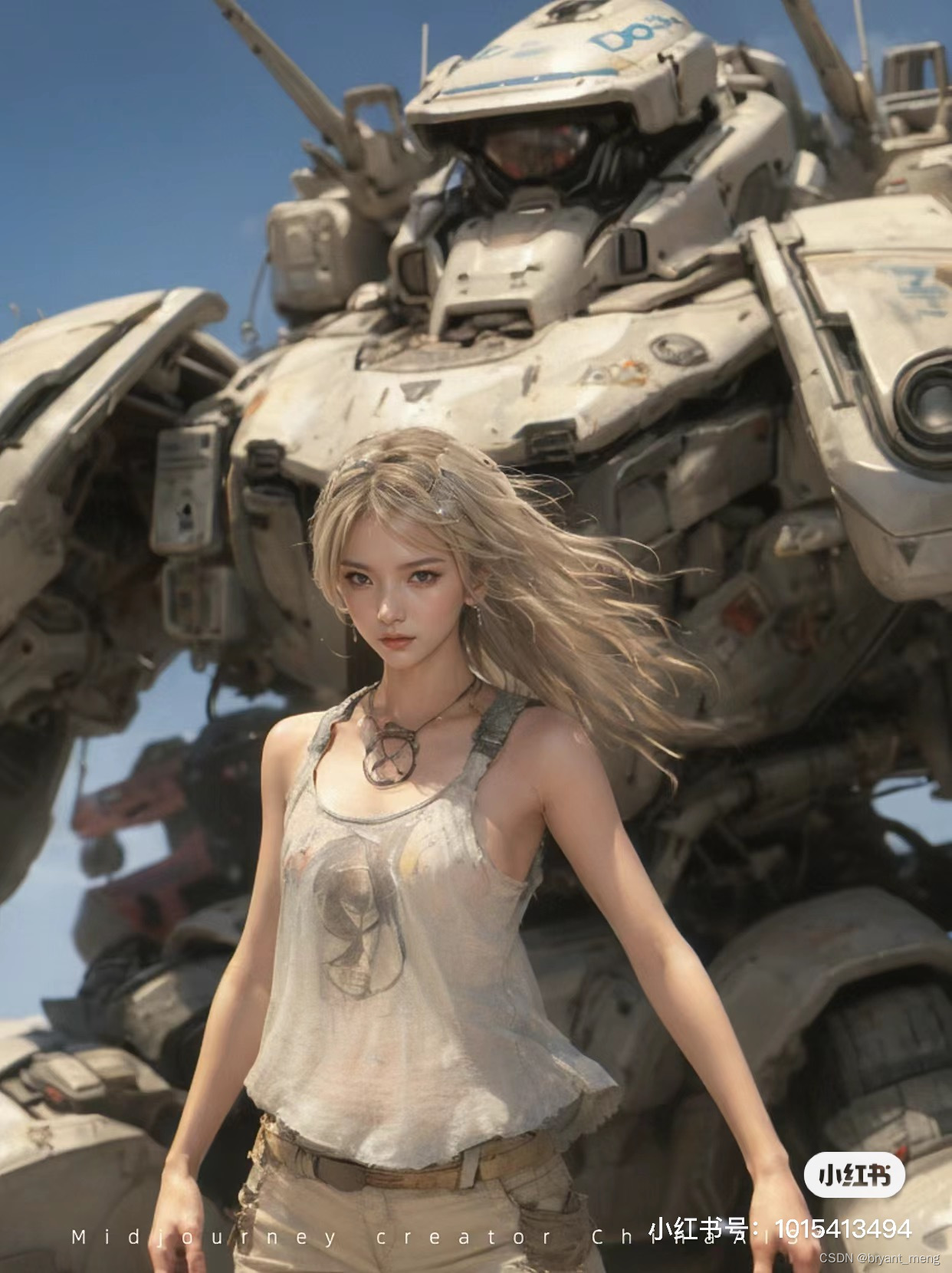
参考学习来自OpenCV基础(23)特征检测与匹配
文章目录
- [1 背景介绍](#1 背景介绍)
- [2 Harris角点检测](#2 Harris角点检测)
- [3 Shi-Tomasi角点检测](#3 Shi-Tomasi角点检测)
- [4 Fast 角点检测](#4 Fast 角点检测)
- [5 BRIEF 特征描述子](#5 BRIEF 特征描述子)
- [6 ORB(Oriented Fast and Rotated Brief) 特征描述子](#6 ORB(Oriented Fast and Rotated Brief) 特征描述子)
- [7 SIFT(Scale Invariant Feature Transform) 特征描述子](#7 SIFT(Scale Invariant Feature Transform) 特征描述子)
- [8 SURF(Speeded Up Robust Features) 特征描述子](#8 SURF(Speeded Up Robust Features) 特征描述子)
- [9 Brute-Force Matcher 特征匹配](#9 Brute-Force Matcher 特征匹配)
- [10 FLANN Matcher 特征匹配](#10 FLANN Matcher 特征匹配)
1 背景介绍
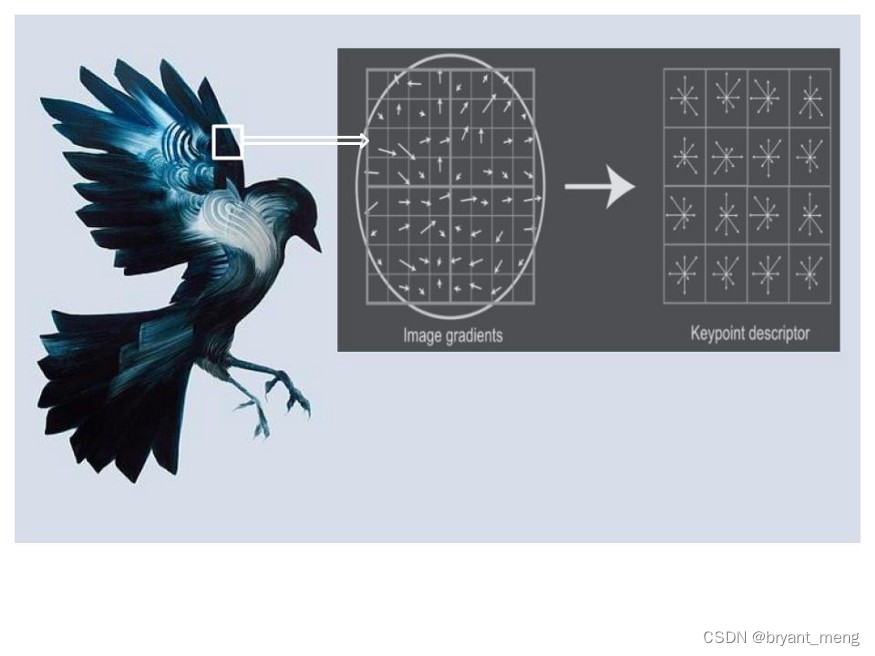
在计算机图像处理中,特征检测和匹配是两个至关重要的环节,它们在多个领域如SLAM(即时定位与地图构建)、SFM(结构从运动恢复)、AR(增强现实)、VR(虚拟现实)等中发挥着关键作用。以下是对特征检测和匹配的详细解析:
一、特征检测
- 定义与意义
特征检测是指使用计算机算法从图像中提取出具有独特性质的特征点,这些特征点能够代表图像中的关键信息,并且通常具有旋转、尺度和光照变化的不变性。这些特性使得特征点在图像的不同位置和角度下都能被准确检测。
- 特征点的性质
- 独特性:特征点应具有足够的独特性,以便在不同图像间进行区分。
- 可重复性:同一场景的不同图像所提取的特征点应保持一致。
- 稳定性:即使图像发生光照、旋转或尺度变化,特征点也应保持稳定。
- 检测方法
- 基于图像的亮度:通过图像导数来检测图像中的局部极值点,如SIFT(尺度不变特征变换)算法。
- 基于边界提取:通过边缘检测和曲率分析来提取特征点,如Canny边缘检测算法后进一步分析边缘的走向。
- 常用算法
- SIFT(尺度不变特征变换):该算法通过构建图像金字塔,在不同尺度下寻找局部极值点,并生成描述子来描述这些特征点。
- ORB(Oriented FAST and Rotated BRIEF):ORB算法结合了FAST特征点检测子和BRIEF特征描述子,并加入了方向信息,提高了特征点的旋转不变性。
- HOG(方向梯度直方图):虽然HOG主要用于目标检测,但它通过计算和统计图像局部区域的梯度方向直方图来构成特征,也是一种有效的特征描述方法。
二、特征匹配
- 定义与意义
特征匹配是指将两个或多个图像中的特征点进行对应,以实现图像间的关联和匹配。通过特征匹配,可以进行目标跟踪、图像配准、三维重建等任务。
- 匹配方法
- 距离计算:常见的距离计算方法包括欧式距离、余弦距离等,用于计算特征向量之间的距离,从而确定特征点之间的匹配关系。
- 匹配算法:常用的匹配算法包括暴力匹配(BruteForce)和基于近似最近邻的匹配(FlannBased)等。
- 匹配策略
- 单应性矩阵估计:在图像配准和拼接中,常通过估计单应性矩阵来实现两幅图像之间的变换关系。
- RANSAC(随机抽样一致算法):在存在大量误匹配点的情况下,RANSAC算法可以通过迭代的方式找到最佳的单应性矩阵估计。
三、总结
在计算机图像处理中,特征检测和匹配是实现图像识别、目标跟踪、图像配准等任务的基础。通过设计和改进特征点检测算法、优化特征描述子、采用多尺度和多模态特征检测与匹配策略,以及应用深度学习等先进技术,可以进一步提高特征检测和匹配的准确性和鲁棒性。这些技术的应用不仅推动了计算机视觉领域的发展,也为自动驾驶、智能监控、虚拟现实等多个领域提供了有力的技术支持。
2 Harris角点检测
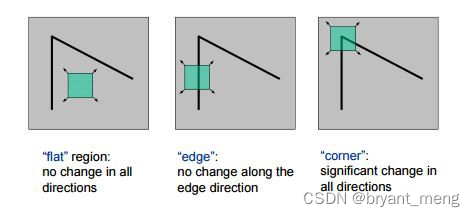
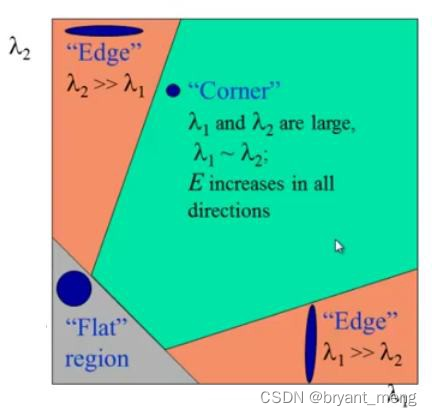
特征值,特征向量(花了10分钟,终于弄懂了特征值和特征向量到底有什么意义)
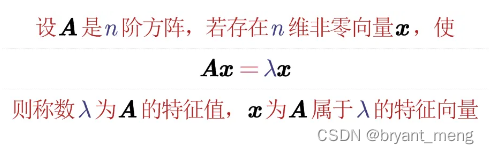
Harris角点检测是一种在计算机视觉中广泛应用的角点检测算法,它能够有效地在图像中检测出角点特征。以下是关于Harris角点检测的详细介绍:
- 定义与原理
- 定义:Harris角点检测用于检测图像中的角点,这些角点通常表示物体的边缘或角落,是图像中具有明显亮度变化的位置。
- 原理:Harris角点检测算法通过计算图像中每个像素的角点响应函数 来确定角点的位置。该算法首先计算图像的梯度,然后计算每个像素周围窗口内的梯度幅值和方向的自相关矩阵。接着,使用这个自相关矩阵来计算每个像素的角点响应函数,该函数度量了像素周围区域的角点特征。最后,通过非极大值抑制来保留局部最大值作为角点。
- 优点
- 鲁棒性强:Harris角点检测算法对图像的旋转、缩放和亮度变化具有较好的鲁棒性。
- 计算效率高:算法的计算复杂度相对较低,适用于实时应用。
- 检测准确性高:Harris角点检测算法能够准确地检测出图像中的角点位置。
- 缺点
- 对噪声敏感:算法对图像中的噪声比较敏感,可能会将噪声误判为角点。
- 对尺度变化不敏感:Harris角点检测算法在处理尺度变化较大的图像时效果较差。
- 对旋转变化不敏感:虽然算法对图像的旋转变化有一定的鲁棒性,但在某些情况下可能会导致角点检测结果不准确。
- 改进方法
为了解决Harris角点检测算法的一些缺点,研究者们提出了一些改进的方法,如尺度不变特征变换(SIFT)和加速稳健特征(SURF)等。这些改进算法在角点检测的准确性和鲁棒性上有所提升。
- 应用领域
Harris角点检测算法在计算机视觉领域有广泛的应用,包括但不限于
- 物体识别:通过检测物体上的角点特征来进行物体识别。
- 图像配准:利用角点特征来对齐和匹配两幅或多幅图像。
- 三维重建:通过角点检测来提取和重建物体的三维结构。
总之,Harris角点检测是一种简单、快速且有效的角点检测方法,在计算机视觉领域具有广泛的应用前景。
实现可参考 :【python】OpenCV---Edge, Corner, Face Detection(3)
python
import cv2
import numpy as np
# 读取原始图像
cv_image = cv2.imread("1.jpg")
cv2.namedWindow("src", 0)
cv2.imshow("src", cv_image)
# 转换为灰度
gray_image = cv2.cvtColor(cv_image, cv2.COLOR_BGR2GRAY)
# 将图像像素数据转换为 float32,以避免进一步的尺寸不兼容冲突
gray_image = np.float32(gray_image)
# syntax cv2.corenrHarris(input_image, block size for neighborhood pixels to be considered, sobel operator size, border type)
result_image = cv2.cornerHarris(gray_image, blockSize=2, ksize=3, k=0.04)
cv2.imshow("result_image", result_image)
cv2.imwrite("result_image.jpg", result_image)
# 膨胀以突出角点
result_image = cv2.dilate(result_image, None)
cv2.imshow("dilate", result_image)
cv2.imwrite("dilate.jpg", result_image)
# 使用最佳阈值恢复到原始图像
cv_image[result_image > 0.01 * result_image.max()] = [0, 0, 255]
cv2.namedWindow("haris", 0)
cv2.imwrite("haris.jpg", cv_image)
cv2.imshow("haris", cv_image)
cv2.waitKey()
输入图片
result_image.jpg
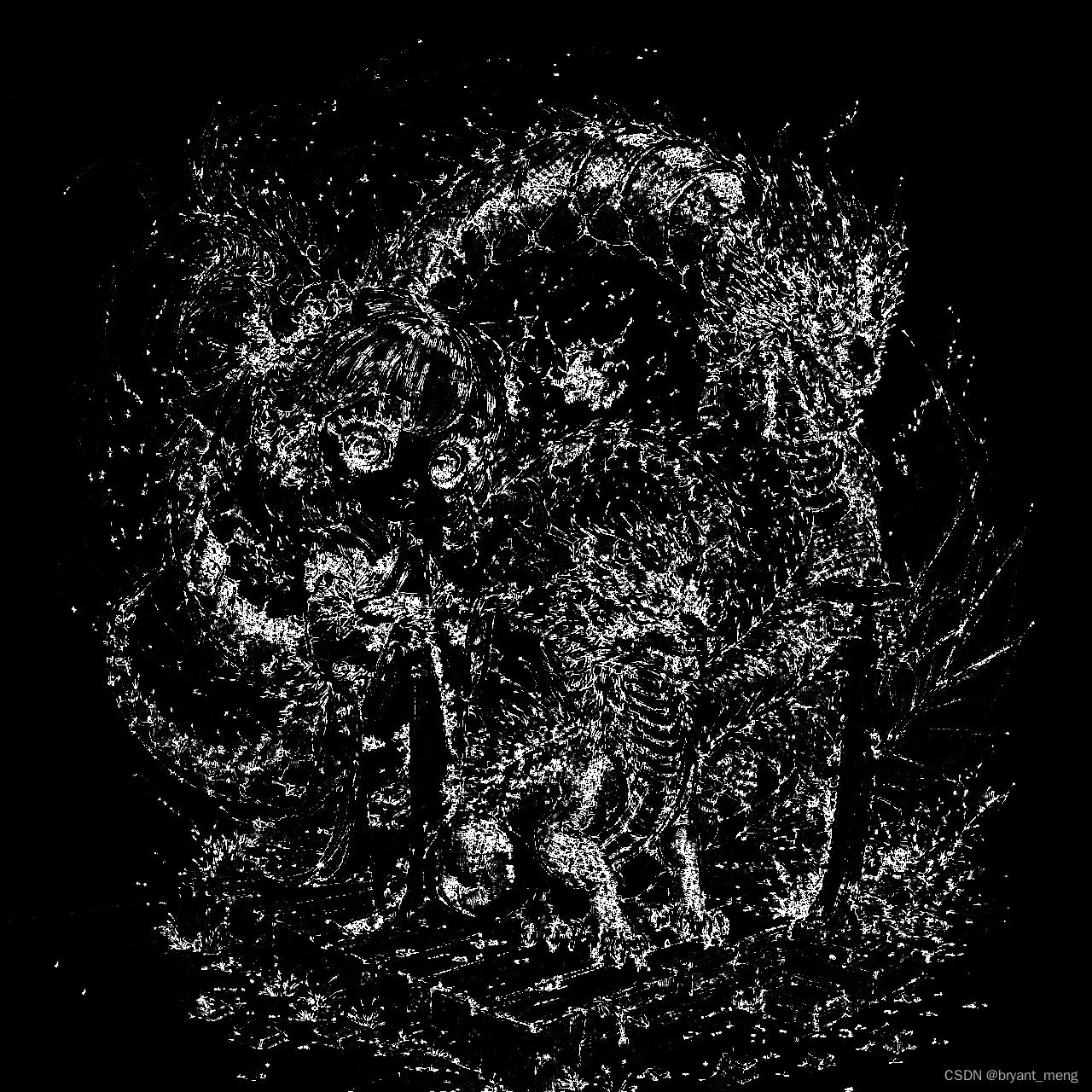
dilate.jpg
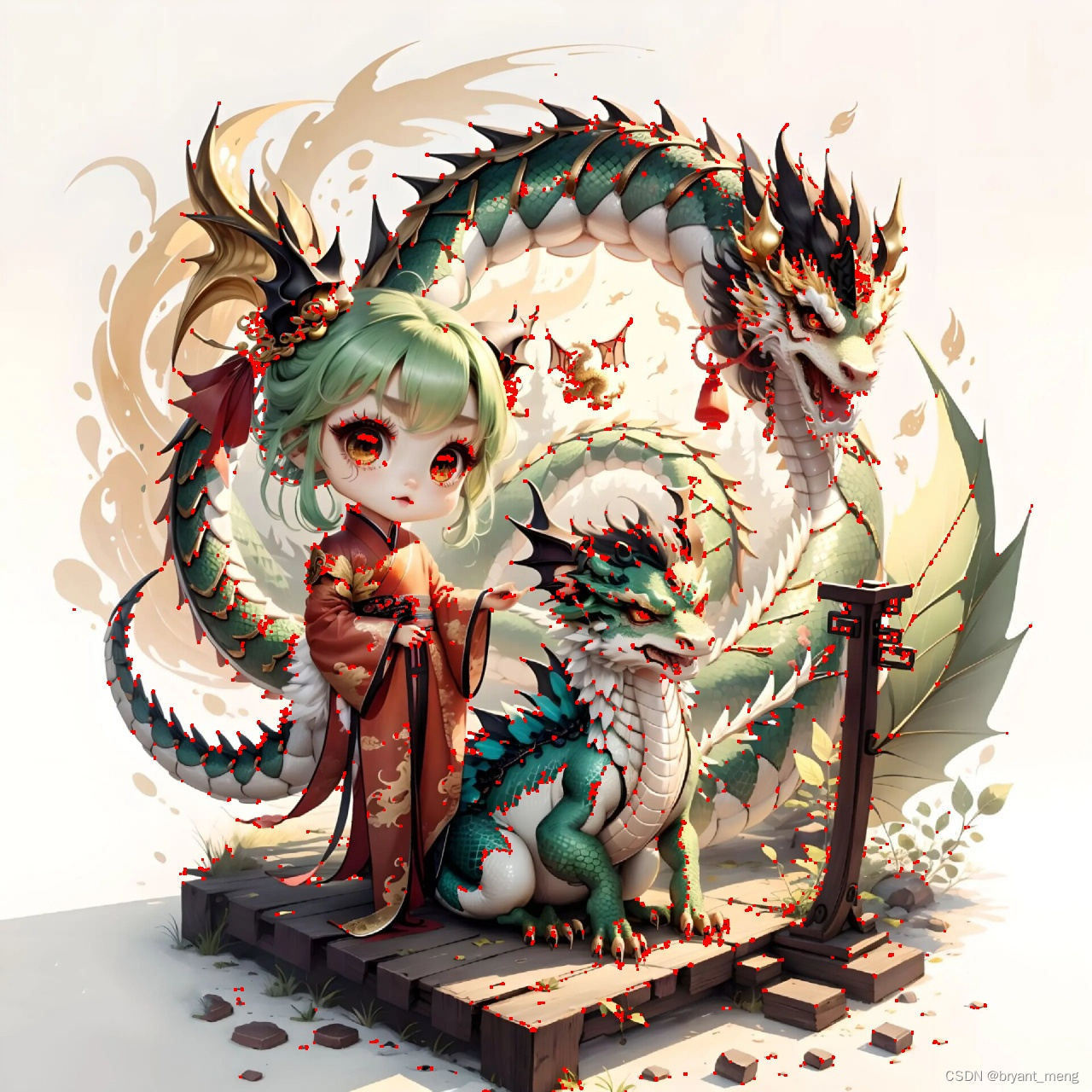
3 Shi-Tomasi角点检测
一、算法概述
- 算法来源:Shi-Tomasi算法于1994年在文章《Good Features to Track》中被提出。
- 算法基础:基于Harris角点检测算法,通过改进角点响应函数的计算方式,提高了角点检测的准确性和效率。
二、算法原理
- 角点定义:在图像中,角点是指那些在各个方向上灰度变化都很大的点。具体来说,当窗口在角点处沿任意方向移动时,窗口内的灰度变化都非常大。
- 特征值分析:Shi-Tomasi算法通过计算图像中每个像素点的梯度,并构建自相关矩阵M。然后,对M进行特征值分解,得到两个特征值λ1和λ2。
- 角点响应函数:Shi-Tomasi算法使用min(λ1, λ2)作为角点响应函数R。如果R大于设定的阈值,则认为该点为角点。这种改进使得算法能够更准确地识别出图像中的强角点。
三、demo
实现可参考 :【python】OpenCV---Edge, Corner, Face Detection(3)
python
import numpy as np
import cv2
# 输入图像
cv_img = cv2.imread('1.jpg')
cv_gray = cv2.cvtColor(cv_img, cv2.COLOR_BGR2GRAY)
# syntax cv2.goodFeaturesToTrack(input_image, max_corner_to_detect, qualityLevel, minDistance)
corners = cv2.goodFeaturesToTrack(cv_gray, maxCorners=25, qualityLevel=0.01, minDistance=10)
corners = np.float32(corners)
for item in corners:
x, y = item[0].astype("int")
cv2.circle(cv_img, (x, y), 3, (0, 0, 255), -1)
cv2.imshow("image", cv_img)
cv2.imwrite("shi_result.jpg", cv_img)
cv2.waitKey()
输入图片
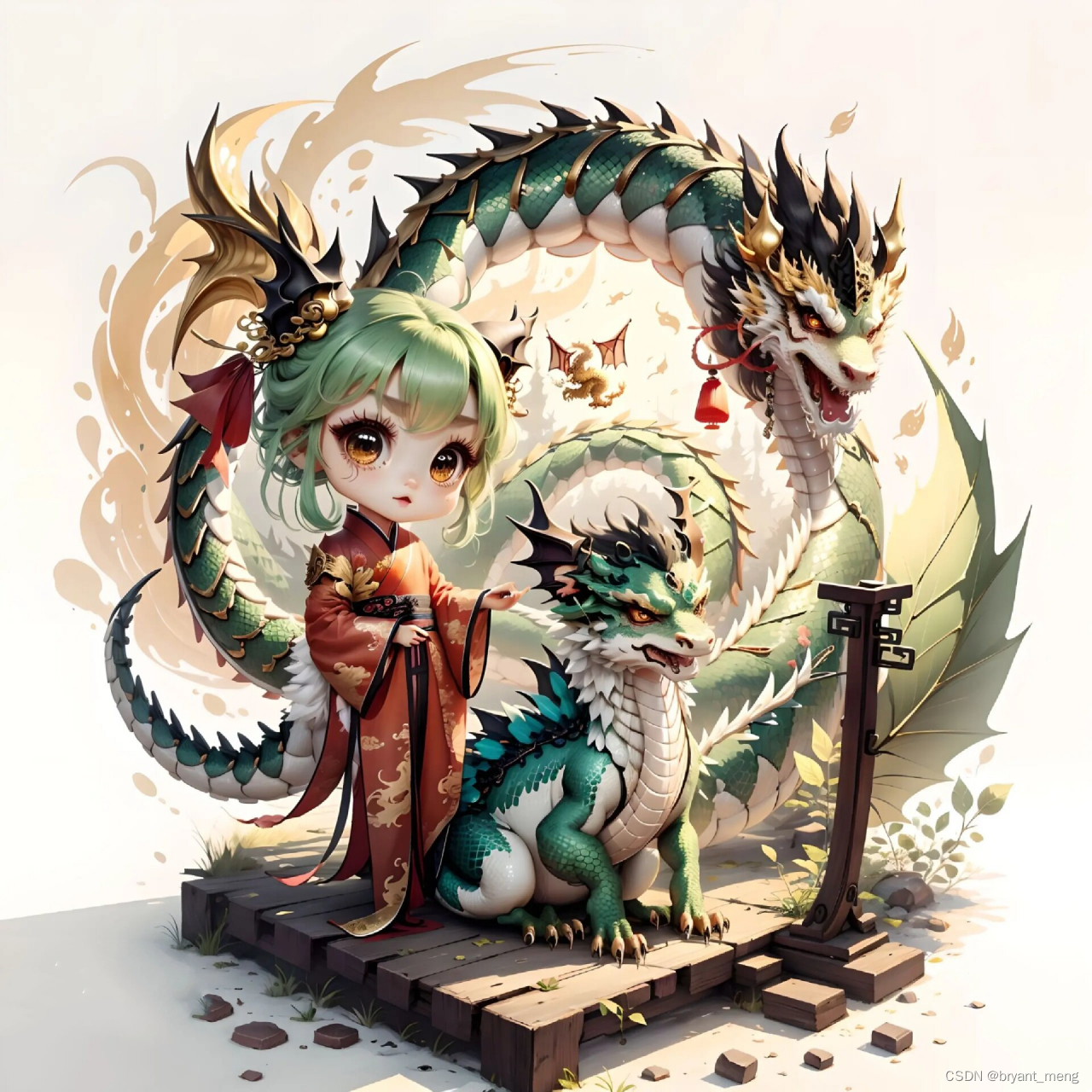
输出
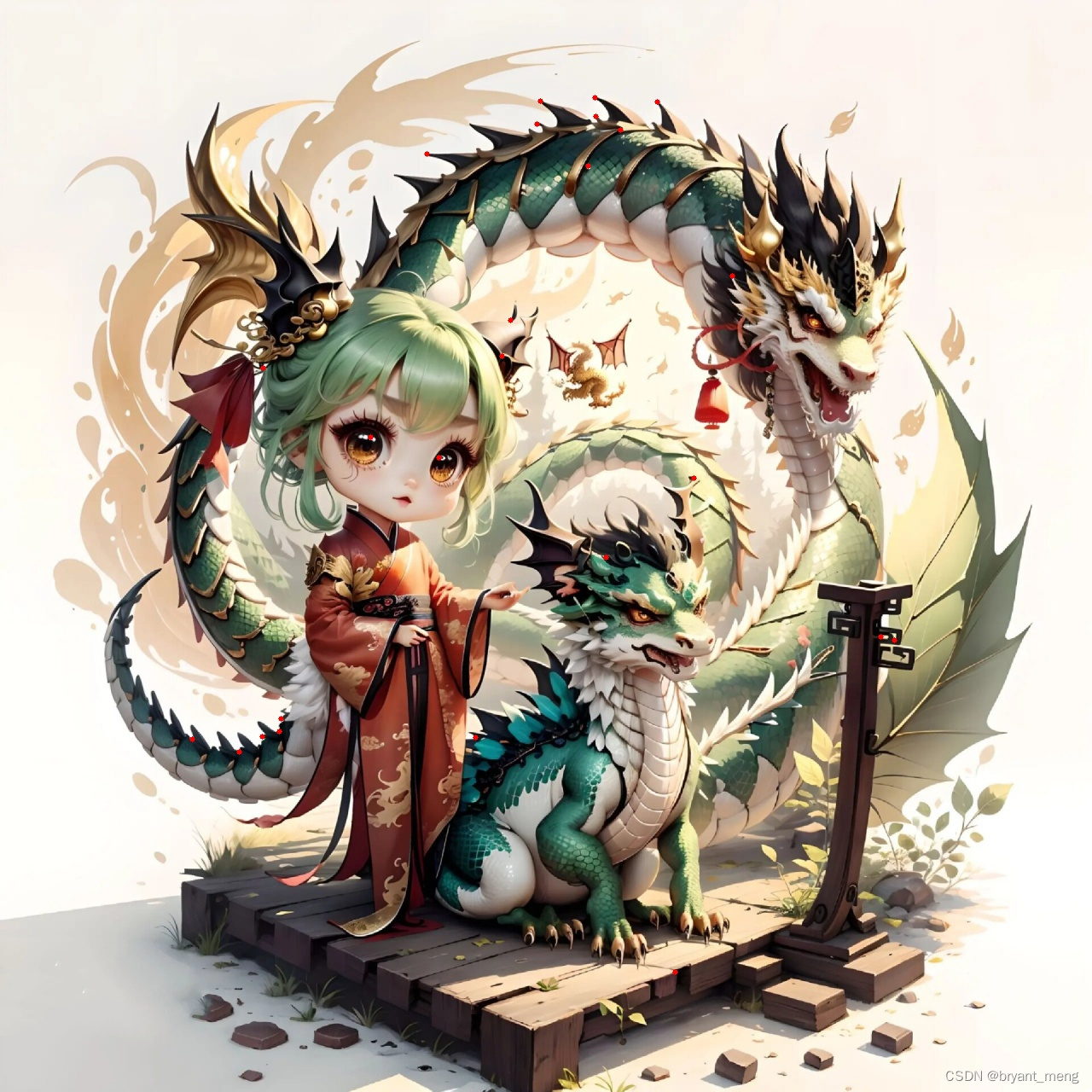
可以看到零星的红色角点,效果一般
4 Fast 角点检测
FAST(Features from Accelerated Segment Test)角点检测算法是一种快速且高效的角点检测方法,广泛应用于计算机视觉和图像处理领域。
一、算法原理
- FAST角点检测算法的基本思想是通过比较一个像素点与其周围像素点的灰度差异来判断该点是否为角点。具体来说,如果一个像素点与其周围足够多的像素点在灰度上存在显著差异(即这些点的灰度值要么都比中心像素点的灰度值大t,要么都比其小t,其中t为设定的阈值),则认为该像素点为角点。
二、算法步骤
- 选择候选点:从图像中选取一个像素点p作为候选点。
- 设置阈值:设定一个灰度阈值t,用于比较像素点之间的灰度差异。
- 选择圆形区域:以候选点p为中心,选择一个半径为3像素的离散化圆(即Bresenham圆),该圆上通常有16个等间隔的像素点作为测试点。
- 比较灰度值:比较候选点p的灰度值Ip与圆周上16个测试点的灰度值。如果在这16个点中,有连续N个点的灰度值要么都比Ip+t大,要么都比Ip-t小(N通常取12,但也可以取9或11等其他值),则认为候选点p是一个角点候选。
- 加速检测:为了提高检测速度,FAST算法采用了一种预测试策略。首先比较圆周上第1、5、9、13这四个点的灰度值,如果这四个点中有三个或三个以上的点与中心点的灰度差异满足条件(即大于Ip+t或小于Ip-t),则继续进行后续的16点检测;否则,直接排除该候选点。
- 非极大值抑制:对检测到的所有角点候选进行非极大值抑制处理,去除局部非极大值点,从而得到最终的角点集合。
三、算法优势
- 速度快:FAST算法通过预测试策略和高效的灰度比较方法,显著提高了角点检测的速度。
- 精度高:尽管FAST算法速度快,但其检测精度也相当高,能够满足大多数应用场景的需求。
- 易于实现:FAST算法的原理相对简单,实现起来较为容易,适用于多种编程语言和开发环境。
四、demo
python
import cv2
# 输入图像
cv_img = cv2.imread('../1.jpg')
cv_gray = cv2.cvtColor(cv_img, cv2.COLOR_BGR2GRAY)
fast = cv2.FastFeatureDetector_create()
fast.setNonmaxSuppression(False)
keypoint = fast.detect(cv_gray, None)
keypoint_image = cv2.drawKeypoints(cv_img, keypoint, None, color=(0, 0, 255))
cv2.imshow("FAST", keypoint_image)
cv2.imwrite("fast.jpg", keypoint_image)
cv2.waitKey()
输出图像,不开启 NMS
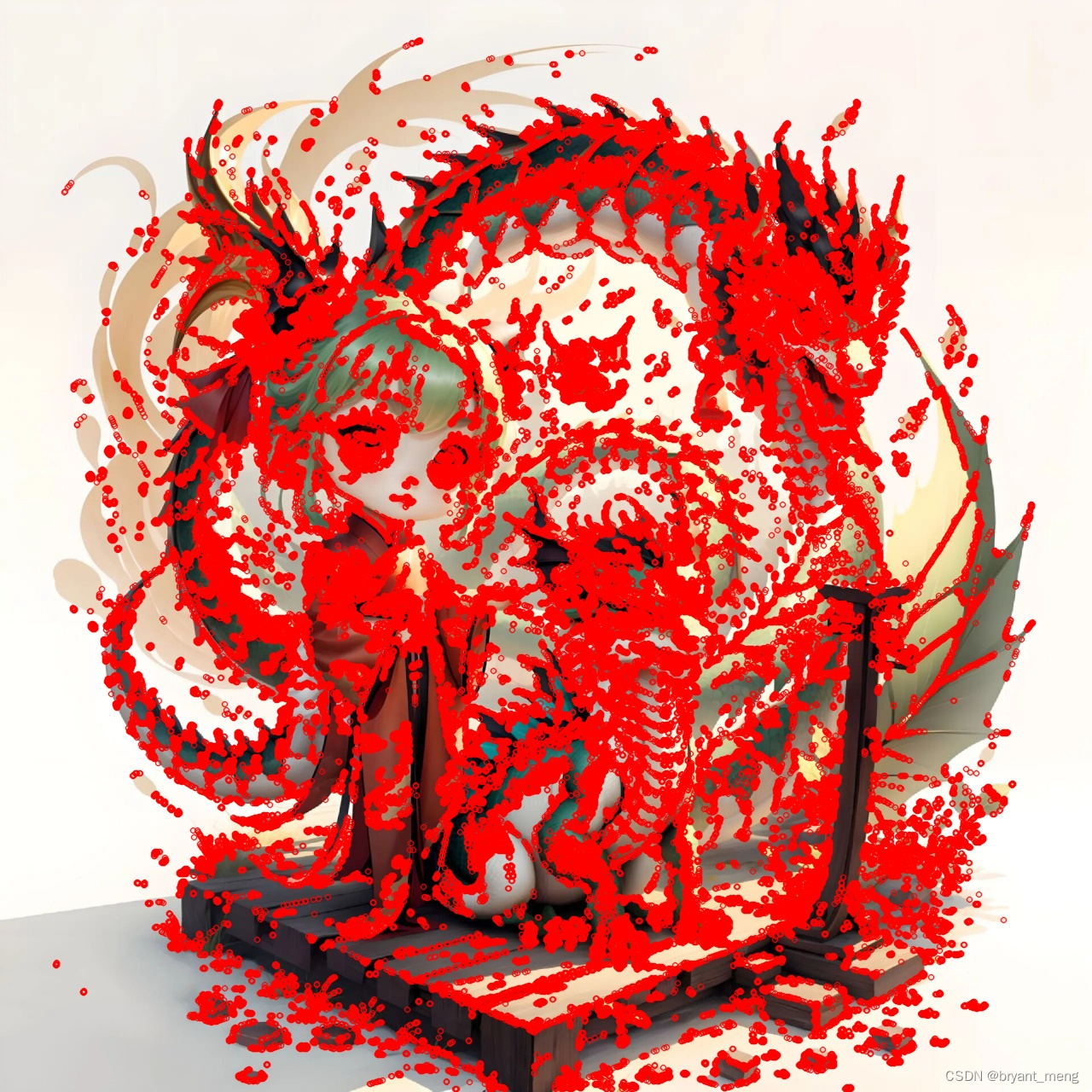
下面对比下开启 NMS 和不开启 NMS 的结果对比
python
#!/usr/bin/python3
# -*- encoding: utf-8 -*-
# FAST
import cv2
import matplotlib.pyplot as plt
import numpy as np
# 加载图像
image = cv2.imread('../1.jpg')
# 将图像转换为 RGB
image = cv2.cvtColor(image, cv2.COLOR_BGR2RGB)
# 将图像转换为灰度
gray = cv2.cvtColor(image, cv2.COLOR_RGB2GRAY)
# 显示图像和灰度图像
fx, plots = plt.subplots(1, 2, figsize=(20,10))
plots[0].set_title("Orignal Image")
plots[0].imshow(image)
plots[0].axis("off")
plots[1].set_title("Gray Image")
plots[1].imshow(gray, cmap="gray")
plots[1].axis("off")
plt.savefig("gray.jpg")
plt.show()
fast = cv2.FastFeatureDetector_create()
# 用非最大抑制检测关键点
keypoints_with_nonmax = fast.detect(gray, None)
# 禁用非最大抑制
fast.setNonmaxSuppression(False)
# 在没有非最大抑制的情况下检测关键点
keypoints_without_nonmax = fast.detect(gray, None)
image_with_nonmax = np.copy(image)
image_without_nonmax = np.copy(image)
# 在输入图像上绘制关键点
cv2.drawKeypoints(image, keypoints_with_nonmax, image_with_nonmax, color=(255,0,0),
flags=cv2.DRAW_MATCHES_FLAGS_DRAW_RICH_KEYPOINTS)
cv2.drawKeypoints(image, keypoints_without_nonmax, image_without_nonmax, color=(255,0,0),
flags=cv2.DRAW_MATCHES_FLAGS_DRAW_RICH_KEYPOINTS)
# 显示有和没有非最大抑制的图像
fx, plots = plt.subplots(1, 2, figsize=(20,10))
plots[0].set_title("With non max suppression")
plots[0].imshow(image_with_nonmax)
plots[0].axis("off")
plt.imsave("nms.jpg", image_with_nonmax)
plots[1].set_title("Without non max suppression")
plots[1].imshow(image_without_nonmax)
plots[1].axis("off")
plt.imsave("nonms.jpg", image_without_nonmax)
# 打印使用NMS在图像中检测到的关键点数
print("Number of Keypoints Detected In The Image With Non Max Suppression: ", len(keypoints_with_nonmax))
# 打印不使用NMS在图像中检测到的关键点数
print("Number of Keypoints Detected In The Image Without Non Max Suppression: ", len(keypoints_without_nonmax))
plt.show()
# Number of Keypoints Detected In The Image With Non Max Suppression: 17295
# Number of Keypoints Detected In The Image Without Non Max Suppression: 89925
输出 gray.jpg
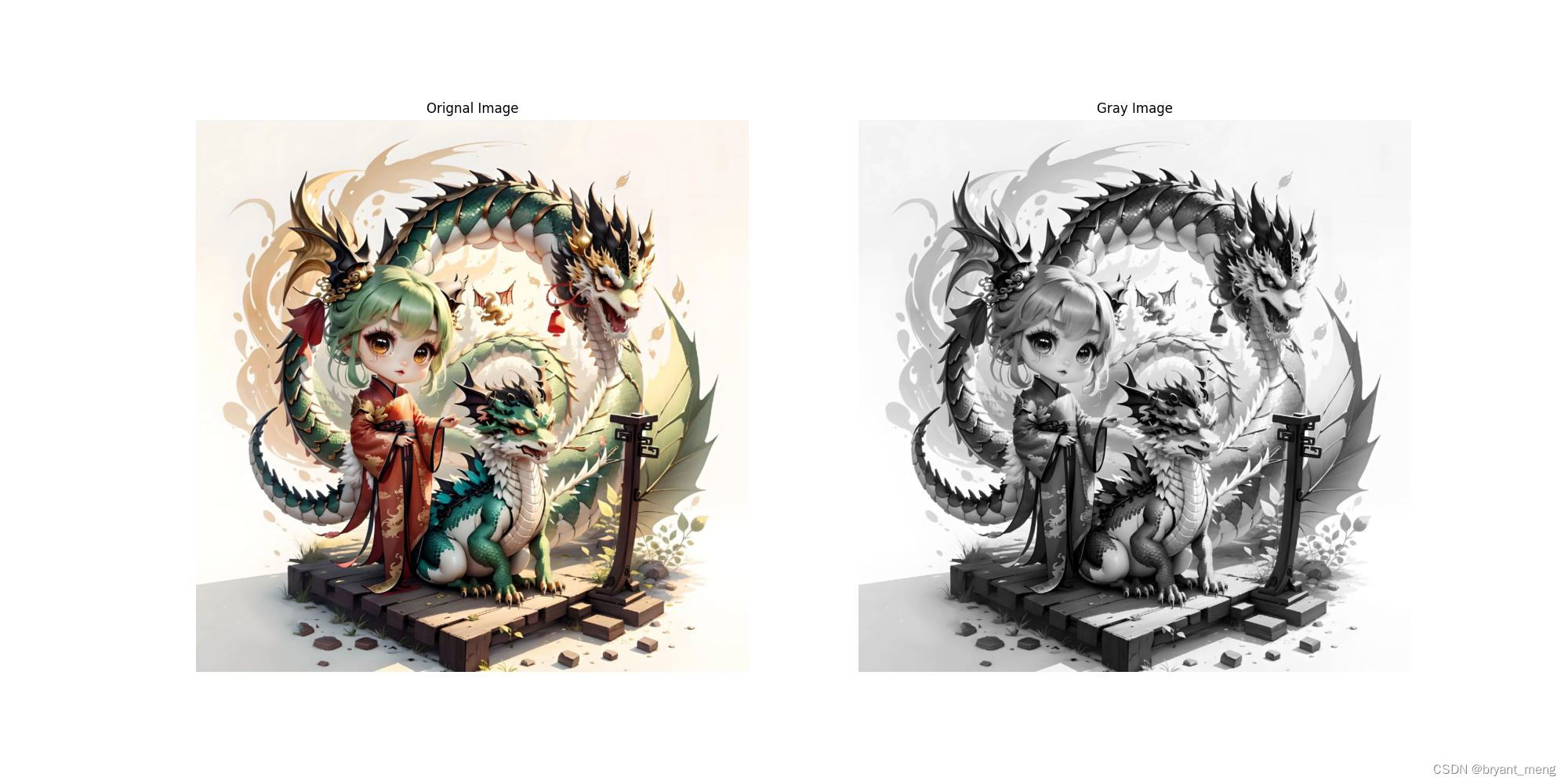
输出 nonms.jpg
输出 nms.jpg
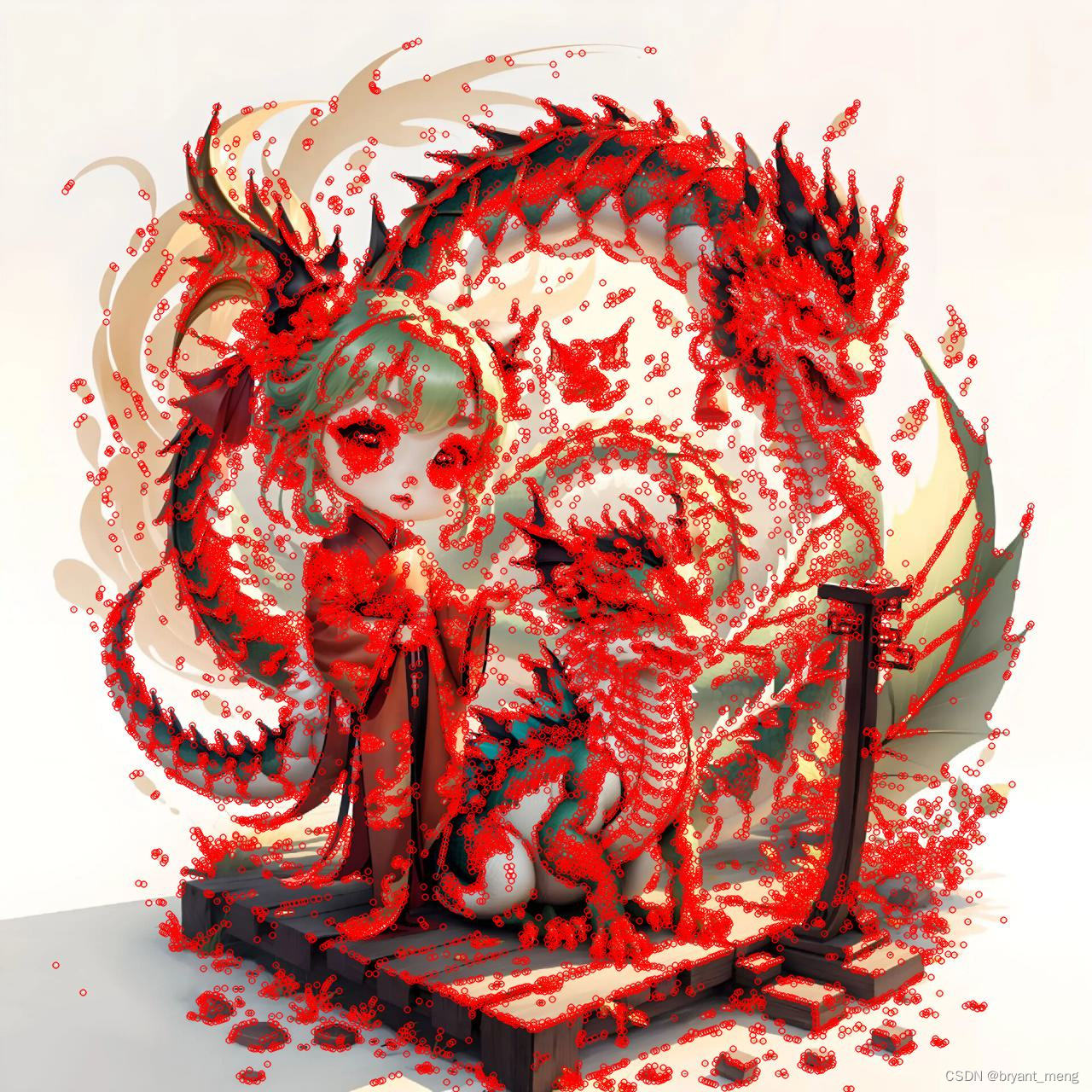
5 BRIEF 特征描述子
在图像领域,特征描述子(Feature Descriptors)是用于描述图像中特定属性或纹理信息的数学表示,它们在图像匹配、对象识别和图像检索等任务中发挥着关键作用。eg:SHIFT(尺度不变特征变换)、SURF(加速稳健特征)、ORB(加速稳健特征)、HOG(方向梯度直方图)、LBP(局部二值模式)
BRIEF(Binary Robust Independent Elementary Features)是一种高效的特征描述子算法,它主要用于对图像中的关键点(如角点)进行描述,以便进行特征匹配、图像识别等任务。BRIEF算法的核心在于通过比较关键点周围随机选取的点对的像素强度差异,来生成一个紧凑的二进制字符串作为该关键点的描述子。
特点
- 二进制字符串:BRIEF描述子是一个二进制字符串,这意味着它非常紧凑,易于存储和传输,同时也便于快速比较。
- 随机采样:在关键点周围的邻域内,BRIEF通过随机选取的点对来生成描述子。这种随机性使得算法对噪声和小的形变具有一定的鲁棒性。
- 快速计算:由于描述子是二进制的,因此其计算和匹配过程都非常快速,特别适合实时应用。
- 非旋转不变性:原始的BRIEF算法并不具有旋转不变性,即如果图像发生旋转,则同一关键点的BRIEF描述子也会发生变化。然而,通过结合具有旋转不变性的关键点检测算法(如ORB中的oFAST)或在描述子生成前对关键点邻域进行旋转校正,可以使得BRIEF描述子具有旋转不变性。
- 尺度不变性:BRIEF算法本身并不直接处理尺度不变性。然而,在实际应用中,通常会与具有尺度不变性的关键点检测算法结合使用,或者通过构建图像金字塔并在多个尺度上检测关键点来间接实现尺度不变性。
Demo
python
#!/usr/bin/python3
# -*- encoding: utf-8 -*-
"""
@File : BRIEF.py
@Time : 2021/10/13 11:07
@Author : David
@Software: PyCharm
"""
# BRIEF(Binary Robust Independent Elementary Features)
# 导入库,显示图像
import cv2
import matplotlib.pyplot as plt
import numpy as np
# 加载图像
image1 = cv2.imread('./2.png')
# 将训练图像转换为RGB
training_image = cv2.cvtColor(image1, cv2.COLOR_BGR2RGB)
# 将训练图像转换为灰度
training_gray = cv2.cvtColor(training_image, cv2.COLOR_RGB2GRAY)
# 通过添加缩放不变性和旋转不变性来创建测试图像
test_image = cv2.pyrDown(training_image)
test_image = cv2.pyrDown(test_image)
num_rows, num_cols = test_image.shape[:2]
rotation_matrix = cv2.getRotationMatrix2D((num_cols / 2, num_rows / 2), 30, 1)
test_image = cv2.warpAffine(test_image, rotation_matrix, (num_cols, num_rows))
test_gray = cv2.cvtColor(test_image, cv2.COLOR_RGB2GRAY)
# 显示训练图像和测试图像
fx, plots = plt.subplots(1, 2, figsize=(20, 10))
plots[0].set_title("Training Image")
plots[0].imshow(training_image)
plots[0].axis("off")
plots[1].set_title("Testing Image")
plots[1].imshow(test_image)
plots[1].axis("off")
plt.savefig("tran-test.jpg")
# 检测关键点并创建描述符
fast = cv2.FastFeatureDetector_create()
brief = cv2.xfeatures2d.BriefDescriptorExtractor_create()
train_keypoints = fast.detect(training_gray, None)
test_keypoints = fast.detect(test_gray, None)
train_keypoints, train_descriptor = brief.compute(training_gray, train_keypoints)
test_keypoints, test_descriptor = brief.compute(test_gray, test_keypoints)
keypoints_without_size = np.copy(training_image)
keypoints_with_size = np.copy(training_image)
cv2.drawKeypoints(training_image, train_keypoints, keypoints_without_size,
color=(0, 255, 0))
cv2.drawKeypoints(training_image, train_keypoints, keypoints_with_size,
flags=cv2.DRAW_MATCHES_FLAGS_DRAW_RICH_KEYPOINTS)
# 显示图像有和没有关键点大小
fx, plots = plt.subplots(1, 2, figsize=(20, 10))
plots[0].set_title("Train keypoints With Size")
plots[0].imshow(keypoints_with_size, cmap='gray')
plots[0].axis("off")
plots[1].set_title("Train keypoints Without Size")
plots[1].imshow(keypoints_without_size, cmap='gray')
plots[1].axis("off")
plt.savefig("keypoints.jpg")
# 打印训练图像中检测到的关键点数量
print("Number of Keypoints Detected In The Training Image: ", len(train_keypoints))
# 打印查询图像中检测到的关键点数量
print("Number of Keypoints Detected In The Query Image: ", len(test_keypoints))
# 匹配关键点
# 创建一个Brute Force Matcher对象。
bf = cv2.BFMatcher(cv2.NORM_HAMMING, crossCheck=True)
# 对训练图像和测试图像的BRIEF描述符进行匹配
matches = bf.match(train_descriptor, test_descriptor)
# 距离较短的是我们想要的。
matches = sorted(matches, key=lambda x: x.distance)
result = cv2.drawMatches(training_image, train_keypoints, test_gray, test_keypoints, matches, test_gray, flags=2)
# 显示最佳匹配点
plt.rcParams['figure.figsize'] = [14.0, 7.0]
plt.title('Best Matching Points')
plt.imsave("matching.jpg", result)
plt.imshow(result)
plt.show()
# 打印训练图像和查询图像之间的匹配点总数
print("\nNumber of Matching Keypoints Between The Training and Query Images: ", len(matches))
# Number of Keypoints Detected In The Training Image: 7975
# Number of Keypoints Detected In The Query Image: 525
# Number of Matching Keypoints Between The Training and Query Images: 194
tran-test.jpg
注意,测试图片进行了两次下采样,大小为原图的 1/4,这里可视化成一样的大小了
keypoints.jpg
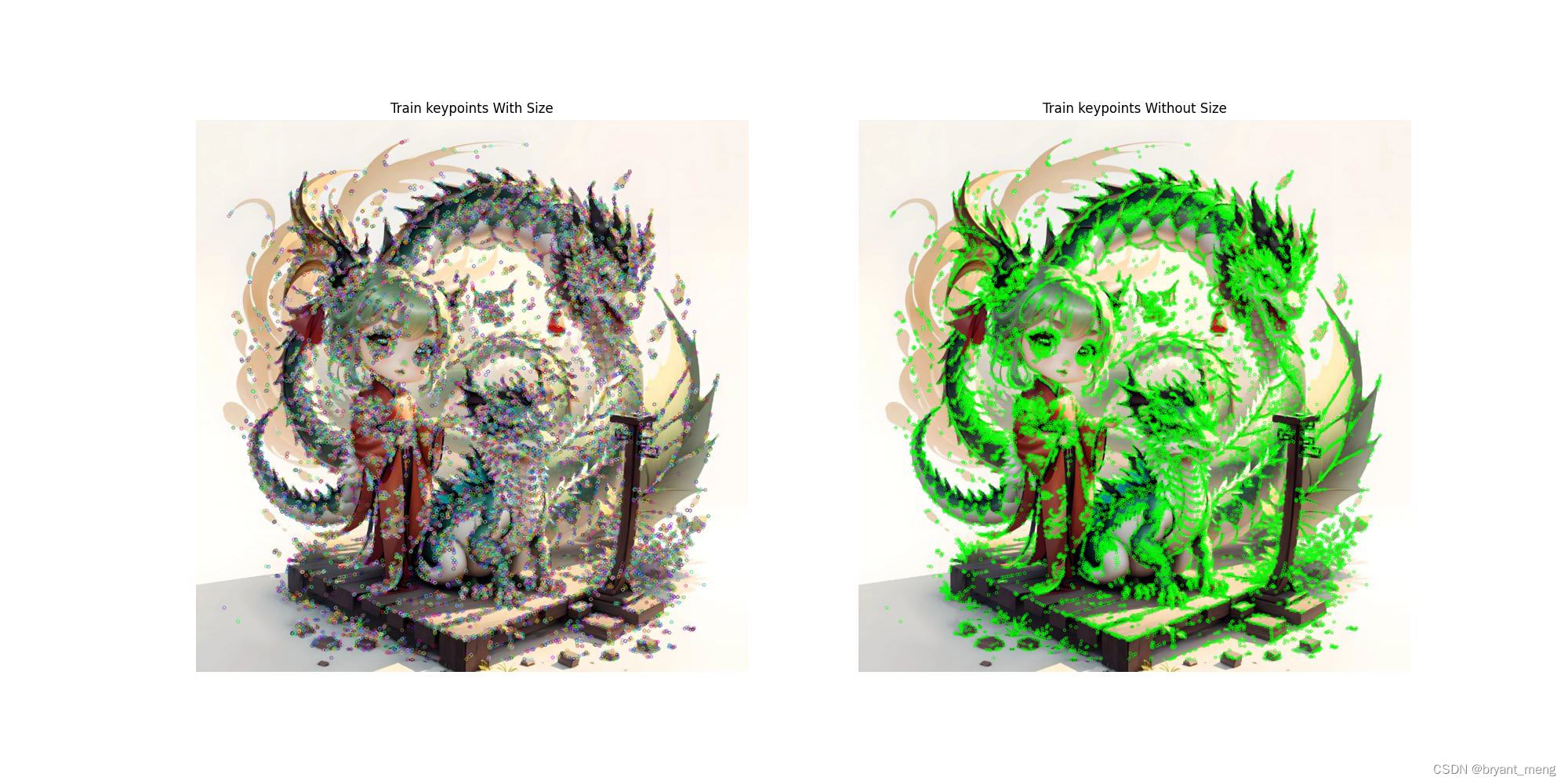
matching.jpg------BRIEF+Brute Force Matcher
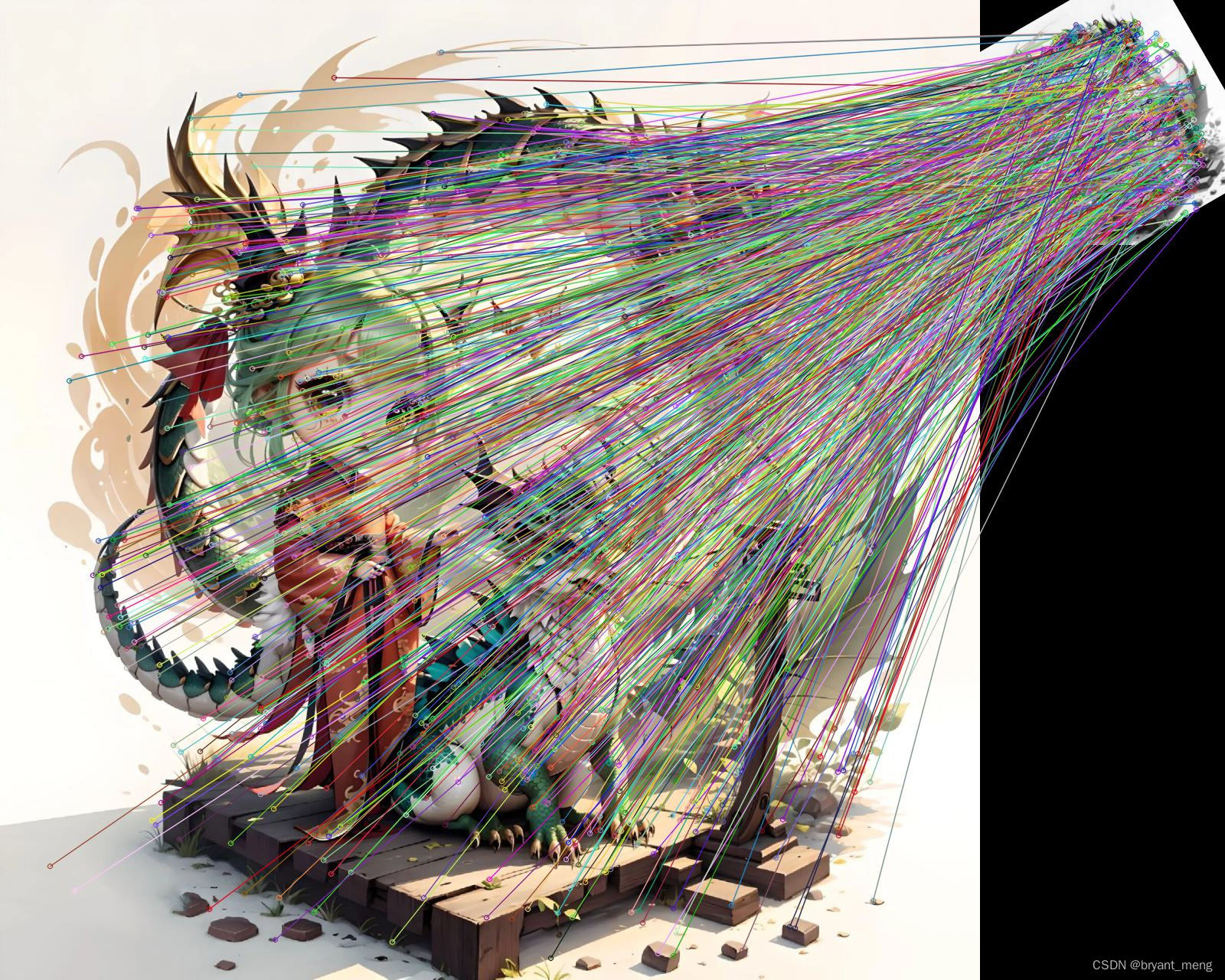
看看另外一张图片的匹配结果
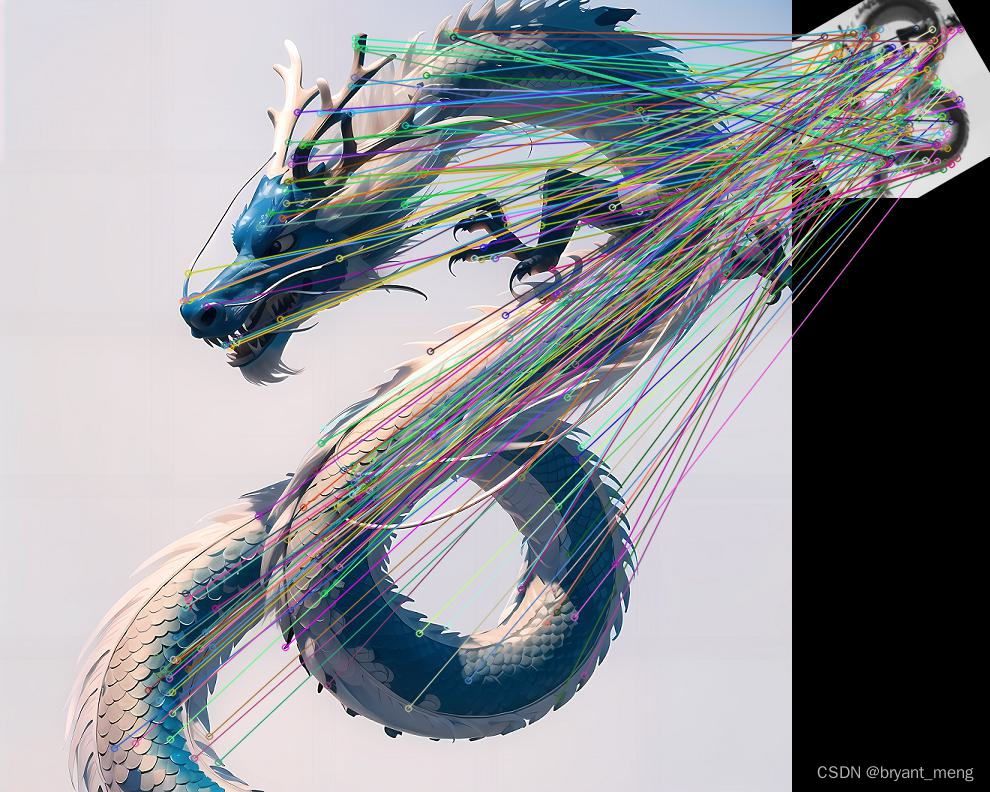
6 ORB(Oriented Fast and Rotated Brief) 特征描述子
python
import cv2
import matplotlib.pyplot as plt
import numpy as np
# 加载图像
image1 = cv2.imread('../2.png')
# 将训练图像转换为RGB
training_image = cv2.cvtColor(image1, cv2.COLOR_BGR2RGB)
# 将训练图像转换为灰度
training_gray = cv2.cvtColor(training_image, cv2.COLOR_RGB2GRAY)
# 通过添加缩放不变性和旋转不变性来创建测试图像
test_image = training_image
num_rows, num_cols = test_image.shape[:2]
rotation_matrix = cv2.getRotationMatrix2D((num_cols/2, num_rows/2), 30, 1)
test_image = cv2.warpAffine(test_image, rotation_matrix, (num_cols, num_rows))
test_gray = cv2.cvtColor(test_image, cv2.COLOR_RGB2GRAY)
# 显示训练图像和测试图像
fx, plots = plt.subplots(1, 2, figsize=(20,10))
plots[0].set_title("Training Image")
plots[0].imshow(training_image)
plots[0].axis("off")
plt.imsave("training_image.jpg", training_image)
plots[1].set_title("Testing Image")
plots[1].imshow(test_image)
plots[1].axis("off")
plt.imsave("test_image.jpg", test_image)
orb = cv2.ORB_create()
train_keypoints, train_descriptor = orb.detectAndCompute(training_gray, None)
test_keypoints, test_descriptor = orb.detectAndCompute(test_gray, None)
keypoints_without_size = np.copy(training_image)
keypoints_with_size = np.copy(training_image)
cv2.drawKeypoints(training_image, train_keypoints, keypoints_without_size, color = (0, 255, 0))
cv2.drawKeypoints(training_image, train_keypoints, keypoints_with_size, flags = cv2.DRAW_MATCHES_FLAGS_DRAW_RICH_KEYPOINTS)
# 显示图像有和没有关键点大小
fx, plots = plt.subplots(1, 2, figsize=(20,10))
plots[0].set_title("Train keypoints With Size")
plots[0].imshow(keypoints_with_size, cmap='gray')
plots[0].axis("off")
plt.imsave("keypoints_with_size.jpg", keypoints_with_size)
plots[1].set_title("Train keypoints Without Size")
plots[1].imshow(keypoints_without_size, cmap='gray')
plots[1].axis("off")
plt.imsave("keypoints_without_size.jpg", keypoints_without_size)
# 打印训练图像中检测到的关键点个数
print("Number of Keypoints Detected In The Training Image: ", len(train_keypoints))
# 打印查询图像中检测到的关键点数量
print("Number of Keypoints Detected In The Query Image: ", len(test_keypoints))
# 创建一个Brute Force Matcher对象。
bf = cv2.BFMatcher(cv2.NORM_HAMMING, crossCheck = True)
# 执行训练图像和测试图像的ORB描述符之间的匹配
matches = bf.match(train_descriptor, test_descriptor)
# 距离较短的是我们想要的。
matches = sorted(matches, key = lambda x : x.distance)
result = cv2.drawMatches(training_image, train_keypoints, test_gray, test_keypoints, matches, test_gray, flags = 2)
# 显示最佳匹配点
plt.rcParams['figure.figsize'] = [14.0, 7.0]
plt.title('Best Matching Points')
plt.imsave("result.jpg", result)
plt.imshow(result)
plt.show()
# 打印训练图像和查询图像之间的匹配点总数
print("\nNumber of Matching Keypoints Between The Training and Query Images: ", len(matches))
# Number of Keypoints Detected In The Training Image: 500
# Number of Keypoints Detected In The Query Image: 500
#
# Number of Matching Keypoints Between The Training and Query Images: 325
输入图片
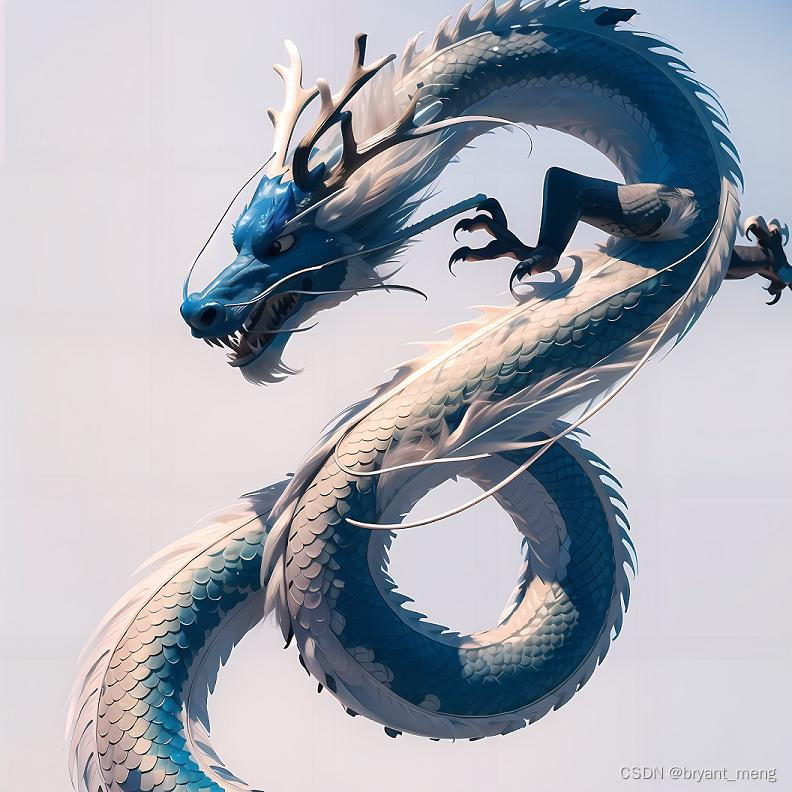
输出
test_image.jpg,这次实验测试图片和原图的大小保持了一致
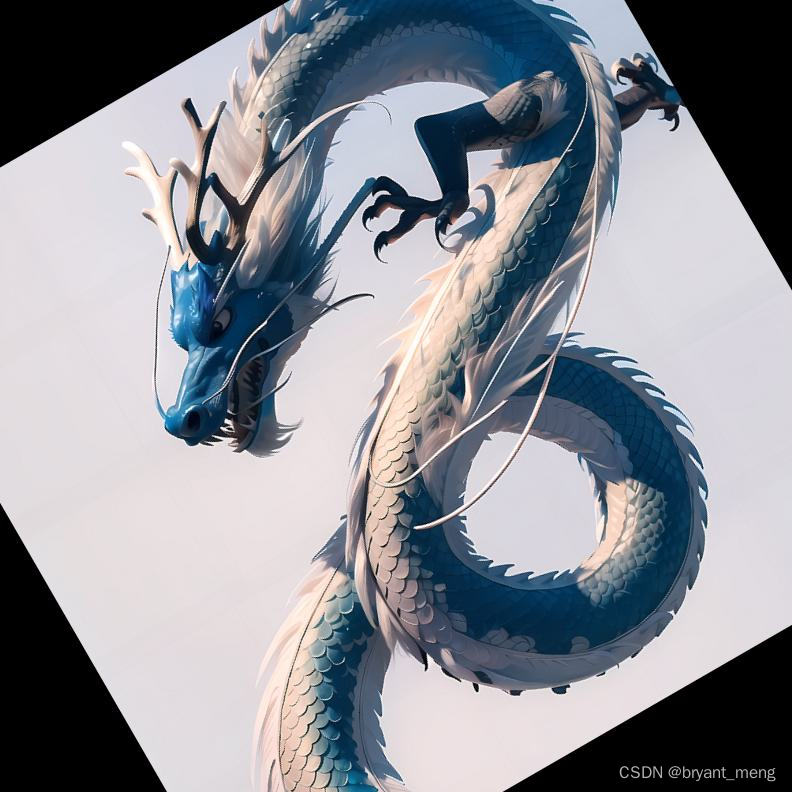
keypoints_with_size.jpg
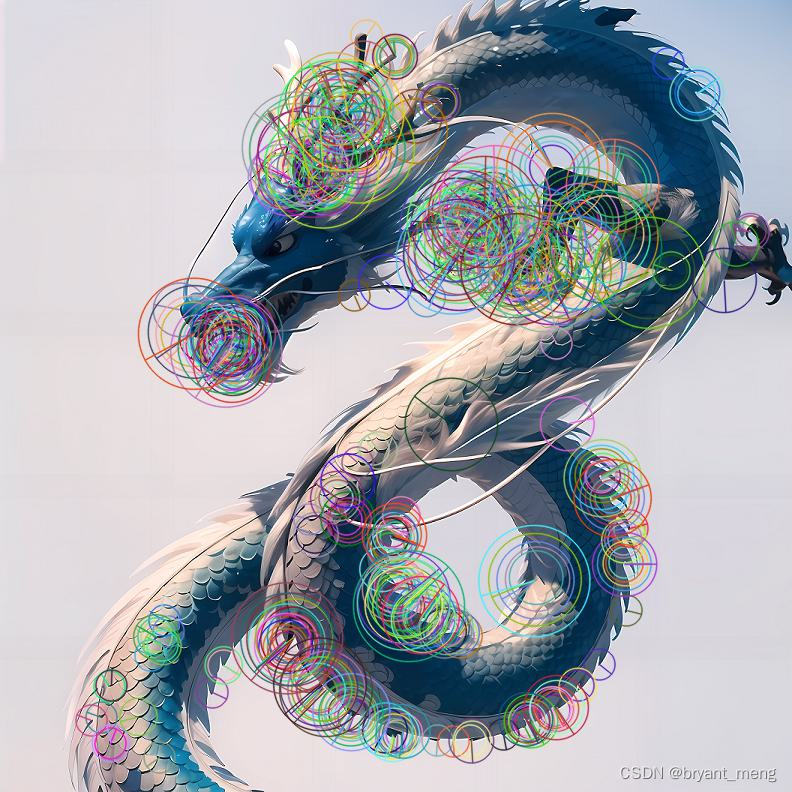
keypoints_without_size.jpg
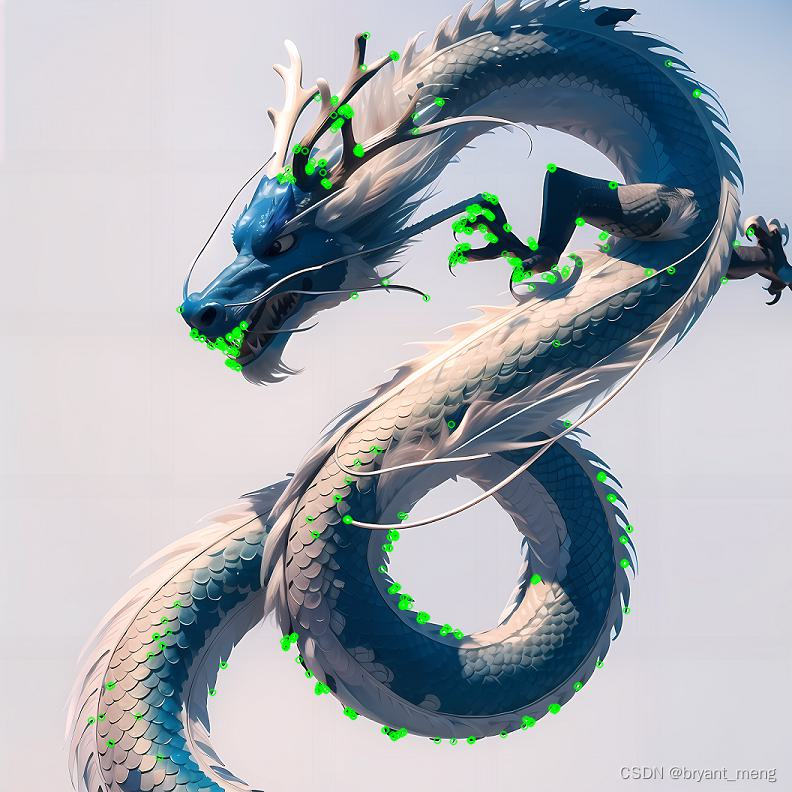
result.jpg------ORB+Brute Force Matcher
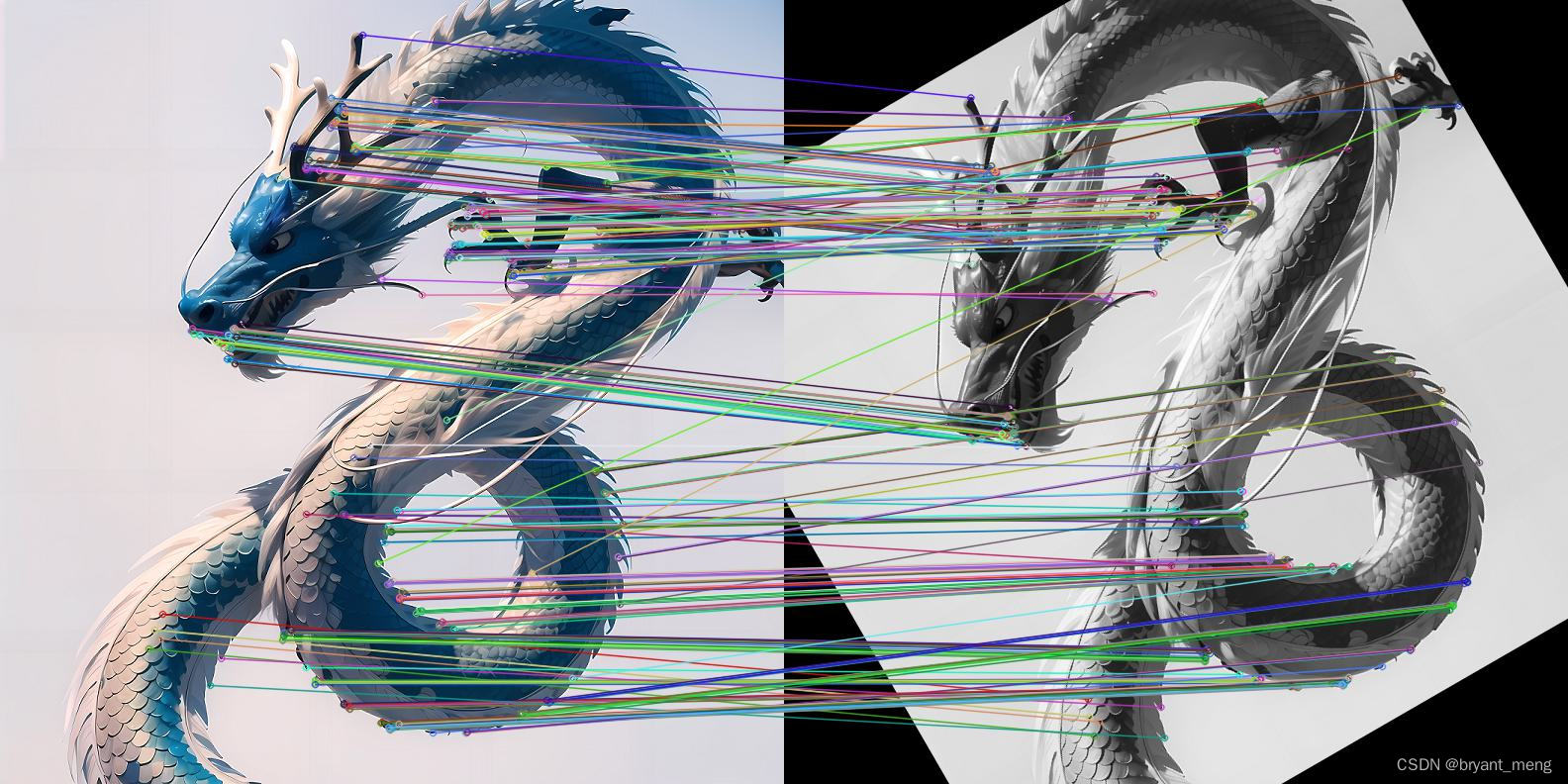
7 SIFT(Scale Invariant Feature Transform) 特征描述子
测试图片 4 倍下采样了
python
#!/usr/bin/python3
# -*- encoding: utf-8 -*-
"""
@File : sift.py
@Time : 2021/10/13 13:01
@Author : David
@Software: PyCharm
"""
# SIFT (Scale-Invariant Feature Transform)
# 导入库函数与显示图像
import cv2
import matplotlib.pyplot as plt
import numpy as np
# 导入图像
image1 = cv2.imread('../2.png')
# 将训练图像转换为RGB
training_image = cv2.cvtColor(image1, cv2.COLOR_BGR2RGB)
# 将训练图像转换为灰度
training_gray = cv2.cvtColor(training_image, cv2.COLOR_RGB2GRAY)
# 通过添加缩放不变性和旋转不变性来创建测试图像
test_image = cv2.pyrDown(training_image)
test_image = cv2.pyrDown(test_image)
num_rows, num_cols = test_image.shape[:2]
rotation_matrix = cv2.getRotationMatrix2D((num_cols / 2, num_rows / 2), 30, 1)
test_image = cv2.warpAffine(test_image, rotation_matrix, (num_cols, num_rows))
test_gray = cv2.cvtColor(test_image, cv2.COLOR_RGB2GRAY)
# 显示训练图像和测试图像
fx, plots = plt.subplots(1, 2, figsize=(20, 10))
plots[0].set_title("Training Image")
plots[0].imshow(training_image)
plots[0].axis("off")
plt.imsave("training_image.jpg", training_image)
plots[1].set_title("Testing Image")
plots[1].imshow(test_image)
plots[1].axis("off")
plt.imsave("test_image.jpg", test_image)
# 检测关键点并创建描述符
sift = cv2.xfeatures2d.SIFT_create()
train_keypoints, train_descriptor = sift.detectAndCompute(training_gray, None)
test_keypoints, test_descriptor = sift.detectAndCompute(test_gray, None)
keypoints_without_size = np.copy(training_image)
keypoints_with_size = np.copy(training_image)
cv2.drawKeypoints(training_image, train_keypoints, keypoints_without_size, color=(0, 255, 0))
cv2.drawKeypoints(training_image, train_keypoints, keypoints_with_size,
flags=cv2.DRAW_MATCHES_FLAGS_DRAW_RICH_KEYPOINTS)
# 显示图像有和没有关键点大小
fx, plots = plt.subplots(1, 2, figsize=(20, 10))
plots[0].set_title("Train keypoints With Size")
plots[0].imshow(keypoints_with_size, cmap='gray')
plots[0].axis("off")
plt.imsave("keypoints_with_size.jpg", keypoints_with_size)
plots[1].set_title("Train keypoints Without Size")
plots[1].imshow(keypoints_without_size, cmap='gray')
plots[1].axis("off")
plt.imsave("keypoints_without_size.jpg", keypoints_without_size)
# 打印训练图像中检测到的关键点个数
print("Number of Keypoints Detected In The Training Image: ", len(train_keypoints))
# 打印查询图像中检测到的关键点数量
print("Number of Keypoints Detected In The Query Image: ", len(test_keypoints))
# 关键点匹配
# 创建一个Brute Force Matcher对象。
bf = cv2.BFMatcher(cv2.NORM_L1, crossCheck=False)
# 对训练图像和测试图像的SIFT描述子进行匹配
matches = bf.match(train_descriptor, test_descriptor)
# 距离较短的是我们想要的。
matches = sorted(matches, key=lambda x: x.distance)
result = cv2.drawMatches(training_image, train_keypoints, test_gray, test_keypoints, matches, test_gray, flags=2)
# 显示最佳匹配点
plt.rcParams['figure.figsize'] = [14.0, 7.0]
plt.title('Best Matching Points')
plt.imsave("result.jpg", result)
plt.imshow(result)
plt.show()
# 打印训练图像和查询图像之间的匹配点总数
print("\nNumber of Matching Keypoints Between The Training and Query Images: ", len(matches))
# Number of Keypoints Detected In The Training Image: 1080
# Number of Keypoints Detected In The Query Image: 245
#
# Number of Matching Keypoints Between The Training and Query Images: 1080
输出
keypoints_with_size.jpg
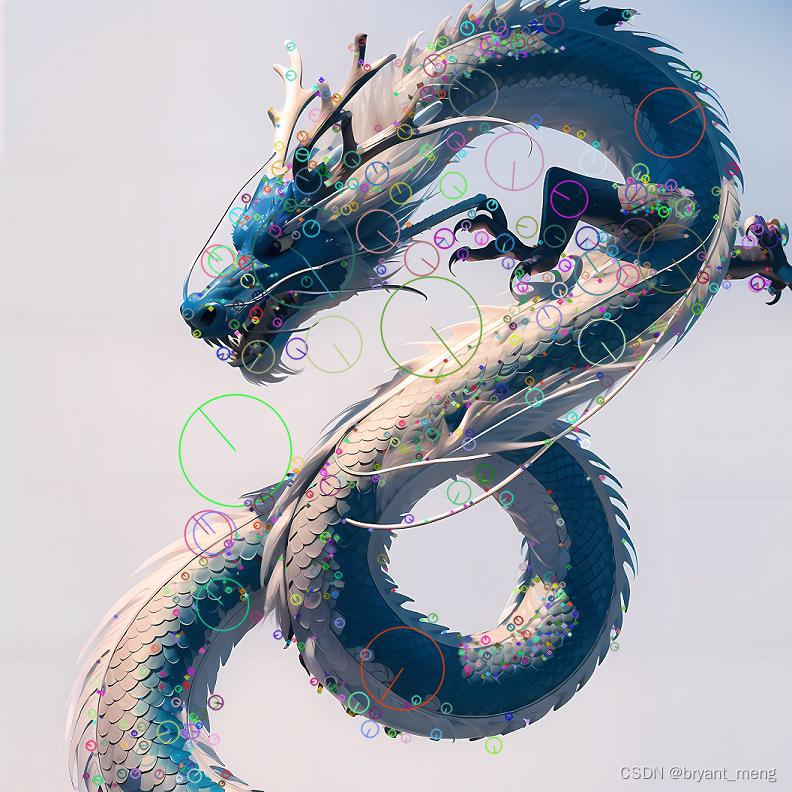
keypoints_without_size.jpg
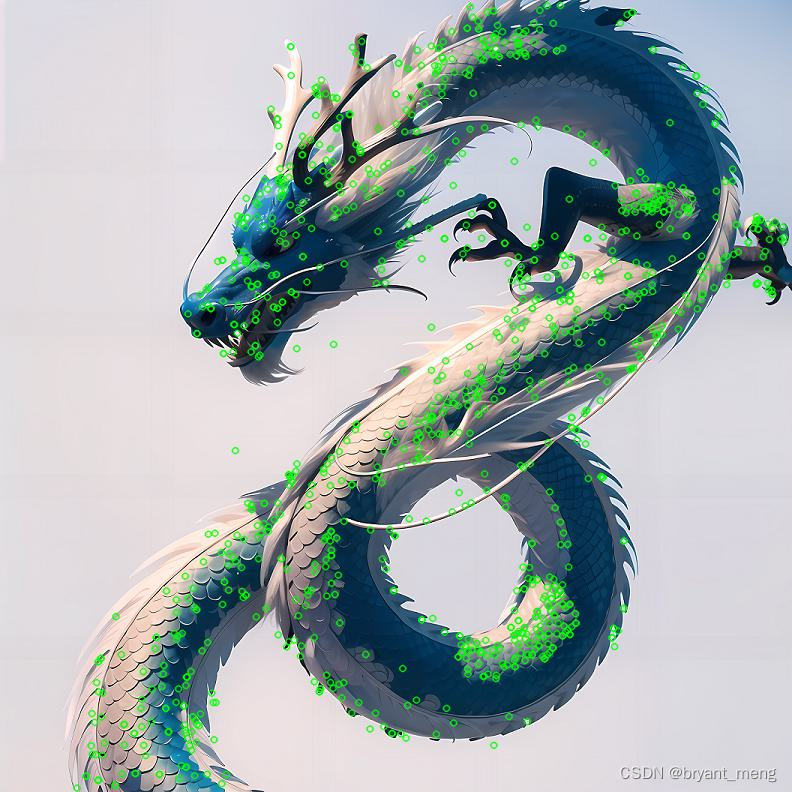
result.jpg------SIFT+Brute Force Matcher
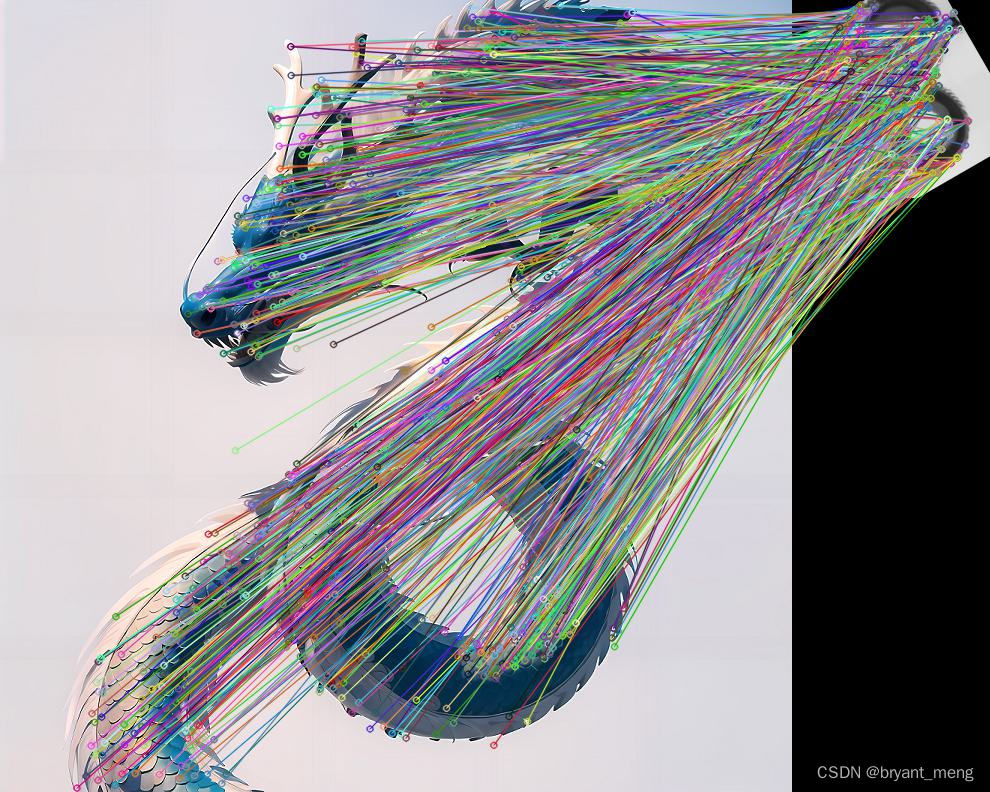
8 SURF(Speeded Up Robust Features) 特征描述子
较新的 opencv 版本不支持 SURF,这里仅展示 Code
python
#!/usr/bin/python3
# -*- encoding: utf-8 -*-
"""
@File : surf.py
# SURF (Speeded-Up Robust Features)
# 新版OpenCV不能使用Surf了,我使用了opencv-contrib-python==3.4.11.45
"""
# 导入库和显示图像
import cv2
import matplotlib.pyplot as plt
import numpy as np
# 加载图像
image1 = cv2.imread('./1.jpg')
# 将训练图像转换为RGB
training_image = cv2.cvtColor(image1, cv2.COLOR_BGR2RGB)
# 将训练图像转换为灰度
training_gray = cv2.cvtColor(training_image, cv2.COLOR_RGB2GRAY)
# 通过添加缩放不变性和旋转不变性来创建测试图像
test_image = cv2.pyrDown(training_image)
test_image = cv2.pyrDown(test_image)
num_rows, num_cols = test_image.shape[:2]
rotation_matrix = cv2.getRotationMatrix2D((num_cols / 2, num_rows / 2), 30, 1)
test_image = cv2.warpAffine(test_image, rotation_matrix, (num_cols, num_rows))
test_gray = cv2.cvtColor(test_image, cv2.COLOR_RGB2GRAY)
# 显示训练图像和测试图像
fx, plots = plt.subplots(1, 2, figsize=(20, 10))
plots[0].set_title("Training Image")
plots[0].imshow(training_image)
plots[0].axis("off")
plt.imsave("training_image.jpg", training_image)
plots[1].set_title("Testing Image")
plots[1].imshow(test_image)
plots[1].axis("off")
plt.imsave("test_image.jpg", test_image)
# 检测关键点并创建描述符
surf = cv2.xfeatures2d.SURF_create(800)
train_keypoints, train_descriptor = surf.detectAndCompute(training_gray, None)
test_keypoints, test_descriptor = surf.detectAndCompute(test_gray, None)
keypoints_without_size = np.copy(training_image)
keypoints_with_size = np.copy(training_image)
cv2.drawKeypoints(training_image, train_keypoints, keypoints_without_size, color=(0, 255, 0))
cv2.drawKeypoints(training_image, train_keypoints, keypoints_with_size,
flags=cv2.DRAW_MATCHES_FLAGS_DRAW_RICH_KEYPOINTS)
# 显示图像有和没有关键点大小
fx, plots = plt.subplots(1, 2, figsize=(20, 10))
plots[0].set_title("Train keypoints With Size")
plots[0].imshow(keypoints_with_size, cmap='gray')
plots[0].axis("off")
plt.imsave("keypoints_with_size.jpg", keypoints_with_size)
plots[1].set_title("Train keypoints Without Size")
plots[1].imshow(keypoints_without_size, cmap='gray')
plots[1].axis("off")
plt.imsave("keypoints_without_size.jpg", keypoints_without_size)
# 打印训练图像中检测到的关键点个数
print("Number of Keypoints Detected In The Training Image: ", len(train_keypoints))
# 打印查询图像中检测到的关键点数量
print("Number of Keypoints Detected In The Query Image: ", len(test_keypoints))
# 关键点匹配
# 创建一个Brute Force Matcher对象。
bf = cv2.BFMatcher(cv2.NORM_L1, crossCheck=True)
# 对训练图像和测试图像的SURF描述符进行匹配
matches = bf.match(train_descriptor, test_descriptor)
# 距离较短的是我们想要的。
matches = sorted(matches, key=lambda x: x.distance)
result = cv2.drawMatches(training_image, train_keypoints, test_gray, test_keypoints, matches, test_gray, flags=2)
# 显示最佳匹配点
plt.rcParams['figure.figsize'] = [14.0, 7.0]
plt.title('Best Matching Points')
plt.imsave("result.jpg", result)
plt.imshow(result)
plt.show()
# 打印训练图像和查询图像之间的匹配点总数
print("\nNumber of Matching Keypoints Between The Training and Query Images: ", len(matches))
# Number of Keypoints Detected In The Training Image: 242
# Number of Keypoints Detected In The Query Image: 29
#
# Number of Matching Keypoints Between The Training and Query Images: 21
9 Brute-Force Matcher 特征匹配
python
import cv2
cv_img1 = cv2.imread('1.jpg', 1)
cv_img2 = cv2.imread('2.jpg', 1)
orb = cv2.ORB_create(nfeatures=500)
kp1, des1 = orb.detectAndCompute(cv_img1, None)
kp2, des2 = orb.detectAndCompute(cv_img2, None)
# matcher接受normType,它被设置为cv2.NORM_L2用于SIFT和SURF, cv2.NORM_HAMMING用于ORB, FAST and BRIEF
bf = cv2.BFMatcher(cv2.NORM_HAMMING, crossCheck=True)
matches = bf.match(des1, des2)
matches = sorted(matches, key=lambda x: x.distance) # 前50匹配点
match_img = cv2.drawMatches(cv_img1, kp1, cv_img2, kp2, matches[:50], None)
cv2.imshow('Matches', match_img)
cv2.imwrite("Match.jpg", match_img)
cv2.waitKey()
输入 1.jpg
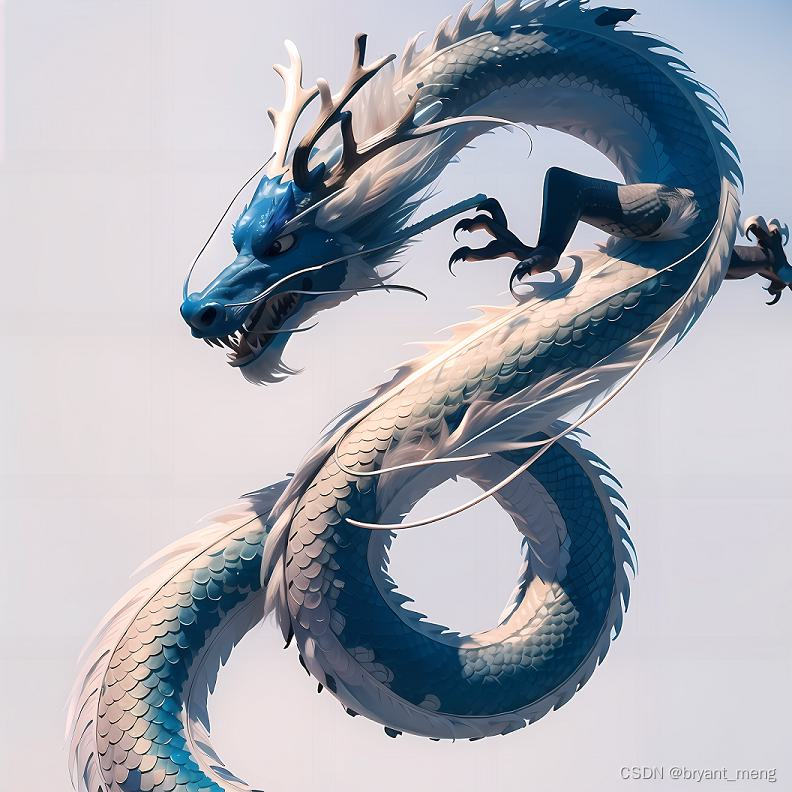
输入 2.jpg
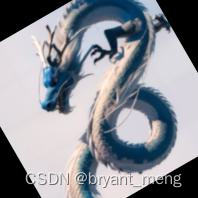
输出
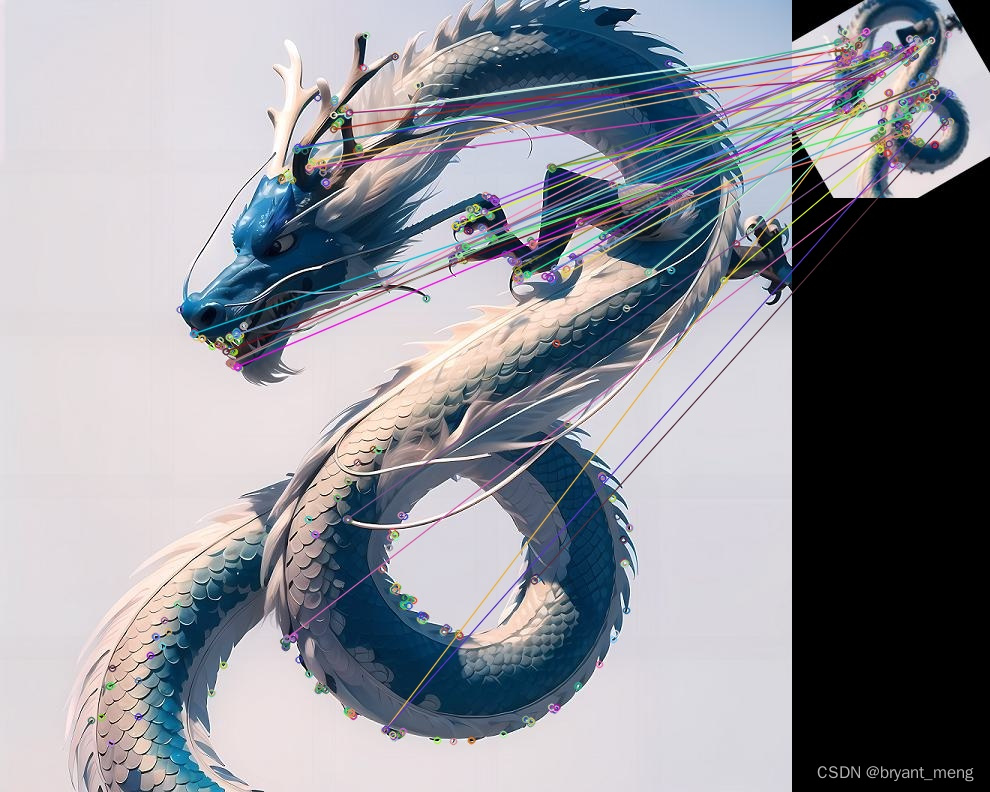
10 FLANN Matcher 特征匹配
使用 ORB 检测器和校正失真图像来实现匹配操作。
python
import cv2
import numpy as np
def get_corrected_img(cv_img1, cv_img2):
MIN_MATCHES = 10
orb = cv2.ORB_create(nfeatures=500)
kp1, des1 = orb.detectAndCompute(cv_img1, None)
kp2, des2 = orb.detectAndCompute(cv_img2, None)
index_params = dict(algorithm=6,
table_number=6,
key_size=12,
multi_probe_level=2)
search_params = {}
flann = cv2.FlannBasedMatcher(index_params, search_params)
matches = flann.knnMatch(des1, des2, k=2)
# 根据 Lowe 的比率测试来过滤良好的匹配
good_matches = []
for m, n in matches:
if m.distance < 0.8 * n.distance:
good_matches.append(m)
if len(good_matches) > MIN_MATCHES:
src_points = np.float32([kp1[m.queryIdx].pt for m in good_matches]).reshape(-1, 1, 2)
dst_points = np.float32([kp2[m.trainIdx].pt for m in good_matches]).reshape(-1, 1, 2)
print(src_points.shape)
good_matches = sorted(good_matches, key=lambda x: x.distance) # 前50匹配点
match_img = cv2.drawMatches(cv_img1, kp1, cv_img2, kp2, good_matches[:50], None)
cv2.imshow('flannMatches', match_img)
cv2.imwrite("flannMatch.jpg", match_img)
cv2.waitKey()
m, mask = cv2.findHomography(src_points, dst_points, cv2.RANSAC, 5.0)
corrected_img = cv2.warpPerspective(cv_img1, m, (cv_img2.shape[1], cv_img2.shape[0]))
return corrected_img
return None
if __name__ == "__main__":
cv_im1 = cv2.imread('1.jpg')
cv_im2 = cv2.imread('2.jpg')
img = get_corrected_img(cv_im2, cv_im1)
if img is not None:
cv2.imshow('Corrected image', img)
cv2.imwrite("corrected_image.jpg", img)
cv2.waitKey()
输入图片
corrected_image.jpg
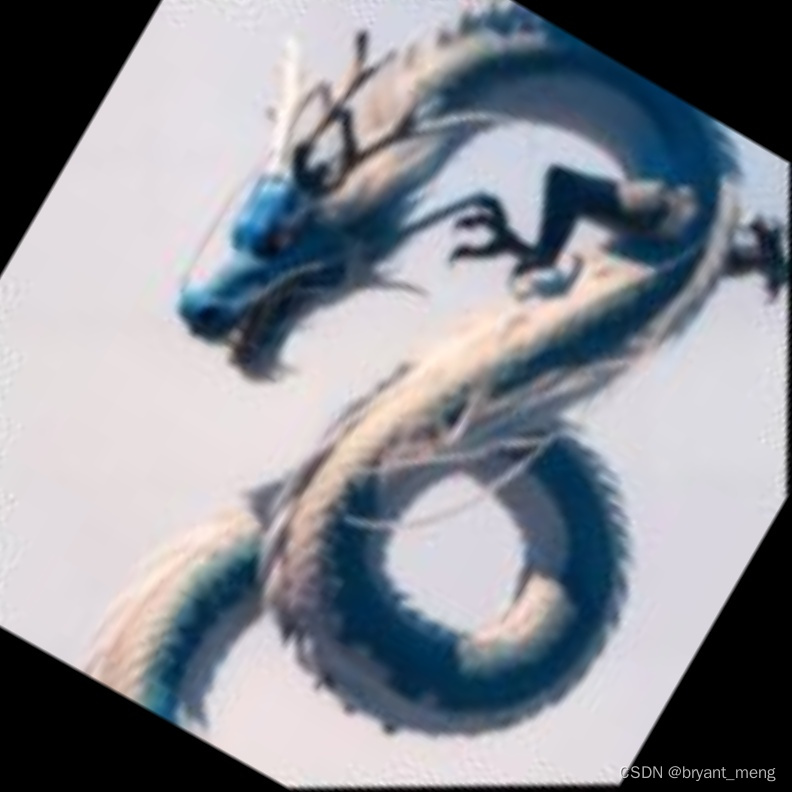
flannMatch.jpg
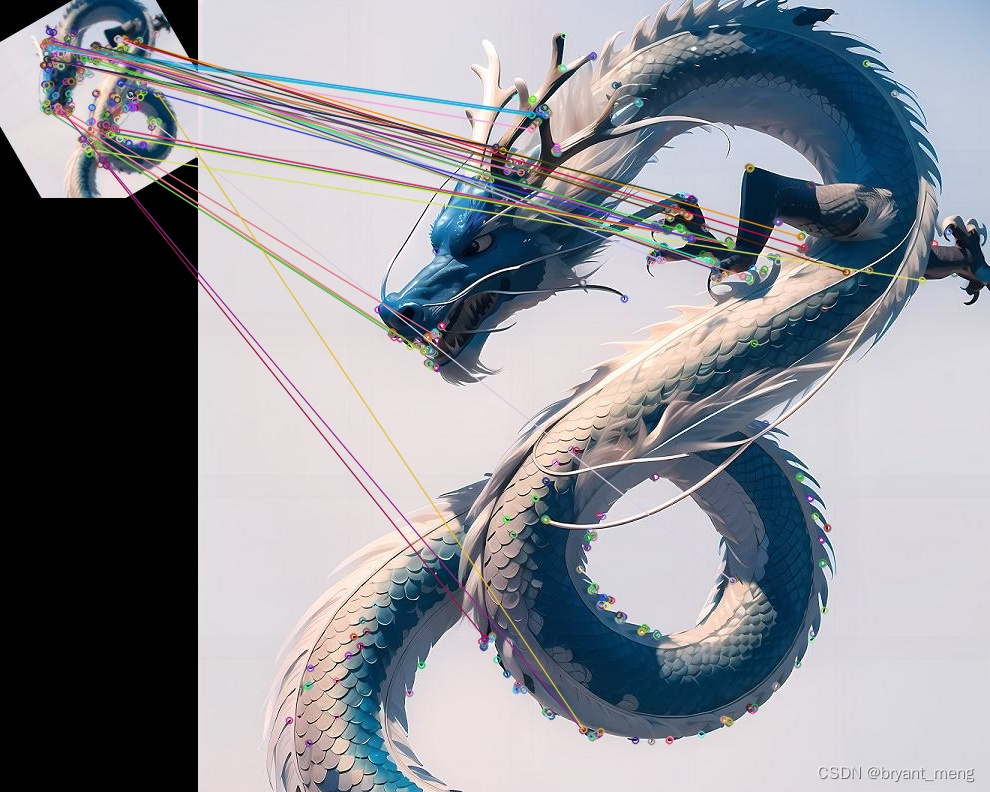