一、什么是直方图
- 由一批长方形构成,通过长方形的面积或高度来代表对应组在数据中所占的比例。
- 用长方形的面积代表对应组的频数与组距的比时,则称为频率分布直方图;
- 当用长方形的高代表对应组的频数时,则称为频数分布直方图。
- 但严格统计意义上的直方图都是指频率分布直方图 ,而且统计意义上的直方图没有纵向刻度。
二、绘制直方图
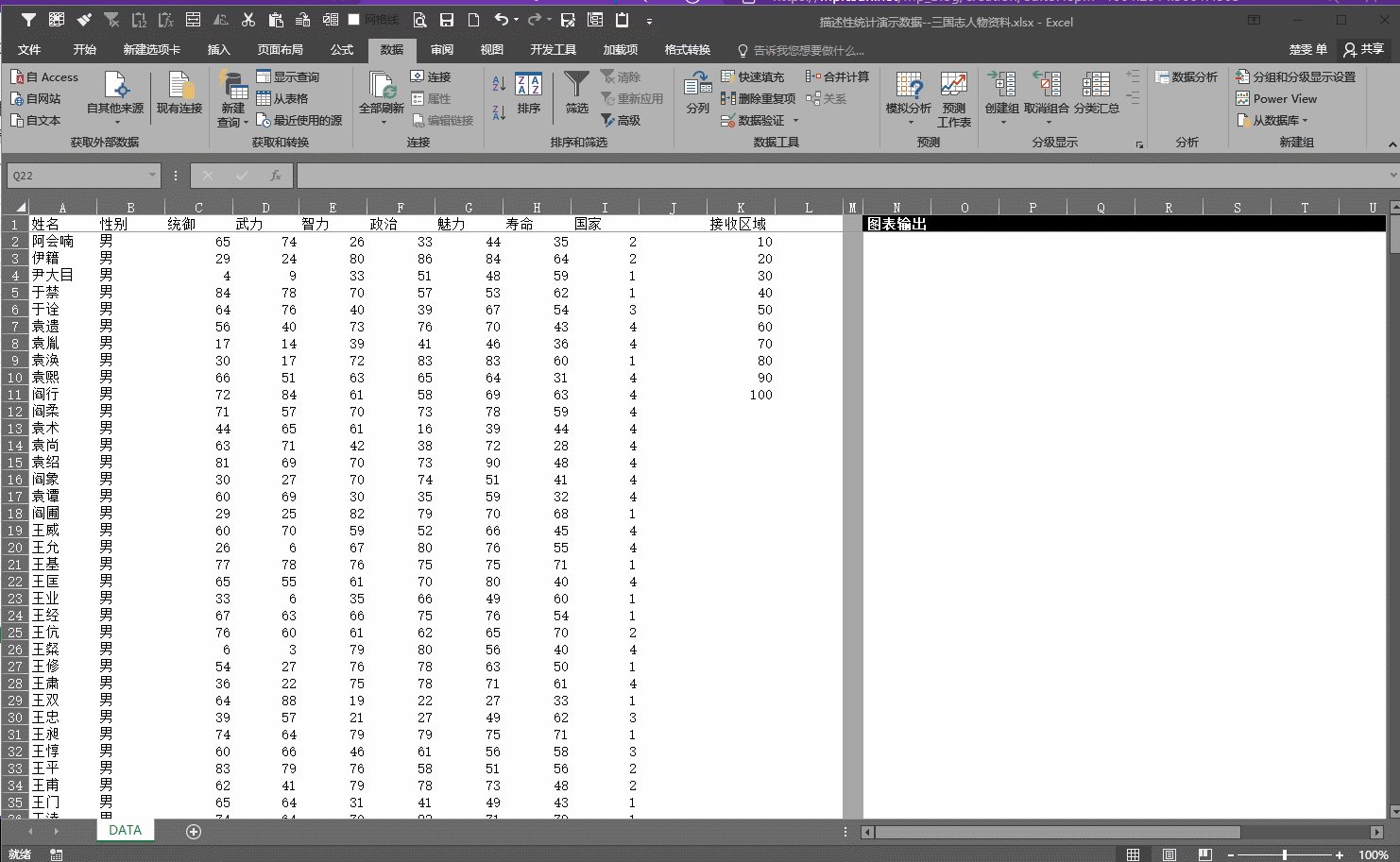
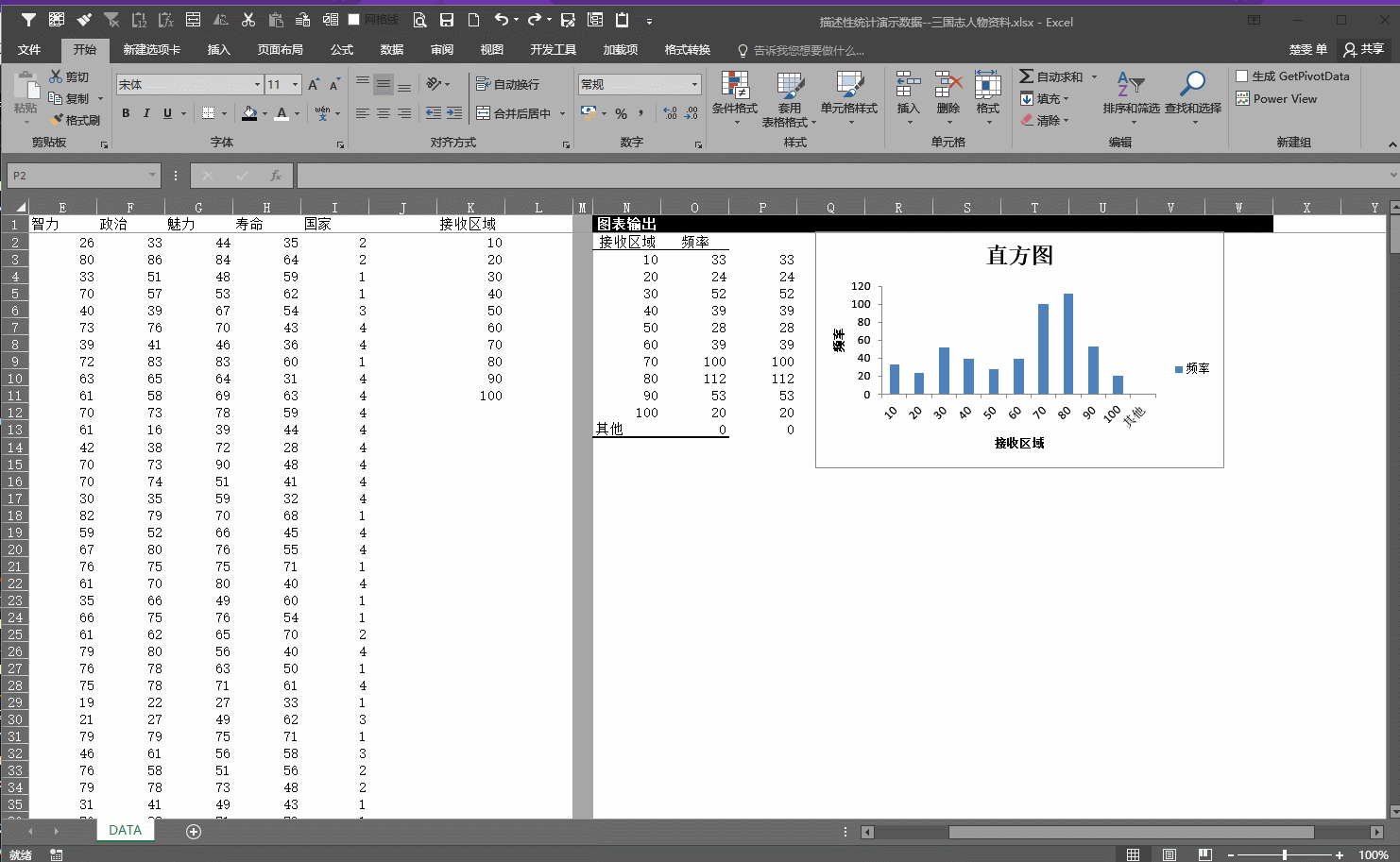
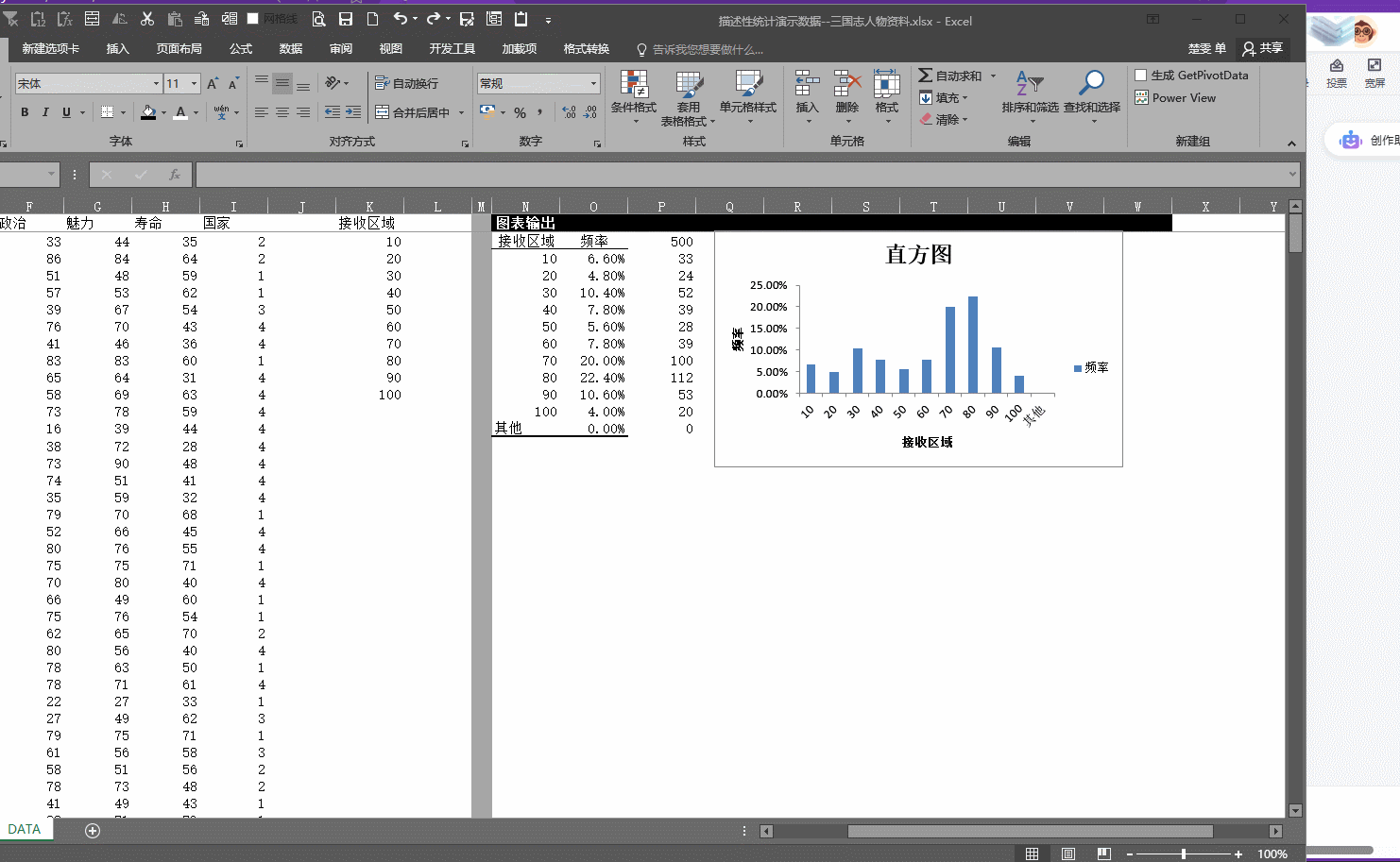
三、Python 绘制直方图
1、使用 pandas 、numpy 和 matplotlib绘制直方图
python
# Using pandas, numpy, matplotlib to draw histograms
import pandas as pd
import numpy as np
import matplotlib.pyplot as plt
import matplotlib
# set the backend of matplotlib to TkAgg
matplotlib.use('TkAgg')
data = pd.DataFrame({
# Generate 1000 random numbers with a standard normal distribution
'Values': pd.Series(np.random.randn(1000))
})
# Draw a histogram
data['Values'].plot.hist(bins=30, alpha=0.5, color='lightblue',
edgecolor='darkblue')
# set chart title and axis labels
plt.title('Histogram of Values')
plt.xlabel('Value')
plt.ylabel('Frequency')
# display chart
plt.show()
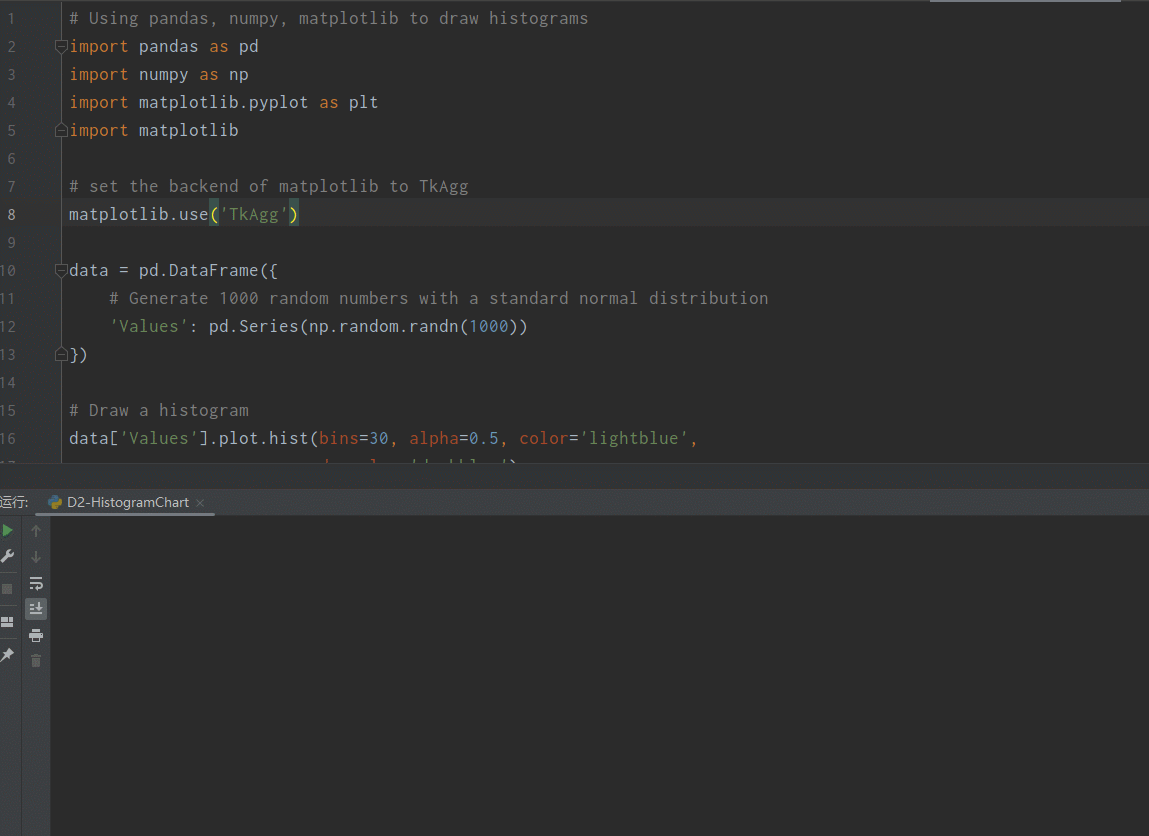
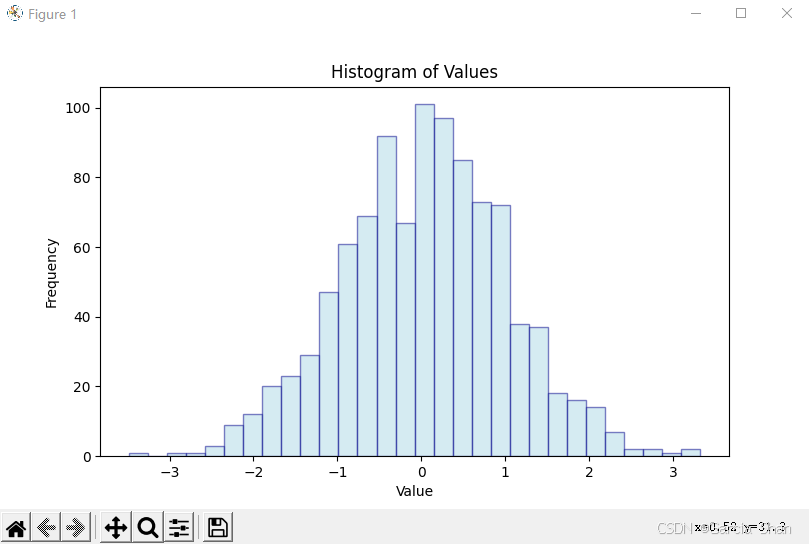
2、使用 pandas 、numpy 和 matplotlib绘制直方图
python
# Using plotly.express to draw histograms
import plotly.express as px
import pandas as pd
import numpy as np
# data = [1, 2, 3, 4, 5, 6, 7, 8, 8, 9, 10, 11, 12, 13, 14, 15, 16, 17]
data = pd.DataFrame({
'Values': pd.Series(np.random.randn(1000))
})
# Nbins defines the number of boxes in the histogram
fig = px.histogram(data, nbins=80,
color_discrete_sequence=['blue'],
labels={'Values': 'Value'},
title='Histogram of Values',
# marginal="box"
)
fig.update_traces(
marker=dict(
color='lightblue',
opacity=0.75,
line=dict(
color='darkblue',
width=1
)
)
)
# display chart
fig.show()
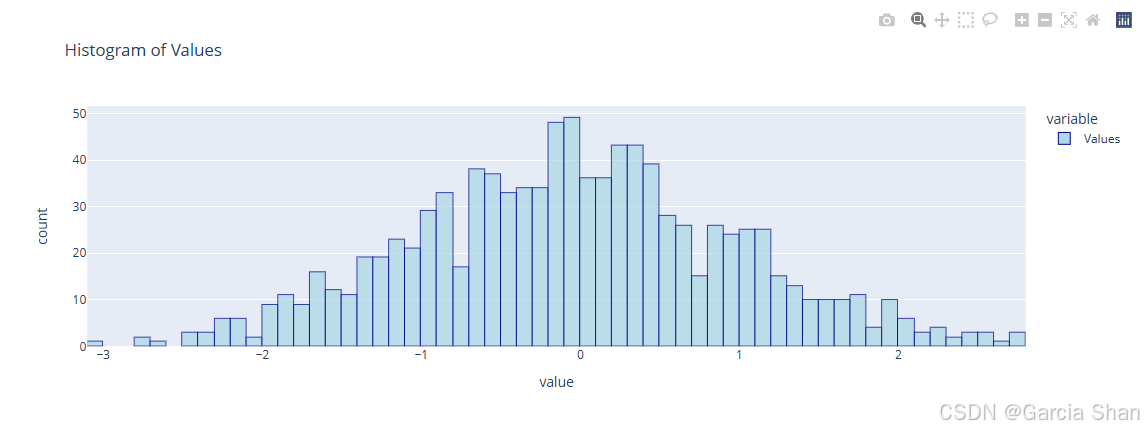
3、使用 pandas 、numpy 、 dash****和 plotly.express绘制直方图
python
# Using dash, plotly.express to draw histogram
import dash
import numpy as np
import pandas as pd
from dash import html, dcc
import plotly.express as px
# create a dash application
app = dash.Dash(__name__)
# create as list
# data = [1, 2, 2, 2, 2, 3, 4, 5, 5, 5, 6, 7, 8, 8, 8, 9, 9, 10, 10, 10, 11, 12, 12, 12, 13, 14, 12, 15, 15, 16, 17, 18, 18, 18, 19, 19, 20, 20, 20]
data = pd.DataFrame({
'Values': pd.Series(np.random.randn(1000))
})
# use plotly.express to draw histogram
fig = px.histogram(data, nbins=60,
color_discrete_sequence=['blue'],
labels={'Values': 'Value'},
title='Histogram of Values',
# marginal="box",
)
fig.update_traces(
marker=dict(
color='lightblue',
opacity=0.75,
line=dict(
color='darkblue',
width=1
)
)
)
# define application layout
app.layout = html.Div([
html.H1('Dash Histogram Example'),
dcc.Graph(figure=fig)
])
# run the application
if __name__ == '__main__':
app.run_server(debug=True)
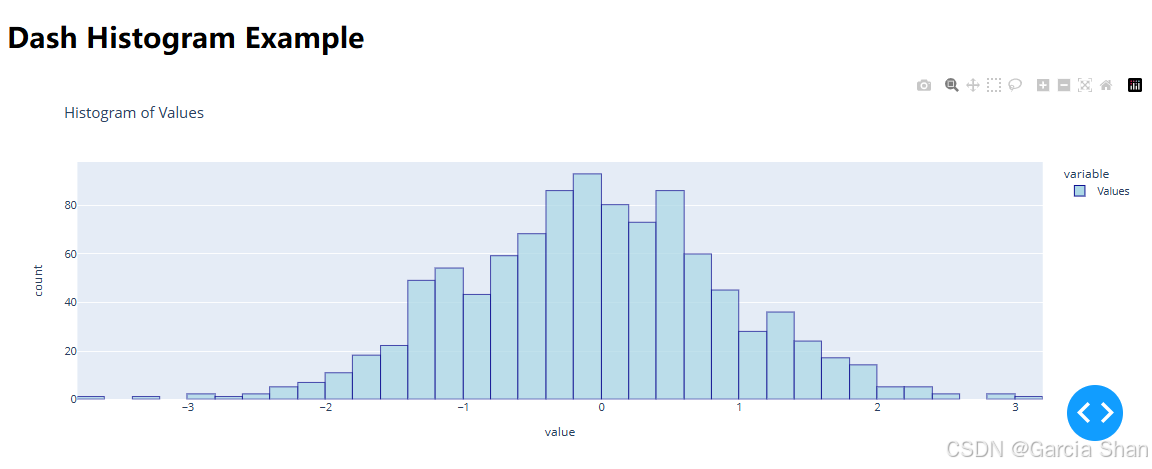