题意:使用存储在向量数据库中的嵌入来表示,以减少大型语言模型(LLM)生成响应的工作量。
问题背景:
I'm trying to understand what the correct strategy is for storing and using embeddings in a vector database, to be used with an LLM. If my goal is to reduce the amount of work the LLM has to do when generating a response, (So you can think of a RAG implementation where I've stored text, embeddings I've created using an LLM, and metadata about the text.) I'm then trying to generate responses using say openai model from queries about the data, and I don't want to have to spend a bunch of money and time chunking up the text and creating embeddings for it every time I want to answer a query about it.
我正在尝试理解在向量数据库中存储和使用嵌入的正确策略,以便与大型语言模型(LLM)一起使用。如果我的目标是减少LLM在生成响应时所需的工作量(你可以想象一个RAG实现,其中我存储了文本、使用LLM创建的嵌入以及关于文本的元数据),那么我试图通过使用例如OpenAI模型从关于数据的查询中生成响应,并且我不想每次想要回答关于数据的查询时都花费大量金钱和时间来分割文本并为其创建嵌入。
If I create a vector database, for example a chroma database and I use an LLM to create embeddings for a corpus I have. I save those embeddings into the vector database, along with the text and metadata. Would the database use those embeddings I created to find the relevant text chunks, or would it make more sense for the vector database to use it's own query process to find the relevant chunks (not using the embeddings the LLM created)?
如果我创建一个向量数据库,例如一个色度数据库,并使用一个大型语言模型(LLM)来为我已有的语料库创建嵌入表示。我将这些嵌入表示与文本和元数据一起保存到向量数据库中。那么,这个数据库会使用我创建的这些嵌入表示来找到相关的文本片段,还是让向量数据库使用它自己的查询过程来找到相关片段(而不是使用LLM创建的嵌入表示)会更合理呢?
Also do I want to pass the embeddings from the vector database to the LLM to generate the response, or do I pass the text that the vectore database found was most relevant to the LLM along with original text query so the LLM can then generate a response?
此外,我是否应该将向量数据库中的嵌入表示传递给大型语言模型(LLM)以生成响应,还是将向量数据库找到的最相关的文本与原始文本查询一起传递给LLM,以便LLM可以据此生成响应?
问题解决:
You'll initially chunk up your own content, create vector embedding using any embedding model (of your choice) and persist them to a vectore store (of your choice). Later, using techniques like prompt engineering, you will ask your questions to interact with your own proprietary data that is stored within the vector store to help find insights. During this process, your question/ask will need to be converted to embeddings (this is where embedding model comes in) to be able to sent to the vector store, which will in-turn use those embeddings to figure out the nearest / closest responses (see benefits of vector search) based on the corpus of data and will return back responses. One will then use these responses and pass it on the OpenAI (can be substituted w/ others of your choice) to prepare the final response.
首先,你需要将自己的内容切分成块,使用任何嵌入模型(你选择的)创建向量嵌入,并将它们保存到向量存储(你选择的)中。之后,通过诸如提示工程等技术,你可以向存储在向量存储中的专有数据提出问题以获取洞察。在这个过程中,你的问题/查询需要被转换为嵌入表示(这就是嵌入模型发挥作用的地方),以便能够发送到向量存储。向量存储将使用这些嵌入表示,基于数据语料库来确定最接近/最相关的响应(这就是向量搜索的优势),并返回这些响应。然后,你将使用这些响应,并将其传递给OpenAI(也可以替换为你选择的其他模型)以准备最终响应。
Would the database use those embeddings I created to find the relevant text chunks...
数据库会使用我创建的这些嵌入表示来找到相关的文本片段...
Yes, the vector store will use the vector embeddings to find the relevant match based on your input embedding
是的,向量存储将使用向量嵌入来根据你输入的嵌入表示找到相关的匹配项。
Also do I want to pass the embeddings from the vector database to the LLM to generate the response, or do I pass the text that the vectore database found was most relevant to the LLM along with original text query so the LLM can then generate a response?
另外,我是否应该将向量数据库中的嵌入表示传递给大型语言模型(LLM)以生成响应,还是将向量数据库找到的最相关的文本与原始文本查询一起传递给LLM,以便LLM可以据此生成响应?
With techniques like RAG, you would pass the text (using own proprietary data corpus) that the vector database found as relevant to the LLM to generate the final resposne.
使用如RAG(Retrieval-Augmented Generation)这样的技术,你会将向量数据库找到的相关文本(使用你自己的专有数据语料库)传递给大型语言模型(LLM),以生成最终响应。
You could check out some techniques as highlighted here to see how to build GenAI apps to take some inspiration.
你可以查看这里提到的一些技术,看看如何构建生成式人工智能(GenAI)应用,以获取一些灵感。
I hope that helps. 希望这有所帮助。
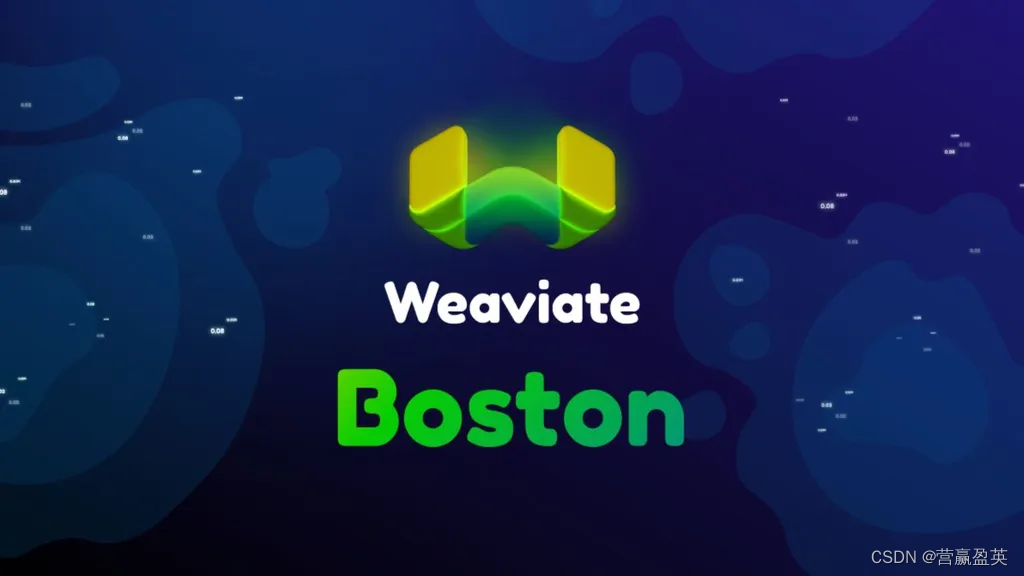