YOLOV8替换Lion优化器
1 优化器介绍博客
论文地址:https://arxiv.org/abs/2302.06675
代码地址:https://github.com/google/automl/blob/master/lion/lion_pytorch.py
python
"""PyTorch implementation of the Lion optimizer."""
import torch
from torch.optim.optimizer import Optimizer
class Lion(Optimizer):
r"""Implements Lion algorithm."""
def __init__(self, params, lr=1e-4, betas=(0.9, 0.99), weight_decay=0.0):
"""Initialize the hyperparameters.
Args:
params (iterable): iterable of parameters to optimize or dicts defining
parameter groups
lr (float, optional): learning rate (default: 1e-4)
betas (Tuple[float, float], optional): coefficients used for computing
running averages of gradient and its square (default: (0.9, 0.99))
weight_decay (float, optional): weight decay coefficient (default: 0)
"""
if not 0.0 <= lr:
raise ValueError('Invalid learning rate: {}'.format(lr))
if not 0.0 <= betas[0] < 1.0:
raise ValueError('Invalid beta parameter at index 0: {}'.format(betas[0]))
if not 0.0 <= betas[1] < 1.0:
raise ValueError('Invalid beta parameter at index 1: {}'.format(betas[1]))
defaults = dict(lr=lr, betas=betas, weight_decay=weight_decay)
super().__init__(params, defaults)
@torch.no_grad()
def step(self, closure=None):
"""Performs a single optimization step.
Args:
closure (callable, optional): A closure that reevaluates the model
and returns the loss.
Returns:
the loss.
"""
loss = None
if closure is not None:
with torch.enable_grad():
loss = closure()
for group in self.param_groups:
for p in group['params']:
if p.grad is None:
continue
# Perform stepweight decay
p.data.mul_(1 - group['lr'] * group['weight_decay'])
grad = p.grad
state = self.state[p]
# State initialization
if len(state) == 0:
# Exponential moving average of gradient values
state['exp_avg'] = torch.zeros_like(p)
exp_avg = state['exp_avg']
beta1, beta2 = group['betas']
# Weight update
update = exp_avg * beta1 + grad * (1 - beta1)
p.add_(torch.sign(update), alpha=-group['lr'])
# Decay the momentum running average coefficient
exp_avg.mul_(beta2).add_(grad, alpha=1 - beta2)
return loss
2 在相应的文件夹内新建lion_pytorch.py文件
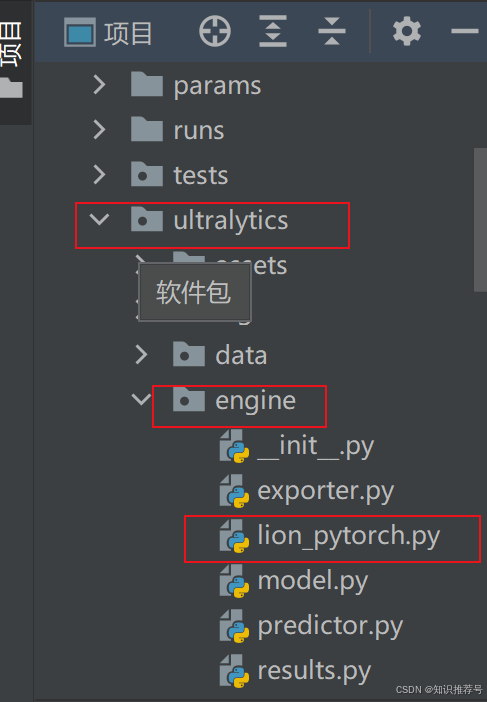
3 在trianer.py中添加Lion优化器
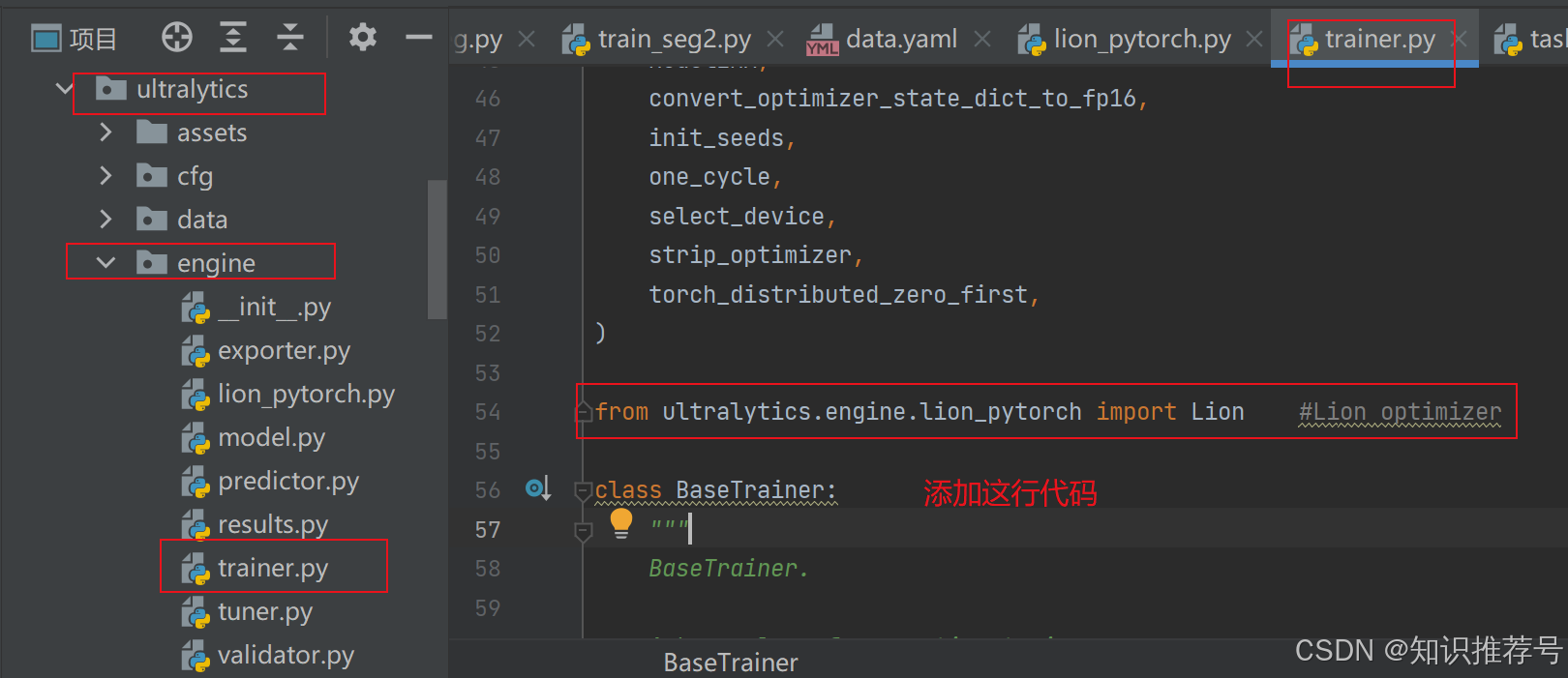
python
from ultralytics.engine.lion_pytorch import Lion #Lion optimizer
然后在末尾build_optimizer函数中添加判断是否使用Lion优化器:
python
def build_optimizer(self, model, name="auto", lr=0.001, momentum=0.9, decay=1e-5, iterations=1e5):
·······
elif name == "Lion":
optimizer = Lion(g[2], lr=lr, betas=(momentum, 0.99), weight_decay=0.0)
·······
4 设置Lion优化器并训练查看
方法1:defalut.yaml中修改默认设置:
方法2:训练文件中自定义设置:
Lion优化器默认的学习率改为为1e-4,不然就是yolov8中默认的0.01。
运行训练文件后可以看到如下提示则修改成功: