【PyTorch】基于YOLO的多目标检测项目(二)
目标检测是对图像中的现有目标进行定位和分类的过程。识别的对象在图像中显示有边界框。一般的目标检测方法有两种:基于区域提议的和基于回归/分类的。这里使用一种基于回归/分类的方法,称为YOLO。
目录
准备COCO数据集
COCO是一个大规模的对象检测,分割和字幕数据集。它包含80个对象类别用于对象检测。
下载以下GitHub存储库
https://github.com/pjreddie/darknethttps://github.com/pjreddie/darknet
创建一个名为config的文件夹,将darknet/cfg/coco.data、darknet/cfg/yolov3.cfg文件复制到config文件夹中。
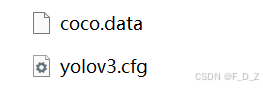
创建一个名为data的文件夹,从以下链接获取coco.names文件,并将其放入data文件夹,coco.names文件包含COCO数据集中80个对象类别的列表。
darknet/data/coco.names at master · pjreddie/darknet · GitHubConvolutional Neural Networks. Contribute to pjreddie/darknet development by creating an account on GitHub.https://github.com/pjreddie/darknet/blob/master/data/coco.names将darknet/scripts/get_coco_dataset.sh文件复制到data文件夹中,并复制get_coco_cocoet.sh到data文件夹。接下来,打开一个终端并执行get_coco_cocoet.sh,该脚本将把完整的COCO数据集下载到名为coco的子文件夹中。也可通过以下链接下载coco数据集。
COCO2014_数据集-飞桨AI Studio星河社区 (baidu.com)https://aistudio.baidu.com/datasetdetail/165195
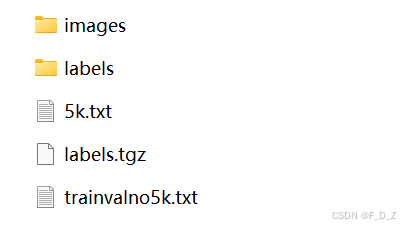
在images文件夹中,有两个名为train 2014和val 2014的文件夹,分别包含82783和40504个图像。在labels文件夹中,有两个名为train 2014和val 2014的标签,分别包含82081和40137文本文件。这些文本文件包含图像中对象的边界框坐标。此外,trainvalno5k.txt文件是一个包含117264张图像的列表,这些图像将用于训练模型。此列表是train2014和val2014中图像的组合,5000个图像除外。5k.txt文件包含将用于验证的5000个图像的列表。
创建自定义数据集
完成数据集下载后,使用PyTorch的Dataset和Dataloader类创建训练和验证数据集和数据加载器。
from torch.utils.data import Dataset
from PIL import Image
import torchvision.transforms.functional as TF
import os
import numpy as np
import torch
device = torch.device("cuda:0" if torch.cuda.is_available() else "cpu")
print(torch.__version__)
#定义CocoDataset类,并展示来自训练和验证数据集的一些示例图像
class CocoDataset(Dataset):
def __init__(self, path2listFile, transform=None, trans_params=None):
with open(path2listFile, "r") as file:
self.path2imgs = file.readlines()
self.path2labels = [
path.replace("images", "labels").replace(".png", ".txt").replace(".jpg", ".txt")
for path in self.path2imgs]
self.trans_params = trans_params
self.transform = transform
def __len__(self):
return len(self.path2imgs)
def __getitem__(self, index):
path2img = self.path2imgs[index % len(self.path2imgs)].rstrip()
img = Image.open(path2img).convert('RGB')
path2label = self.path2labels[index % len(self.path2imgs)].rstrip()
labels= None
if os.path.exists(path2label):
labels = np.loadtxt(path2label).reshape(-1, 5)
if self.transform:
img, labels = self.transform(img, labels, self.trans_params)
return img, labels, path2img
root_data="./data/coco"
path2trainList=os.path.join(root_data, "trainvalno5k.txt")
coco_train = CocoDataset(path2trainList)
print(len(coco_train))
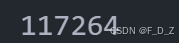
# 从coco_train中获取图像、标签和图像路径
img, labels, path2img = coco_train[1]
print("image size:", img.size, type(img))
print("labels shape:", labels.shape, type(labels))
print("labels \n", labels)
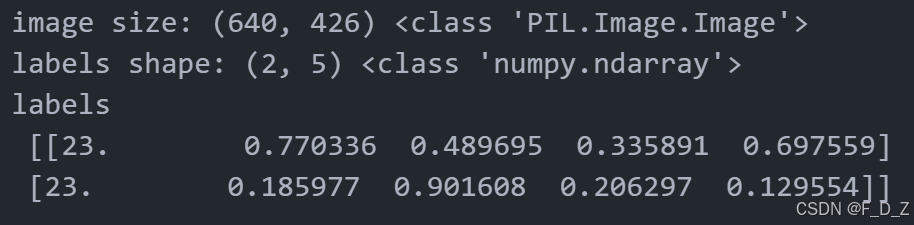
path2valList=os.path.join(root_data, "5k.txt")
coco_val = CocoDataset(path2valList, transform=None, trans_params=None)
print(len(coco_val))
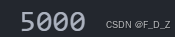
img, labels, path2img = coco_val[7]
print("image size:", img.size, type(img))
print("labels shape:", labels.shape, type(labels))
print("labels \n", labels)
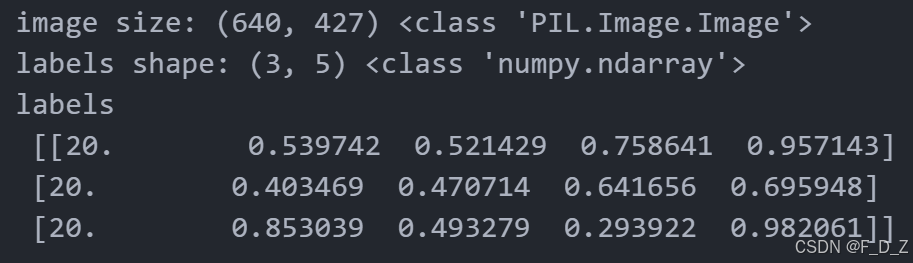
import matplotlib.pylab as plt
import numpy as np
from PIL import Image, ImageDraw, ImageFont
from torchvision.transforms.functional import to_pil_image
import random
%matplotlib inline
path2cocoNames="./data/coco.names"
fp = open(path2cocoNames, "r")
coco_names = fp.read().split("\n")[:-1]
print("number of classese:", len(coco_names))
print(coco_names)

def rescale_bbox(bb,W,H):
x,y,w,h=bb
return [x*W, y*H, w*W, h*H]
COLORS = np.random.randint(0, 255, size=(80, 3),dtype="uint8")
# fnt = ImageFont.truetype('Pillow/Tests/fonts/FreeMono.ttf', 16)
fnt = ImageFont.truetype('arial.ttf', 16)
def show_img_bbox(img,targets):
if torch.is_tensor(img):
img=to_pil_image(img)
if torch.is_tensor(targets):
targets=targets.numpy()[:,1:]
W, H=img.size
draw = ImageDraw.Draw(img)
for tg in targets:
id_=int(tg[0])
bbox=tg[1:]
bbox=rescale_bbox(bbox,W,H)
xc,yc,w,h=bbox
color = [int(c) for c in COLORS[id_]]
name=coco_names[id_]
draw.rectangle(((xc-w/2, yc-h/2), (xc+w/2, yc+h/2)),outline=tuple(color),width=3)
draw.text((xc-w/2,yc-h/2),name, font=fnt, fill=(255,255,255,0))
plt.imshow(np.array(img))
np.random.seed(1)
rnd_ind=np.random.randint(len(coco_train))
img, labels, path2img = coco_train[rnd_ind]
print(img.size, labels.shape)
plt.rcParams['figure.figsize'] = (20, 10)
show_img_bbox(img,labels)

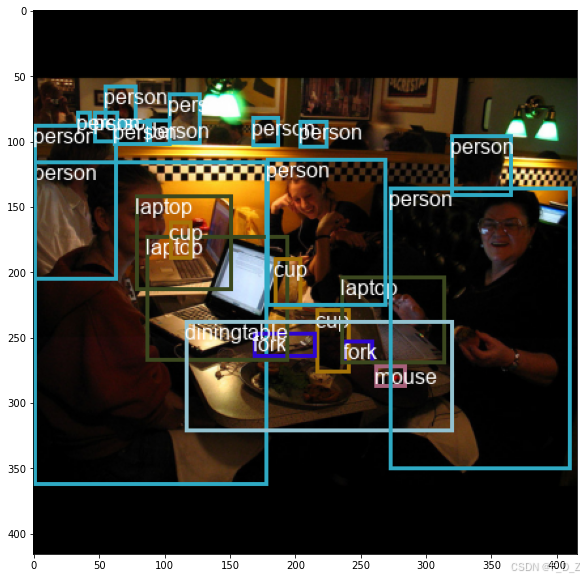
np.random.seed(1)
rnd_ind=np.random.randint(len(coco_val))
img, labels, path2img = coco_val[rnd_ind]
print(img.size, labels.shape)
plt.rcParams['figure.figsize'] = (20, 10)
show_img_bbox(img,labels)
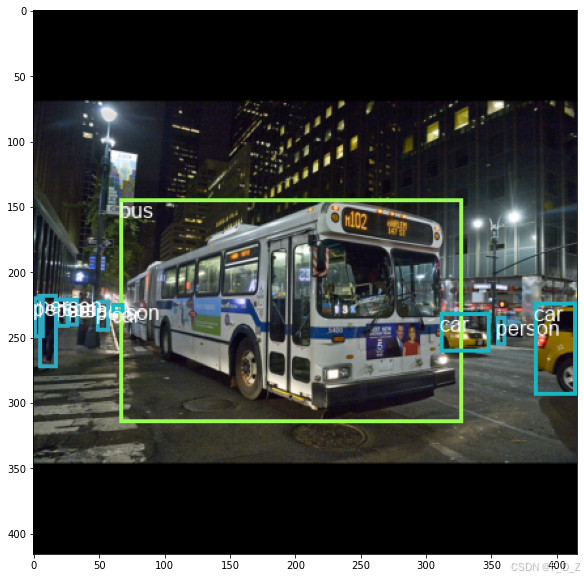
转换数据
定义一个转换函数和传递给CocoDataset类的参数
def pad_to_square(img, boxes, pad_value=0, normalized_labels=True):
w, h = img.size
w_factor, h_factor = (w,h) if normalized_labels else (1, 1)
dim_diff = np.abs(h - w)
pad1= dim_diff // 2
pad2= dim_diff - pad1
if h<=w:
left, top, right, bottom= 0, pad1, 0, pad2
else:
left, top, right, bottom= pad1, 0, pad2, 0
padding= (left, top, right, bottom)
img_padded = TF.pad(img, padding=padding, fill=pad_value)
w_padded, h_padded = img_padded.size
x1 = w_factor * (boxes[:, 1] - boxes[:, 3] / 2)
y1 = h_factor * (boxes[:, 2] - boxes[:, 4] / 2)
x2 = w_factor * (boxes[:, 1] + boxes[:, 3] / 2)
y2 = h_factor * (boxes[:, 2] + boxes[:, 4] / 2)
x1 += padding[0] # 左
y1 += padding[1] # 上
x2 += padding[2] # 右
y2 += padding[3] # 下
boxes[:, 1] = ((x1 + x2) / 2) / w_padded
boxes[:, 2] = ((y1 + y2) / 2) / h_padded
boxes[:, 3] *= w_factor / w_padded
boxes[:, 4] *= h_factor / h_padded
return img_padded, boxes
def hflip(image, labels):
image = TF.hflip(image)
labels[:, 1] = 1.0 - labels[:, 1]
return image, labels
def transformer(image, labels, params):
if params["pad2square"] is True:
image,labels= pad_to_square(image, labels)
image = TF.resize(image,params["target_size"])
if random.random() < params["p_hflip"]:
image,labels=hflip(image,labels)
image=TF.to_tensor(image)
targets = torch.zeros((len(labels), 6))
targets[:, 1:] = torch.from_numpy(labels)
return image, targets
trans_params_train={
"target_size" : (416, 416),
"pad2square": True,
"p_hflip" : 1.0,
"normalized_labels": True,
}
coco_train=CocoDataset(path2trainList,transform=transformer,trans_params=trans_params_train)
np.random.seed(100)
rnd_ind=np.random.randint(len(coco_train))
img, targets, path2img = coco_train[rnd_ind]
print("image shape:", img.shape)
print("labels shape:", targets.shape)
plt.rcParams['figure.figsize'] = (20, 10)
COLORS = np.random.randint(0, 255, size=(80, 3),dtype="uint8")
show_img_bbox(img,targets)

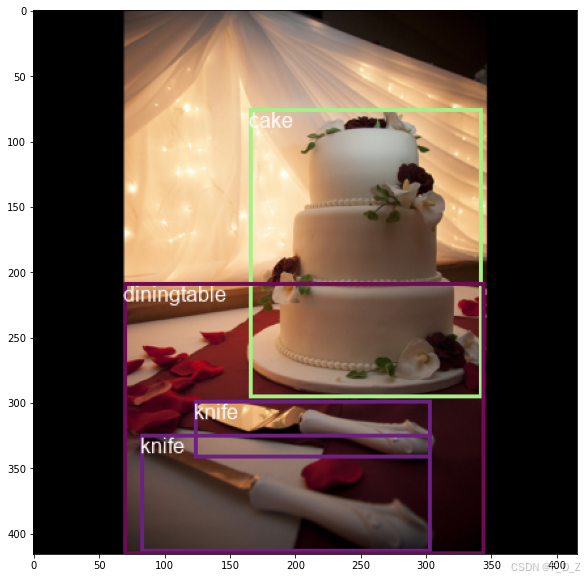
通过传递 transformer 函数来定义 CocoDataset 的一个对象来验证数据
trans_params_val={
"target_size" : (416, 416),
"pad2square": True,
"p_hflip" : 0.0,
"normalized_labels": True,
}
coco_val= CocoDataset(path2valList,
transform=transformer,
trans_params=trans_params_val)
np.random.seed(55)
rnd_ind=np.random.randint(len(coco_val))
img, targets, path2img = coco_val[rnd_ind]
print("image shape:", img.shape)
print("labels shape:", targets.shape)
plt.rcParams['figure.figsize'] = (20, 10)
COLORS = np.random.randint(0, 255, size=(80, 3),dtype="uint8")
show_img_bbox(img,targets)

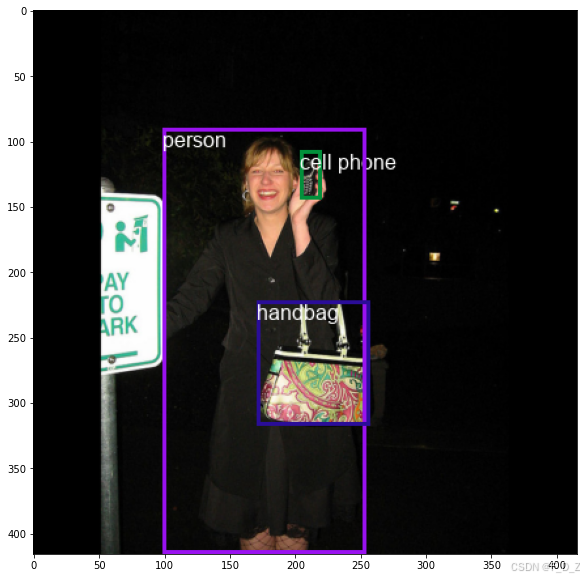
定义数据加载器
定义两个用于训练和验证数据集的数据加载器,从coco_train和coco_val中获取小批量数据。
from torch.utils.data import DataLoader
batch_size=8
def collate_fn(batch):
imgs, targets, paths = list(zip(*batch))
targets = [boxes for boxes in targets if boxes is not None]
for b_i, boxes in enumerate(targets):
boxes[:, 0] = b_i
targets = torch.cat(targets, 0)
imgs = torch.stack([img for img in imgs])
return imgs, targets, paths
train_dl = DataLoader(
coco_train,
batch_size=batch_size,
shuffle=True,
num_workers=0,
pin_memory=True,
collate_fn=collate_fn,
)
torch.manual_seed(0)
for imgs_batch,tg_batch,path_batch in train_dl:
break
print(imgs_batch.shape)
print(tg_batch.shape,tg_batch.dtype)

val_dl = DataLoader(
coco_val,
batch_size=batch_size,
shuffle=False,
num_workers=0,
pin_memory=True,
collate_fn=collate_fn,
)
torch.manual_seed(0)
for imgs_batch,tg_batch,path_batch in val_dl:
break
print(imgs_batch.shape)
print(tg_batch.shape,tg_batch.dtype)
