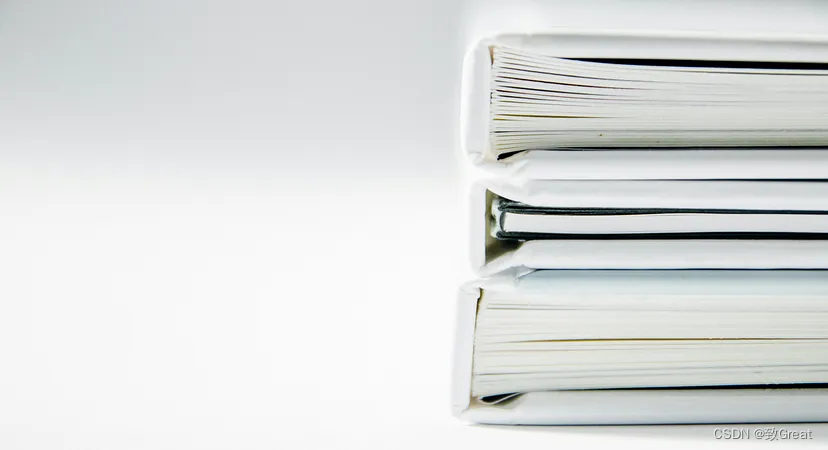
Langchain 使用文档加载器从各种来源获取信息并准备处理。这些加载器充当数据连接器,获取信息并将其转换为 Langchain 可以理解的格式。
LangChain 中有几十个文档加载器,可以在这查看https://python.langchain.com/v0.2/docs/integrations/document_loaders/
但是实际使用过程中,这些解析的效果层次补齐,需要结合自己的文件去写如何加载具体文档。这个也是在后续开发框架的过程中,我们可以选取langchian的document作为处理对象,但是文件解析需要自己去写和实现。
在本章中,我们将介绍其中的一些:
- TextLoader
- CSVLoader
- UnstructuredFileLoader
- DirectoryLoader
- UnstructuredHTMLLoader
- JSONLoader
- PyPDFLoader
- ArxivLoader
- Docx2txtLoader
TextLoader
from langchain_community.document_loaders import TextLoader
loader = TextLoader("text.txt")
loader.load()
"""
[Document(page_content='I have some instructions here.\nThis is the second row.', metadata={'source': 'text.txt'})]
"""
loader = TextLoader("index.md")
loader.load()
"""
[Document(page_content='some instructions\n', metadata={'source': 'index.md'})]
"""
CSVLoader
import pandas as pd
# Create a simple DataFrame
data = {
'Name': ['Alice', 'Bob', 'Charlie'],
'Age': [25, 30, 35],
'City': ['New York', 'Los Angeles', 'Chicago']
}
df = pd.DataFrame(data)
# Export the DataFrame to a CSV file
csv_file_path = 'sample_data.csv'
df.to_csv(csv_file_path, index=False)
from langchain_community.document_loaders.csv_loader import CSVLoader
loader = CSVLoader(file_path='sample_data.csv')
data = loader.load()
data
"""
[Document(page_content='Name: Alice\nAge: 25\nCity: New York', metadata={'source': 'sample_data.csv', 'row': 0}),
Document(page_content='Name: Bob\nAge: 30\nCity: Los Angeles', metadata={'source': 'sample_data.csv', 'row': 1}),
Document(page_content='Name: Charlie\nAge: 35\nCity: Chicago', metadata={'source': 'sample_data.csv', 'row': 2})]
"""
如有必要,我们可以在读取文件时自定义 CSV 参数:
loader = CSVLoader(file_path='sample_data.csv', csv_args={
'delimiter': ',',
'quotechar': '"',
'fieldnames': ['Name', 'Age', 'City']
})
data = loader.load()
data
# now the headers are also a row.
"""
[Document(page_content='Name: Name\nAge: Age\nCity: City', metadata={'source': 'sample_data.csv', 'row': 0}),
Document(page_content='Name: Alice\nAge: 25\nCity: New York', metadata={'source': 'sample_data.csv', 'row': 1}),
Document(page_content='Name: Bob\nAge: 30\nCity: Los Angeles', metadata={'source': 'sample_data.csv', 'row': 2}),
Document(page_content='Name: Charlie\nAge: 35\nCity: Chicago', metadata={'source': 'sample_data.csv', 'row': 3})]
"""
当从 CSV 文件加载数据时,加载器通常会为 CSV 中的每一行数据创建一个单独的"文档"对象。
默认情况下,每个文档的来源都设置为 CSV 本身的整个文件路径。如果想跟踪 CSV 中每条信息的来源,这可能并不理想。
可以使用 source_column 指定 CSV 文件中的列名。然后,每行特定列中的值将用作从该行创建的相应文档的单独来源
loader = CSVLoader(file_path='sample_data.csv', source_column="Name")
data = loader.load()
data
"""
[Document(page_content='Name: Alice\nAge: 25\nCity: New York',
metadata={'source': 'Alice', 'row': 0}),
Document(page_content='Name: Bob\nAge: 30\nCity: Los Angeles',
metadata={'source': 'Bob', 'row': 1}),
Document(page_content='Name: Charlie\nAge: 35\nCity: Chicago',
metadata={'source': 'Charlie', 'row': 2})]
"""
这在使用涉及根据信息来源回答问题的"链"(可能是数据处理管道)时特别有用。通过为每个文档提供单独的源信息,这些链可以在处理时考虑数据的来源,并可能提供更细致入微或更可靠的答案。
UnstructuredCSVLoader
与CSVLoader
不同,CSVLoader
将每一行视为一个单独的文档,并使用标题定义数据,而在 UnstructuredCSVLoader
中,整个 CSV 文件被视为单个"非结构化表"元素。当您想要将数据作为整个表而不是单个条目进行分析时,这很有用。
from langchain_community.document_loaders.csv_loader import UnstructuredCSVLoader
loader = UnstructuredCSVLoader(
file_path="sample_data.csv", mode="elements"
)
docs = loader.load()
docs
"""
[Document(page_content='\n\n\nName\nAge\nCity\n\n\nAlice\n25\nNew York\n\n\nBob\n30\nLos Angeles\n\n\nCharlie\n35\nChicago\n\n\n', metadata={'source': 'sample_data.csv', 'filename': 'sample_data.csv', 'languages': ['eng'], 'last_modified': '2024-03-04T18:05:41', 'text_as_html': '<table border="1" class="dataframe">\n <tbody>\n <tr>\n <td>Name</td>\n <td>Age</td>\n <td>City</td>\n </tr>\n <tr>\n <td>Alice</td>\n <td>25</td>\n <td>New York</td>\n </tr>\n <tr>\n <td>Bob</td>\n <td>30</td>\n <td>Los Angeles</td>\n </tr>\n <tr>\n <td>Charlie</td>\n <td>35</td>\n <td>Chicago</td>\n </tr>\n </tbody>\n</table>', 'filetype': 'text/csv', 'category': 'Table'})]
"""
如果在"元素"模式下操作,则表的 HTML 表示将可在元数据中访问。
print(docs[0].metadata["text_as_html"])
"""
<table border="1" class="dataframe">
<tbody>
<tr>
<td>Name</td>
<td>Age</td>
<td>City</td>
</tr>
<tr>
<td>Alice</td>
<td>25</td>
<td>New York</td>
</tr>
<tr>
<td>Bob</td>
<td>30</td>
<td>Los Angeles</td>
</tr>
<tr>
<td>Charlie</td>
<td>35</td>
<td>Chicago</td>
</tr>
</tbody>
</table>
"""
UnstructuredFileLoader
与 TextLoader
等专为特定格式设计的加载器不同,UnstructuredFileLoader
会自动检测您提供的文件类型。
加载器利用了底层的"unstructured"库。该库会分析文件内容并尝试根据文件类型提取有意义的信息。
from langchain_community.document_loaders import UnstructuredFileLoader
loader = UnstructuredFileLoader("text.txt")
docs = loader.load()
docs
"""
[Document(page_content='I have some instructions here.\n\nThis is the second row.', metadata={'source': 'text.txt'})]
"""
loader = UnstructuredFileLoader(
"text.txt", mode="elements"
)
docs = loader.load()
docs
"""
[Document(page_content='I have some instructions here.', metadata={'source': 'text.txt', 'filename': 'text.txt', 'last_modified': '2024-03-04T18:15:12', 'languages': ['eng'], 'filetype': 'text/plain', 'category': 'NarrativeText'}),
Document(page_content='This is the second row.', metadata={'source': 'text.txt', 'filename': 'text.txt', 'last_modified': '2024-03-04T18:15:12', 'languages': ['eng'], 'filetype': 'text/plain', 'category': 'NarrativeText'})]
"""
loader = UnstructuredFileLoader("your_report.html")
docs = loader.load()
docs
"""
[Document(page_content='Toggle navigation\n\nPandas Profiling Report\n\nOverview\n\nVariables\n\nInteractions\n\nCorrelations\n\nMissing values\n\nSample\n\nOverview\n\nOverview\n\nAlerts 44\n\nReproduction\n\nDataset statistics\n\nNumber of variables 44 Number of observations 58592 Missing cells 0 Missing cells (%) 0.0% Duplicate rows 0 Duplicate rows (%) 0.0% Total size in memory 19.7 MiB Average record size in memory 352.0 B\n\nVariable types\n\nText 1 Numeric 10 Categorical 16 Boolean 17\n\nairbags is highly overall correlated with cylinder and 28 other fields High correlation cylinder is highly overall correlated with airbags and 22 other fields High correlation displacement is highly overall correlated with airbags and 33 other fields High correlation engine_type is highly overall correlated with airbags and 30 other fields High correlation fuel_type is highly overall correlated with airbags and 30 other fields High correlation gear_box is highly overall correlated with airbags and 23 other fields High correlation gross_weight is highly overall correlated with airbags and 32 other fields High correlation height is highly overall correla
"""
# pip install "unstructured[pdf]"
loader = UnstructuredFileLoader("ticket.pdf")
docs = loader.load()
docs
"""
[Document(page_content='Event\n\nCommence Date\n\nReference\n\nPaul Kalkbrenner\n\n10 September,Satu
info@biletino.com', metadata={'source': 'ticket.pdf'})]
"""
DirectoryLoader
DirectoryLoader
可帮助一次性从整个目录加载多个文档。它利用了 UnstructuredFileLoader
。
from langchain_community.document_loaders import DirectoryLoader
loader = DirectoryLoader('folder/')
docs = loader.load()
print(len(docs)) # 3
# we can declare extension, display progress bar, use multithreading
loader = DirectoryLoader('folder/', glob="*.txt", show_progress=True, use_multithreading=True)
docs = loader.load()
print(len(docs)) # 1
UnstructuredHTMLLoader
它利用"非结构化"库的功能从存储为 HTML 文件的网页中提取有意义的内容。
<!DOCTYPE html>
<html lang="en">
<head>
<meta charset="UTF-8" />
<meta name="viewport" content="width=device-width, initial-scale=1.0" />
<title>Document</title>
</head>
<body>
<div>A div element</div>
<p>a p element</p>
<div>
<p>a p inside of a div</p>
</div>
</body>
</html
from langchain_community.document_loaders import UnstructuredHTMLLoader
loader = UnstructuredHTMLLoader("index.html")
data = loader.load()
data
"""
[Document(page_content='A div element\n\na p element\n\na p inside of a div', metadata={'source': 'index.html'})]
"""
我们可以使用BeautifulSoup4
通过BSHTMLLoader
来解析 HTML 文档。
from langchain_community.document_loaders import BSHTMLLoader
loader = BSHTMLLoader("index.html")
data = loader.load()
data
"""
[Document(page_content='\n\n\n\nDocument\n\n\nA div element\na p element\n\na p inside of a div\n\n\n\n', metadata={'source': 'index.html', 'title': 'Document'})]
"""
JSONLoader
JSONLoader 被设计用于处理以 JSON 形式存储的数据。
[
{
"id": 1,
"name": "John Doe",
"email": "john.doe@example.com",
"age": 30,
"city": "New York"
},
{
"id": 2,
"name": "Jane Smith",
"email": "jane.smith@example.com",
"age": 25,
"city": "Los Angeles"
},
{
"id": 3,
"name": "Alice Johnson",
"email": "alice.johnson@example.com",
"age": 28,
"city": "Chicago"
}
]
JSONLoaders
利用 JQ 库来解析 JSON 数据。JQ 提供了一种专为处理 JSON 结构而设计的强大查询语言。
jq_schema
参数允许在 JSONLoader 函数中提供 JQ 表达式。
from langchain_community.document_loaders import JSONLoader
loader = JSONLoader(
file_path='example.json',
jq_schema='map({ name, email })',
text_content=False)
data = loader.load()
data
"""
[Document(page_content="[{'name': 'John Doe', 'email': 'john.doe@example.com'},
{'name': 'Jane Smith', 'email': 'jane.smith@example.com'}, {'name': 'Alice Johnson', 'email': 'alice.johnson@example.com'}]", metadata={'source': '/Users/okanyenigun/Desktop/codes/python__general/example.json', 'seq_num': 1})]
"""
JSON 行文件是一个文本文件,其中每行都是一个有效的 JSON 对象,由换行符分隔。
{"name": "John Doe", "age": 30}
{"name": "Jane Smith", "age": 25}
{"name": "Alice Johnson", "age": 28}
loader = JSONLoader(
file_path='example.jsonl',
jq_schema='.content',
text_content=False,
json_lines=True)
data = loader.load()
from pprint import pprint
pprint(data)
"""
[Document(page_content='', metadata={'source': '/Users/okanyenigun/Desktop/codes/python__general/example.jsonl', 'seq_num': 1}),
Document(page_content='', metadata={'source': '/Users/okanyenigun/Desktop/codes/python__general/example.jsonl', 'seq_num': 2}),
Document(page_content='', metadata={'source': '/Users/okanyenigun/Desktop/codes/python__general/example.jsonl', 'seq_num': 3})]
"""
PyPDFLoader
它利用 pypdf 库来加载 PDF 文件。
from langchain_community.document_loaders import PyPDFLoader
loader = PyPDFLoader("ticket.pdf")
pages = loader.load_and_split()
pages[0]
"""
Document(page_content='Paul Kalkbrenner\nThis electronically generated document will grant you entry to the event and time specified on this ticket. The security of the ticket belongs to the\nowner
...
Sarıyer, İstanbul', metadata={'source': 'ticket.pdf', 'page': 0})
"""
我们还可以使用 UnstructuredPDFLoader 来加载 PDF。
from langchain_community.document_loaders import UnstructuredPDFLoader
loader = UnstructuredPDFLoader("ticket.pdf")
data = loader.load()
我们有 OnlinePDFLoader 来加载在线 PDF。
from langchain_community.document_loaders import OnlinePDFLoader
loader = OnlinePDFLoader("https://arxiv.org/pdf/2302.03803.pdf")
data = loader.load()
data
"""
[Document(page_content='3 2 0 2\n\nb e F 7\n\n]\n\nG A . h t a m\n\n[\n\n1 v 3 0 8 3 0 . 2 0 3 2 : v i X r a\n\nA WEAK (k, k)-LEFSCHETZ THEOREM FOR PROJECTIVE TORIC ORBI...
"""
还有更多利用不同来源的......
# PyPDFium2Loader
from langchain_community.document_loaders import PyPDFium2Loader
loader = PyPDFium2Loader("ticket.pdf")
data = loader.load()
# PDFMinerLoader
from langchain_community.document_loaders import PDFMinerLoader
loader = PDFMinerLoader("ticket.pdf")
data = loader.load()
# PDFMinerPDFasHTMLLoader
from langchain_community.document_loaders import PDFMinerPDFasHTMLLoader
loader = PDFMinerPDFasHTMLLoader("ticket.pdf")
data = loader.load()[0] # entire PDF is loaded as a single Document
# PyMuPDFLoader
from langchain_community.document_loaders import PyMuPDFLoader
loader = PyMuPDFLoader("ticket.pdf")
data = loader.load()
# Directory loader for PDF
from langchain_community.document_loaders import PyPDFDirectoryLoader
loader = PyPDFDirectoryLoader("folder/")
docs = loader.load()
ArxivLoader
它旨在从 arXiv 开放存取库中获取和处理文档。
# pip install arxiv
from langchain_community.document_loaders import ArxivLoader
docs = ArxivLoader(query="1605.08386", load_max_docs=2).load()
print(len(docs))
print()
print(docs[0].metadata)
"""
1
{'Published': '2016-05-26', 'Title': 'Heat-bath random walks with Markov
bases', 'Authors': 'Caprice Stanley, Tobias Windisch', 'Summary':
'Graphs on lattice points are studied whose edges come from a finite set of\nallowed moves of arbitrary length. We show that the diameter of these graphs on\nfibers of a fixed integer matrix can be bounded from above by a constant. We\nthen study the mixing behaviour of heat-bath random walks on these graphs. We\nalso state explicit conditions on the set of moves so that the heat-bath random\nwalk, a generalization of the Glauber dynamics, is an expander in fixed\ndimension.'}
"""
Docx2txtLoader
它适用于 Microsoft Office Word 文档。
from langchain_community.document_loaders import Docx2txtLoader
loader = Docx2txtLoader("example_data/fake.docx")
data = loader.load()
data
"""
[Document(page_content='Lorem ipsum dolor sit amet.',
metadata={'source': 'ex...
"""