参考
https://apple.github.io/coremltools/docs-guides/source/opt-palettization-overview.html
https://apple.github.io/coremltools/docs-guides/source/opt-palettization-algos.html
Apple Intelligence Foundation Language Models
GPTVQ: The Blessing of Dimensionality for LLM Quantization
向量量化基础
针对大语言模型权重的4bit量化,除了常规的广泛使用的group-wise均匀量化,如GPTQ, AWQ等等,苹果提出了一种称为Palettization的lookup table (LUT)查找表量化技术,高通也提出了新的一种向量量化技术,其实这两种技术原理基本上大体是相同的。
首先,均匀量化就不多说了,基于一个仿射变换来映射量化后的整数值和非量化的浮点数值,一般采用r=(q-z)*s,可以参考深度学习模型量化基础_深度学习 量化-CSDN博客
常规的查找表量化则是一种非均匀量化,比如同样的4bit量化为0-15的整数,查找表LUT可以建立这0-15的每个整数到他们分别对应的一个浮点数的对应关系。这个对应关系可以是任意采样方式的,因此为非均匀量化。
Vector Quantization (VQ)向量量化,其实第一性原理也挺简单的:相对于上面所描述的标量量化把一个标量的浮点映射到其对应的一个整数。而向量量化则是要把一个浮点的d维向量映射为一个n bit的标量整数。具体实现通常采用聚类算法,在d维空间中进行聚类为k=2^n个类别,每个聚类中心采用一个整数表示,从而建立一个整数到聚类中心d维向量的查找表。量化阶段根据输入的d维向量到每个聚类中心的距离分配其对应的量化值,而反量化则根据每个量化后的整数,根据查找表恢复聚类中心的d维向量,这显然是一个非均匀有损量化。
Product quantization:把一个大D维的向量均匀split为多个更短的d维向量,每个d维向量采用VQ量化。Vector Quantization和Product quantization本身是从其他领域引入到大语言模型量化,麻烦读者查询相关的资料进行进一步了解。
Vector Quantization量化LLM权重
假设每2个元素一起作为一个向量,每个元素4bit,那么2个元素一起量化就有4x2=8bit的budget,那么LUT查找表大小将为2^8=256大小。而维度更高,LUT粒度更小。但是LUT大小将剧烈增长:
we use bits per dimension (b) to indicate the number of index bits stored for each individual weight. This means that, for VQ with dimension d, the total number of index bits is d × b, and the number of centroids in a codebook is k = 2^(d×b).
可以看到高通的这个方法基本上只能用于2维向量量化,更高维度意味着急剧增长的查找表大小。
苹果的方法
只有一些粗浅的博客描述,没有找到相关的论文。但是从这些内容可以看到应该也是使用了向量量化的方法,但是其采用了更高维度的向量量化,而高维度量化方案应该跟高通有较大区别(似乎高维度向量仍然采用2d聚类)。
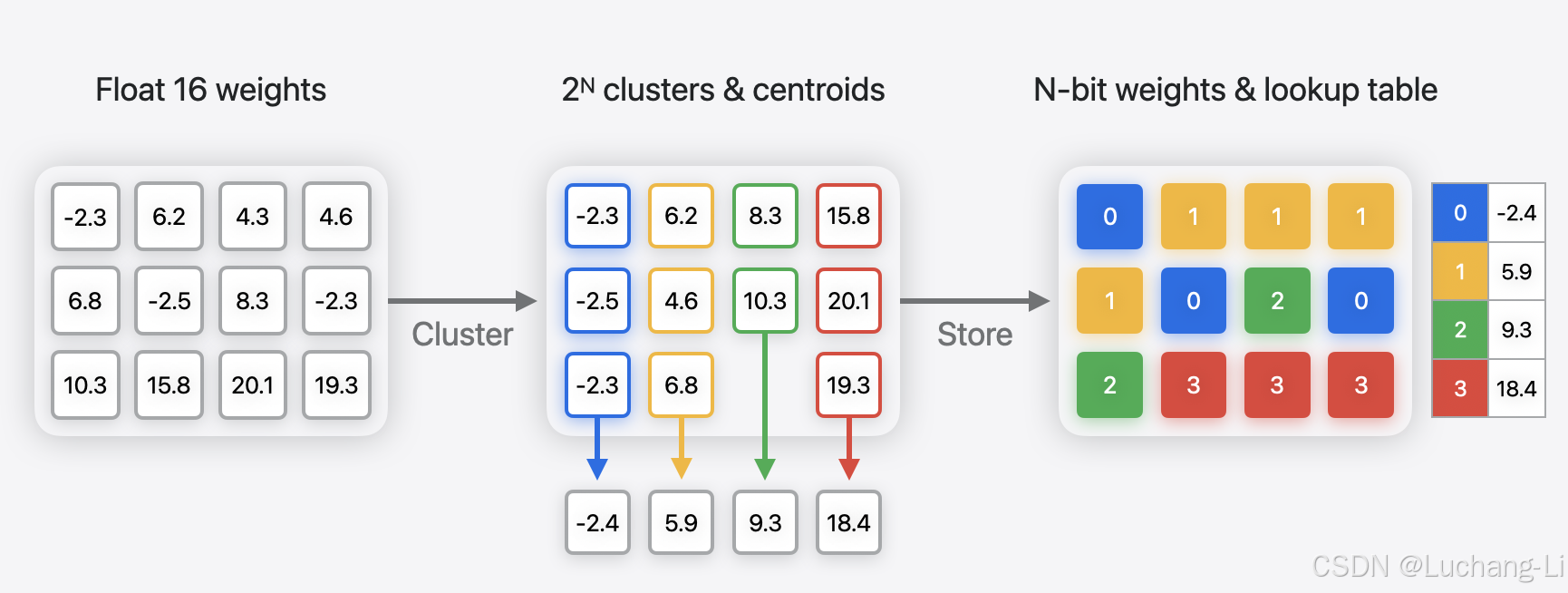
Palettization, also referred to as weight clustering, compresses a model by clustering the model's float
weights, and creating a lookup table (LUT) of centroids, and then storing the original weight values with indices pointing to the entries in the LUT.
Weights with similar values are grouped together and represented using the value of the cluster centroid they belong to, as shown in the following figure. The original weight matrix is converted to an index table in which each element points to the corresponding cluster center.
N={1,2,3,4,6,8}
are supported, where N
is the number of bits used for palettization.
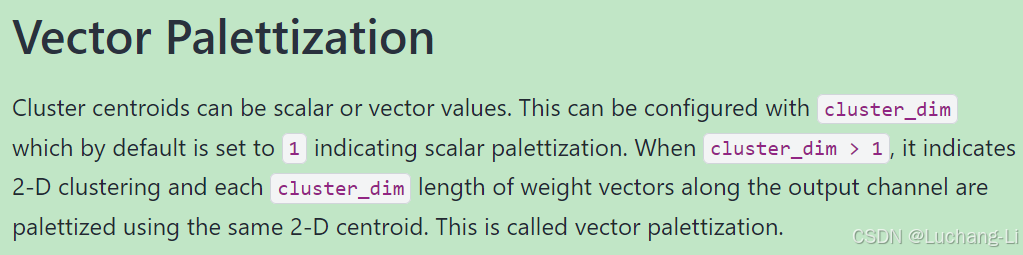
