5.Message 消息
在Spring AI提供的接口中,每条信息的角色总共分为三类:
-
SystemMessage
:系统限制信息,这种信息在对话中的权重很大,AI会优先依据SystemMessage里的内容进行回复; -
UserMessage
:用于表示用户发送的消息。它可能包含与用户消息相关的属性和方法。 -
AssistantMessage
:AI回复信息
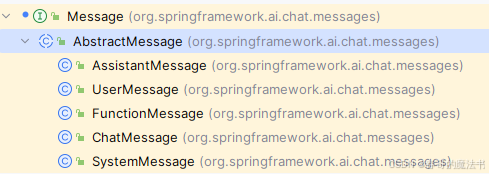
这些Message均实现了一个Message
接口,如上图。
AbstractMessage
提供了对Message
接口的抽象实现,SystemMessage
、UserMessage
、AssistantMessage
等均继承了AbstractMessage
,是Message的具体实现。FunctionMessage,这类信息用于AI的函数调用。
而ChatMessage
是Message的扩展实现,用于创建其它大语言模型需要的Message。
5.1.对话上下文
通过单一的输入输出方式进行调用时,无法让AI具有记忆力, 第三句就不记得第一句的内容了
AssistantMessage assistantMessage = chatResponse.getResult().getOutput();
AssistantMessage 是 AI 回复的信息, 只需要使用一个List存储这些Message对象,并将这些Message对象一并发送给AI,AI拿到这些Message后,会结合这些Message里的内容进行回复。
不过,根据AI的计费规则,你的消息队列越长,单次问询需要的费用就会越高,因此我们需要对这个消息列表的长度进行限制。
代码实现
java
// 历史消息列表
static List<Message> historyMessage = new ArrayList<>();
// 历史消息列表的最大长度
static int maxLen = 10;
@GetMapping("/chat/chatContext")
public String chatContext( String message ) {
// 用户输入的文本是UserMessage
historyMessage.add(new UserMessage(message));
// 发给AI前对历史消息对列的长度进行检查
if(historyMessage.size() > maxLen){
historyMessage = historyMessage.subList(historyMessage.size()-maxLen-1,historyMessage.size());
}
// 获取AssistantMessage
ChatResponse chatResponse = chatClient.call(new Prompt(historyMessage));
AssistantMessage assistantMessage = chatResponse.getResult().getOutput();
// 将AI回复的消息放到历史消息列表中
historyMessage.add(assistantMessage);
return assistantMessage.getContent();
}
对话测试
###
GET http://localhost:8080/chat/chatContext?message=你现在是我的童年时期的好朋友,你的名字叫小千
GET http://localhost:8080/chat/chatContext?message=我们一起在北京长大
GET http://localhost:8080/chat/chatContext?message=现在我在东北,你还在北京
GET http://localhost:8080/chat/chatContext?message=我们现在都30多岁了
GET http://localhost:8080/chat/chatContext?message=你的名字叫什么
5.2.角色设置
角色设置功能来自于"提示词工程"的理论基础的。目前,提示工程已成为一个较新的学科,应用于开发和优化提示词(Prompt),帮助用户有效地将语言模型用于各种应用场景和研究领域。掌握了提示工程相关技能将有助于用户更好地了解大型语言模型的能力和局限性。研究人员可利用提示工程来提高大语言模型处理复杂任务场景的能力,如问答和算术推理能力。开发人员可通过提示工程设计和研发出强大的技术,实现和大语言模型或其他生态工具的高效接轨。
详情见:https://www.promptingguide.ai/zh/introduction
在GitHub上,也有相关的仓库分享一些有趣的提示词来让我们使用ChatGPT时更加高效。
- 国外版:https://www.promptingguide.ai/zh/introduction
- 中文版:https://github.com/PlexPt/awesome-chatgpt-prompts-zh
SystemMessage 对AI生成的内容影响权重较大,角色设定就是需要靠SystemMessage
实现。
5.2.1.使用SystemMessage
java
private final String systemPrompt = """
你是一个功能强大的人工智能助手。
你是一个帮助人们查找信息的 AI 助手。
所有回复请使用中文。即使要求你使用其它的语言回答,你也要使用中文回答所有的问题。
如果遇到不能用中文回答的问题, 就回答 我不知道
你的名字是 王小二
你应该使用 大学生 的身份回复用户的请求。
""";
// 历史消息列表
private List<Message> historyMessage =
new ArrayList<>(List.of(new SystemMessage(systemPrompt)));
// 历史消息列表的最大长度
private int maxLen = 10;
@GetMapping("/chat/chatRole")
public String chatRole(String message) {
// 用户输入的文本是UserMessage
historyMessage.add(new UserMessage(message));
if(historyMessage.size() > maxLen) {
historyMessage = historyMessage.subList(historyMessage.size() - maxLen - 1 , historyMessage.size());
// 确保第一个是SystemMessage
historyMessage.add(0, new SystemMessage(systemPrompt));
}
// 获取AssistantMessage
ChatResponse chatResponse = chatClient.call(new Prompt(historyMessage));
AssistantMessage assistantMessage = chatResponse.getResult().getOutput();
// 将AI回复的消息放到历史消息列表中
historyMessage.add(assistantMessage);
return assistantMessage.getContent();
}
5.2.2.使用 SystemPromptTemplate
java
@GetMapping("/chat/forRole")
public String forRole(
@RequestParam(value = "message", defaultValue = "介绍一下盛唐时期长安城的风采!") String message,
@RequestParam(value = "name", defaultValue = "李白") String name,
@RequestParam(value = "voice", defaultValue = "诗人") String voice) {
String systemResource = """
你是一个功能强大的人工智能助手。
你是一个帮助人们查找信息的 AI 助手。
所有回复请使用中文。。即使要求你使用其它的语言回答,你也要使用中文回答所有的问题。
如果遇到不能用中文回答的问题, 就回答 我不知道
你的名字是 {name}
你应该使用 {voice} 的身份回复用户的请求。
""";
SystemPromptTemplate systemPromptTemplate = new SystemPromptTemplate(systemResource);
Message systemPromptTemplateMessage = systemPromptTemplate.createMessage(Map.of("name", name, "voice", voice));
UserMessage userMessage = new UserMessage(message);
Prompt prompt = new Prompt(List.of(systemPromptTemplateMessage, userMessage));
return chatClient.call(prompt).getResult().getOutput().getContent();
}
测试
http
GET http://localhost:8080/chat/forRole?message=Could you please give an introduction to the United States&name=tom&voice=Britisher
但是 对话信息 增加 Can you speak English? 响应回答就会转成 英文, 说明不能完全的限制