欢迎关注我的CSDN:https://spike.blog.csdn.net/
免责声明:本文来源于个人知识与公开资料,仅用于学术交流,欢迎讨论,不支持转载。
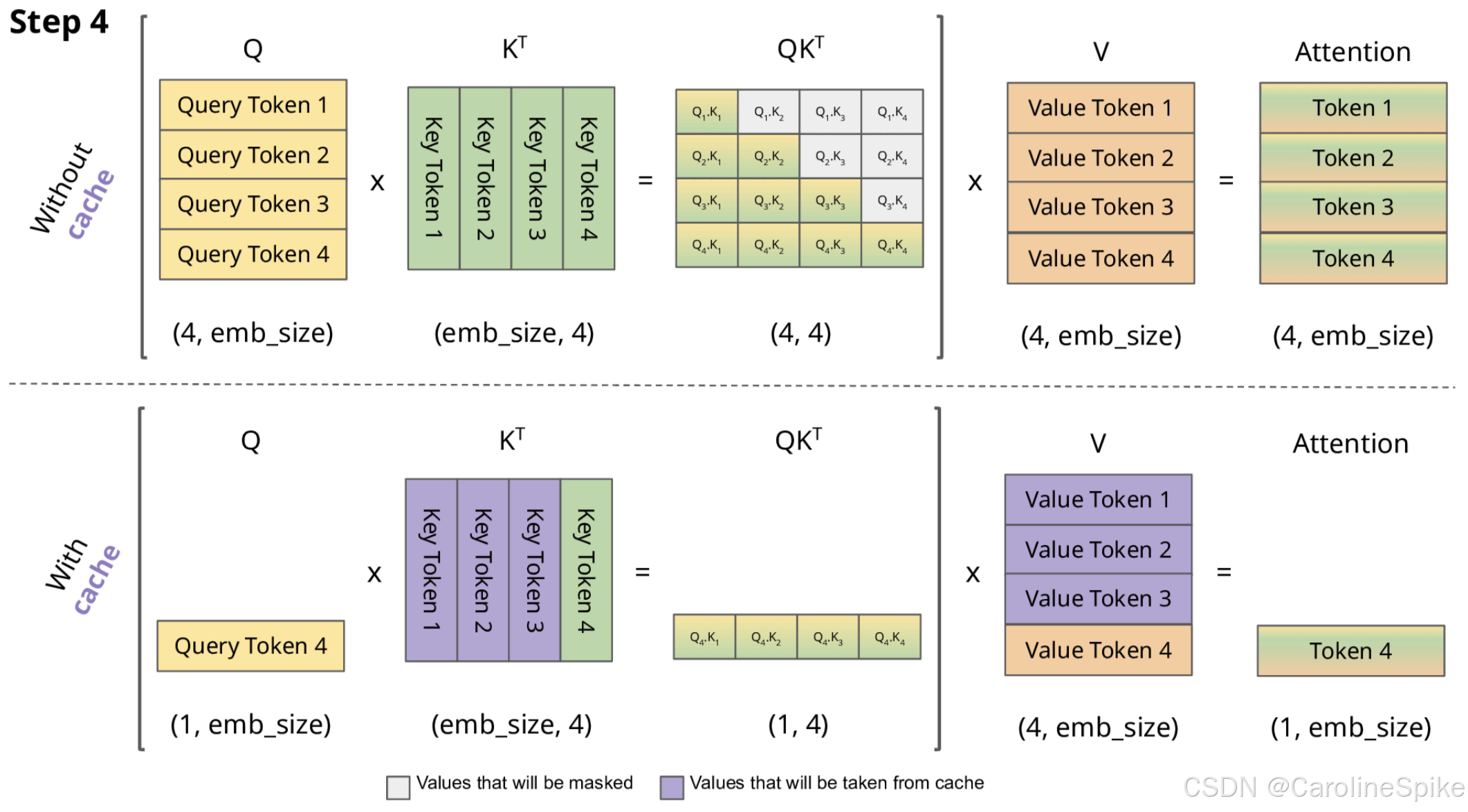
在 GPT 类模型中,KV Cache (键值缓存) 是用于优化推理效率的重要技术,基本思想是通过缓存先前计算的 键(Key) 和 值(Value),避免在推理过程中,重复计算 Mask 的 注意力(Attention) 矩阵,从而加速生成过程。
1. 公式
矩阵乘法的基础性质:
A ⋅ B = [ A 1 A 2 ... A n ] ⋅ [ B 1 B 2 ⋮ B n ] = A 1 B 1 + A 2 B 2 + ⋯ + A n B n A \cdot B = \begin{bmatrix} A_{1} & A_{2} & \dots & A_{n} \end{bmatrix} \cdot \begin{bmatrix} B_{1} \\ B_{2} \\ \vdots \\ B_{n} \end{bmatrix} = A_{1}B_{1} + A_{2}B_{2} + \dots + A_{n}B_{n} A⋅B=[A1A2...An]⋅ B1B2⋮Bn =A1B1+A2B2+⋯+AnBn
其中 A i A_{i} Ai 是 A A A 的列向量, B i B_{i} Bi 是 B B B 的行向量,也就是说相同维度的向量相乘,可拆解成行向量乘以列向量,即 A A A 有 n n n 列, B B B 有 n n n 行。如图:

例如:基础的矩阵乘法:
A = [ 1 2 3 4 ] , B = [ 5 6 7 8 ] C = [ 1 ∗ 5 + 2 ∗ 7 1 ∗ 6 + 2 ∗ 8 3 ∗ 5 + 4 ∗ 7 3 ∗ 6 + 4 ∗ 8 ] = [ 19 22 43 50 ] A = \begin{bmatrix} 1 & 2 \\ 3 & 4 \end{bmatrix}, \quad B = \begin{bmatrix} 5 & 6 \\ 7 & 8 \end{bmatrix} \\ C = \begin{bmatrix} 1*5 + 2*7 & 1*6 + 2*8 \\ 3*5 + 4*7 & 3*6 + 4*8 \end{bmatrix} = \begin{bmatrix} 19 & 22 \\ 43 & 50 \end{bmatrix} A=[1324],B=[5768]C=[1∗5+2∗73∗5+4∗71∗6+2∗83∗6+4∗8]=[19432250]
也可以写成,行列向量相乘的形式,即 A 拆分出多个行向量,B 拆分出多个列向量,即:
C = [ 1 3 ] ⋅ [ 5 6 ] + [ 2 4 ] ⋅ [ 7 8 ] = [ 1 ∗ 5 1 ∗ 6 3 ∗ 5 3 ∗ 6 ] + [ 2 ∗ 7 2 ∗ 8 4 ∗ 7 4 ∗ 8 ] C = \begin{bmatrix} 1 \\ 3 \end{bmatrix} \cdot \begin{bmatrix} 5 & 6 \end{bmatrix} + \begin{bmatrix} 2 \\ 4 \end{bmatrix} \cdot \begin{bmatrix} 7 & 8 \end{bmatrix}= \begin{bmatrix} 1*5 & 1*6 \\ 3*5 & 3*6 \end{bmatrix} + \begin{bmatrix} 2*7 & 2*8 \\ 4*7 & 4*8 \end{bmatrix} C=[13]⋅[56]+[24]⋅[78]=[1∗53∗51∗63∗6]+[2∗74∗72∗84∗8]
= [ 1 ∗ 5 + 2 ∗ 7 1 ∗ 6 + 2 ∗ 8 3 ∗ 5 + 4 ∗ 7 3 ∗ 6 + 4 ∗ 8 ] = [ 19 22 43 50 ] =\begin{bmatrix} 1*5 + 2*7 & 1*6 + 2*8 \\ 3*5 + 4*7 & 3*6 + 4*8 \end{bmatrix} = \begin{bmatrix} 19 & 22 \\ 43 & 50 \end{bmatrix} =[1∗5+2∗73∗5+4∗71∗6+2∗83∗6+4∗8]=[19432250]
进一步拆解:
A ⋅ B = A 1 B 1 + A 2 B 2 + ⋯ + A n B n = [ a 1 , 1 B 1 a 2 , 1 B 2 ⋮ a m , 1 B n ] + [ a 1 , 2 B 1 a 2 , 2 B 2 ⋮ a m , 2 B n ] + ⋯ + [ a 1 , n B 1 a 2 , n B 2 ⋮ a m , n B n ] = [ a 1 , 1 B 1 + a 1 , 2 B 1 + ⋯ + a 1 , n B 1 a 2 , 1 B 2 + a 2 , 2 B 2 + ⋯ + a 2 , n B 2 ⋯ a m , 1 B n + a m , 2 B n + ⋯ + a m , n B n ] A \cdot B = A_{1}B_{1} + A_{2}B_{2} + \dots + A_{n}B_{n} \\ = \begin{bmatrix} a_{1,1}B_{1} \\ a_{2,1}B_{2} \\ \vdots \\ a_{m,1}B_{n} \end{bmatrix} + \begin{bmatrix} a_{1,2}B_{1} \\ a_{2,2}B_{2} \\ \vdots \\ a_{m,2}B_{n} \end{bmatrix} + \cdots + \begin{bmatrix} a_{1,n}B_{1} \\ a_{2,n}B_{2} \\ \vdots \\ a_{m,n}B_{n} \end{bmatrix} \\ = \begin{bmatrix} a_{1,1}B_{1} + a_{1,2}B_{1} + \cdots + a_{1,n}B_{1} \\ a_{2,1}B_{2} + a_{2,2}B_{2} + \cdots + a_{2,n}B_{2} \\ \cdots \\ a_{m,1}B_{n} + a_{m,2}B_{n} + \cdots + a_{m,n}B_{n} \end{bmatrix} A⋅B=A1B1+A2B2+⋯+AnBn= a1,1B1a2,1B2⋮am,1Bn + a1,2B1a2,2B2⋮am,2Bn +⋯+ a1,nB1a2,nB2⋮am,nBn = a1,1B1+a1,2B1+⋯+a1,nB1a2,1B2+a2,2B2+⋯+a2,nB2⋯am,1Bn+am,2Bn+⋯+am,nBn
基础的矩阵乘法的另一种形式:
C = [ 1 3 ] ⋅ [ 5 , 6 ] + [ 2 4 ] ⋅ [ 7 , 8 ] C=\begin{bmatrix} 1 \\ 3 \end{bmatrix} \cdot \begin{bmatrix} 5,6 \end{bmatrix} + \begin{bmatrix} 2 \\ 4 \end{bmatrix} \cdot \begin{bmatrix} 7,8 \end{bmatrix} C=[13]⋅[5,6]+[24]⋅[7,8]
[ 1 ∗ [ 5 6 ] 3 ∗ [ 5 6 ] ] + [ 2 ∗ [ 7 8 ] 4 ∗ [ 7 8 ] ] \begin{bmatrix} 1*[5&6] \\ 3*[5&6] \end{bmatrix} + \begin{bmatrix} 2*[7&8] \\ 4*[7&8] \end{bmatrix} [1∗[53∗[56]6]]+[2∗[74∗[78]8]]
[ 1 ∗ 5 1 ∗ 6 3 ∗ 5 3 ∗ 6 ] + [ 2 ∗ 7 2 ∗ 8 4 ∗ 7 4 ∗ 8 ] = [ 19 22 43 50 ] \begin{bmatrix} 1*5 & 1*6 \\ 3*5 & 3*6 \end{bmatrix} + \begin{bmatrix} 2*7 & 2*8 \\ 4*7 & 4*8 \end{bmatrix} = \begin{bmatrix} 19 & 22 \\ 43 & 50 \end{bmatrix} [1∗53∗51∗63∗6]+[2∗74∗72∗84∗8]=[19432250]
如果 A A A 是下三角矩阵,即包含 Mask 信息,Decoder 无法观察到之后的推理部分,则 A ⋅ B A \cdot B A⋅B,输出:
A ⋅ B = [ a 1 , 1 B 1 a 2 , 1 B 2 + a 2 , 2 B 2 ⋯ a m , 1 B n + a m , 2 B n + ⋯ + a m , n B n ] A \cdot B = \left[ \begin{array}{llll} a_{1,1}B_{1}\\ a_{2,1}B_{2} + a_{2,2}B_{2}\\ \cdots \\ a_{m,1}B_{n} + a_{m,2}B_{n} + \cdots + a_{m,n}B_{n} \end{array} \right] A⋅B= a1,1B1a2,1B2+a2,2B2⋯am,1Bn+am,2Bn+⋯+am,nBn
2. 推理
第1步:
在 Decoder 解码过程中,只关注 Transformer 的 自注意力(Self-Attention),输入第 1 个 Token,将 Token 转换成 输入特征 I n p u t 1 = [ 1 , d e m b ] Input_{1}=[1,d_{emb}] Input1=[1,demb],暂时忽略 batch_size
, d e m b d_{emb} demb 表示 Embedding Size。
-
输入特征 I n p u t 0 = [ 1 , d e m b ] Input_{0}=[1,d_{emb}] Input0=[1,demb],乘以权重 W = [ d e m b , 3 ∗ d e m b ] W=[d_{emb}, 3*d_{emb}] W=[demb,3∗demb] (已训练完成,值是固定的),输出维度 [ 1 , 3 ∗ d e m b ] [1, 3*d_{emb}] [1,3∗demb],即作为 Q\K\V,每个向量 [ 1 , d e m b ] [1,d_{emb}] [1,demb]。
- Q 1 = [ 1 , d e m b ] Q_{1}=[1,d_{emb}] Q1=[1,demb]、 K 1 = [ 1 , d e m b ] K_{1}=[1,d_{emb}] K1=[1,demb]、 V 1 = [ 1 , d e m d ] V_{1}=[1,d_{emd}] V1=[1,demd],只与输入特征 I n p u t 0 Input_{0} Input0 的 Embedding 相关。
-
根据 Self-Attention 的公式,忽略 d \sqrt{d} d ,只有1维,mask 不起作用,即
A t t ( Q , K , V ) = s o f t m a x ( Q K ⊤ + m a s k ) ∗ V A t t 1 ( Q , K , V ) = s o f t m a x ( Q 1 K 1 ⊤ ) V 1 其中 s o f t m a x ( x i ) = e x i ∑ j = 1 n e x j Att(Q,K,V)=softmax(QK^{\top}+mask)*V \\ Att_{1}(Q,K,V)=softmax(Q_{1}K_{1}^{\top})V_{1} \\ 其中 \ softmax(x_i) = \frac{e^{x_i}}{\sum_{j=1}^{n} e^{x_j}} Att(Q,K,V)=softmax(QK⊤+mask)∗VAtt1(Q,K,V)=softmax(Q1K1⊤)V1其中 softmax(xi)=∑j=1nexjexi -
A t t 0 Att_{0} Att0 ( [ 1 , d e m b ] [1,d_{emb}] [1,demb]) 经过一系列推理,最后输出 [ 1 , d v ] [1, d_{v}] [1,dv], d v d_{v} dv 是全部词元 Token 的数量,根据概率值即可获得最后的 Token。
第 2 步:
将第 1 步输出的 Token 转换成 [ 1 , d e m b ] [1,d_{emb}] [1,demb],与第 1 步组合至一起,即 输入特征 I n p u t 1 = [ 2 , d e m b ] Input_{1}=[2,d_{emb}] Input1=[2,demb]
-
输入特征 I n p u t 1 = [ 2 , d e m b ] Input_{1}=[2,d_{emb}] Input1=[2,demb],乘以权重 W = [ d e m b , 3 ∗ d e m b ] W=[d_{emb}, 3*d_{emb}] W=[demb,3∗demb],权重是固定的,因此只需要计算第 2 个输入的特征 [ 1 , d e m b ] [1,d_{emb}] [1,demb],第 1 个不需要计算,也就是说 Q\K\V 的维度是 [ 2 , d e m b ] [2, d_{emb}] [2,demb],只需计算一次即可,剩余的可以直接 c o n c a t concat concat 到一起。
-
根据 Self-Attention 的公式,忽略 d \sqrt{d} d ,注意第1行,已经计算,第2行,需要使用 Q 2 Q_{2} Q2、 K 2 K_{2} K2、 V 2 V_{2} V2,进行计算,即:
A t t 2 ( Q , K , V ) = s o f t m a x ( Q K ⊤ + m a s k ) ∗ V s o f t m a x ( [ Q 1 K 1 ⊤ Q 2 K 1 ⊤ + Q 2 K 2 ⊤ ] ) ⋅ [ V 1 V 2 ] = [ s o f t m a x ( Q 1 K 1 ⊤ ) V 1 s o f t m a x ( Q 2 K 1 ⊤ ) V 1 + s o f t m a x ( Q 2 K 2 ⊤ ) V 2 ] = [ A t t 1 ( Q , K , V ) s o f t m a x ( Q 2 K 1 ⊤ ) V 1 + s o f t m a x ( Q 2 K 2 ⊤ ) V 2 ] Att_{2}(Q,K,V) = softmax(QK^{\top}+mask)*V \\ softmax(\left[ \begin{array}{ll} Q_{1}K_{1}^{\top}\\ Q_{2}K_{1}^{\top} + Q_{2}K_{2}^{\top}\\ \end{array} \right]) \cdot \begin{bmatrix} V_{1} \\ V_{2} \\ \end{bmatrix} \\= \left[ \begin{array}{ll} softmax(Q_{1}K_{1}^{\top})V_{1}\\ softmax(Q_{2}K_{1}^{\top})V_{1} + softmax(Q_{2}K_{2}^{\top})V_{2}\\ \end{array} \right] \\ = \left[ \begin{array}{} Att_{1}(Q,K,V) \\ softmax(Q_{2}K_{1}^{\top})V_{1} + softmax(Q_{2}K_{2}^{\top})V_{2}\\ \end{array} \right] Att2(Q,K,V)=softmax(QK⊤+mask)∗Vsoftmax([Q1K1⊤Q2K1⊤+Q2K2⊤])⋅[V1V2]=[softmax(Q1K1⊤)V1softmax(Q2K1⊤)V1+softmax(Q2K2⊤)V2]=[Att1(Q,K,V)softmax(Q2K1⊤)V1+softmax(Q2K2⊤)V2] -
KV 都是成对出现的,如果 缓存 KV,则可以加快推理速度。
第 3 步:重复进行。
3. 缓存占用
关于 Llama3 的 KV Cache 源码,参考 model.py:
python
xq = xq.view(bsz, seqlen, self.n_local_heads, self.head_dim)
xk = xk.view(bsz, seqlen, self.n_local_kv_heads, self.head_dim)
xv = xv.view(bsz, seqlen, self.n_local_kv_heads, self.head_dim)
xq, xk = apply_rotary_emb(xq, xk, freqs_cis=freqs_cis)
self.cache_k = self.cache_k.to(xq)
self.cache_v = self.cache_v.to(xq)
self.cache_k[:bsz, start_pos : start_pos + seqlen] = xk
self.cache_v[:bsz, start_pos : start_pos + seqlen] = xv
keys = self.cache_k[:bsz, : start_pos + seqlen]
values = self.cache_v[:bsz, : start_pos + seqlen]
关于 KV 的缓存内存占用:
相关参数 batch_size=32
,head=32
,layer=32
,dim_size=4096
,seq_length=2048
,float32(4个字节)类,计算 KV cache 的缓存占用:
M = 2 ∗ N b s ∗ ( N d i m / N h e a d ∗ N h e a d ) ∗ N l a y e r ∗ N s e q ∗ 4 = 2 ∗ 32 ∗ 4096 ∗ 32 ∗ 2048 ∗ 4 / 1024 / 1024 / 1024 = 64 G M=2*N_{bs}*(N_{dim}/N_{head}*N_{head})*N_{layer}*N_{seq}*4 \\ =2*32*4096*32*2048*4/1024/1024/1024=64G M=2∗Nbs∗(Ndim/Nhead∗Nhead)∗Nlayer∗Nseq∗4=2∗32∗4096∗32∗2048∗4/1024/1024/1024=64G
也就是说 head 数量无关,因为维度除以 Head 再乘以 Head。Llama3 使用 GQA (Grouped Query Attention) 分组查询注意力机制,降低 4 倍的 KV Cache,head=32,kv_head=8
,即 scale=head/kv_head=4
。
参考: