一、本文介绍
本文记录的是基于AIFI模块的YOLOv9目标检测改进方法研究。AIFI
是RT-DETR
中高效混合编码器的一部分,利用其改进YOLOv9
模型,使网络在深层能够更好的捕捉到概念实体之间的联系,并有助于后续模块对对象进行定位和识别。
文章目录
- 一、本文介绍
- 二、AIFI设计原理
- 三、AIFI模块的实现代码
- 四、添加步骤
-
- [4.1 修改common.py](#4.1 修改common.py)
- [4.2 修改yolo.py](#4.2 修改yolo.py)
- 五、yaml模型文件
-
- [5.1 模型改进⭐](#5.1 模型改进⭐)
- 六、成功运行结果
二、AIFI设计原理
RT-DETR
模型结构:
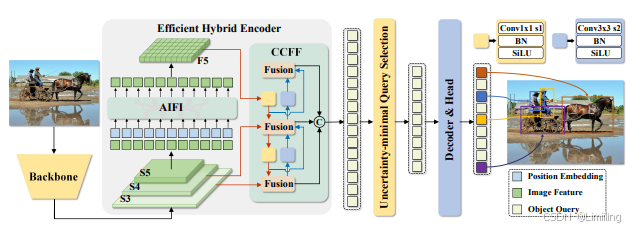
AIFI(Attention-based Intra-scale Feature Interaction)
模块的相关信息如下:
2.1、设计原理
AIFI
是RT-DETR
中高效混合编码器的一部分。为了克服多尺度Transformer编码器
中存在的计算瓶颈,RT-DETR
对编码器结构进行了重新思考。
由于从低级特征中提取出的高级特征包含了关于对象的丰富语义信息,对级联的多尺度特征进行特征交互是冗余的。因此,AIFI
基于此设计,通过使用单尺度Transformer
编码器仅在S5特征层
上进行尺度内交互,进一步降低了计算成本。
对高级特征应用自注意力操作,能够捕捉到概念实体之间的联系,这有助于后续模块对对象进行定位和识别。而低级特征由于缺乏语义概念,且与高级特征交互存在重复和混淆的风险,因此其尺度内交互是不必要的。
2.2、优势
与基准模型相比,AIFI不仅显著降低了延迟(快35%),而且提高了准确性(AP高0.4%)。
三、AIFI模块的实现代码
AIFI模块
的实现代码如下:
python
class TransformerEncoderLayer(nn.Module):
"""Defines a single layer of the transformer encoder."""
def __init__(self, c1, cm=2048, num_heads=8, dropout=0.0, act=nn.GELU(), normalize_before=False):
"""Initialize the TransformerEncoderLayer with specified parameters."""
super().__init__()
from ...utils.torch_utils import TORCH_1_9
if not TORCH_1_9:
raise ModuleNotFoundError(
"TransformerEncoderLayer() requires torch>=1.9 to use nn.MultiheadAttention(batch_first=True)."
)
self.ma = nn.MultiheadAttention(c1, num_heads, dropout=dropout, batch_first=True)
# Implementation of Feedforward model
self.fc1 = nn.Linear(c1, cm)
self.fc2 = nn.Linear(cm, c1)
self.norm1 = nn.LayerNorm(c1)
self.norm2 = nn.LayerNorm(c1)
self.dropout = nn.Dropout(dropout)
self.dropout1 = nn.Dropout(dropout)
self.dropout2 = nn.Dropout(dropout)
self.act = act
self.normalize_before = normalize_before
@staticmethod
def with_pos_embed(tensor, pos=None):
"""Add position embeddings to the tensor if provided."""
return tensor if pos is None else tensor + pos
def forward_post(self, src, src_mask=None, src_key_padding_mask=None, pos=None):
"""Performs forward pass with post-normalization."""
q = k = self.with_pos_embed(src, pos)
src2 = self.ma(q, k, value=src, attn_mask=src_mask, key_padding_mask=src_key_padding_mask)[0]
src = src + self.dropout1(src2)
src = self.norm1(src)
src2 = self.fc2(self.dropout(self.act(self.fc1(src))))
src = src + self.dropout2(src2)
return self.norm2(src)
def forward_pre(self, src, src_mask=None, src_key_padding_mask=None, pos=None):
"""Performs forward pass with pre-normalization."""
src2 = self.norm1(src)
q = k = self.with_pos_embed(src2, pos)
src2 = self.ma(q, k, value=src2, attn_mask=src_mask, key_padding_mask=src_key_padding_mask)[0]
src = src + self.dropout1(src2)
src2 = self.norm2(src)
src2 = self.fc2(self.dropout(self.act(self.fc1(src2))))
return src + self.dropout2(src2)
def forward(self, src, src_mask=None, src_key_padding_mask=None, pos=None):
"""Forward propagates the input through the encoder module."""
if self.normalize_before:
return self.forward_pre(src, src_mask, src_key_padding_mask, pos)
return self.forward_post(src, src_mask, src_key_padding_mask, pos)
class AIFI(TransformerEncoderLayer):
"""Defines the AIFI transformer layer."""
def __init__(self, c1, cm=2048, num_heads=8, dropout=0, act=nn.GELU(), normalize_before=False):
"""Initialize the AIFI instance with specified parameters."""
super().__init__(c1, cm, num_heads, dropout, act, normalize_before)
def forward(self, x):
"""Forward pass for the AIFI transformer layer."""
c, h, w = x.shape[1:]
pos_embed = self.build_2d_sincos_position_embedding(w, h, c)
# Flatten [B, C, H, W] to [B, HxW, C]
x = super().forward(x.flatten(2).permute(0, 2, 1), pos=pos_embed.to(device=x.device, dtype=x.dtype))
return x.permute(0, 2, 1).view([-1, c, h, w]).contiguous()
@staticmethod
def build_2d_sincos_position_embedding(w, h, embed_dim=256, temperature=10000.0):
"""Builds 2D sine-cosine position embedding."""
assert embed_dim % 4 == 0, "Embed dimension must be divisible by 4 for 2D sin-cos position embedding"
grid_w = torch.arange(w, dtype=torch.float32)
grid_h = torch.arange(h, dtype=torch.float32)
grid_w, grid_h = torch.meshgrid(grid_w, grid_h, indexing="ij")
pos_dim = embed_dim // 4
omega = torch.arange(pos_dim, dtype=torch.float32) / pos_dim
omega = 1.0 / (temperature**omega)
out_w = grid_w.flatten()[..., None] @ omega[None]
out_h = grid_h.flatten()[..., None] @ omega[None]
return torch.cat([torch.sin(out_w), torch.cos(out_w), torch.sin(out_h), torch.cos(out_h)], 1)[None]
四、添加步骤
4.1 修改common.py
此处需要修改的文件是models/common.py
common.py中定义了网络结构的通用模块
,我们想要加入新的模块就只需要将模块代码放到这个文件内即可。
此时需要将上方实现的代码添加到common.py
中。
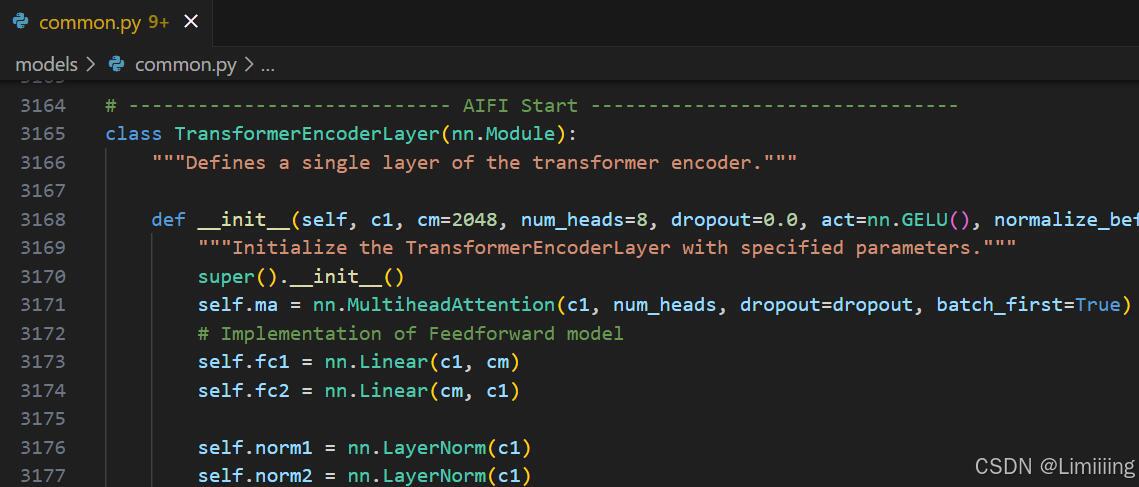
注意❗:在4.2小节
中的yolo.py
文件中需要声明的模块名称为:AIFI
。
4.2 修改yolo.py
此处需要修改的文件是models/yolo.py
yolo.py用于函数调用
,我们只需要将common.py
中定义的新的模块名添加到parse_model函数
下即可。
AIFI
模块添加后如下:
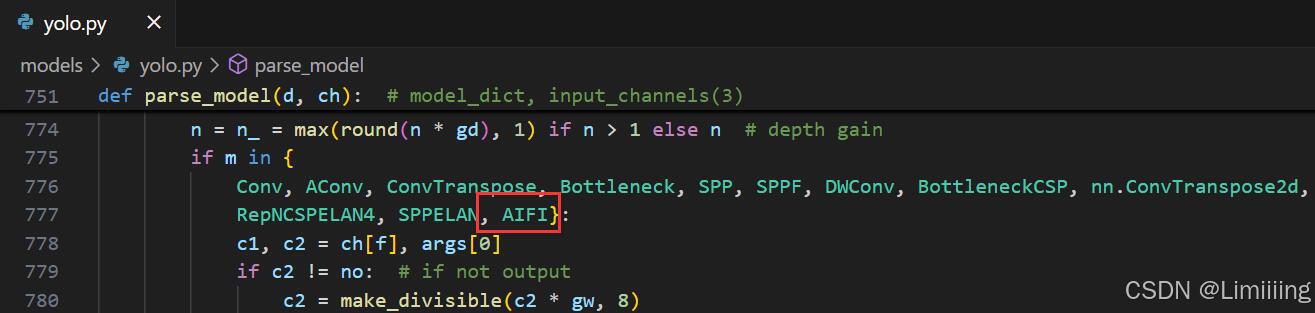
还需在此函数下添加如下代码:
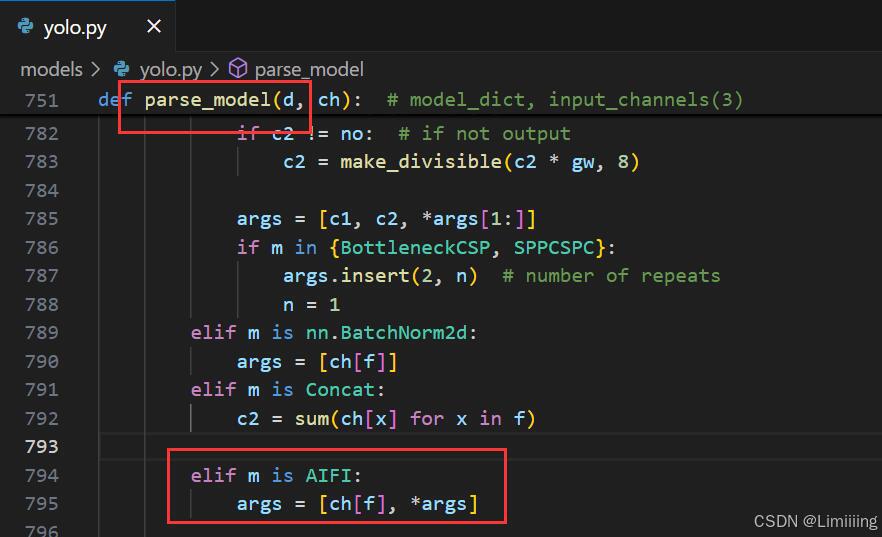
python
elif m is AIFI:
args = [ch[f], *args]
五、yaml模型文件
5.1 模型改进⭐
在代码配置完成后,配置模型的YAML文件。
此处以models/detect/yolov9-c.yaml
为例,在同目录下创建一个用于自己数据集训练的模型文件yolov9-c-AIFI.yaml
。
将yolov9-c.yaml
中的内容复制到yolov9-c-AIFI.yaml
文件下,修改nc
数量等于自己数据中目标的数量。
📌 模型的修改方法是将颈部网络 中的SPPELAN模块
替换成AIFI模块
。
结构如下:
python
# YOLOv9
# parameters
nc: 1 # number of classes
depth_multiple: 1.0 # model depth multiple
width_multiple: 1.0 # layer channel multiple
#activation: nn.LeakyReLU(0.1)
#activation: nn.ReLU()
# anchors
anchors: 3
# YOLOv9 backbone
backbone:
[
[-1, 1, Silence, []],
# conv down
[-1, 1, Conv, [64, 3, 2]], # 1-P1/2
# conv down
[-1, 1, Conv, [128, 3, 2]], # 2-P2/4
# elan-1 block
[-1, 1, RepNCSPELAN4, [256, 128, 64, 1]], # 3
# avg-conv down
[-1, 1, ADown, [256]], # 4-P3/8
# elan-2 block
[-1, 1, RepNCSPELAN4, [512, 256, 128, 1]], # 5
# avg-conv down
[-1, 1, ADown, [512]], # 6-P4/16
# elan-2 block
[-1, 1, RepNCSPELAN4, [512, 512, 256, 1]], # 7(可替换)
# avg-conv down
[-1, 1, ADown, [512]], # 8-P5/32
# elan-2 block
[-1, 1, RepNCSPELAN4, [512, 512, 256, 1]], # 9(可替换)
]
# YOLOv9 head
head:
[
# elan-spp block
[-1, 1, AIFI, [512, 8]], # 10
# up-concat merge
[-1, 1, nn.Upsample, [None, 2, 'nearest']],
[[-1, 7], 1, Concat, [1]], # cat backbone P4
# elan-2 block
[-1, 1, RepNCSPELAN4, [512, 512, 256, 1]], # 13
# up-concat merge
[-1, 1, nn.Upsample, [None, 2, 'nearest']],
[[-1, 5], 1, Concat, [1]], # cat backbone P3
# elan-2 block
[-1, 1, RepNCSPELAN4, [256, 256, 128, 1]], # 16 (P3/8-small)
# avg-conv-down merge
[-1, 1, ADown, [256]],
[[-1, 13], 1, Concat, [1]], # cat head P4
# elan-2 block
[-1, 1, RepNCSPELAN4, [512, 512, 256, 1]], # 19 (P4/16-medium)
# avg-conv-down merge
[-1, 1, ADown, [512]],
[[-1, 10], 1, Concat, [1]], # cat head P5
# elan-2 block
[-1, 1, RepNCSPELAN4, [512, 512, 256, 1]], # 22 (P5/32-large)
# multi-level reversible auxiliary branch
# routing
[5, 1, CBLinear, [[256]]], # 23
[7, 1, CBLinear, [[256, 512]]], # 24
[9, 1, CBLinear, [[256, 512, 512]]], # 25
# conv down
[0, 1, Conv, [64, 3, 2]], # 26-P1/2
# conv down
[-1, 1, Conv, [128, 3, 2]], # 27-P2/4
# elan-1 block
[-1, 1, RepNCSPELAN4, [256, 128, 64, 1]], # 28
# avg-conv down fuse
[-1, 1, ADown, [256]], # 29-P3/8
[[23, 24, 25, -1], 1, CBFuse, [[0, 0, 0]]], # 30
# elan-2 block
[-1, 1, RepNCSPELAN4, [512, 256, 128, 1]], # 31
# avg-conv down fuse
[-1, 1, ADown, [512]], # 32-P4/16
[[24, 25, -1], 1, CBFuse, [[1, 1]]], # 33
# elan-2 block
[-1, 1, RepNCSPELAN4, [512, 512, 256, 1]], # 34
# avg-conv down fuse
[-1, 1, ADown, [512]], # 35-P5/32
[[25, -1], 1, CBFuse, [[2]]], # 36
# elan-2 block
[-1, 1, RepNCSPELAN4, [512, 512, 256, 1]], # 37
# detection head
# detect
[[31, 34, 37, 16, 19, 22], 1, DualDDetect, [nc]], # DualDDetect(A3, A4, A5, P3, P4, P5)
]
六、成功运行结果
分别打印网络模型可以看到AIFI
已经加入到模型中,并可以进行训练了。
yolov9-c-AIFI:
from n params module arguments
0 -1 1 0 models.common.Silence []
1 -1 1 1856 models.common.Conv [3, 64, 3, 2]
2 -1 1 73984 models.common.Conv [64, 128, 3, 2]
3 -1 1 212864 models.common.RepNCSPELAN4 [128, 256, 128, 64, 1]
4 -1 1 164352 models.common.ADown [256, 256]
5 -1 1 847616 models.common.RepNCSPELAN4 [256, 512, 256, 128, 1]
6 -1 1 656384 models.common.ADown [512, 512]
7 -1 1 2857472 models.common.RepNCSPELAN4 [512, 512, 512, 256, 1]
8 -1 1 656384 models.common.ADown [512, 512]
9 -1 1 2857472 models.common.RepNCSPELAN4 [512, 512, 512, 256, 1]
10 -1 1 1577984 models.common.AIFI [512, 512, 8]
11 -1 1 0 torch.nn.modules.upsampling.Upsample [None, 2, 'nearest']
12 [-1, 7] 1 0 models.common.Concat [1]
13 -1 1 3119616 models.common.RepNCSPELAN4 [1024, 512, 512, 256, 1]
14 -1 1 0 torch.nn.modules.upsampling.Upsample [None, 2, 'nearest']
15 [-1, 5] 1 0 models.common.Concat [1]
16 -1 1 912640 models.common.RepNCSPELAN4 [1024, 256, 256, 128, 1]
17 -1 1 164352 models.common.ADown [256, 256]
18 [-1, 13] 1 0 models.common.Concat [1]
19 -1 1 2988544 models.common.RepNCSPELAN4 [768, 512, 512, 256, 1]
20 -1 1 656384 models.common.ADown [512, 512]
21 [-1, 10] 1 0 models.common.Concat [1]
22 -1 1 3119616 models.common.RepNCSPELAN4 [1024, 512, 512, 256, 1]
23 5 1 131328 models.common.CBLinear [512, [256]]
24 7 1 393984 models.common.CBLinear [512, [256, 512]]
25 9 1 656640 models.common.CBLinear [512, [256, 512, 512]]
26 0 1 1856 models.common.Conv [3, 64, 3, 2]
27 -1 1 73984 models.common.Conv [64, 128, 3, 2]
28 -1 1 212864 models.common.RepNCSPELAN4 [128, 256, 128, 64, 1]
29 -1 1 164352 models.common.ADown [256, 256]
30 [23, 24, 25, -1] 1 0 models.common.CBFuse [[0, 0, 0]]
31 -1 1 847616 models.common.RepNCSPELAN4 [256, 512, 256, 128, 1]
32 -1 1 656384 models.common.ADown [512, 512]
33 [24, 25, -1] 1 0 models.common.CBFuse [[1, 1]]
34 -1 1 2857472 models.common.RepNCSPELAN4 [512, 512, 512, 256, 1]
35 -1 1 656384 models.common.ADown [512, 512]
36 [25, -1] 1 0 models.common.CBFuse [[2]]
37 -1 1 2857472 models.common.RepNCSPELAN4 [512, 512, 512, 256, 1]
38[31, 34, 37, 16, 19, 22] 1 21542822 DualDDetect [1, [512, 512, 512, 256, 512, 512]]
yolov9-c-AIFI summary: 960 layers, 51920678 parameters, 51920646 gradients, 238.8 GFLOPs