NVIDIA DLI RAG课程(Course Detail | NVIDIA ),并获得该课程证书。
1 07的ipynb文件中设定,使用这两个模型配置
embedder = NVIDIAEmbeddings(model="nvidia/nv-embed-v1", truncate="END")
# ChatNVIDIA.get_available_models()
instruct_llm = ChatNVIDIA(model="mistralai/mixtral-8x7b-instruct-v0.1")
大约会在Part3的 Task3出错,否则会早早出错。
08不用改
运行完 所有cell后,点击下面的绿色的 Link To Gradio Frontend 文字,跳转到我们服务启动的页面
35的ipynb文件脚本需要修改几处:
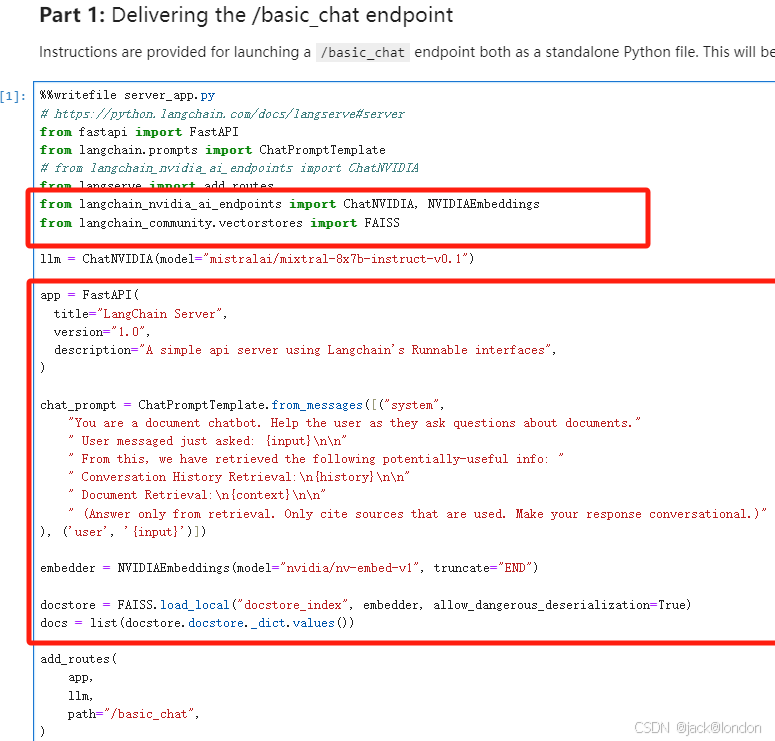
从07/08两个脚本中复制代码出来
从07 Part3复制如下
chat_prompt = ChatPromptTemplate.from_messages([("system",
"You are a document chatbot. Help the user as they ask questions about documents."
" User messaged just asked: {input}\n\n"
" From this, we have retrieved the following potentially-useful info: "
" Conversation History Retrieval:\n{history}\n\n"
" Document Retrieval:\n{context}\n\n"
" (Answer only from retrieval. Only cite sources that are used. Make your response conversational.)"
), ('user', '{input}')])
embedder = NVIDIAEmbeddings(model="nvidia/nv-embed-v1", truncate="END")
08 Part3 Task1 复制如下
from langchain_nvidia_ai_endpoints import NVIDIAEmbeddings
from langchain_community.vectorstores import FAISS
docstore = FAISS.load_local("docstore_index", embedder, allow_dangerous_deserialization=True)
docs = list(docstore.docstore._dict.values())
再手写补充如下
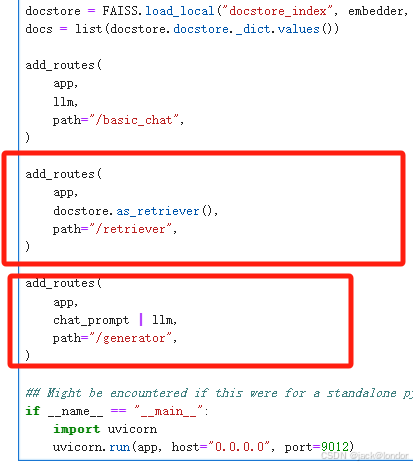
add_routes(
app,
docstore.as_retriever(),
path="/retriever",
)
add_routes(
app,
chat_prompt | llm,
path="/generator",
)
然后将 08 中的问题复制粘贴到 到输入框中,运行即可。