【测试环境】
matlab2023a
【源码文件截图】
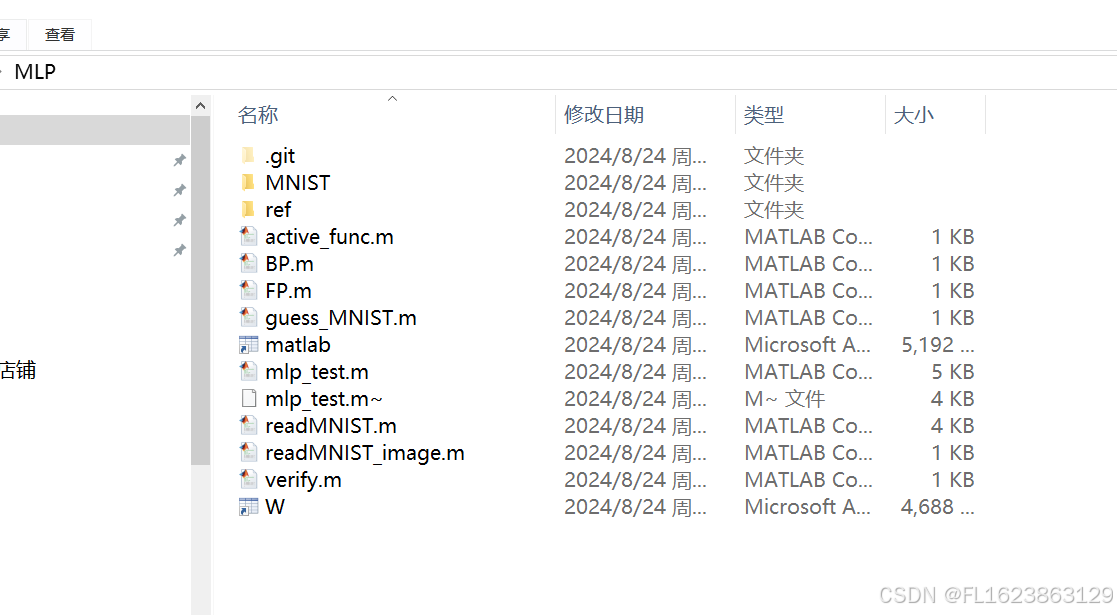
【实现部分代码】
mlp_test.m
%% MLP 2-layer to test XOR
clear;
clc;
Mode = 'MNIST'
%Mode = 'XOR'
if (strcmp(Mode,'MNIST'))
% Load the digits into workspace (MNIST Test, from
% http://yann.lecun.com/exdb/mnist/)
num_train = 1000;
[train_IMG,train_labels,test_IMG,test_labels] = readMNIST(num_train);
input =cell(num_train,1);
output =cell(num_train,1);
test_input=cell(length(test_IMG),1);
test_output=cell(length(test_labels),1);
for i=1:num_train
%input_img = double(train_IMG{i});
%Pre processing - prewitt
input_img = edge(train_IMG{i},'prewitt');
[width height] = size(input_img);
img_vec = reshape(input_img,1,width*height);
input{i}=double(img_vec);
labels_arr = zeros(1,10);
labels_arr(train_labels(i)+1)=1;
output{i} = labels_arr;
end
for i=1:length(test_input)
%input_img = double(test_IMG{i});
%Pre processing - prewitt
input_img = edge(test_IMG{i},'prewitt');
[width height] = size(input_img);
img_vec = reshape(input_img,1,width*height);
test_input{i} = double(img_vec);
labels_arr = zeros(1,10);
labels_arr(test_labels(i)+1)=1;
test_output{i} = labels_arr;
end
elseif (strcmp(Mode,'XOR'))
num_train = 4;
input =cell(num_train,1);
output =cell(num_train,1);
input{1} = [0 0];
input{2} = [0 1];
input{3} = [1 0];
input{4} = [1 1];
output{1} = [0];
output{2} = [1];
output{3} = [1];
output{4} = [0];
end
if (length(input)~=length(output))
error('len_input does not equal to len_output');
end
%% Determine # of nodes in hidden layer & output layer
num_node_il = length(input{1});
%num_node_hl = [num_node_il*2];
num_node_hl = [num_node_il];
num_node_ol = length(output{1});
set_node =[num_node_il num_node_hl num_node_ol];
%% Init. template (random)
rand('state',sum(100*clock));
num_layer = length(set_node);
W=cell(num_layer-1,1);
B=cell(num_layer-1);
for i=1:num_layer-1
%% [Xavier10] shows that the interval ~ from https://deeplearning.net/tutorial/mlp.html
min_W = -4*sqrt(6/(set_node(i)+set_node(i+1)));
max_W = 4*sqrt(6/(set_node(i)+set_node(i+1)));
W{i} = min_W+(2*max_W).*rand(set_node(i),set_node(i+1));
B{i} = rand(1,set_node(i+1));
end
%% Learning coeff = 0.7 & Iteration = 10
% 141108, Success rate = 0.725
%lrn_rate = 0.3;
%max_iter = 100;
lrn_rate = 0.3;
max_iter = 50;%最大迭代次数,越大训练时间越长精度越高
tic
Act=cell(num_layer,1);
Err=cell(num_layer-1,1);
err_trace=[];
for index_inter= 1:max_iter
if mod(index_inter,50) ==0
index_inter
end
Act_trace=[];
Train_trace = [];
for j= 1:num_train
P = randperm(num_train);
train_input = input{P(j)};
train_output = output{P(j)};
% Forward Propagation
[Act] = FP(train_input,Act,W,B,num_layer);
% Backward Propagation & Template update
[W,B,Err] = BP(train_output,Act,W,B,num_layer,lrn_rate,Err);
% Debug
[row,col]=find(Act{end}==max(Act{end}));
Act_trace(end+1)=col-1;
[row,col]=find(train_output==max(train_output));
Train_trace(end+1)=col-1;
end
All_arr(index_inter).act = Act_trace;
All_arr(index_inter).train=Train_trace;
All_arr(index_inter).err = Act_trace-Train_trace;
end
toc
save
disp('Training Ends')
if (strcmp(Mode,'XOR'))
grid = [0:0.01:1];
Z=-1*ones(length(grid),length(grid));
for i=1:length(grid)
for j=1:length(grid)
test = [grid(i) grid(j)];
Act_new = FP(test,Act,W,B,num_layer);
Z(i,j) = Act_new{3};
end
end
[X,Y] = meshgrid(grid);
mesh(X,Y,Z)
elseif (strcmp(Mode,'MNIST'))
Guess_arr = [];
for i=1:length(test_input)
[guess_result] = FP(test_input{i},Act,W,B,num_layer);
[row,col]=find(guess_result{end}==max(guess_result{end}));
Guess_arr(end+1)=col-1;
end
end
Z=zeros(10,10);
for i =1:length(test_labels)
Z(Guess_arr(i)+1,test_labels(i)+1)=Z(Guess_arr(i)+1,test_labels(i)+1)+1;
end
Abs_err = Guess_arr-double(test_labels)';
success_rate = sum(Guess_arr-double(test_labels)'==0)/1000
%plot(abs(Guess_arr-double(test_labels)'))
%figure(1);scatter((Guess_arr*10),test_labels)
%figure(2);plot(err_trace);
运行结果:
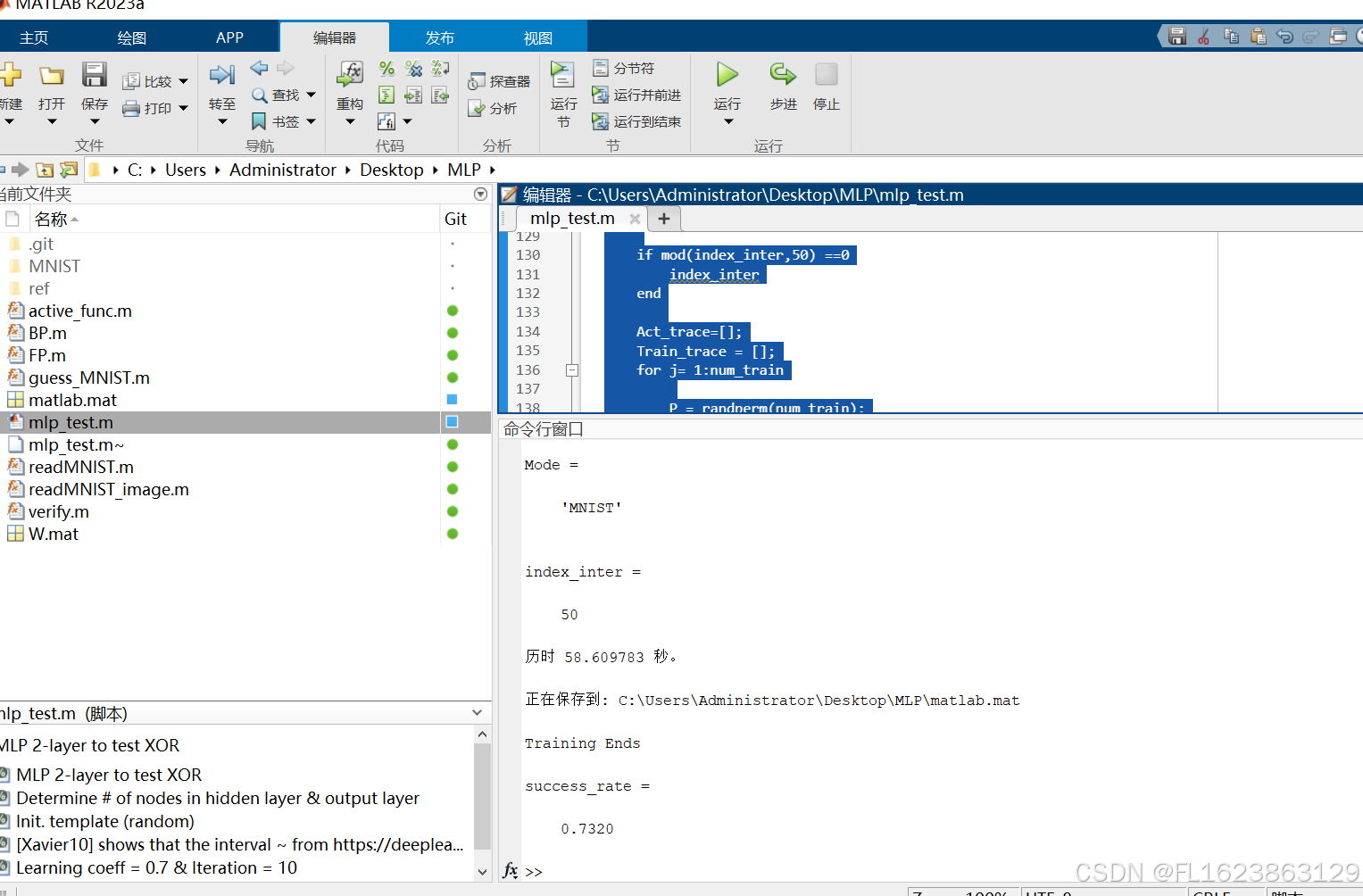
【源码下载地址】 https://download.csdn.net/download/FL1623863129/88600486