MultiModalityCausalLM = AutoModelForCausalLM.from_pretrained( model_path, trust_remote_code=True ) vl_gpt = vl_gpt.to(torch.bfloat16).cuda().eval() conversation = [ { "role": "<|User|>", "content": f"<image_placeholder>\n{question}", "images": [image], }, {"role": "<|Assistant|>", "content": ""}, ] # load images and prepare for inputs pil_images = load_pil_images(conversation) prepare_inputs = vl_chat_processor( conversations=conversation, images=pil_images, force_batchify=True ).to(vl_gpt.device) # # run image encoder to get the image embeddings inputs_embeds = vl_gpt.prepare_inputs_embeds(**prepare_inputs) # # run the model to get the response outputs = vl_gpt.language_model.generate( inputs_embeds=inputs_embeds, attention_mask=prepare_inputs.attention_mask, pad_token_id=tokenizer.eos_token_id, bos_token_id=tokenizer.bos_token_id, eos_token_id=tokenizer.eos_token_id, max_new_tokens=512, do_sample=False, use_cache=True, ) answer = tokenizer.decode(outputs[0].cpu().tolist(), skip_special_tokens=True) print(f"{prepare_inputs['sft_format'][0]}", answer)
下载一张图片,地址:https://pics6.baidu.com/feed/09fa513d269759ee74c8d049640fcc1b6f22df9e.jpeg
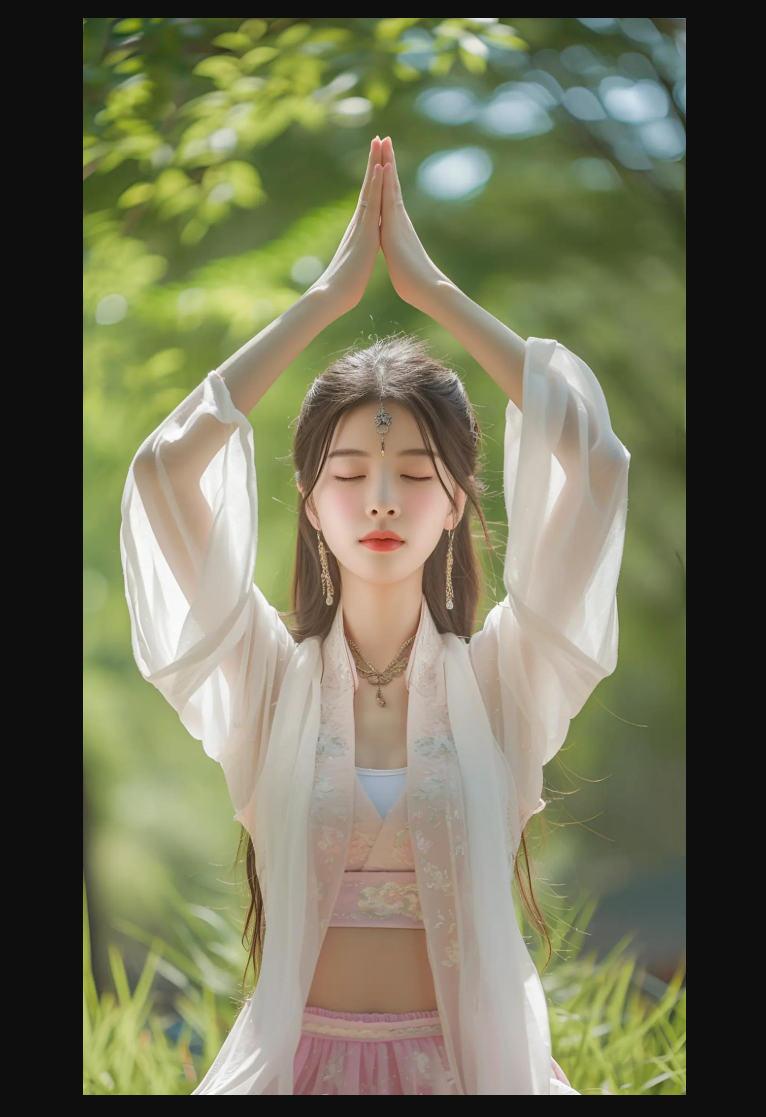
将此图片,重命名为aa.jpeg,存放在Janus目录
最终Janus目录,文件如下:
# ll
total 2976
drwxr-xr-x 8 root root 4096 May 8 18:59 ./
drwxr-xr-x 4 root root 4096 May 8 18:59 ../
drwxr-xr-x 8 root root 4096 May 7 18:11 .git/
-rw-r--r-- 1 root root 115 May 7 18:11 .gitattributes
-rw-r--r-- 1 root root 7301 May 7 18:11 .gitignore -rw-r--r-- 1 root root 1065 May 7 18:11 LICENSE-CODE -rw-r--r-- 1 root root 13718 May 7 18:11 LICENSE-MODEL -rw-r--r-- 1 root root 3069 May 7 18:11 Makefile -rwxr-xr-x 1 root root 26781 May 7 18:11 README.md* -rw-r--r-- 1 root root 62816 May 8 14:59 aa.jpeg drwxr-xr-x 2 root root 4096 May 7 18:11 demo/ drwxr-xr-x 2 root root 4096 May 8 17:19 generated_samples/ -rw-r--r-- 1 root root 4515 May 7 18:11 generation_inference.py -rw-r--r-- 1 xiao xiao 4066 May 8 18:50 image_generation.py -rw-r--r-- 1 root root 1594 May 8 18:58 image_understanding.py drwxr-xr-x 2 root root 4096 May 7 18:11 images/ -rw-r--r-- 1 root root 2642 May 7 18:11 inference.py -rw-r--r-- 1 root root 5188 May 7 18:11 interactivechat.py drwxr-xr-x 6 root root 4096 May 7 19:01 janus/ drwxr-xr-x 2 root root 4096 May 7 18:11 janus.egg-info/ -rw-r--r-- 1 root root 2846268 May 7 18:11 janus_pro_tech_report.pdf -rw-r--r-- 1 root root 1111 May 7 18:11 pyproject.toml -rw-r--r-- 1 root root 278 May 7 18:11 requirements.txt
运行代码,效果如下:
# python image_understanding.py
Python version is above 3.10, patching the collections module.
/root/anaconda3/envs/vll-Janus-Pro-7B/lib/python3.12/site-packages/transformers/models/auto/image_processing_auto.py:604: FutureWarning: The image_processor_class argument is deprecated and will be removed in v4.42. Please use `slow_image_processor_class`, or `fast_image_processor_class` instead
warnings.warn(
Using a slow image processor as `use_fast` is unset and a slow processor was saved with this model. `use_fast=True` will be the default behavior in v4.52, even if the model was saved with a slow processor. This will result in minor differences in outputs. You'll still be able to use a slow processor with `use_fast=False`.
You are using the default legacy behaviour of the <class 'transformers.models.llama.tokenization_llama_fast.LlamaTokenizerFast'>. This is expected, and simply means that the `legacy` (previous) behavior will be used so nothing changes for you. If you want to use the new behaviour, set `legacy=False`. This should only be set if you understand what it means, and thoroughly read the reason why this was added as explained in https://github.com/huggingface/transformers/pull/24565 - if you loaded a llama tokenizer from a GGUF file you can ignore this message.
Loading checkpoint shards: 100%|██████████████████████████████████████████████████████████| 2/2 [00:10<00:00, 5.18s/it]
You are a helpful language and vision assistant. You are able to understand the visual content that the user provides, and assist the user with a variety of tasks using natural language.
<|User|>: <image_placeholder>
请说明一下这张图片
<|Assistant|>: 这张图片展示了一位身穿传统服饰的女性,她正坐在户外,双手合十,闭着眼睛,似乎在进行冥想或祈祷。背景是绿色的树木和植物,阳光透过树叶洒在她的身上,营造出一种宁静、祥和的氛围。她的服装以淡雅的白色和粉色为主,带有精致的花纹,整体风格非常优雅。
描述还是比较准确的
五、测试图片生成
在Janus目录,新建image_generation.py脚本,代码如下:
import os
import torch
import numpy as np
from PIL import Image
from transformers import AutoModelForCausalLM
from janus.models import MultiModalityCausalLM, VLChatProcessor
model_path = "../deepseek-ai/Janus-Pro-7B"
vl_chat_processor: VLChatProcessor = VLChatProcessor.from_pretrained(model_path)
tokenizer = vl_chat_processor.tokenizer
vl_gpt: MultiModalityCausalLM = AutoModelForCausalLM.from_pretrained(
model_path, trust_remote_code=True
)
vl_gpt = vl_gpt.to(torch.bfloat16).cuda().eval()
conversation = [
{"role": "<|User|>", "content": "超写实8K渲染,一位具有东方古典美的中国女性,瓜子脸,西昌的眉毛如弯弯的月牙,双眼明亮而深邃,犹如夜空中闪烁的星星。高挺的鼻梁,樱桃小嘴微微上扬,透露出一丝诱人的微笑。她的头发如黑色的瀑布般垂直落在减胖两侧,微风轻轻浮动发色。肌肤白皙如雪,在阳光下泛着微微的光泽。她身着乙烯白色的透薄如纱的连衣裙,裙摆在海风中轻轻飘动。"},
{"role": "<|Assistant|>", "content": ""},
]
sft_format = vl_chat_processor.apply_sft_template_for_multi_turn_prompts(
conversations=conversation,
sft_format=vl_chat_processor.sft_format,
system_prompt=""
)
prompt = sft_format + vl_chat_processor.image_start_tag
@torch.inference_mode()
def generate(
mmgpt: MultiModalityCausalLM,
vl_chat_processor: VLChatProcessor,
prompt: str,
temperature: float = 1,
parallel_size: int = 1, # 减小 parallel_size
cfg_weight: float = 5,
image_token_num_per_image: int = 576,
img_size: int = 384,
patch_size: int = 16,
):
input_ids = vl_chat_processor.tokenizer.encode(prompt)
input_ids = torch.LongTensor(input_ids)
tokens = torch.zeros((parallel_size * 2, len(input_ids)), dtype=torch.int).cuda()
for i in range(parallel_size * 2):
tokens[i, :] = input_ids
if i % 2 != 0:
tokens[i, 1:-1] = vl_chat_processor.pad_id
inputs_embeds = mmgpt.language_model.get_input_embeddings()(tokens)
generated_tokens = torch.zeros((parallel_size, image_token_num_per_image), dtype=torch.int).cuda()
for i in range(image_token_num_per_image):
outputs = mmgpt.language_model.model(inputs_embeds=inputs_embeds, use_cache=True,
past_key_values=outputs.past_key_values if i != 0 else None)
hidden_states = outputs.last_hidden_state
logits = mmgpt.gen_head(hidden_states[:, -1, :])
logit_cond = logits[0::2, :]
logit_uncond = logits[1::2, :]
logits = logit_uncond + cfg_weight * (logit_cond - logit_uncond)
probs = torch.softmax(logits / temperature, dim=-1)
next_token = torch.multinomial(probs, num_samples=1)
generated_tokens[:, i] = next_token.squeeze(dim=-1)
next_token = torch.cat([next_token.unsqueeze(dim=1),
next_token.unsqueeze(dim=1)], dim=1).view(-1)
img_embeds = mmgpt.prepare_gen_img_embeds(next_token)
inputs_embeds = img_embeds.unsqueeze(dim=1)
# 添加显存清理
del logits, logit_cond, logit_uncond, probs
torch.cuda.empty_cache()
dec = mmgpt.gen_vision_model.decode_code(generated_tokens.to(dtype=torch.int),
shape=[parallel_size, 8, img_size // patch_size, img_size // patch_size])
dec = dec.to(torch.float32).cpu().numpy().transpose(0, 2, 3, 1)
dec = np.clip((dec + 1) / 2 * 255, 0, 255)
visual_img = np.zeros((parallel_size, img_size, img_size, 3), dtype=np.uint8)
visual_img[:, :, :] = dec
os.makedirs('generated_samples', exist_ok=True)
for i in range(parallel_size):
save_path = os.path.join('generated_samples', f"img_{i}.jpg")
img = Image.fromarray(visual_img[i])
img.save(save_path)
generate(
vl_gpt,
vl_chat_processor,
prompt,
)
注意:提示词是可以写中文的,不一定非要是英文。
运行代码,效果如下:
# python image_generation.py
Python version is above 3.10, patching the collections module.
/root/anaconda3/envs/vll-Janus-Pro-7B/lib/python3.12/site-packages/transformers/models/auto/image_processing_auto.py:604: FutureWarning: The image_processor_class argument is deprecated and will be removed in v4.42. Please use `slow_image_processor_class`, or `fast_image_processor_class` instead
warnings.warn(
Using a slow image processor as `use_fast` is unset and a slow processor was saved with this model. `use_fast=True` will be the default behavior in v4.52, even if the model was saved with a slow processor. This will result in minor differences in outputs. You'll still be able to use a slow processor with `use_fast=False`.
You are using the default legacy behaviour of the <class 'transformers.models.llama.tokenization_llama_fast.LlamaTokenizerFast'>. This is expected, and simply means that the `legacy` (previous) behavior will be used so nothing changes for you. If you want to use the new behaviour, set `legacy=False`. This should only be set if you understand what it means, and thoroughly read the reason why this was added as explained in https://github.com/huggingface/transformers/pull/24565 - if you loaded a llama tokenizer from a GGUF file you can ignore this message.
Loading checkpoint shards: 100%|██████████████████████████████████████████████████████████| 1/1 [00:09<00:00, 4.58s/it]
注意观察一下GPU使用情况,这里会很高。
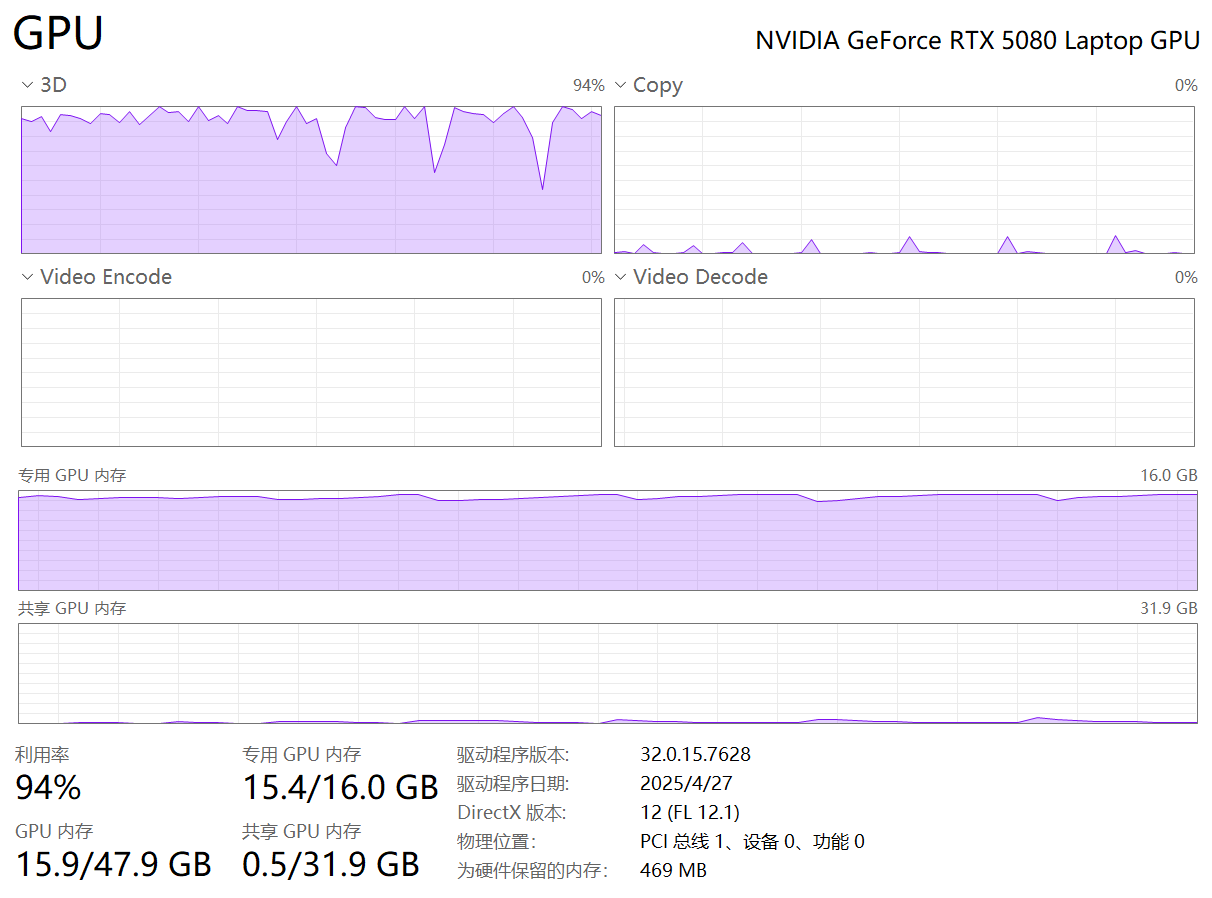
RTX 5080显卡,16GB显存,几乎已经占满了。
等待30秒左右,就会生成一张图片。
打开小企鹅,进入目录\home\xiao\vllm\Janus\generated_samples
这里会出现一张图片
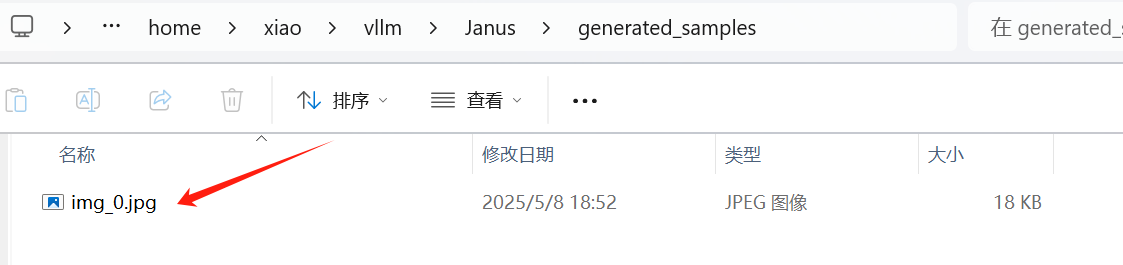
打开图片,效果如下:
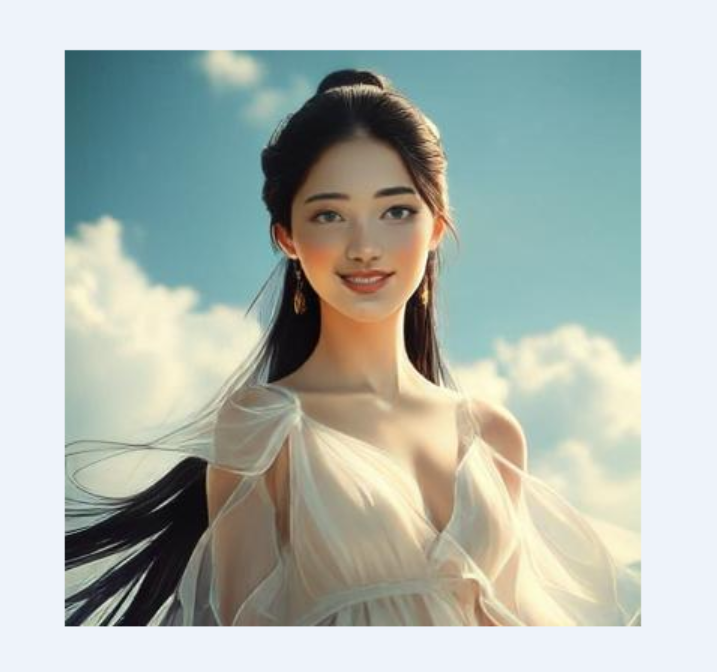
效果还算可以。