Title
题目
MIST: Multi-instance selective transformer for histopathological subtype prediction
MIST:用于组织病理学亚型预测的多实例选择性Transformer
01
文献速递介绍
组织病理学亚型预测在癌症疾病的诊断和治疗中具有重要的临床意义。组织病理学亚型预测旨在识别全视野图像(WSI)中与病理组织相关的不同子类别(图1(1)),例如,正常黏膜、碎屑、病理性良性组织、淋巴细胞和侵袭性癌(Han等,2022)。组织病理学亚型预测通常依赖于临床决策,以制定最佳治疗方案(Han等,2017)。通过了解组织病理学亚型,病理学家可以及早控制肿瘤细胞的转移,并根据多种癌症的特殊临床表现和预后结果制定有效的治疗方案(Han等,2017),如乳腺癌和结直肠癌。此外,组织病理学亚型的预测为肿瘤微环境分析提供了新的见解,并对临床终点产生了重大影响(Gurcan等,2009;Kather等,2019,2018,2017)。
Abatract
摘要
Accurate histopathological subtype prediction is clinically significant for cancer diagnosis and tumor microenvironment analysis. However, achieving accurate histopathological subtype prediction is a challengingtask due to (1) instance-level discrimination of histopathological images, (2) low inter-class and large intraclass variances among histopathological images in their shape and chromatin texture, and (3) heterogeneousfeature distribution over different images. In this paper, we formulate subtype prediction as fine-grainedrepresentation learning and propose a novel multi-instance selective transformer (MIST) framework, effectivelyachieving accurate histopathological subtype prediction. The proposed MIST designs an effective selective selfattention mechanism with multi-instance learning (MIL) and vision transformer (ViT) to adaptive identifyinformative instances for fine-grained representation. Innovatively, the MIST entrusts each instance withdifferent contributions to the bag representation based on its interactions with instances and bags. Specifically,a SiT module with selective multi-head self-attention (S-MSA) is well-designed to identify the representativeinstances by modeling the instance-to-instance interactions. On the contrary, a MIFD module with theinformation bottleneck is proposed to learn the discriminative fine-grained representation for histopathologicalimages by modeling instance-to-bag interactions with the selected instances. Substantial experiments on fiveclinical benchmarks demonstrate that the MIST achieves accurate histopathological subtype prediction andobtains state-of-the-art performance with an accuracy of 0.936. The MIST shows great potential to handlefine-grained medical image analysis, such as histopathological subtype prediction in clinical applications.
准确的组织病理学亚型预测对于癌症诊断和肿瘤微环境分析具有重要的临床意义。然而,由于以下几个挑战,实现准确的组织病理学亚型预测是一项艰巨的任务:(1) 组织病理学图像的实例级别区分,(2) 组织病理学图像在形状和染色质纹理上的类间差异小而类内差异大,以及(3) 不同图像之间异质特征分布。在本文中,我们将亚型预测表述为细粒度表征学习,并提出了一种新颖的多实例选择性Transformer(MIST)框架,有效实现了准确的组织病理学亚型预测。所提出的MIST设计了一种结合多实例学习(MIL)和视觉Transformer(ViT)的有效选择性自注意机制,自适应地识别出细粒度表征的有用实例。创新性地,MIST根据实例与袋之间的相互作用赋予每个实例在袋表示中的不同贡献。具体而言,MIST中的SiT模块设计了选择性多头自注意力机制(S-MSA),通过建模实例与实例之间的相互作用来识别具有代表性的实例。相反,MIFD模块通过信息瓶颈策略,基于与所选实例的实例与袋之间的相互作用,学习组织病理图像的判别性细粒度表征。在五个临床基准上的大量实验表明,MIST实现了准确的组织病理学亚型预测,并以0.936的准确率获得了最先进的性能。MIST显示了在处理细粒度医学图像分析(如临床应用中的组织病理学亚型预测)方面的巨大潜力。
Method
方法
The proposed MIST (Fig. 3) formulates histopathological subtypeprediction as fine-grained representation learning and achieveshistopathological subtype prediction by constructing a multiple-stagevision transformer coupled with multi-instance learning. The newlydesigned MIST learns the instance-level fine-grained features by adaptive entrusting each instance with different contributions for thehistopathological image representation. Therefore, the MIST has threetightly connected components:
(1) selective instance transformer(SiT) with selective self-attention mechanism (S-MSA) to adaptivelyidentify the representative instances from the bag for discriminative representation of the histopathological image. The SiT conductsinstance selection by modeling the instance-to-instance interactionswith a selective self-attention mechanism; (2) multiple instance feature decoupling (MIFD) to gradually learn instance-level fine-grainedfeature representation for histopathological subtype prediction by modeling the instance-to-bag interactions;
(3) loss function with information bottleneckto classify the histopathological subtypes with thebag representation learned with MIST by integrating the instance-toinstance and instance-to-bag interactions.
所提出的MIST(图3)将组织病理学亚型预测表述为细粒度表征学习,并通过构建多阶段视觉Transformer结合多实例学习来实现组织病理学亚型预测。新设计的MIST通过自适应地赋予每个实例不同的贡献,来学习实例级别的细粒度特征,以表征组织病理学图像。因此,MIST包含三个紧密连接的组件:
(1) 选择性实例Transformer(SiT):结合选择性自注意机制(S-MSA),自适应地从袋中识别代表性的实例,以实现组织病理学图像的判别性表征。SiT通过选择性自注意机制建模实例与实例之间的相互作用来进行实例选择;
(2) 多实例特征解耦(MIFD):通过建模实例与袋之间的相互作用,逐步学习用于组织病理学亚型预测的实例级别细粒度特征表征;
(3) 结合信息瓶颈的损失函数:通过整合实例与实例以及实例与袋之间的相互作用,利用MIST学习到的袋表示来分类组织病理学亚型。
Conclusion
结论
In this paper, the multi-instance selection transformer (MIST) framework is proposed to achieve histopathological subtype prediction forcancer prognosis. MIST designs a novel structure to simultaneouslymodel the instance-to-bag and instance-to-instance interactions, creatively proposing a selective instance transformer (SiT) with selective multi-head self-attention (S-MSA) to progressively extract the significant instance-level feature in the instance-to-bag interactions forfine-grained representation, developing a multiple instance feature decoupling (MIFD) progressively learns the task-related representationby leveraging bag-level prior knowledge for redundancy alleviationthat prevents prediction performance degradation by modeling theinstance-to-bag interactions, constructing an information bottleneckloss function for guiding the network to learn a minimum adequatediscriminative representation. Experimental results show that MIST iscapable of achieving impressive performance for fine-grained prediction of the histopathological image. The proposed method has greatpotential in clinical cancer diagnoses.
在本文中,提出了多实例选择性Transformer(MIST)框架,以实现癌症预后中的组织病理学亚型预测。MIST设计了一种新颖的结构,能够同时建模实例与袋以及实例与实例之间的交互,创造性地提出了选择性实例Transformer(SiT),结合选择性多头自注意力机制(S-MSA),逐步在实例与袋的交互中提取显著的实例级特征以实现细粒度表征。此外,MIST还开发了多实例特征解耦(MIFD)模块,通过利用袋级先验知识进行冗余缓解,逐步学习任务相关的表征,从而防止预测性能下降,同时建构了信息瓶颈损失函数,用于引导网络学习最小的、足够的判别性表征。实验结果表明,MIST在组织病理学图像的细粒度预测中表现出色。该方法在临床癌症诊断中具有巨大的潜力。
Figure
图
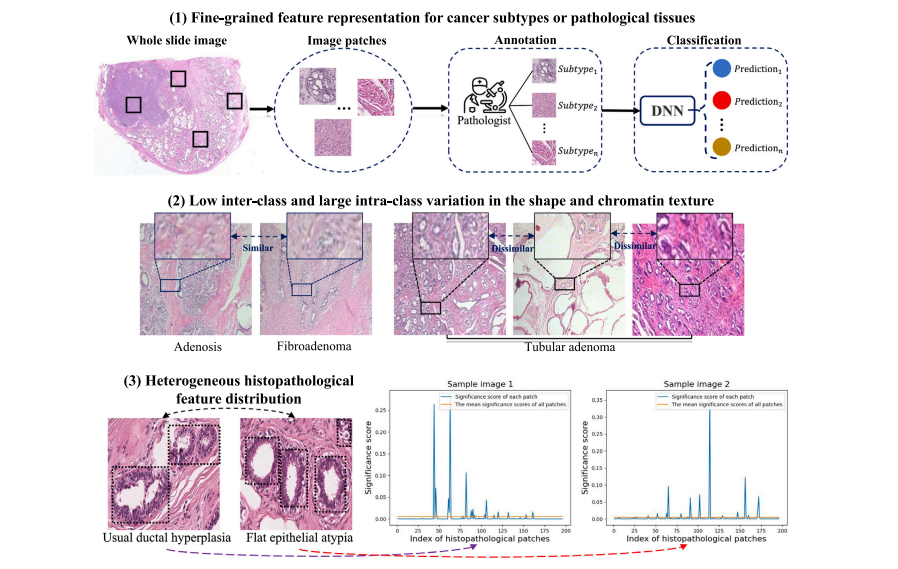
Fig. 1. Three challenges hinder the accurate histopathological subtype prediction. (1) Fine-grained feature representation for cancer subtypes or pathological tissues. (2) Lowinter-class and large intra-class variances in their shape and chromatin texture, which are extremely hard to recognize. (3) Heterogeneous histopathological feature distributionover different images that uniform feature extraction is prone to lead to degraded prediction performance which needs to adaptively select highly significant pathological regionsin different histopathology images.
图1. 三个挑战阻碍了准确的组织病理学亚型预测:(1) 癌症亚型或病理组织的细粒度特征表示。(2) 形状和染色质纹理的类间差异小、类内差异大,这些特征非常难以识别。(3) 不同图像中异质的组织病理学特征分布,统一的特征提取容易导致预测性能下降,需要自适应地选择不同组织病理学图像中高度重要的病理区域。
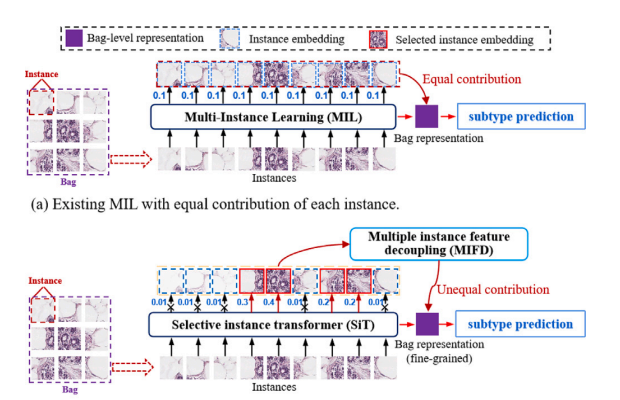
Fig. 2. Different from existing multi-instance learning (MIL), the proposed MISTinnovatively formulates subtype prediction as fine-grained representation learning withMIL and ViT, and constructs novel selective self-attention mechanism to conducthistopathological subtype prediction, where each instance conducts unequal contributions to the representation of the histopathological image by the instance selection andfeature decoupling.
图2. 与现有的多实例学习(MIL)方法不同,提出的MIST创新性地将亚型预测表述为结合MIL和视觉Transformer(ViT)的细粒度表征学习,并构建了新颖的选择性自注意机制来进行组织病理学亚型预测。通过实例选择和特征解耦,每个实例对组织病理学图像的表征贡献不均等。
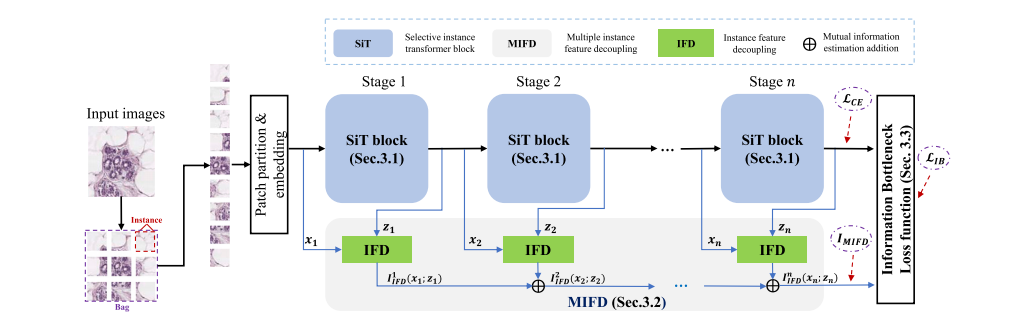
Fig. 3. The MIST formulates histopathological subtype prediction as a fine-grained representation learning problem and provides a novel learning paradigm of selective self-attentionmechanism by advancing vision transformer architecture and multi-instance learning (MIL). The MIST achieves histopathological subtype prediction by progressively learning thefine-grained histopathological representation. The MIST consists of three key components: (1) selective instance transformer (SiT) with S-MSA to adaptively identify the representativeinstances for the representation of the histopathological image by modeling the instance-to-instance interactions with selective self-attention mechanism; (2) multiple instance featuredecoupling (MIFD) to gradually learn instance-level fine-grained feature representation for histopathological subtype prediction by modeling the instance-to-bag interactions; (3)information bottleneck loss function to train the multiple-stage transformer with the idea of multi-instance learning by integrating the instance-to-instance and instance-to-baginteractions.
图3. MIST将组织病理学亚型预测表述为一个细粒度表征学习问题,并通过改进视觉Transformer架构和多实例学习(MIL)提供了一种新颖的选择性自注意机制学习范式。MIST通过逐步学习细粒度的组织病理学表征来实现亚型预测。MIST包括三个关键组件:(1) 选择性实例Transformer(SiT),通过选择性自注意机制建模实例与实例之间的相互作用,自适应地识别代表组织病理学图像表征的实例;(2) 多实例特征解耦(MIFD),通过建模实例与袋之间的相互作用,逐步学习用于组织病理学亚型预测的实例级别细粒度特征表征;(3) 信息瓶颈损失函数,通过整合实例与实例以及实例与袋之间的相互作用,以多实例学习的理念训练多阶段Transformer。
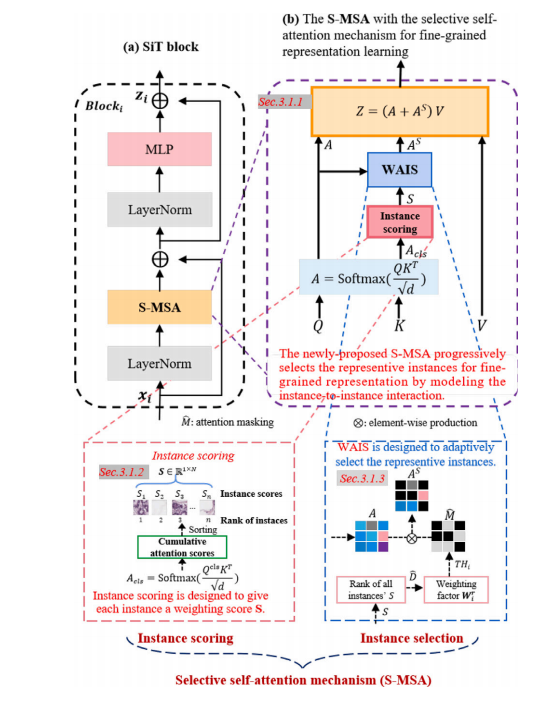
Fig. 4. (a) The SiT learns the fine-grained representation of the histopathologicalimage. (b) The S-MSA with selective self-attention mechanism, which consists ofinstance scoring and WAIS, progressively selects the representative instances forfine-grained representation by modeling the instance-to-instance interaction. Instancescoring gives each instance a score of significance and WAIS adaptively selects therepresentative instances as fine-grained features.
图4. (a) SiT学习组织病理学图像的细粒度表征。(b) S-MSA与选择性自注意机制相结合,通过实例评分和WAIS,逐步通过建模实例与实例之间的相互作用来选择用于细粒度表征的代表性实例。实例评分为每个实例赋予重要性评分,而WAIS则自适应地选择代表性实例作为细粒度特征。
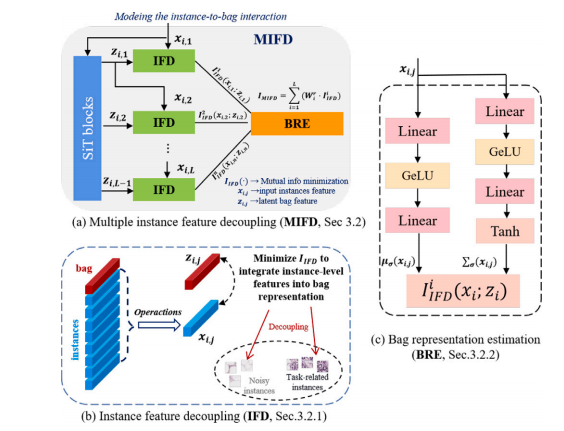
Fig. 5. The MIFD learns the fine-grained representation of histopathological imagesfor subtype prediction by integrating the discriminative instance-level features intobag representation with the instance-to-bag interaction. It reduces the correlationbetween bag-level representation and task-independent information of input instancefeatures in the instance-to-bag interaction, preventing performance degradation ofhistopathological subtype prediction.
图5. MIFD通过将判别性的实例级特征整合到袋表示中,并结合实例与袋之间的相互作用,学习组织病理学图像的细粒度表征以进行亚型预测。它减少了袋级表示与输入实例特征中与任务无关的信息之间的相关性,从而防止组织病理学亚型预测性能的下降。
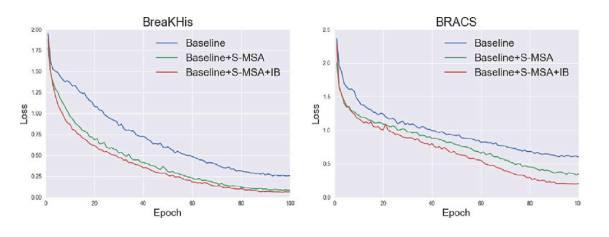
Fig. 6. The curve of train loss demonstrates significant improvements in the training convergence of the MIST with different modules on different datasets.
图6. 训练损失曲线显示了在不同数据集上,MIST结合不同模块在训练收敛性方面的显著提升。
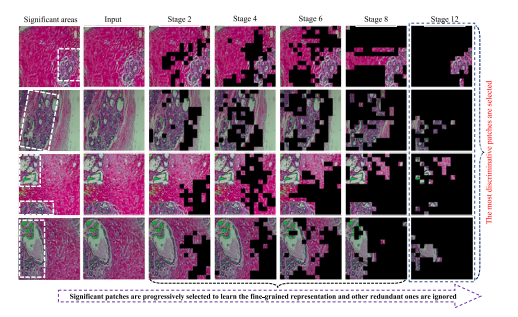
Fig. 7. Visualization results demonstrate that the MIST with WAIS of S-MSA progressively focuses on the most discriminative instances, where the dashed box representsthe significant area of histopathology and the masked regions represent the unessentialinstances that are discarded after the 12 stages. This phenomenon indicates that theS-MSA has adequate interpretability.
图7. 可视化结果表明,使用S-MSA的WAIS的MIST逐步聚焦于最具判别性的实例,其中虚线框表示组织病理学的重要区域,遮盖的区域表示在12个阶段后被舍弃的不重要实例。这一现象表明S-MSA具有足够的可解释性。
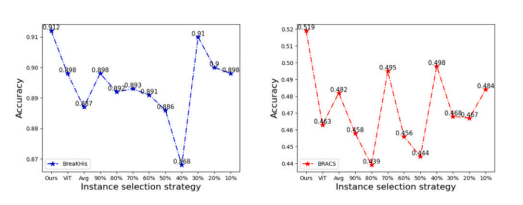
Fig. 8. The S-MSA of MIST yields the highest accuracy in histopathological subtypeprediction by capturing the discriminative subtle features, compared with differentinstance selection strategies i.e., fixed and average instance selection methods onBreaKHis and BRACS datasets.
图8. 在BreaKHis和BRACS数据集上,与不同的实例选择策略(例如,固定和平均实例选择方法)相比,MIST的S-MSA通过捕捉判别性细微特征,在组织病理学亚型预测中获得了最高的准确性。
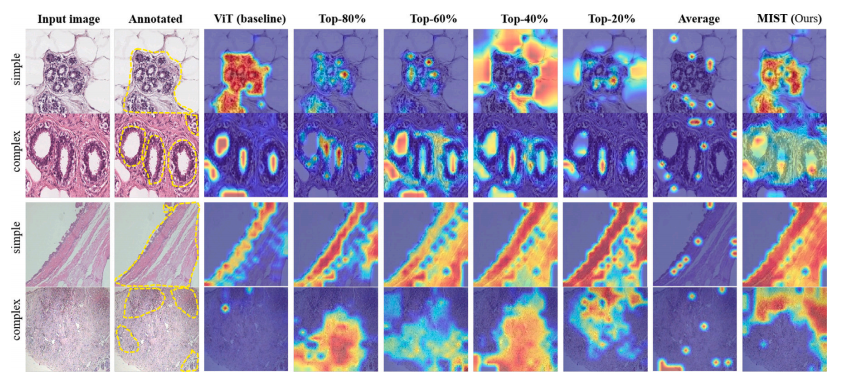
Fig. 9. Visualization results illustrate that owed to the proposed selective self-attention mechanism, the MIST can effectively identify the most informative regions in images witheither simple informative regions (in the first and third rows) or complex informative regions (in the second and last rows), comparing with different instance selection methods.Note that the second-column annotated images with dashed polygon represent the significant histopathological regions.
图9. 可视化结果显示,得益于提出的选择性自注意机制,MIST能够有效识别图像中最具信息量的区域,无论是简单的(第一和第三行)还是复杂的(第二和最后一行)信息区域,与不同的实例选择方法相比,表现更优。注意,第二列用虚线多边形标注的图像表示重要的组织病理学区域。
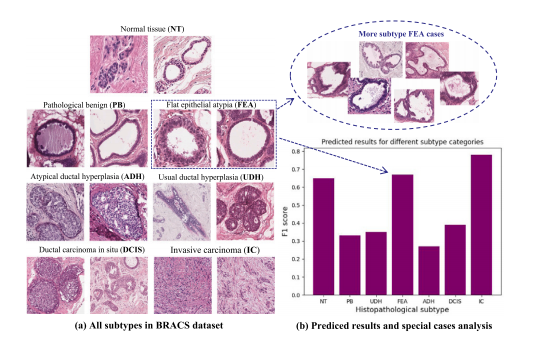
Fig. 10. Visualization of special histopathological subtype cases. (a) Several imagesof 7 different histopathological subtypes from the BRACS dataset, FEA subtype imagesusually have large background areas located in their centers. (b) Prediction results forthe seven different subtypes of the BRACS test set with bar chart presentation, ourMIST achieves the second-best result for special subtype cases whose background isincluded in the center.
图10. 特殊组织病理学亚型案例的可视化。(a) 来自BRACS数据集的7种不同组织病理学亚型的几张图像,FEA亚型的图像通常在中心区域有大面积的背景。(b) 采用柱状图展示的BRACS测试集中七种不同亚型的预测结果,对于背景包含在中心的特殊亚型案例,我们的MIST取得了第二好的结果。
Table
表
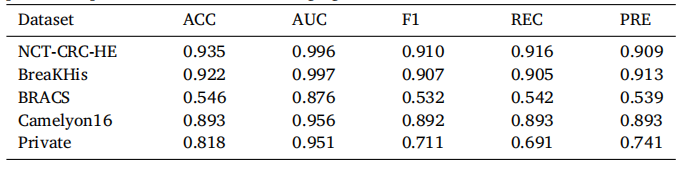
Table 1Experiment results illustrate MIST achieves advanced histopathological subtypeprediction performance on five challenging datasets
表1 实验结果表明,MIST在五个具有挑战性的数据集上实现了先进的组织病理学亚型预测性能。
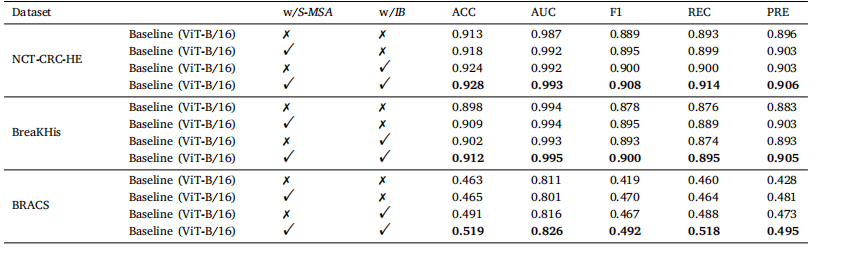
Table 2Performance of the MIST under different configurations for histopathological subtype prediction with five evaluation criteria on three datasets.
表2 在三个数据集上使用五个评估标准评估不同配置下MIST在组织病理学亚型预测中的性能表现。
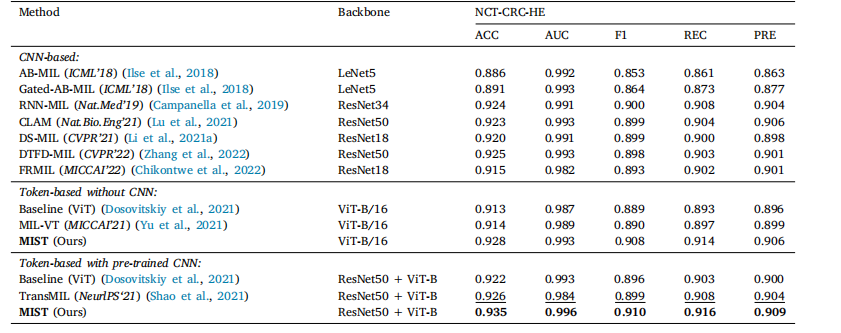
Table 3Experimental results show that the MIST obtains the competitive performance compared with the SOTA methods on the NCT-CRC-HE dataset.Notes: the bold indicates the SOTA result, while the underline states the second-best result.
表3 实验结果显示,MIST在NCT-CRC-HE数据集上与最先进的方法相比取得了具有竞争力的性能。注:粗体表示最先进的结果,下划线表示第二好的结果
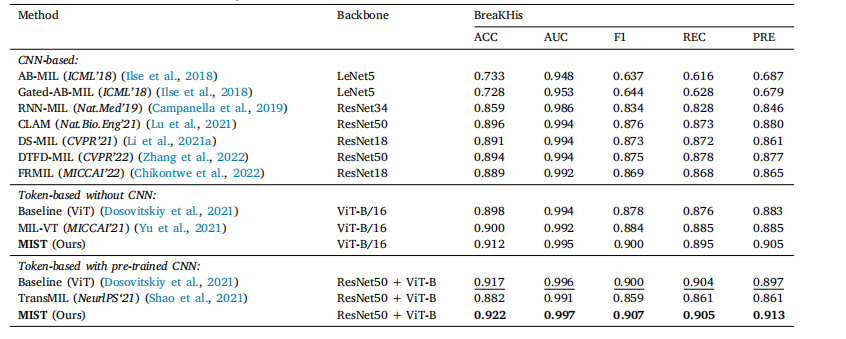
Table 4Experimental results show that the MIST obtains the competitive performance compared with the SOTA methods on the BreaKHis dataset.Notes: the bold indicates the SOTA result, while the underline states the second-best result.
表4 实验结果显示,MIST在BreaKHis数据集上与最先进的方法相比取得了具有竞争力的性能。注:粗体表示最先进的结果,下划线表示第二好的结果。
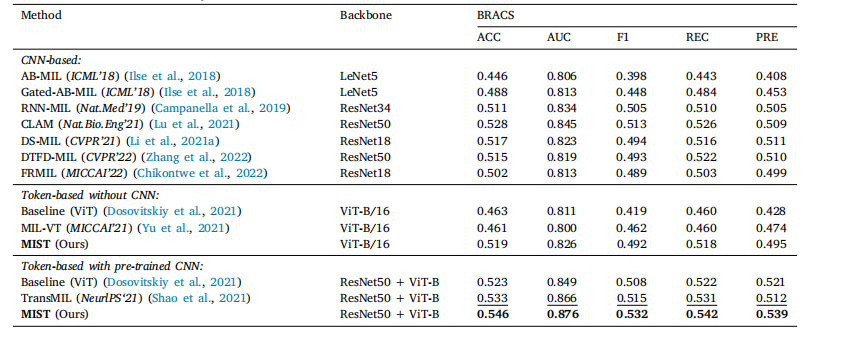
Table 5Experimental results show that the MIST obtains the competitive performance compared with the SOTA methods on the BRACS dataset. Notes:the bold indicates the SOTA result, while the underline states the second-best result.
表5 实验结果显示,MIST在BRACS数据集上与最先进的方法相比取得了具有竞争力的性能。注:粗体表示最先进的结果,下划线表示第二好的结果。
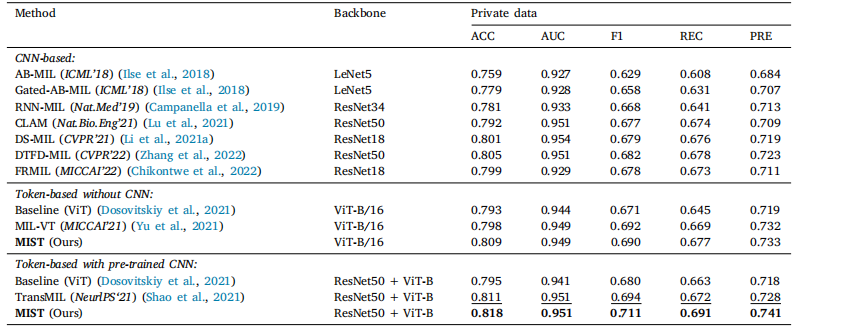
Table 6Experimental results show that the MIST obtains a competitive performance compared with the SOTA methods on the histopathological subtypedataset of private data from Fujian Medical University Union Hospital. Notes: the bold indicates the SOTA result, while the underline states thesecond-best result.
表6实验结果显示,MIST在福建医科大学附属协和医院的私有数据组织病理学亚型数据集上,与最先进的方法相比取得了具有竞争力的性能。注:粗体表示最先进的结果,下划线表示第二好的结果。
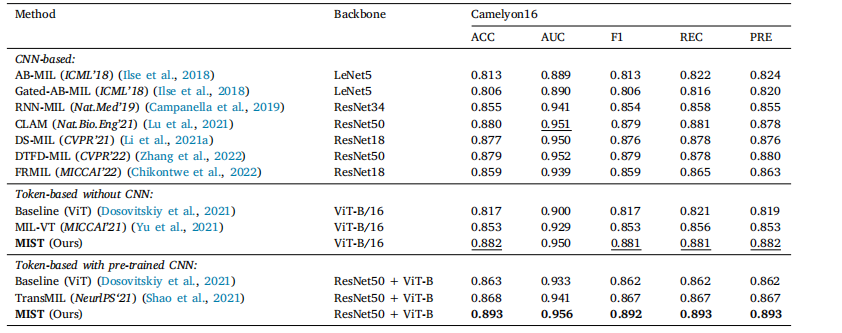
Table 7Experimental results show that the MIST obtains a competitive performance compared with the SOTA methods on the Camelyon16 dataset.Notes: the bold indicates the SOTA result, while the underline states the second-best result.
表7 实验结果显示,MIST在Camelyon16数据集上与最先进的方法相比取得了具有竞争力的性能。注:粗体表示最先进的结果,下划线表示第二好的结果。
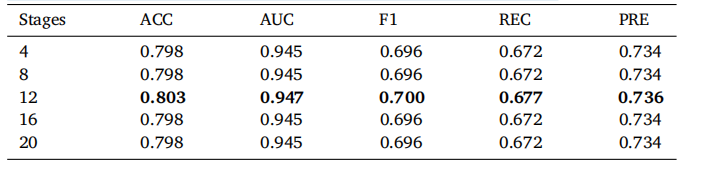
Table 8Experiments of S-MSA Sensitivity with different stages on histopathological subtypedataset of private data from Fujian Medical University Union Hospital.
表8 S-MSA在不同阶段对福建医科大学附属协和医院私有数据组织病理学亚型数据集的敏感性实验。
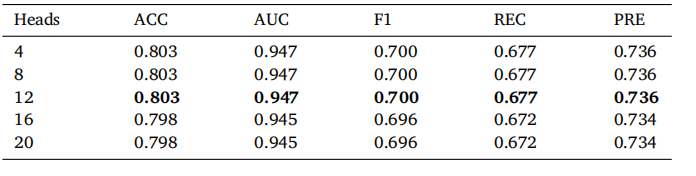
Table9ExperimentsofSMSASensitivitywithdifferentheadsonhistopathologicalsubtypedatasetofprivatedatafromFujianMedicalUniversityUnionHospital.
表9 S-MSA在不同头数下对福建医科大学附属协和医院私有数据组织病理学亚型数据集的敏感性实验。
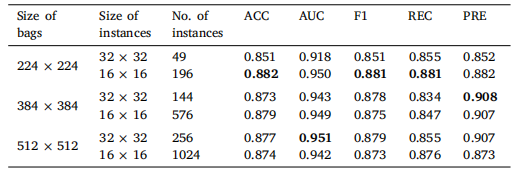
Table 10Experiments of Scalability of different size of bags and instances on Camelyon16dataset.
表10 在Camelyon16数据集上不同袋大小和实例数量的可扩展性实验。
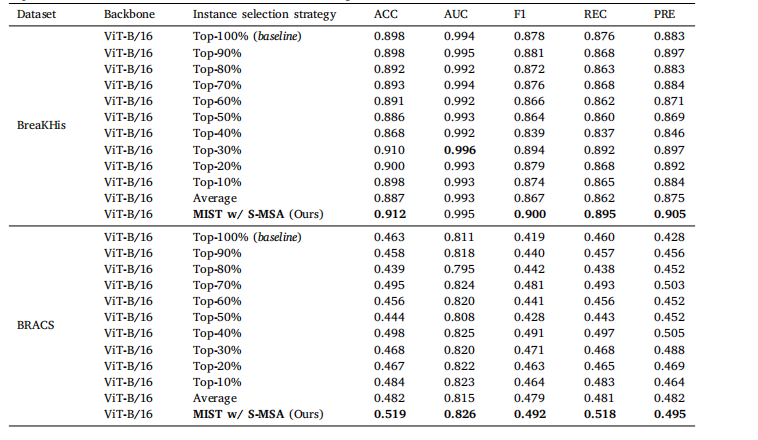
Table 11Experimental results under different instances selection strategies on BreaKHis and BRACS datasets.
表11 不同实例选择策略在BreaKHis和BRACS数据集上的实验结果。
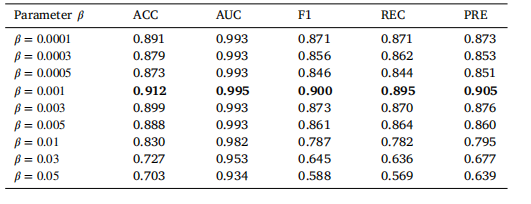
Table 12Experiments of Lagrange multipliers 𝛽 with different assignments on BreaKHis dataset.
表12 在BreaKHis数据集上对不同Lagrange乘数𝛽赋值的实验结果。
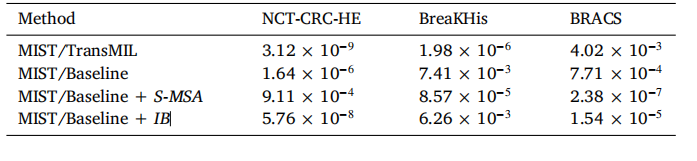
Table 13Statistical significance of MIST versus baseline model is examined by the t-test with asignificance level of 0.1. A lower p-value than 0.001 indicates that the MIST achievesa significantly different performance than the baseline model.
表13 通过t检验在显著性水平0.1下检验MIST与基线模型的统计显著性。p值低于0.001表示MIST的性能与基线模型之间存在显著差异。