目录
606、pandas.DataFrame.sparse.from_spmatrix方法
607、pandas.DataFrame.sparse.to_coo方法
608、pandas.DataFrame.sparse.to_dense方法
609、pandas.DataFrame.from_dict方法
610、pandas.DataFrame.from_records方法

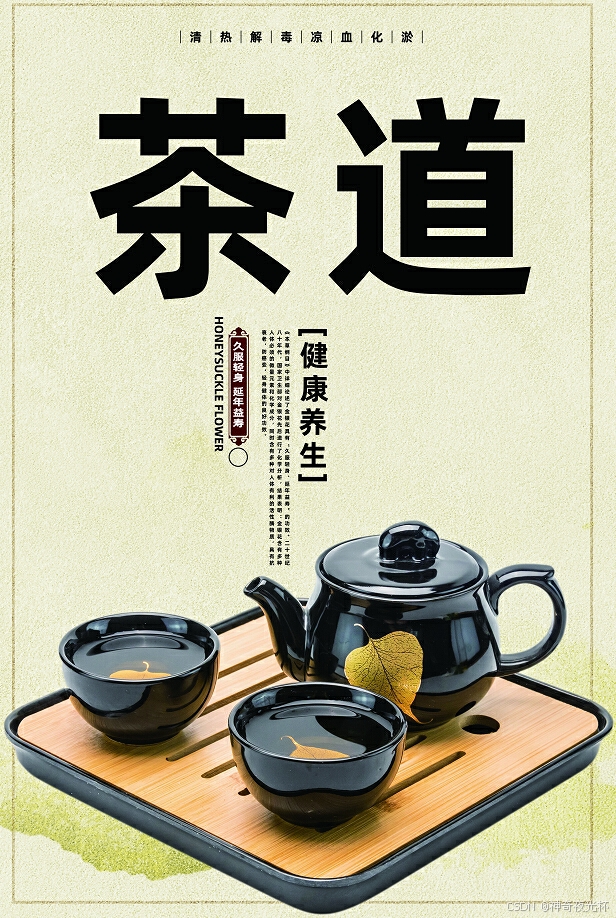

一、用法精讲
606、pandas.DataFrame.sparse.from_spmatrix方法
606-1、语法
python
# 606、pandas.DataFrame.sparse.from_spmatrix方法
classmethod pandas.DataFrame.sparse.from_spmatrix(data, index=None, columns=None)
Create a new DataFrame from a scipy sparse matrix.
Parameters:
data
scipy.sparse.spmatrix
Must be convertible to csc format.
index, columns
Index, optional
Row and column labels to use for the resulting DataFrame. Defaults to a RangeIndex.
Returns:
DataFrame
Each column of the DataFrame is stored as a arrays.SparseArray.
606-2、参数
606-2-1、data**(必须)****:**scipy.sparse矩阵,表示输入的稀疏矩阵,可以是多种稀疏格式(如CSR、CSC等),该参数是必需的,表示待转换的稀疏数据。
606-2-2、index**(可选,默认值为None)****:**array-like,用于指定DataFrame的行索引,必须和稀疏矩阵的行数匹配,如果没有指定,默认会生成整数索引。
606-2-3、columns**(可选,默认值为None)****:**array-like,用于指定DataFrame的列索引,必须和稀疏矩阵的列数匹配,如果没有指定,默认会生成整数索引。
606-3、功能
快速将一个scipy的稀疏矩阵转换为Pandas的稀疏DataFrame,这种转换使得后续的数据分析和处理更加方便,同时有效利用内存,特别是当数据集中含有大量零值时。
606-4、返回值
返回一个新的稀疏DataFrame,其中仅保留稀疏矩阵中的非零元素,并使用Pandas的稀疏数据结构进行存储,这个DataFrame可以利用Pandas提供的各种数据操作、筛选和分析功能。
606-5、说明
无
606-6、用法
606-6-1、数据准备
python
无
606-6-2、代码示例
python
# 606、pandas.DataFrame.sparse.from_spmatrix方法
import pandas as pd
import numpy as np
from scipy.sparse import csr_matrix
# 创建一个稀疏矩阵
data = np.array([[0, 0, 3], [4, 0, 0], [0, 5, 6]])
sparse_matrix = csr_matrix(data)
# 从稀疏矩阵创建稀疏DataFrame
sparse_df = pd.DataFrame.sparse.from_spmatrix(sparse_matrix,
index=['row1', 'row2', 'row3'],
columns=['col1', 'col2', 'col3'])
print(sparse_df)
606-6-3、结果输出
python
# 606、pandas.DataFrame.sparse.from_spmatrix方法
# col1 col2 col3
# row1 0 0 3
# row2 4 0 0
# row3 0 5 6
607、pandas.DataFrame.sparse.to_coo方法
607-1、语法
python
# 607、pandas.DataFrame.sparse.to_coo方法
pandas.DataFrame.sparse.to_coo()
Return the contents of the frame as a sparse SciPy COO matrix.
Returns:
scipy.sparse.spmatrix
If the caller is heterogeneous and contains booleans or objects, the result will be of dtype=object. See Notes.
Notes
The dtype will be the lowest-common-denominator type (implicit upcasting); that is to say if the dtypes (even of numeric types) are mixed, the one that accommodates all will be chosen.
e.g. If the dtypes are float16 and float32, dtype will be upcast to float32. By numpy.find_common_type convention, mixing int64 and and uint64 will result in a float64 dtype.
607-2、参数
无
607-3、功能
将一个Pandas稀疏DataFrame转换为scipy.sparse提供的COO格式的稀疏矩阵,使用COO格式能够高效地存储和操作稀疏数据,并便于后续的计算和分析。
607-4、返回值
返回一个COO格式的稀疏矩阵,包含稀疏DataFrame中非零元素的坐标和数值,这种结构在进行稀疏矩阵的加法、乘法等操作时非常方便。
607-5、说明
无
607-6、用法
607-6-1、数据准备
python
无
607-6-2、代码示例
python
# 607、pandas.DataFrame.sparse.to_coo方法
import pandas as pd
import numpy as np
from scipy import sparse
# 创建一个普通DataFrame
data = {'A': [0, 0, 3], 'B': [4, 0, 0], 'C': [0, 5, 6]}
df = pd.DataFrame(data)
# 将DataFrame转换为稀疏DataFrame
sparse_df = df.astype(pd.SparseDtype("float", 0))
# 将稀疏DataFrame转换为COO格式稀疏矩阵
coo_matrix = sparse_df.sparse.to_coo()
print(coo_matrix)
607-6-3、结果输出
python
# 607、pandas.DataFrame.sparse.to_coo方法
# (2, 0) 3.0
# (0, 1) 4.0
# (1, 2) 5.0
# (2, 2) 6.0
608、pandas.DataFrame.sparse.to_dense方法
608-1、语法
python
# 608、pandas.DataFrame.sparse.to_dense方法
pandas.DataFrame.sparse.to_dense()
Convert a DataFrame with sparse values to dense.
Returns:
DataFrame
A DataFrame with the same values stored as dense arrays.
608-2、参数
无
608-3、功能
将一个稀疏DataFrame(即其中的空值或零值以更节省空间的方式存储)转换为一个密集DataFrame,这将使得数据不再使用稀疏存储技术,但更易于作一般性的数据处理、计算以及与使用普通DataFrame方法的兼容性。
608-4、返回值
返回一个标准的pandas.DataFrame对象,其中所有原本稀疏存储的数据都被完整地表示出来,转换后,这个DataFrame与任何在非稀疏情况下创建的DataFrame完全一致,没有稀疏结构。
608-5、说明
无
608-6、用法
608-6-1、数据准备
python
无
608-6-2、代码示例
python
# 608、pandas.DataFrame.sparse.to_dense方法
import pandas as pd
import numpy as np
# 创建一个稀疏数据矩阵
data = {
'A': pd.arrays.SparseArray([0, 1, 0, 0, 2]),
'B': pd.arrays.SparseArray([0, 0, 0, 5, 0]),
}
# 将稀疏数据转换为DataFrame
sparse_df = pd.DataFrame(data)
print("稀疏DataFrame:")
print(sparse_df)
# 使用to_dense()方法将稀疏DataFrame转换为密集DataFrame
dense_df = sparse_df.sparse.to_dense()
print("\n密集DataFrame:")
print(dense_df)
608-6-3、结果输出
python
# 608、pandas.DataFrame.sparse.to_dense方法
# 稀疏DataFrame:
# A B
# 0 0 0
# 1 1 0
# 2 0 0
# 3 0 5
# 4 2 0
#
# 密集DataFrame:
# A B
# 0 0 0
# 1 1 0
# 2 0 0
# 3 0 5
# 4 2 0
609、pandas.DataFrame.from_dict方法
609-1、语法
python
# 609、pandas.DataFrame.from_dict方法
classmethod pandas.DataFrame.from_dict(data, orient='columns', dtype=None, columns=None)
Construct DataFrame from dict of array-like or dicts.
Creates DataFrame object from dictionary by columns or by index allowing dtype specification.
Parameters:
datadict
Of the form {field : array-like} or {field : dict}.
orient{'columns', 'index', 'tight'}, default 'columns'
The "orientation" of the data. If the keys of the passed dict should be the columns of the resulting DataFrame, pass 'columns' (default). Otherwise if the keys should be rows, pass 'index'. If 'tight', assume a dict with keys ['index', 'columns', 'data', 'index_names', 'column_names'].
New in version 1.4.0: 'tight' as an allowed value for the orient argument
dtypedtype, default None
Data type to force after DataFrame construction, otherwise infer.
columnslist, default None
Column labels to use when orient='index'. Raises a ValueError if used with orient='columns' or orient='tight'.
Returns:
DataFrame.
609-2、参数
609-2-1、data**(必须)****:**字典,表示包含数据的字典,其中键表示列标签(当orient='columns'时)或行索引(当orient='index'时),而值是可迭代对象(如列表、数组等),表示数据。
609-2-2、orient**(可选,默认值为'columns')****:**字符串,指定字典的布局方式,可选值包括:
- **'columns':**数据的键作为列标签,值作为列数据(默认设置)。
- **'index':**数据的键作为行索引,值作为行数据。
609-2-3、dtype**(可选,默认值为None)****:**dtype或None,指定DataFrame所有列的数据类型,如果未提供,将根据数据类型自动推断。
609-2-4、columns**(可选,默认值为None)****:**list-like,指定DataFrame的列标签(仅在orient='index'模式中使用时有效),如果提供,它应该是data中所有键的一个子集或全部。
609-3、功能
将复杂结构(如嵌套字典)转换为DataFrame,方便后续的数据分析和操作,支持从多种形式的数据来源快速构建DataFrame,特别适合处理字典格式的数据输入,通过参数控制最终生成的DataFrame的结构和数据类型。
609-4、返回值
返回一个DataFrame对象,根据data和其他参数决定最终的结构。
609-5、说明
无
609-6、用法
609-6-1、数据准备
python
无
609-6-2、代码示例
python
# 609、pandas.DataFrame.from_dict方法
# 609-1、使用默认参数
import pandas as pd
data = {'A': [1, 2, 3], 'B': [4, 5, 6]}
df = pd.DataFrame.from_dict(data)
print(df, end='\n\n')
# 609-2、设置orient='index'
import pandas as pd
data = {0: [1, 4], 1: [2, 5], 2: [3, 6]}
df = pd.DataFrame.from_dict(data, orient='index', columns=['A', 'B'])
print(df, end='\n\n')
# 609-3、设置dtype
import pandas as pd
data = {'A': [1, 2, 3], 'B': [4, 5, 6]}
df = pd.DataFrame.from_dict(data, dtype='float')
print(df)
609-6-3、结果输出
python
# 609、pandas.DataFrame.from_dict方法
# 609-1、使用默认参数
# A B
# 0 1 4
# 1 2 5
# 2 3 6
# 609-2、设置orient='index'
# A B
# 0 1 4
# 1 2 5
# 2 3 6
# 609-3、设置dtype
# A B
# 0 1.0 4.0
# 1 2.0 5.0
# 2 3.0 6.0
610、pandas.DataFrame.from_records方法
610-1、语法
python
# 610、pandas.DataFrame.from_records方法
classmethod pandas.DataFrame.from_records(data, index=None, exclude=None, columns=None, coerce_float=False, nrows=None)
Convert structured or record ndarray to DataFrame.
Creates a DataFrame object from a structured ndarray, sequence of tuples or dicts, or DataFrame.
Parameters:
datastructured ndarray, sequence of tuples or dicts, or DataFrame
Structured input data.
Deprecated since version 2.1.0: Passing a DataFrame is deprecated.
indexstr, list of fields, array-like
Field of array to use as the index, alternately a specific set of input labels to use.
excludesequence, default None
Columns or fields to exclude.
columnssequence, default None
Column names to use. If the passed data do not have names associated with them, this argument provides names for the columns. Otherwise this argument indicates the order of the columns in the result (any names not found in the data will become all-NA columns).
coerce_floatbool, default False
Attempt to convert values of non-string, non-numeric objects (like decimal.Decimal) to floating point, useful for SQL result sets.
nrowsint, default None
Number of rows to read if data is an iterator.
Returns:
DataFrame.
610-2、参数
610-2-1、data**(必须)****:**array-like(如列表、元组等),表示输入数据,可以是嵌套的序列(如列表、元组),也可以是字典或其他结构化形式的记录。
610-2-2、index**(可选,默认值为None)****:**array-like或None,用于指定DataFrame的行索引,如果没有提供,默认将使用0到n-1的范围。
610-2-3、exclude**(可选,默认值为None)****:**array-like或None,指定要排除的列名(仅适用于数据为字典形式时)。
610-2-4、columns**(可选,默认值为None)****:**array-like,指定最终DataFrame的列名。
610-2-5、coerce_float**(可选,默认值为False)****:**布尔值,是否将非整数字符串转换为浮点数。
610-2-6、nrows**(可选,默认值为None)****:**整数,指定要读取的行数,如果为None,则读取所有行。
610-3、功能
将一组结构化的记录快速转换为DataFrame,适合处理外部数据源(如数据库查询结果)或程序生成的数据,支持灵活的数据筛选,使用户能够排除某些列、设置行索引、指定列名等,方便的类型转换选项(如coerce_float)可以确保数据类型一致性。
610-4、返回值
返回生成的DataFrame对象,结构根据data和其他参数决定。
610-5、说明
无
610-6、用法
610-6-1、数据准备
python
无
610-6-2、代码示例
python
# 610、pandas.DataFrame.from_records方法
# 610-1、从列表构建DataFrame
import pandas as pd
data = [(1, 4), (2, 5), (3, 6)]
df = pd.DataFrame.from_records(data, columns=['A', 'B'])
print(df, end='\n\n')
# 610-2、自定义行索引
import pandas as pd
data = [(1, 'Myelsa'), (2, 'Bryce'), (3, 'Jimmy')]
df = pd.DataFrame.from_records(data, index=[101, 102, 103], columns=['ID', 'Name'])
print(df, end='\n\n')
# 610-3、排除某些列
import pandas as pd
data = [{'A': 1, 'B': 2, 'C': 3}, {'A': 4, 'B': 5, 'C': 6}]
df = pd.DataFrame.from_records(data, exclude=['B'])
print(df, end='\n\n')
# 610-4、强制转换为浮点数
import pandas as pd
data = [{'A': '1.0', 'B': '4.0'}, {'A': '2.5', 'B': '5.5'}]
df = pd.DataFrame.from_records(data, coerce_float=True)
print(df)
610-6-3、结果输出
python
# 610、pandas.DataFrame.from_records方法
# 610-1、从列表构建DataFrame
# A B
# 0 1 4
# 1 2 5
# 2 3 6
# 610-2、自定义行索引
# ID Name
# 101 1 Myelsa
# 102 2 Bryce
# 103 3 Jimmy
# 610-3、排除某些列
# A C
# 0 1 3
# 1 4 6
# 610-4、强制转换为浮点数
# A B
# 0 1.0 4.0
# 1 2.5 5.5