一. LightWeight概述
light weight openpose是openpose的简化版本,使用了openpose的大体流程。
Light weight openpose和openpose的区别是:
a 前者使用的是Mobilenet V1(到conv5_5),后者使用的是Vgg19(前10层)。
b 前者部分层使用了空洞卷积(dilated convolution)来提升感受视野,后者使用一般的卷积。
c 前者卷积核大小为3*3,后者为7*7。
d 前者只有一个refine stage,后者有5个stage。
e 前者的initial stage和refine stage里面的两个分支(hotmaps和pafs)使用权值共享,后者则是并行的两个分支
二. LightWeight的网络结构
openpose的每个stage使用下图中左侧的两个并行的分支,分别预测hotmaps和pafs,为了进一步降低计算量,light weight openpose中将前几层进行权值共享,如下图右侧所示。
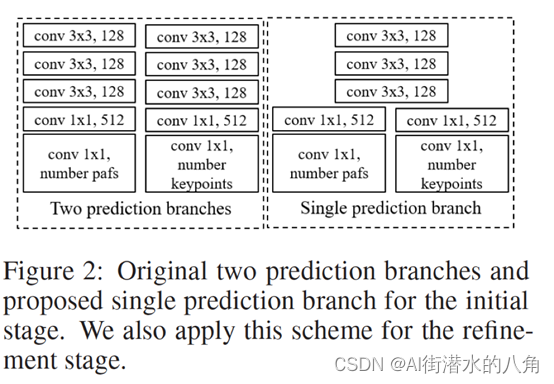
其网络流程:
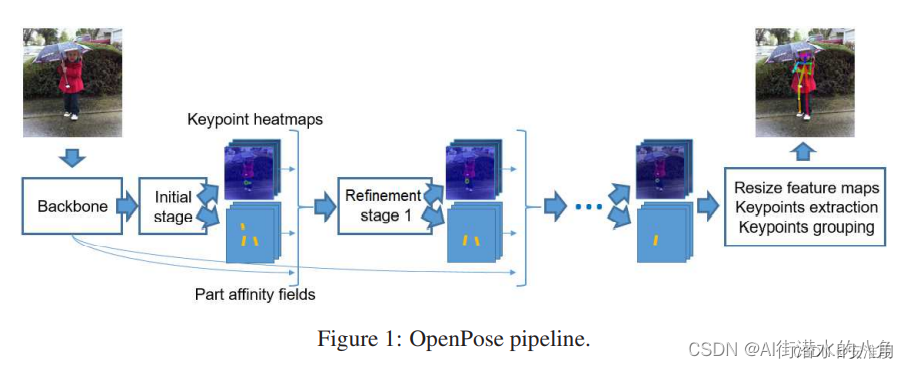
三. LightWeight的网络结构代码
python
import torch
from torch import nn
from modules.conv import conv, conv_dw, conv_dw_no_bn
class Cpm(nn.Module):
def __init__(self, in_channels, out_channels):
super().__init__()
self.align = conv(in_channels, out_channels, kernel_size=1, padding=0, bn=False)
self.trunk = nn.Sequential(
conv_dw_no_bn(out_channels, out_channels),
conv_dw_no_bn(out_channels, out_channels),
conv_dw_no_bn(out_channels, out_channels)
)
self.conv = conv(out_channels, out_channels, bn=False)
def forward(self, x):
x = self.align(x)
x = self.conv(x + self.trunk(x))
return x
class InitialStage(nn.Module):
def __init__(self, num_channels, num_heatmaps, num_pafs):
super().__init__()
self.trunk = nn.Sequential(
conv(num_channels, num_channels, bn=False),
conv(num_channels, num_channels, bn=False),
conv(num_channels, num_channels, bn=False)
)
self.heatmaps = nn.Sequential(
conv(num_channels, 512, kernel_size=1, padding=0, bn=False),
conv(512, num_heatmaps, kernel_size=1, padding=0, bn=False, relu=False)
)
self.pafs = nn.Sequential(
conv(num_channels, 512, kernel_size=1, padding=0, bn=False),
conv(512, num_pafs, kernel_size=1, padding=0, bn=False, relu=False)
)
def forward(self, x):
trunk_features = self.trunk(x)
heatmaps = self.heatmaps(trunk_features)
pafs = self.pafs(trunk_features)
return [heatmaps, pafs]
class RefinementStageBlock(nn.Module):
def __init__(self, in_channels, out_channels):
super().__init__()
self.initial = conv(in_channels, out_channels, kernel_size=1, padding=0, bn=False)
self.trunk = nn.Sequential(
conv(out_channels, out_channels),
conv(out_channels, out_channels, dilation=2, padding=2)
)
def forward(self, x):
initial_features = self.initial(x)
trunk_features = self.trunk(initial_features)
return initial_features + trunk_features
class RefinementStage(nn.Module):
def __init__(self, in_channels, out_channels, num_heatmaps, num_pafs):
super().__init__()
self.trunk = nn.Sequential(
RefinementStageBlock(in_channels, out_channels),
RefinementStageBlock(out_channels, out_channels),
RefinementStageBlock(out_channels, out_channels),
RefinementStageBlock(out_channels, out_channels),
RefinementStageBlock(out_channels, out_channels)
)
self.heatmaps = nn.Sequential(
conv(out_channels, out_channels, kernel_size=1, padding=0, bn=False),
conv(out_channels, num_heatmaps, kernel_size=1, padding=0, bn=False, relu=False)
)
self.pafs = nn.Sequential(
conv(out_channels, out_channels, kernel_size=1, padding=0, bn=False),
conv(out_channels, num_pafs, kernel_size=1, padding=0, bn=False, relu=False)
)
def forward(self, x):
trunk_features = self.trunk(x)
heatmaps = self.heatmaps(trunk_features)
pafs = self.pafs(trunk_features)
return [heatmaps, pafs]
class PoseEstimationWithMobileNet(nn.Module):
def __init__(self, num_refinement_stages=1, num_channels=128, num_heatmaps=19, num_pafs=38):
super().__init__()
self.model = nn.Sequential(
conv( 3, 32, stride=2, bias=False),
conv_dw( 32, 64),
conv_dw( 64, 128, stride=2),
conv_dw(128, 128),
conv_dw(128, 256, stride=2),
conv_dw(256, 256),
conv_dw(256, 512), # conv4_2
conv_dw(512, 512, dilation=2, padding=2),
conv_dw(512, 512),
conv_dw(512, 512),
conv_dw(512, 512),
conv_dw(512, 512) # conv5_5
)
self.cpm = Cpm(512, num_channels)
self.initial_stage = InitialStage(num_channels, num_heatmaps, num_pafs)
self.refinement_stages = nn.ModuleList()
for idx in range(num_refinement_stages):
self.refinement_stages.append(RefinementStage(num_channels + num_heatmaps + num_pafs, num_channels,
num_heatmaps, num_pafs))
def forward(self, x):
backbone_features = self.model(x)
backbone_features = self.cpm(backbone_features)
stages_output = self.initial_stage(backbone_features)
for refinement_stage in self.refinement_stages:
stages_output.extend(
refinement_stage(torch.cat([backbone_features, stages_output[-2], stages_output[-1]], dim=1)))
return stages_output
四. LightWeight是怎么去识别跌倒呢
LightWeight可以检测到人体的关键点,所以可以通过两种方式来判断是否跌倒,第一种方法是通过计算角度,第二种方式,是通过判断整体的关键点(把抠出的关键点图送入到分类网络),本文的做法是第二种方式
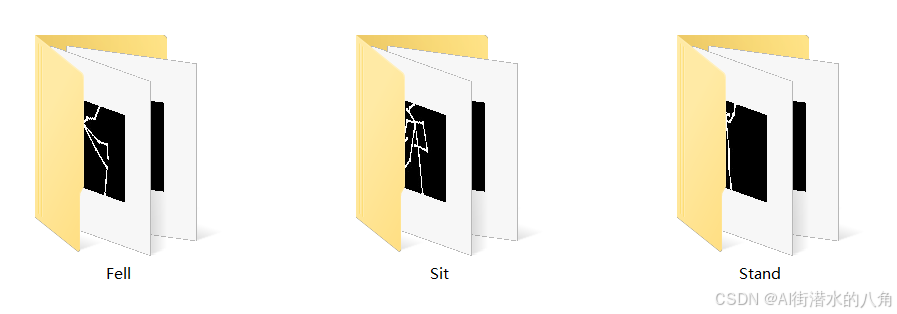
五. LightWeight的演示效果(具有图片和摄像头识别功能)
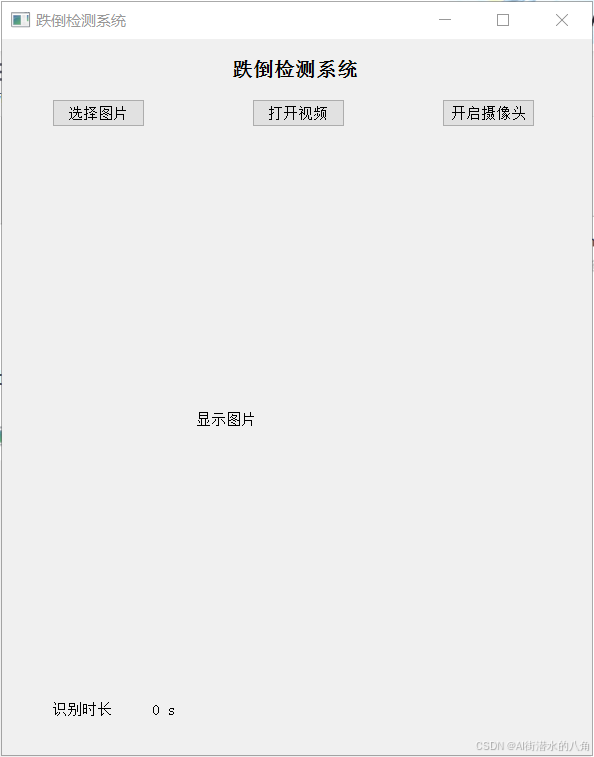
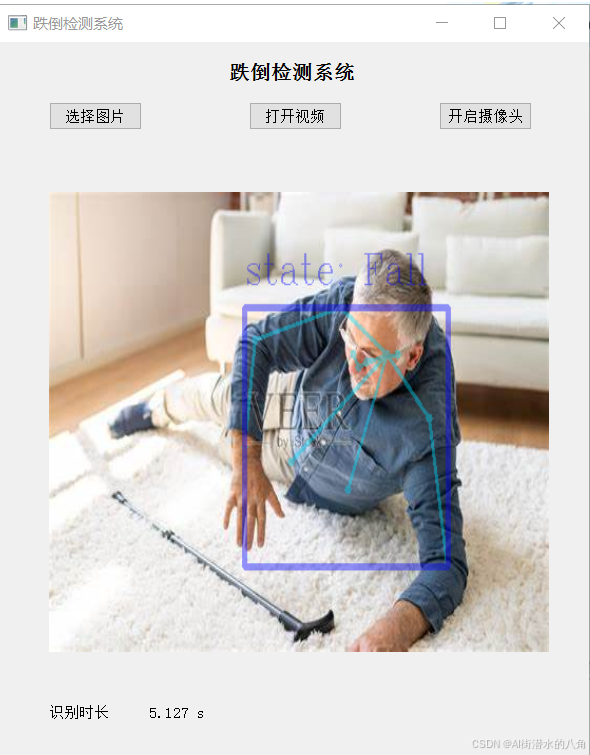
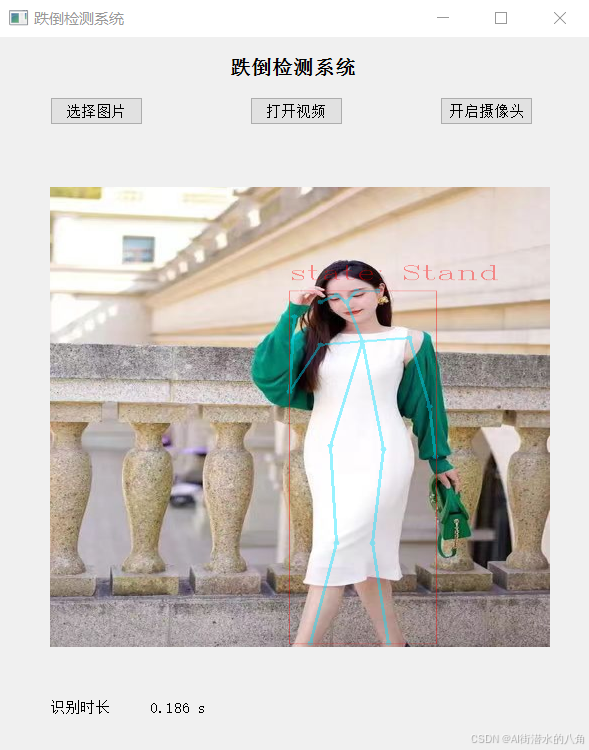
六. 整个工程的内容
提供源代码,模型,提供GUI界面代码
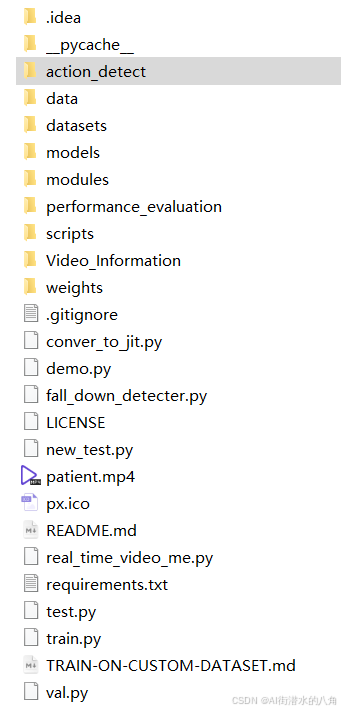
代码的下载路径 (新窗口打开链接): 基于深度学习LightWeight的人体姿态检测跌倒系统源码
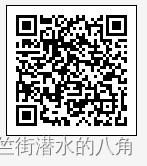
有问题可以私信或者留言,有问必答