什么是GQA?
多个head的Query共用一组K和V。llama模型就用到该技术。
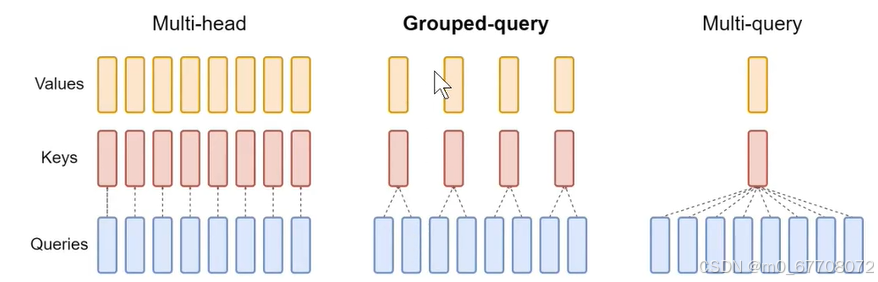
需要明确几点:
1.group有几组
2.每个group对应几个head
3.q以head为单位 k,v以group为单位 每个head/group特征维度都是head_dim
代码实现
python
import torch.nn as nn
import torch
import math
# 自注意力
class GroupQueryAttention(nn.Module):
def __init__(self, d_model, n_heads, n_groups):
super().__init__()
self.d_model = d_model
self.n_heads = n_heads
self.n_groups = n_groups
assert d_model % n_heads == 0
self.head_dim = d_model // n_heads
# 每个group对应几个head
self.heads_per_group = n_heads // n_groups
# 以head为单位
self.w_q = nn.Linear(d_model, n_heads*self.head_dim) # n_heads*head_dim=d_model
# 以group为单位
self.w_k = nn.Linear(d_model, n_groups*self.head_dim)
self.w_v = nn.Linear(d_model, n_groups*self.head_dim)
self.w_combine = nn.Linear(d_model, d_model)
self.softmax = nn.Softmax(dim=-1)
# 给k,v进行复制,假设每个组对应3个head,那就要把每个组的数据复制3遍
def expand(self, data): # data:k/v [b, group, seq_len, head_dim]
b,_,seq_len,_ = data.shape
data = data[:,:,None,:,:].expand(b, self.n_groups, self.heads_per_group, seq_len, self.head_dim)
data = data.contiguous().view(b, -1, seq_len, self.head_dim)
return data # [b, group*heads_per_group, seq_len, head_dim]
def forward(self, x, use_mask=False): # x: [b, seq_len, d_model]
b, seq_len, _ = x.shape
q,k,v = self.w_q(x), self.w_k(x), self.w_v(x)
q = q.view(b, seq_len, self.n_heads, self.head_dim).permute(0,2,1,3) # 以head为单位
k = k.view(b, seq_len, self.n_groups, self.head_dim).permute(0,2,1,3) # 以group为单位
v = v.view(b, seq_len, self.n_groups, self.head_dim).permute(0,2,1,3)
# 复制
k,v = self.expand(k), self.expand(v)
score = q @ k.transpose(-1,-2) / math.sqrt(self.head_dim)
if use_mask:
mask = torch.tril(torch.ones(seq_len, seq_len))
score = score.masked_fill(mask==0, float('-inf'))
score = self.softmax(score) @ v
score = score.permute(0,2,1,3).contiguous().view(b, seq_len, self.n_heads*self.head_dim)
out = self.w_combine(score)
return out
x = torch.rand(2,100,384)
model = GroupQueryAttention(384, 4, 2)
out = model(x)
print(out.shape)