目录
一、SG网络功能介绍
DiAD论文最主要的创新点就是使用SG网络解决多类别异常检测中的语义信息丢失问题,那么它是怎么实现的保留原始图像语义信息的同时重建异常区域?
与稳定扩散去噪网络的连接: SG网络被设计为与稳定扩散(Stable Diffusion, SD)去噪网络相连接。SD去噪网络本身具有强大的图像生成能力,但可能无法在多类异常检测任务中保持图像的语义信息一致性。SG网络通过引入语义引导机制,使得在重构异常区域时能够参考并保留原始图像的语义上下文。整个框架图中,SG网络与去噪网络的连接如下图所示。
这是论文给出的最终输出,我认为图中圈出来的地方有问题,应该改为SG网络的编码器才对。
语义一致性保持: SG网络在重构过程中,通过在不同尺度下处理噪声,并利用空间感知特征融合(Spatial-aware Feature Fusion, SFF)块融合特征,确保重建过程中保留语义信息。这样,即使在重构异常区域时,也能使修复后的区域与原始图像的语义上下文保持一致。
多尺度特征融合: SFF块将高尺度的语义信息集成到低尺度中,使得在保留原始正常样本信息的同时,能够处理大规模异常区域的重建。这种机制有助于在处理需要广泛重构的区域时,最大化重构的准确性,同时保持图像的语义一致性。从下图中可以看到,特征融合模块还是很好理解的。
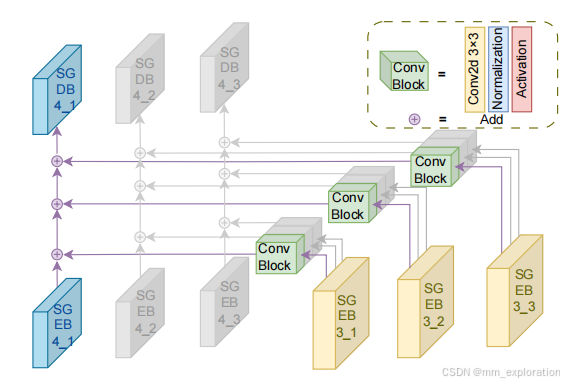
与预训练特征提取器的结合: SG网络还与特征空间中的预训练特征提取器相结合。预训练特征提取器能够处理输入图像和重建图像,并在不同尺度上提取特征。通过比较这些特征,系统能够生成异常图(anomaly maps),这些图显示了图像中可能存在的异常区域,并给出了异常得分或置信度。这一步骤进一步验证了SG网络在保留语义信息方面的有效性。
避免类别错误: 相比于传统的扩散模型(如DDPM),SG网络通过引入类别条件解决了在多类异常检测任务中可能出现的类别错误问题。LDM虽然通过交叉注意力引入了条件约束,但在随机高斯噪声下去噪时仍可能丢失语义信息。SG网络则通过其语义引导机制,有效地避免了这一问题。
二、SG网络代码实现
这部分代码大概有300行
python
class SemanticGuidedNetwork(nn.Module):
def __init__(
self,
image_size,
in_channels,
model_channels,
hint_channels,
num_res_blocks,
attention_resolutions,
dropout=0,
channel_mult=(1, 2, 4, 8),
conv_resample=True,
dims=2,
use_checkpoint=False,
use_fp16=False,
num_heads=-1,
num_head_channels=-1,
num_heads_upsample=-1,
use_scale_shift_norm=False,
resblock_updown=False,
use_new_attention_order=False,
use_spatial_transformer=False, # custom transformer support
transformer_depth=1, # custom transformer support
context_dim=None, # custom transformer support
n_embed=None, # custom support for prediction of discrete ids into codebook of first stage vq model
legacy=True,
disable_self_attentions=None,
num_attention_blocks=None,
disable_middle_self_attn=False,
use_linear_in_transformer=False,
):
super().__init__()
if use_spatial_transformer:
assert context_dim is not None, 'Fool!! You forgot to include the dimension of your cross-attention conditioning...'
if context_dim is not None:
assert use_spatial_transformer, 'Fool!! You forgot to use the spatial transformer for your cross-attention conditioning...'
from omegaconf.listconfig import ListConfig
if type(context_dim) == ListConfig:
context_dim = list(context_dim)
if num_heads_upsample == -1:
num_heads_upsample = num_heads
if num_heads == -1:
assert num_head_channels != -1, 'Either num_heads or num_head_channels has to be set'
if num_head_channels == -1:
assert num_heads != -1, 'Either num_heads or num_head_channels has to be set'
self.dims = dims
self.image_size = image_size
self.in_channels = in_channels
self.model_channels = model_channels
if isinstance(num_res_blocks, int):
self.num_res_blocks = len(channel_mult) * [num_res_blocks]
else:
if len(num_res_blocks) != len(channel_mult):
raise ValueError("provide num_res_blocks either as an int (globally constant) or "
"as a list/tuple (per-level) with the same length as channel_mult")
self.num_res_blocks = num_res_blocks
if disable_self_attentions is not None:
# should be a list of booleans, indicating whether to disable self-attention in TransformerBlocks or not
assert len(disable_self_attentions) == len(channel_mult)
if num_attention_blocks is not None:
assert len(num_attention_blocks) == len(self.num_res_blocks)
assert all(map(lambda i: self.num_res_blocks[i] >= num_attention_blocks[i], range(len(num_attention_blocks))))
print(f"Constructor of UNetModel received num_attention_blocks={num_attention_blocks}. "
f"This option has LESS priority than attention_resolutions {attention_resolutions}, "
f"i.e., in cases where num_attention_blocks[i] > 0 but 2**i not in attention_resolutions, "
f"attention will still not be set.")
self.attention_resolutions = attention_resolutions
self.dropout = dropout
self.channel_mult = channel_mult
self.conv_resample = conv_resample
self.use_checkpoint = use_checkpoint
self.dtype = th.float16 if use_fp16 else th.float32
self.num_heads = num_heads
self.num_head_channels = num_head_channels
self.num_heads_upsample = num_heads_upsample
self.predict_codebook_ids = n_embed is not None
time_embed_dim = model_channels * 4
self.time_embed = nn.Sequential(
linear(model_channels, time_embed_dim),
nn.SiLU(),
linear(time_embed_dim, time_embed_dim),
)
self.input_blocks = nn.ModuleList(
[
TimestepEmbedSequential(
conv_nd(dims, in_channels, model_channels, 3, padding=1)
)
]
)
self.zero_convs = nn.ModuleList([self.make_zero_conv(model_channels)])
self.input_hint_block = TimestepEmbedSequential(
conv_nd(dims, hint_channels, 16, 3, padding=1),
nn.SiLU(),
conv_nd(dims, 16, 16, 3, padding=1),
nn.SiLU(),
conv_nd(dims, 16, 32, 3, padding=1, stride=2),
nn.SiLU(),
conv_nd(dims, 32, 32, 3, padding=1),
nn.SiLU(),
conv_nd(dims, 32, 96, 3, padding=1, stride=2),
nn.SiLU(),
conv_nd(dims, 96, 96, 3, padding=1),
nn.SiLU(),
conv_nd(dims, 96, 256, 3, padding=1, stride=2),
nn.SiLU(),
zero_module(conv_nd(dims, 256, model_channels, 3, padding=1))
)
self._feature_size = model_channels
input_block_chans = [model_channels]
ch = model_channels
ds = 1
for level, mult in enumerate(channel_mult):
for nr in range(self.num_res_blocks[level]):
layers = [
ResBlock(
ch,
time_embed_dim,
dropout,
out_channels=mult * model_channels,
dims=dims,
use_checkpoint=use_checkpoint,
use_scale_shift_norm=use_scale_shift_norm,
)
]
ch = mult * model_channels
if ds in attention_resolutions:
if num_head_channels == -1:
dim_head = ch // num_heads
else:
num_heads = ch // num_head_channels
dim_head = num_head_channels
if legacy:
# num_heads = 1
dim_head = ch // num_heads if use_spatial_transformer else num_head_channels
if exists(disable_self_attentions):
disabled_sa = disable_self_attentions[level]
else:
disabled_sa = False
if not exists(num_attention_blocks) or nr < num_attention_blocks[level]:
layers.append(
AttentionBlock(
ch,
use_checkpoint=use_checkpoint,
num_heads=num_heads,
num_head_channels=dim_head,
use_new_attention_order=use_new_attention_order,
) if not use_spatial_transformer else SpatialTransformer(
ch, num_heads, dim_head, depth=transformer_depth, context_dim=context_dim,
disable_self_attn=disabled_sa, use_linear=use_linear_in_transformer,
use_checkpoint=use_checkpoint
)
)
self.input_blocks.append(TimestepEmbedSequential(*layers))
self.zero_convs.append(self.make_zero_conv(ch))
self._feature_size += ch
input_block_chans.append(ch)
if level != len(channel_mult) - 1:
out_ch = ch
self.input_blocks.append(
TimestepEmbedSequential(
ResBlock(
ch,
time_embed_dim,
dropout,
out_channels=out_ch,
dims=dims,
use_checkpoint=use_checkpoint,
use_scale_shift_norm=use_scale_shift_norm,
down=True,
)
if resblock_updown
else Downsample(
ch, conv_resample, dims=dims, out_channels=out_ch
)
)
)
ch = out_ch
input_block_chans.append(ch)
self.zero_convs.append(self.make_zero_conv(ch))
ds *= 2
self._feature_size += ch
if num_head_channels == -1:
dim_head = ch // num_heads
else:
num_heads = ch // num_head_channels
dim_head = num_head_channels
if legacy:
# num_heads = 1
dim_head = ch // num_heads if use_spatial_transformer else num_head_channels
self.middle_block = TimestepEmbedSequential(
ResBlock(
ch,
time_embed_dim,
dropout,
dims=dims,
use_checkpoint=use_checkpoint,
use_scale_shift_norm=use_scale_shift_norm,
),
AttentionBlock(
ch,
use_checkpoint=use_checkpoint,
num_heads=num_heads,
num_head_channels=dim_head,
use_new_attention_order=use_new_attention_order,
) if not use_spatial_transformer else SpatialTransformer( # always uses a self-attn
ch, num_heads, dim_head, depth=transformer_depth, context_dim=context_dim,
disable_self_attn=disable_middle_self_attn, use_linear=use_linear_in_transformer,
use_checkpoint=use_checkpoint
),
ResBlock(
ch,
time_embed_dim,
dropout,
dims=dims,
use_checkpoint=use_checkpoint,
use_scale_shift_norm=use_scale_shift_norm,
),
)
self.middle_block_out = self.make_zero_conv(ch)
self._feature_size += ch
#SFF Block
self.down11 = nn.Sequential(
zero_module(nn.Conv2d(640, 1280, kernel_size=3, stride=2, padding=1, bias=False)),
nn.InstanceNorm2d(1280),
nn.SiLU(),
)
self.down12 = nn.Sequential(
zero_module(nn.Conv2d(640, 1280, kernel_size=3, stride=2, padding=1, bias=False)),
nn.InstanceNorm2d(1280),
nn.SiLU(),
)
self.down13 = nn.Sequential(
zero_module(nn.Conv2d(640, 1280, kernel_size=3, stride=2, padding=1, bias=False)),
nn.InstanceNorm2d(1280),
nn.SiLU(),
)
self.down21 = nn.Sequential(
zero_module(nn.Conv2d(1280, 1280, kernel_size=3, stride=2, padding=1, bias=False)),
nn.InstanceNorm2d(1280),
nn.SiLU(),
)
self.down22 = nn.Sequential(
zero_module(nn.Conv2d(1280, 1280, kernel_size=3, stride=2, padding=1, bias=False)),
nn.InstanceNorm2d(1280),
nn.SiLU(),
)
self.down23 = nn.Sequential(
zero_module(nn.Conv2d(1280, 1280, kernel_size=3, stride=2, padding=1, bias=False)),
nn.InstanceNorm2d(1280),
nn.SiLU(),
)
self.down31 = nn.Sequential(
zero_module(nn.Conv2d(1280, 1280, kernel_size=3, stride=2, padding=1, bias=False)),
nn.InstanceNorm2d(1280),
nn.SiLU(),
)
self.down32 = nn.Sequential(
zero_module(nn.Conv2d(1280, 1280, kernel_size=3, stride=2, padding=1, bias=False)),
nn.InstanceNorm2d(1280),
nn.SiLU(),
)
self.down33 = nn.Sequential(
zero_module(nn.Conv2d(1280, 1280, kernel_size=3, stride=2, padding=1, bias=False)),
nn.InstanceNorm2d(1280),
nn.SiLU(),
)
self.silu = nn.SiLU()
def make_zero_conv(self, channels):
return TimestepEmbedSequential(zero_module(conv_nd(self.dims, channels, channels, 1, padding=0)))
def forward(self, x, hint, timesteps, context, **kwargs):
t_emb = timestep_embedding(timesteps, self.model_channels, repeat_only=False)
emb = self.time_embed(t_emb)
guided_hint = self.input_hint_block(hint, emb, context)
outs = []
h = x.type(self.dtype)
for module, zero_conv in zip(self.input_blocks, self.zero_convs):
if guided_hint is not None:
h = module(h, emb, context)
h += guided_hint
guided_hint = None
else:
h = module(h, emb, context)
outs.append(zero_conv(h, emb, context))
#SFF Block Implementation
outs[9] = self.silu(outs[9]+self.down11(outs[6])+self.down21(outs[7])+self.down31(outs[8]))
outs[10] = self.silu(outs[10]+self.down12(outs[6])+self.down22(outs[7])+self.down32(outs[8]))
outs[11] = self.silu(outs[11]+self.down13(outs[6])+self.down23(outs[7])+self.down33(outs[8]))
h = self.middle_block(h, emb, context)
outs.append(self.middle_block_out(h, emb, context))
return outs