模块出处
ICCV 23\] [\[link\]](https://openaccess.thecvf.com/content/ICCV2023/html/Lin_Scale-Aware_Modulation_Meet_Transformer_ICCV_2023_paper.html) [\[code\]](https://github.com/AFeng-x/SMT) Scale-Aware Modulation Meet Transformer *** ** * ** *** ##### 模块名称 Scale-Aware Modulation (SAM) *** ** * ** *** ##### 模块作用 改进的自注意力 *** ** * ** *** ##### 模块结构 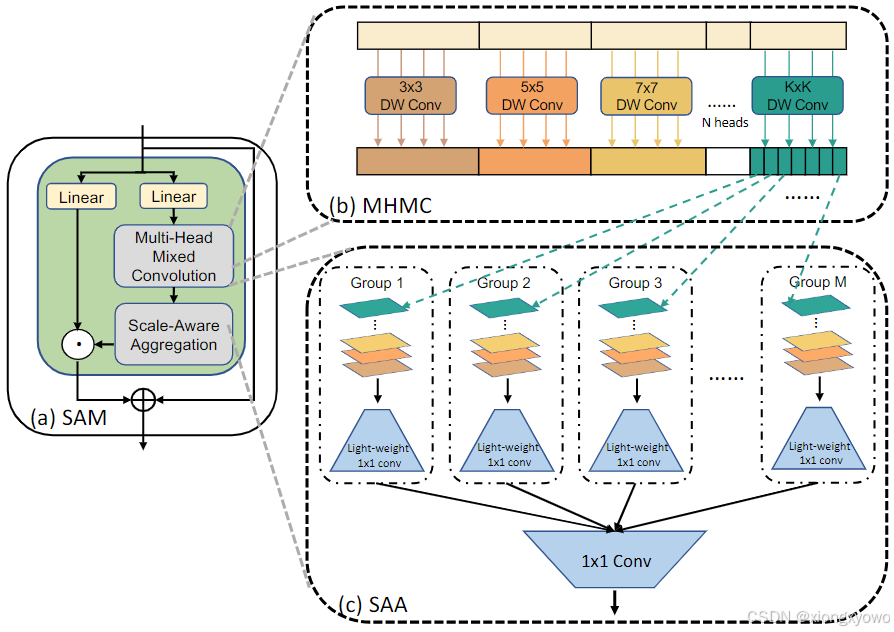 *** ** * ** *** ##### 模块代码 ```python3 import torch import torch.nn as nn import torch.nn.functional as F class SAM(nn.Module): def __init__(self, dim, ca_num_heads=4, sa_num_heads=8, qkv_bias=False, qk_scale=None, attn_drop=0., proj_drop=0., expand_ratio=2): super().__init__() self.ca_attention = 1 self.dim = dim self.ca_num_heads = ca_num_heads self.sa_num_heads = sa_num_heads assert dim % ca_num_heads == 0, f"dim {dim} should be divided by num_heads {ca_num_heads}." assert dim % sa_num_heads == 0, f"dim {dim} should be divided by num_heads {sa_num_heads}." self.act = nn.GELU() self.proj = nn.Linear(dim, dim) self.proj_drop = nn.Dropout(proj_drop) self.split_groups=self.dim//ca_num_heads self.v = nn.Linear(dim, dim, bias=qkv_bias) self.s = nn.Linear(dim, dim, bias=qkv_bias) for i in range(self.ca_num_heads): local_conv = nn.Conv2d(dim//self.ca_num_heads, dim//self.ca_num_heads, kernel_size=(3+i*2), padding=(1+i), stride=1, groups=dim//self.ca_num_heads) setattr(self, f"local_conv_{i + 1}", local_conv) self.proj0 = nn.Conv2d(dim, dim*expand_ratio, kernel_size=1, padding=0, stride=1, groups=self.split_groups) self.bn = nn.BatchNorm2d(dim*expand_ratio) self.proj1 = nn.Conv2d(dim*expand_ratio, dim, kernel_size=1, padding=0, stride=1) def forward(self, x, H, W): # In B, N, C = x.shape v = self.v(x) s = self.s(x).reshape(B, H, W, self.ca_num_heads, C//self.ca_num_heads).permute(3, 0, 4, 1, 2) # Multi-Head Mixed Convolution for i in range(self.ca_num_heads): local_conv = getattr(self, f"local_conv_{i + 1}") s_i= s[i] s_i = local_conv(s_i).reshape(B, self.split_groups, -1, H, W) if i == 0: s_out = s_i else: s_out = torch.cat([s_out,s_i],2) s_out = s_out.reshape(B, C, H, W) # Scale-Aware Aggregation (SAA) s_out = self.proj1(self.act(self.bn(self.proj0(s_out)))) self.modulator = s_out s_out = s_out.reshape(B, C, N).permute(0, 2, 1) x = s_out * v # Out x = self.proj(x) x = self.proj_drop(x) return x if __name__ == '__main__': x = torch.randn([3, 1024, 256]) # B, N, C sam = SAM(dim=256) out = sam(x, H=32, W=32) # H=N*W print(out.shape) # 3, 1024, 256 ``` *** ** * ** *** ##### 原文表述 我们提出了一种新颖的卷积调制,称为尺度感知调制 (SAM),它包含两个新模块:多头混合卷积 (MHMC) 和尺度感知聚合 (SAA)。MHMC 模块旨在增强感受野并同时捕获多尺度特征。SAA 模块旨在有效地聚合不同头部之间的特征,同时保持轻量级架构。