要求
1、数据集stock-daily,包含A股近4000只股票的今年以来的日数据;数据集stock-daily-30d仅包含最近30个交易日数据,根据自己计算机性能选择。
数据来源:https://www.joinquant.com/help/api/help?name=JQData
2、数据集stock-concept,包含A股近4000只股票所有的股票代码、名称和概念。
数据来源:万德金融终端
根据此stock-daily数据集计算每只股票的5日滚动收益为正的概率,滚动收益:某个投资标的(如:股票、基金、黄金、期货、债券、房子等)在任意时刻进入后持有固定时间,如:5日(一周)、22日(一月)、44日(两月)、66日(一季度)、123日(半年)、245日(一年)等。后获取的收益,是描述某个投资标的赚钱概率的数学模型,也可用来衡量股票、基金、债券等证券的业绩。
3、滚动收益率计算方法:
(1) 忽略N/A所在日的股票数据,思考:可使用插值算法填充异常N/A数据,但退市股票同样会造成N/A数据,需要识别那种数据是退市造成的,而哪种数据是异常形成的。
(2)第t日的5日滚动收益
Rt= (C t - C t-5 ) / Ct-5 ,Ct:第t日收盘价 Rt:第t日滚动收益
(3) 5日滚动正收益率
所有交易日的5日滚动收益为正(赚钱)的概率
* 所有计算忽略非交易日(节假日)
数据集
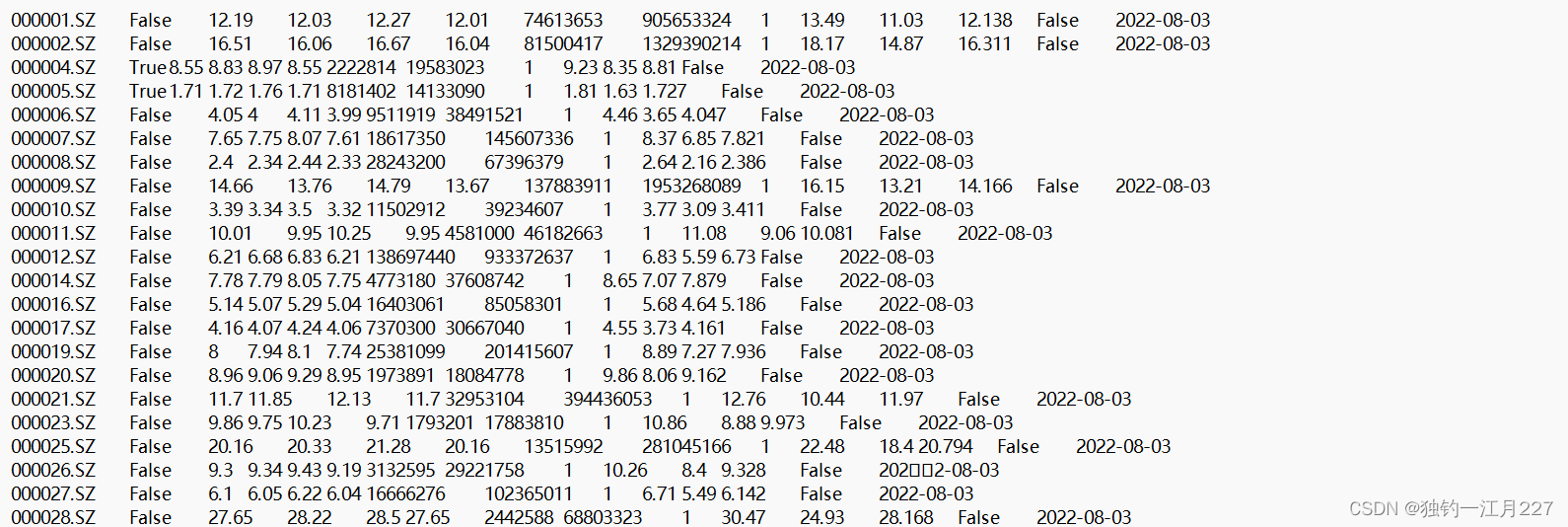
代码
配置pom文件和建包
XML
<?xml version="1.0" encoding="UTF-8"?>
<project xmlns="http://maven.apache.org/POM/4.0.0"
xmlns:xsi="http://www.w3.org/2001/XMLSchema-instance"
xsi:schemaLocation="http://maven.apache.org/POM/4.0.0 http://maven.apache.org/xsd/maven-4.0.0.xsd">
<modelVersion>4.0.0</modelVersion>
<groupId>org.example</groupId>
<artifactId>stock_daily</artifactId>
<version>1.0-SNAPSHOT</version>
<properties>
<maven.compiler.source>8</maven.compiler.source>
<maven.compiler.target>8</maven.compiler.target>
<project.build.sourceEncoding>UTF-8</project.build.sourceEncoding>
</properties>
<dependencies>
<!-- https://mvnrepository.com/artifact/org.apache.hadoop/hadoop-hdfs -->
<dependency>
<groupId>org.apache.hadoop</groupId>
<artifactId>hadoop-hdfs</artifactId>
<version>3.1.2</version>
<scope>test</scope>
</dependency>
<!-- https://mvnrepository.com/artifact/org.apache.hadoop/hadoop-client -->
<dependency>
<groupId>org.apache.hadoop</groupId>
<artifactId>hadoop-client</artifactId>
<version>3.1.2</version>
</dependency>
<!-- https://mvnrepository.com/artifact/org.apache.hadoop/hadoop-common -->
<dependency>
<groupId>org.apache.hadoop</groupId>
<artifactId>hadoop-common</artifactId>
<version>3.1.2</version>
</dependency>
<dependency>
<groupId>org.apache.commons</groupId>
<artifactId>commons-configuration2</artifactId>
<version>2.7</version>
</dependency>
<dependency>
<groupId>org.slf4j</groupId>
<artifactId>slf4j-api</artifactId>
<version>1.7.30</version>
</dependency>
<dependency>
<groupId>org.apache.hadoop</groupId>
<artifactId>hadoop-common</artifactId>
<version>3.1.2</version>
</dependency>
</dependencies>
</project>
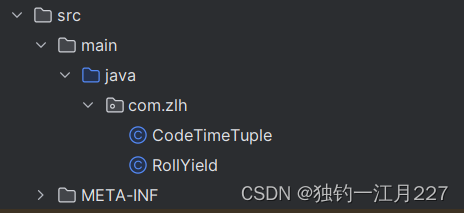
MapReduce 任务类
创建类继承 Configured 类实现 Tool 接口
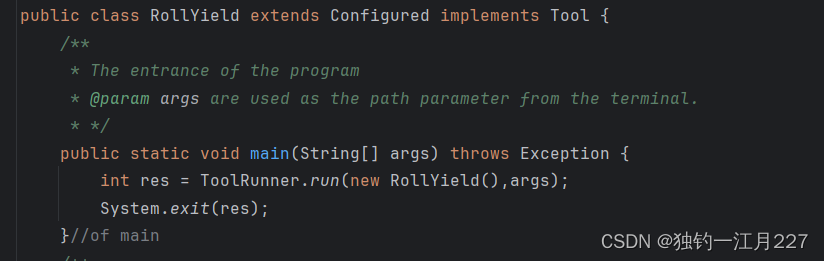
重写 run 方法,配置 mepreduce 的相关内容,指定 map , reduce , group 类。并启动 job 。
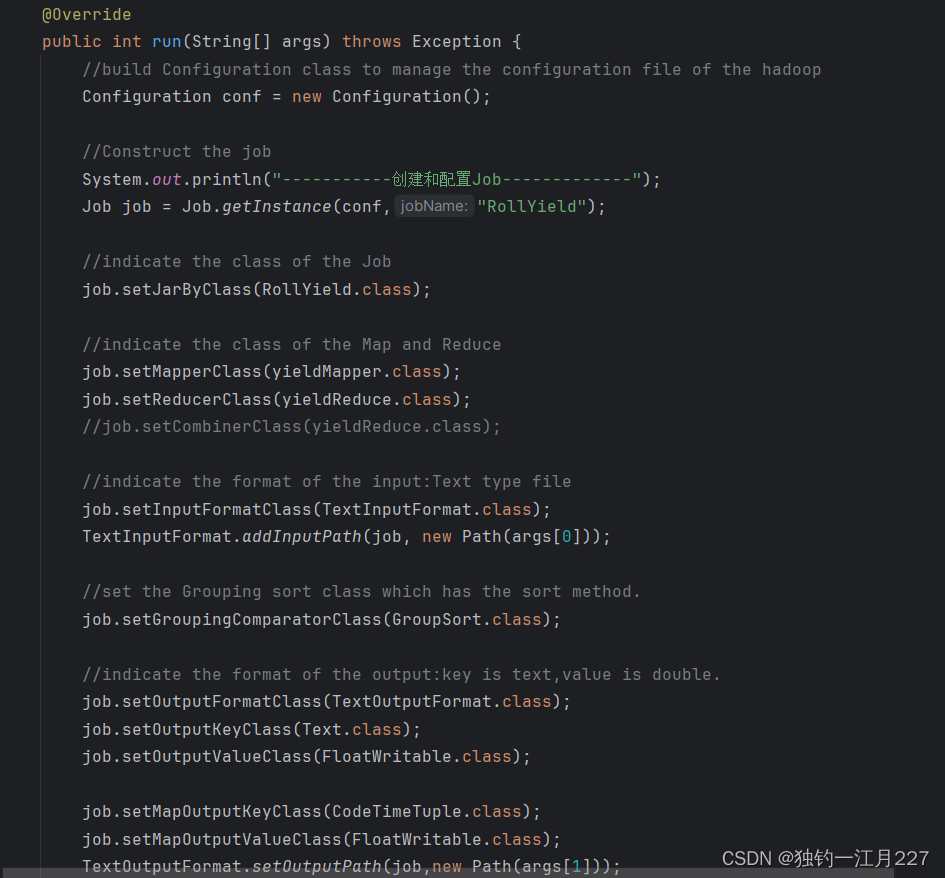
Map 类继承 mapper 类,指定输入和输出格式
基本代码逻辑为将输入的文件按行切割,然后按照元素位置取出对应的元素,将股票代码和时间封装到 CodeTimeTuple 类里面作为键,将收盘价作为值写入到 context 里面交给 reduce 类。这里日期转换用了 SipleDataFormat 类进行转换,设置格式为 yyyy-MM-dd HH : mm : ss 。
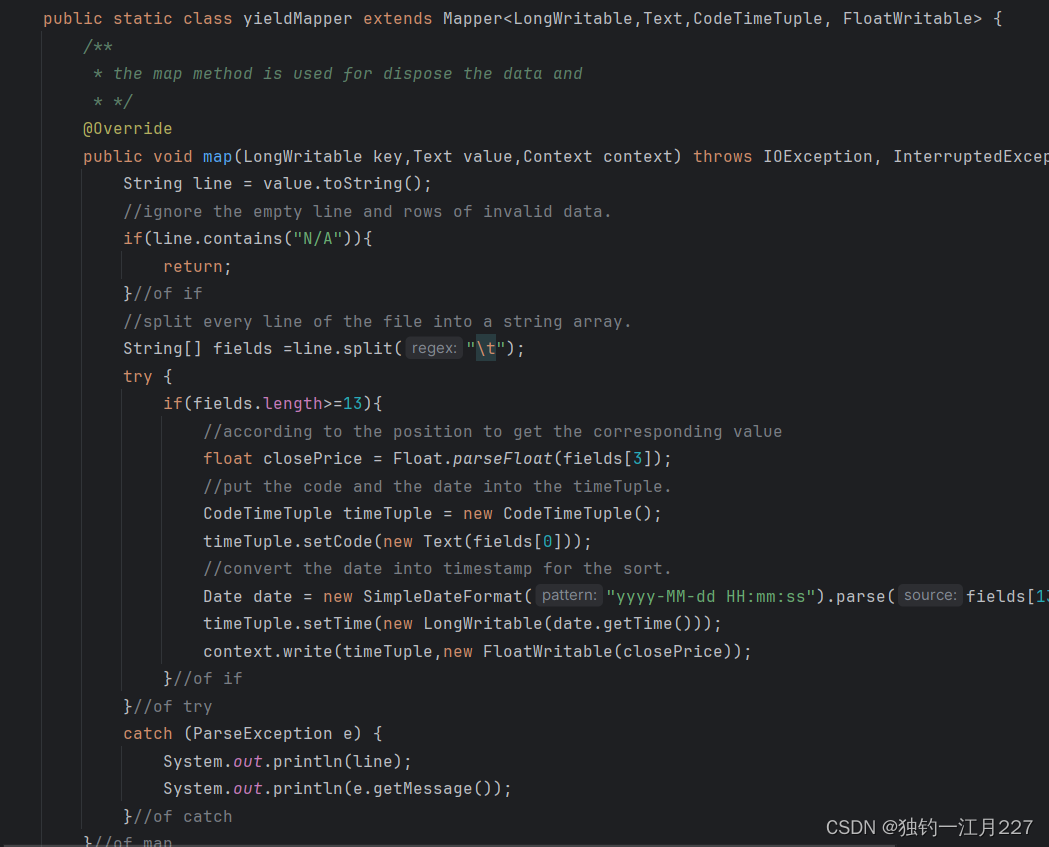
CodeTimeTuple 类
里面设置两个属性,一个是 time ,一个是 code 。这个类的读取和写入都要采用字节流的方式。在这里还要实现比较的方法以便实现后面的组排序,排序分为两次,一次是根据股票代码排序,如果股票代码相同再根据时间进行排序。
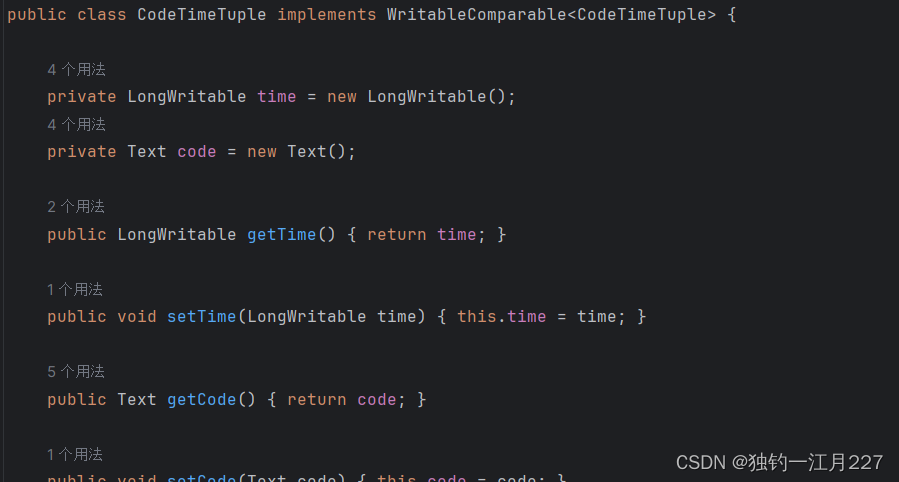
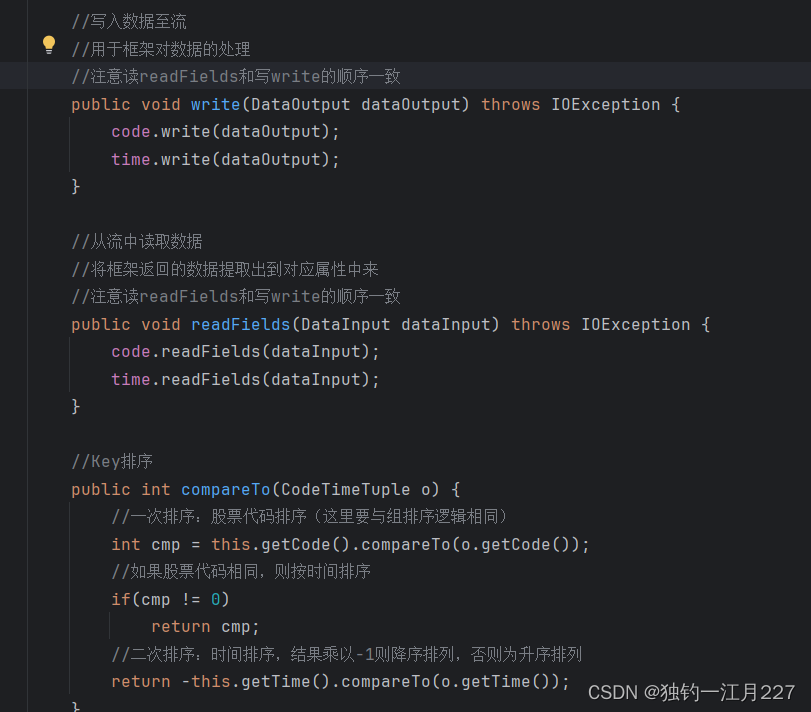
组排序类
将排序对象转化为 CodeTimeTuple 类调用排序方法
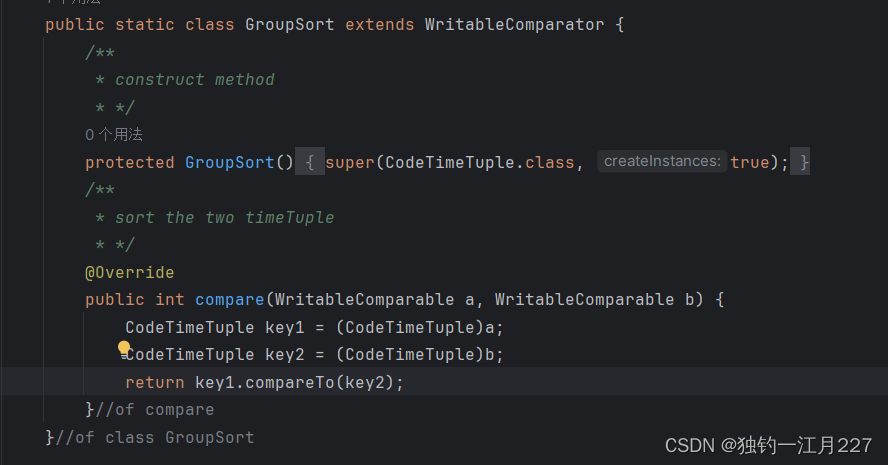
Reduce 类
将排完序的内容拉取过来,设置一个数组来存取每一天的收盘价(为后面计算滚动收益做准备),遍历容器,每只股票前四天的收盘价直接作为收益,后面的收盘价计算公式为Rt= (C t - C t-5 ) / Ct-5 ,Ct:第t日收盘价 Rt:第t日滚动收益。然后计算收益为正的概率。
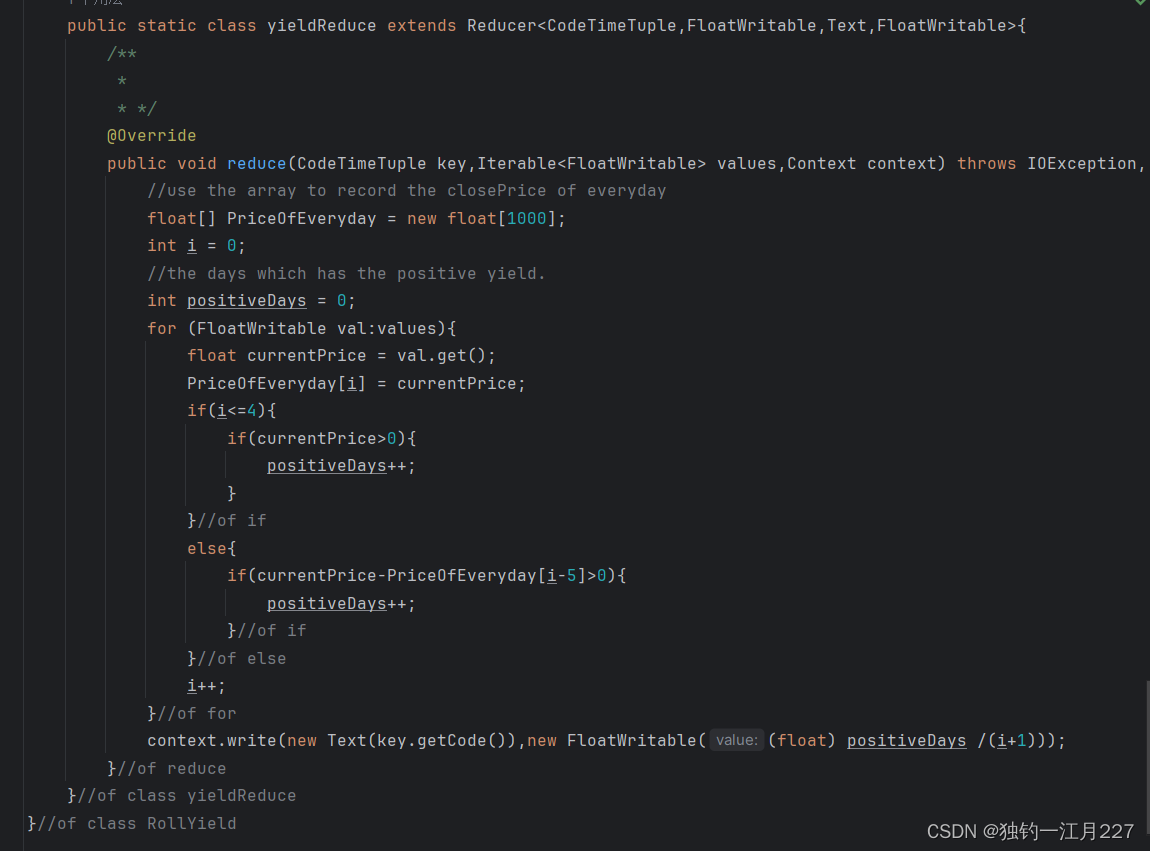
完整代码
java
import org.apache.hadoop.conf.Configuration;
import org.apache.hadoop.conf.Configured;
import org.apache.hadoop.fs.Path;
import org.apache.hadoop.io.*;
import org.apache.hadoop.mapreduce.Job;
import org.apache.hadoop.mapreduce.Mapper;
import org.apache.hadoop.mapreduce.Reducer;
import org.apache.hadoop.mapreduce.lib.input.TextInputFormat;
import org.apache.hadoop.mapreduce.lib.output.TextOutputFormat;
import org.apache.hadoop.util.Tool;
import org.apache.hadoop.util.ToolRunner;
import java.io.IOException;
import java.text.ParseException;
import java.text.SimpleDateFormat;
import java.util.Date;
/**
* Calculate the stock five days' roll yield.
* */
public class RollYield extends Configured implements Tool {
/**
* The entrance of the program
* @param args are used as the path parameter from the terminal.
* */
public static void main(String[] args) throws Exception {
int res = ToolRunner.run(new RollYield(),args);
System.exit(res);
}//of main
/**
* Set the map class and reduce class and construct the job.
* */
@Override
public int run(String[] args) throws Exception {
//build Configuration class to manage the configuration file of the hadoop
Configuration conf = new Configuration();
//Construct the job
System.out.println("-----------创建和配置Job-------------");
Job job = Job.getInstance(conf,"RollYield");
//indicate the class of the Job
job.setJarByClass(RollYield.class);
//indicate the class of the Map and Reduce
job.setMapperClass(yieldMapper.class);
job.setReducerClass(yieldReduce.class);
//job.setCombinerClass(yieldReduce.class);
//indicate the format of the input:Text type file
job.setInputFormatClass(TextInputFormat.class);
TextInputFormat.addInputPath(job, new Path(args[0]));
//set the Grouping sort class which has the sort method.
job.setGroupingComparatorClass(GroupSort.class);
//indicate the format of the output:key is text,value is double.
job.setOutputFormatClass(TextOutputFormat.class);
job.setOutputKeyClass(Text.class);
job.setOutputValueClass(FloatWritable.class);
job.setMapOutputKeyClass(CodeTimeTuple.class);
job.setMapOutputValueClass(FloatWritable.class);
TextOutputFormat.setOutputPath(job,new Path(args[1]));
//Execute the mapreduce
boolean res = job.waitForCompletion(true);
if(res){
return 0;
}//of if
else{
return -1;
}//of else
}//of run
/**
* The map class for converting the input data into key-value pair.
*/
public static class yieldMapper extends Mapper<LongWritable,Text,CodeTimeTuple, FloatWritable> {
/**
* the map method is used for dispose the data and
* */
@Override
public void map(LongWritable key,Text value,Context context) throws IOException, InterruptedException {
String line = value.toString();
//ignore the empty line and rows of invalid data.
if(line.contains("N/A")){
return;
}//of if
//split every line of the file into a string array.
String[] fields =line.split("\t");
try {
if(fields.length>=13){
//according to the position to get the corresponding value
float closePrice = Float.parseFloat(fields[3]);
//put the code and the date into the timeTuple.
CodeTimeTuple timeTuple = new CodeTimeTuple();
timeTuple.setCode(new Text(fields[0]));
//convert the date into timestamp for the sort.
Date date = new SimpleDateFormat("yyyy-MM-dd HH:mm:ss").parse(fields[13]+" 00:00:00");
timeTuple.setTime(new LongWritable(date.getTime()));
context.write(timeTuple,new FloatWritable(closePrice));
}//of if
}//of try
catch (ParseException e) {
System.out.println(line);
System.out.println(e.getMessage());
}//of catch
}//of map
}//of class
/**
*
*/
public static class GroupSort extends WritableComparator {
/**
* construct method
* */
protected GroupSort() {
super(CodeTimeTuple.class, true);
}
/**
* sort the two timeTuple
* */
@Override
public int compare(WritableComparable a, WritableComparable b) {
CodeTimeTuple key1 = (CodeTimeTuple)a;
CodeTimeTuple key2 = (CodeTimeTuple)b;
return key1.compareTo(key2);
}//of compare
}//of class GroupSort
/**
*
* */
public static class yieldReduce extends Reducer<CodeTimeTuple,FloatWritable,Text,FloatWritable>{
/**
*
* */
@Override
public void reduce(CodeTimeTuple key,Iterable<FloatWritable> values,Context context) throws IOException, InterruptedException {
//use the array to record the closePrice of everyday
float[] PriceOfEveryday = new float[1000];
int i = 0;
//the days which has the positive yield.
int positiveDays = 0;
for (FloatWritable val:values){
float currentPrice = val.get();
PriceOfEveryday[i] = currentPrice;
if(i<=4){
if(currentPrice>0){
positiveDays++;
}
}//of if
else{
if(currentPrice-PriceOfEveryday[i-5]>0){
positiveDays++;
}//of if
}//of else
i++;
}//of for
context.write(new Text(key.getCode()),new FloatWritable((float) positiveDays /(i+1)));
}//of reduce
}//of class yieldReduce
}//of class RollYield
java
import org.apache.hadoop.io.LongWritable;
import org.apache.hadoop.io.Text;
import org.apache.hadoop.io.WritableComparable;
import java.io.DataInput;
import java.io.DataOutput;
import java.io.IOException;
//自定义Tuple
public class CodeTimeTuple implements WritableComparable<CodeTimeTuple> {
private LongWritable time = new LongWritable();
private Text code = new Text();
public LongWritable getTime() { return time; }
public void setTime(LongWritable time) { this.time = time; }
public Text getCode() { return code; }
public void setCode(Text code) { this.code = code; }
//写入数据至流
//用于框架对数据的处理
//注意读readFields和写write的顺序一致
public void write(DataOutput dataOutput) throws IOException {
code.write(dataOutput);
time.write(dataOutput);
}
//从流中读取数据
//将框架返回的数据提取出到对应属性中来
//注意读readFields和写write的顺序一致
public void readFields(DataInput dataInput) throws IOException {
code.readFields(dataInput);
time.readFields(dataInput);
}
//Key排序
public int compareTo(CodeTimeTuple o) {
//一次排序:股票代码排序(这里要与组排序逻辑相同)
int cmp = this.getCode().compareTo(o.getCode());
//如果股票代码相同,则按时间排序
if(cmp != 0)
return cmp;
//二次排序:时间排序,结果乘以-1则降序排列,否则为升序排列
return -this.getTime().compareTo(o.getTime());
}
}
创建 jar 包和打包上传到 hadoop 上
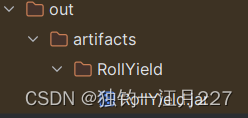

启动 job
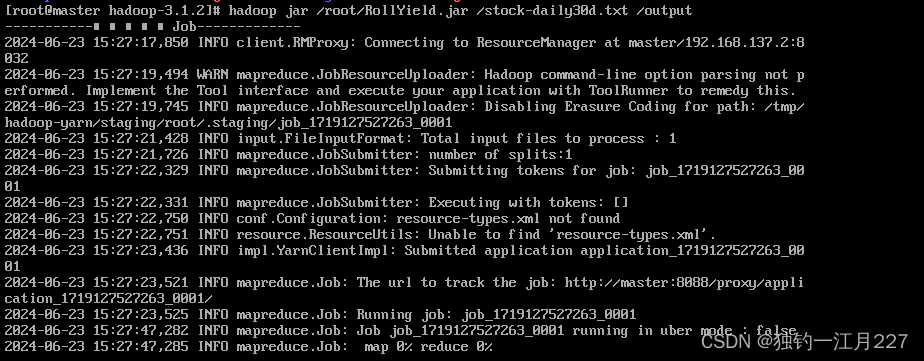
ps:
由于启用combine必须要求reduce的输出跟输入类型相对应,但是这里的reduce输出和输入类型不一样,所以要么重写一个combine类,要么直接不使用combine类,我选择的第二种,所以combine没有输入输出,但是会加重reduce的负担。原因是combine相当于一个小的reduce,所以也会有输入输出类型,且和指定的类有关,所以执行会报reduce输入不匹配的问题。
打印输出的内容在logs下面的userlog里面,也可以在集群的网页上面看,有对应的log文件。
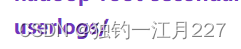