Title
题目
Automated anomaly-aware 3D segmentation of bones and cartilages in kneeMR images from the Osteoarthritis Initiative
来自骨关节炎计划的膝关节MR图像的自动异常感知3D骨骼和软骨分割
Background
背景
近年来,多个机器学习算法被提出用于图像中的自动异常检测。无监督方法,尤其是生成模型在医学成像领域展现了很大的潜力,因为获取标注数据非常困难。近期的研究表明,像Transformer这样的先进技术也能应用于脑部图像的异常检测和分割,但其在三维(3D)图像中的应用仍面临数据和计算资源的挑战。相比之下,基于卷积自编码器(如U-Net)的模型在计算资源方面要求较低。U-Net是一种流行的卷积神经网络(CNN)模型,专为生物医学图像的语义分割设计,具有独特的跳跃连接结构,用于恢复图像下采样时丢失的空间信息。
自动医学图像分析的主要挑战包括缺乏标注数据和解剖结构中异常的存在,尤其在大型数据集上进行手动标注非常费时。U-Net等模型常用于三维图像的体积分割,并通过"深度监督"来提高分割的准确性。除了分割任务外,U-Net结构的CNN还被用于图像修复和异常检测,最近的研究采用了U-Net进行图像修复,以识别并定位视觉异常。
上下文聚合网络(CAN)是另一种语义分割模型,不同于U-Net的编码器--解码器结构,它采用膨胀卷积而非下采样来进行多尺度上下文聚合。近年来,CNN被广泛用于膝关节MR图像的骨骼和软骨分割,但当图像中存在异常时,分割任务的准确性下降。因此,基于我们对U-Net和CAN的初步实验,提出了一种异常感知的分割机制,以更好地处理图像中的异常。
Aastract
摘要
In medical image analysis, automated segmentation of multi-component anatomical entities, with the possiblepresence of variable anomalies or pathologies, is a challenging task. In this work, we develop a multi-stepapproach using U-Net-based models to initially detect anomalies (bone marrow lesions, bone cysts) in the distalfemur, proximal tibia and patella from 3D magnetic resonance (MR) images in individuals with varying gradesof knee osteoarthritis. Subsequently, the extracted data are used for downstream tasks involving semanticsegmentation of individual bone and cartilage volumes as well as bone anomalies. For anomaly detection,U-Net-based models were developed to reconstruct bone volume profiles of the femur and tibia in images viainpainting so anomalous bone regions could be replaced with close to normal appearances. The reconstructionerror was used to detect bone anomalies. An anomaly-aware segmentation network, which was compared toanomaly-naïve segmentation networks, was used to provide a final automated segmentation of the individualfemoral, tibial and patellar bone and cartilage volumes from the knee MR images which contain a spectrumof bone anomalies. The anomaly-aware segmentation approach provided up to 58% reduction in Hausdorffdistances for bone segmentations compared to the results from anomaly-naïve segmentation networks. Inaddition, the anomaly-aware networks were able to detect bone anomalies in the MR images with greatersensitivity and specificity (area under the receiver operating characteristic curve [AUC] up to 0.896) comparedto anomaly-naïve segmentation networks (AUC up to 0.874).
在医学图像分析中,对包含多个解剖结构成分且可能存在各种异常或病理的实体进行自动分割是一项极具挑战性的任务。在本研究中,我们开发了一种多步骤的方法,使用基于U-Net的模型,首先检测在不同膝关节骨关节炎等级患者的三维磁共振(MR)图像中,远端股骨、近端胫骨和髌骨的异常(如骨髓病变、骨囊肿)。随后,提取的数据用于下游任务,涉及单个骨骼和软骨体积的语义分割以及骨骼异常的分割。在异常检测中,我们开发了基于U-Net的模型,通过图像修复法重建股骨和胫骨的骨骼体积轮廓,以便将异常的骨骼区域替换为接近正常外观。重建误差被用于检测骨骼异常。随后使用一种异常感知分割网络,与不感知异常的分割网络进行对比,最终从包含一系列骨骼异常的膝关节MR图像中,自动分割出股骨、胫骨和髌骨的单个骨骼和软骨体积。与不感知异常的分割网络相比,异常感知分割方法在骨骼分割的Hausdorff距离上最多减少了58%。此外,异常感知网络在MR图像中检测骨骼异常时的灵敏度和特异性更高(受试者工作特征曲线下面积[AUC]最高可达0.896),相比之下,不感知异常的分割网络的AUC最高为0.874。
Method
方法
The overall pipeline for the current work has 3 major components(Fig. 1). In Component 1, the anatomical regions of interest---here, thedistal femur and proximal tibia profiles---were erased from the imagesusing the reference segmentation masks, and then these regions wereinpainted using a 3D U-Net-based model (𝐺). In Component 2, the outputs from Component 1 and another 3D U-Net-based model (𝐴) wereused to change anomalous bone regions in the original images to closeto normal appearances. The anomaly detection task was unsupervisedbecause the anomalies in the images were not labeled. The anomalieswere detected indirectly through the inpainting/reconstruction process.In Component 3, a 3D CNN-based segmentation network (𝑆), whichutilizes the information extracted from Component 2, was used to guidethe automated segmentation of bone and cartilage volumes (''anomalyaware'' segmentation), specifically aiming to improve segmentation ofthe femoral and tibial bone and cartilage volumes from images containing visible bone anomalies when compared to the vanilla (anomalyna´'ive) segmentation networks.
当前工作的整体流程包含三个主要部分(图1)。在组件1中,感兴趣的解剖区域------这里指远端股骨和近端胫骨轮廓------通过参考分割掩膜从图像中擦除,然后使用基于3D U-Net的模型(𝐺)对这些区域进行修复(inpainting)。在组件2中,使用来自组件1的输出和另一个基于3D U-Net的模型(𝐴)对原始图像中的异常骨骼区域进行处理,使其接近正常外观。异常检测任务是无监督的,因为图像中的异常没有被标注,异常通过修复/重建过程间接检测。在组件3中,使用从组件2中提取的信息,利用基于3D CNN的分割网络(𝑆)进行自动骨骼和软骨体积的分割("异常感知"分割)。与普通的(不感知异常的)分割网络相比,该方法旨在改善包含明显骨骼异常的图像中的股骨和胫骨骨骼及软骨体积的分割。
Conclusion
结论
In summary, this work demonstrated how simple U-Net-like neuralnetworks can be used for detecting bone lesions in knee MR imagesthrough reconstruction via inpainting. Moreover, it showed how thedetected anomalies can be further utilized for downstream tasks such assegmentation. The anomaly-aware networks gave a better performanceon average than their baseline networks in the segmentation tasks aswell as in the detection of bone lesions. The stable convergence behavior and performance with the new labels in the OAI ZIB--UQ and OAIAKOA datasets are promising and suggest that the proposed methodhas an advantage when there are relatively few training images and/orthe classes are highly imbalanced. It is hoped that future works willshow additional improvements and further applications of the anomalydetection and anomaly-aware segmentation models in medical imaging.
总而言之,本研究展示了如何通过修复(inpainting)重建,使用简单的类似U-Net的神经网络检测膝关节MR图像中的骨损伤。此外,研究还展示了如何将检测到的异常进一步应用于后续的分割任务中。在分割任务和骨损伤检测中,异常感知网络的平均性能优于其基线网络。研究中使用的新标签在 OAI ZIB--UQ 和 OAI AKOA 数据集上的稳定收敛行为和表现令人鼓舞,这表明该方法在训练图像相对较少和/或类别高度不平衡时具有优势。希望未来的工作能够展示更多改进,并进一步应用异常检测和异常感知分割模型于医学影像领域。
Results
结果
5.1. Anomaly detection
Fig. 5 shows example output images from the anomaly detectionnetworks 𝐺 and 𝐴. The input MR images from the knee show somevisible bone anomalies including BMLs and osteophytes. The networkoutputs are lossy reconstructions of the input images with the brightsignal bone anomalies within the cancellous bone mostly removed fromthe images. Some of the osteophytes were incompletely reconstructed.The network 𝐴 only had the original images as inputs, but the outputsfrom 𝐴 still have most of the anomalies appropriately blurred out. Thelast column of Fig. 5 shows the reconstruction error images from 𝐴 inwhich the anomalous regions detected within the cancellous bones arehighlighted.
5.1. 异常检测
图5显示了来自异常检测网络 𝐺 和 𝐴 的示例输出图像。膝关节的输入MR图像显示了一些明显的骨骼异常,包括骨髓病变(BMLs)和骨赘。网络的输出是输入图像的有损重建,图像中的亮信号骨骼异常大多从松质骨中移除。一些骨赘的重建不完全。网络 𝐴 仅使用原始图像作为输入,但 𝐴 的输出仍然将大多数异常适当地模糊化。图5的最后一列显示了来自 𝐴 的重建误差图像,其中检测到的松质骨内的异常区域被高亮显示。
Figure
图
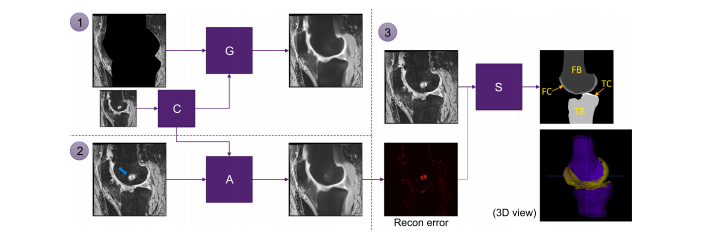
Fig. 1. Overall pipeline for anomaly detection and the downstream segmentation task. Components 1 and 2 are the anomaly detection models. 𝐺 performs image inpainting and𝐶 performs image compression (Section 3.1), and 𝐴 is a separate model that aims to reconstruct the original images without the visible anomalies (Section 3.2). The blue arrowpoints to a bone lesion (anomaly). Component 3 (model 𝑆) is the downstream segmentation task using the anomaly-aware segmentation approach (Section 3.3). FB: femoral bone;FC: femoral cartilage; TB: tibial bone; TC: tibial cartilage.
图1. 异常检测和后续分割任务的总体流程。组件1和2是异常检测模型。𝐺执行图像修复,𝐶执行图像压缩(第3.1节),𝐴是一个单独的模型,旨在重建不含明显异常的原始图像(第3.2节)。蓝色箭头指向骨损伤(异常)。组件3(模型𝑆)是使用异常感知分割方法进行的后续分割任务(第3.3节)。FB:股骨;FC:股骨软骨;TB:胫骨;TC:胫骨软骨。
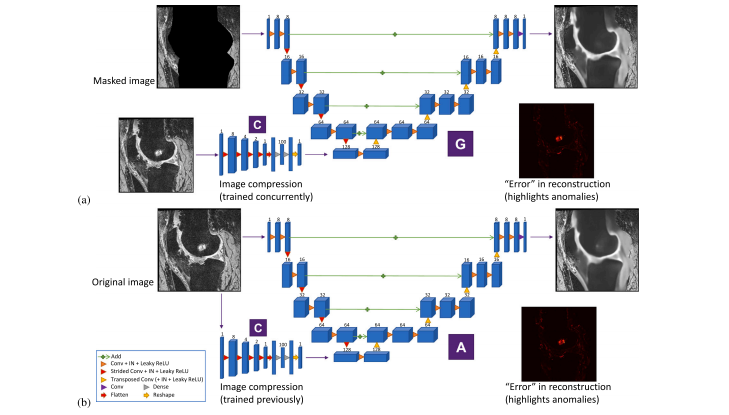
Fig. 2. The two anomaly detection networks with a 3D U-Net-based architecture. Blue boxes represent feature maps, with the number of channels denoted above each box. (a)Network 𝐺 regenerates the original images from masked images through inpainting and decoding of compressed images. The compressed images are provided by a small network𝐶 trained concurrently with 𝐺. (b) Network 𝐴 i
图2. 两个基于3D U-Net架构的异常检测网络。蓝色框表示特征图,框上方的数字表示通道数。(a) 网络𝐺通过修复(inpainting)和解码压缩图像,从被遮盖的图像中重生成原始图像。压缩图像由与𝐺同时训练的小型网络𝐶提供。(b) 网络𝐴
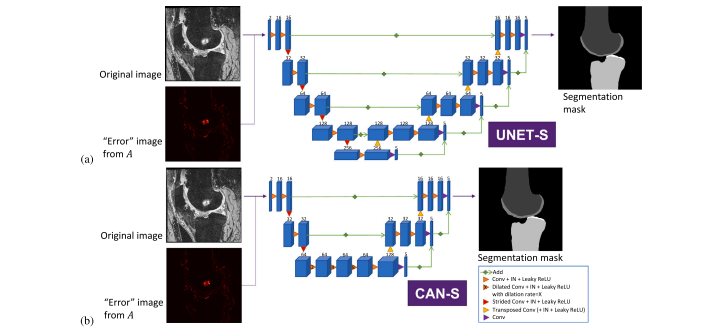
Fig. 3. The anomaly-aware segmentation network 𝑆 based on (a) 3D U-Net and (b) 3D CAN with deep supervision. The information extracted from the anomaly detector 𝐴 wasutilized to inform the segmentation of the distal femur and proximal tibia from the knee MR images containing bone abnormalities
图3. 基于 (a) 3D U-Net 和 (b) 带深度监督的 3D CAN 的异常感知分割网络 𝑆。从异常检测器 𝐴 提取的信息被用于指导从包含骨骼异常的膝关节MR图像中分割远端股骨和近端胫骨。
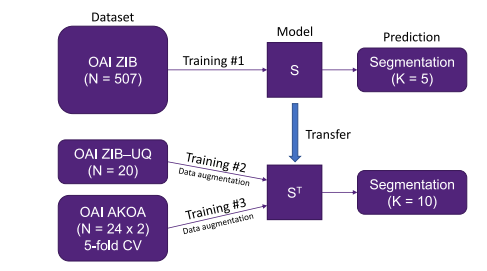
Fig. 4. Transfer learning for further segmentation of knee MR images. Here, 𝑁 refers tothe number of MR examinations while 𝐾 refers to the number of segmentation classes(including the background) in the dataset. See Table 1 for the list of segmentationclasses.
图4. 用于膝关节MR图像进一步分割的迁移学习。这里,𝑁 代表MR检查的次数,𝐾 代表数据集中分割类别的数量(包括背景)。有关分割类别的列表,请参见表1。
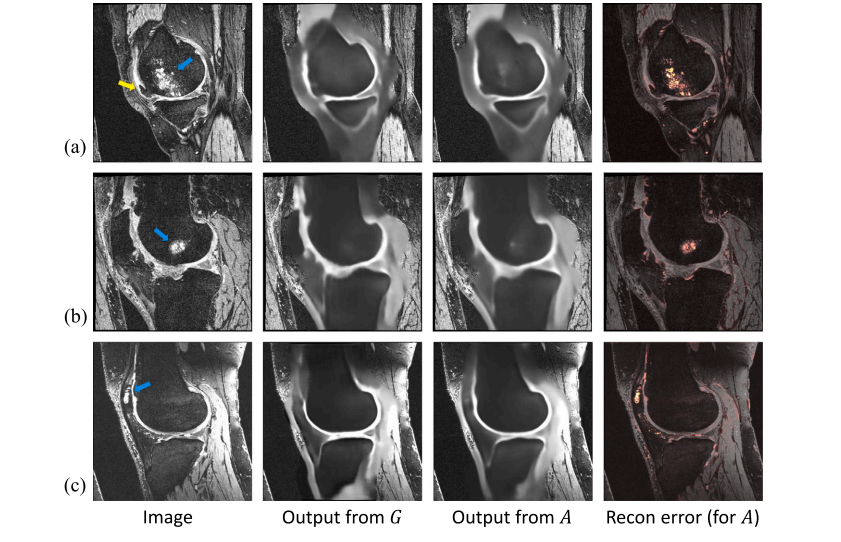
Fig. 5. Example outputs from the bone anomaly detection networks 𝐺 and 𝐴. Figures (a) and (b) are images from the OAI ZIB dataset, and Figure (c) is an image from the OAIAKOA dataset. The last column shows the error images (color-mapped and overlaid on the input images) highlighting the difference between the input image and the output from𝐴. Regions of BMLs (blue arrows) in the femur and patella and part of an osteophyte (yellow arrow) on the femur had high reconstruction errors.
图5. 来自骨骼异常检测网络 𝐺 和 𝐴 的示例输出。(a) 和 (b) 图像来自 OAI ZIB 数据集,(c) 图像来自 OAI AKOA 数据集。最后一列显示了误差图像(颜色映射并叠加在输入图像上),突出显示了输入图像与 𝐴 输出之间的差异。股骨和髌骨中的骨髓病变区域(蓝色箭头)以及股骨上的部分骨赘(黄色箭头)显示出较高的重建误差。
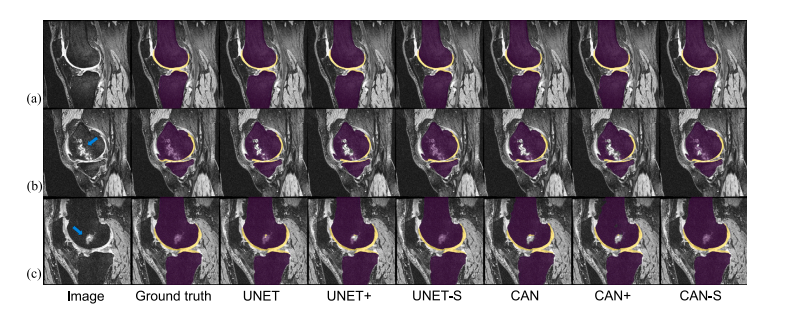
Fig. 6. Example segmentation outputs for the femoral and tibial bones (purple) and cartilages (yellow) generated by the individual network implementation with the OAI ZIB dataset.The examples show (a) images with little to no visible bone anomalies where all networks produced good segmentation masks and (b,c) images with visible bone anomalies (bluearrows) where segmentation networks tended to fail to produce plausible segmentation masks. The anomaly-aware networks, especially 𝑈𝑁𝐸𝑇 -𝑆, were better able to correctlysegment the images with anomalies
图6. 使用 OAI ZIB 数据集生成的股骨和胫骨(紫色)以及软骨(黄色)的示例分割输出。示例显示了 (a) 几乎没有可见骨骼异常的图像,所有网络都生成了良好的分割掩膜,以及 (b, c) 具有可见骨骼异常的图像(蓝色箭头),其中分割网络往往无法生成合理的分割掩膜。异常感知网络,特别是 𝑈𝑁𝐸𝑇-𝑆,在正确分割带有异常的图像时表现更好。
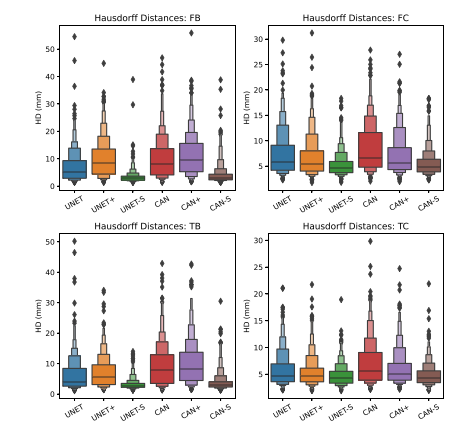
Fig. 7. Boxplots of Hausdorff distance (HD) values for the proposed anomaly-awaresegmentation approach (𝑈𝑁𝐸𝑇 -𝑆 and 𝐶𝐴𝑁-𝑆) and baseline networks, evaluated onthe OAI ZIB dataset using 5-fold cross-validation. Note that these HDs are results afterpost-processing
图7. 提出了基于异常感知分割方法(𝑈𝑁𝐸𝑇-𝑆 和 𝐶𝐴𝑁-𝑆)与基线网络的Hausdorff距离(HD)值的箱线图,使用 OAI ZIB 数据集通过5折交叉验证进行评估。请注意,这些HD值是在后处理后的结果。
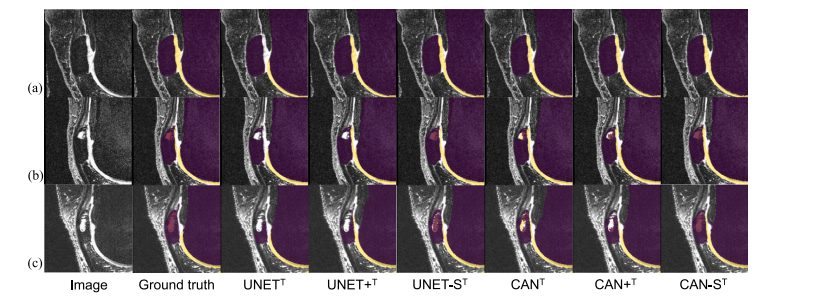
Fig. 8. Example outputs from the segmentation networks for images from the OAI AKOA dataset, segmenting the patella and visible bone lesions in addition to the femur and tibia.Note that the images are zoomed in to view the patella more closely. The masks are overlaid on the input images (purple: bones; yellow: cartilages; red: bone lesions). (a) Whenthe images had no visible anomalies, all networks except 𝑈𝑁𝐸𝑇 𝑇 produced good segmentation of the patella. The network 𝑈𝑁𝐸𝑇 𝑇 failed to converge for the patellar cartilagelabel. (b,c) The anomaly-aware networks were able to detect and segment most of the visible lesions along with the anatomical structures on images that the other segmentationnetworks had difficulty with.
图8. 分割网络从 OAI AKOA 数据集中生成的示例输出,分割髌骨和可见骨损伤,此外还包括股骨和胫骨。请注意,这些图像经过放大以更清晰地查看髌骨。掩膜叠加在输入图像上(紫色:骨骼;黄色:软骨;红色:骨损伤)。(a) 当图像没有明显的异常时,除了 𝑈𝑁𝐸𝑇 𝑇 外,所有网络都能很好地分割髌骨。𝑈𝑁𝐸𝑇 𝑇 网络未能对髌骨软骨标签进行收敛。(b, c) 异常感知网络能够检测并分割大多数可见损伤以及图像中的解剖结构,而其他分割网络在这些图像上表现较差。
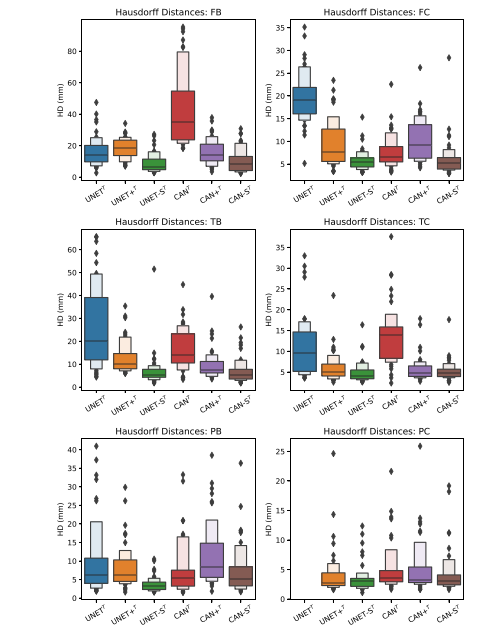
Fig. 9. Boxplots of Hausdorff distance (HD) values for the proposed anomaly-awaresegmentation approach (𝑈𝑁𝐸𝑇 -𝑆 𝑇 and 𝐶𝐴𝑁-𝑆 𝑇 ) and baseline networks with transferlearning, evaluated on the OAI AKOA dataset using 5-fold cross-validation. These HDsare results after post-processing. Note also that 𝑈𝑁𝐸𝑇 𝑇 failed to converge for the PClabel, so it was excluded from the plot.
图9. 基于异常感知分割方法(𝑈𝑁𝐸𝑇-𝑆 𝑇 和 𝐶𝐴𝑁-𝑆 𝑇)与迁移学习的基线网络的Hausdorff距离(HD)值的箱线图,使用 OAI AKOA 数据集通过5折交叉验证进行评估。这些HD值是在后处理后的结果。请注意,由于 𝑈𝑁𝐸𝑇 𝑇 在髌骨软骨(PC)标签上未能收敛,因此该标签已从图中排除。
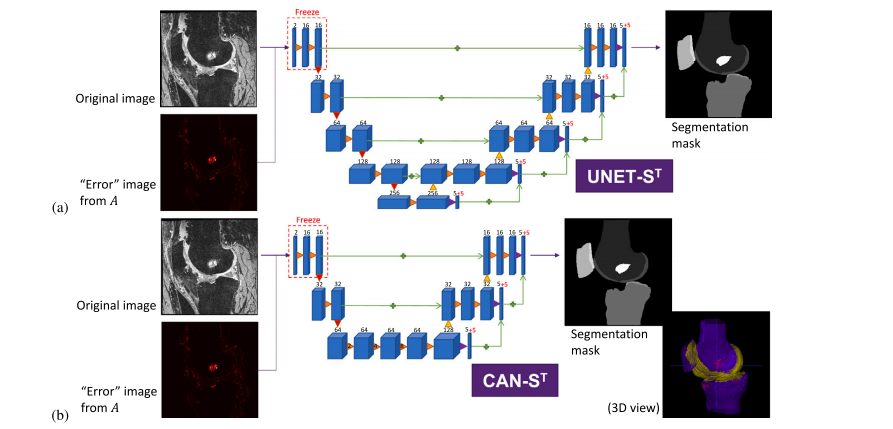
Fig. A.10. The anomaly-aware segmentation network 𝑆 𝑇 for transfer learning based on (a) 3D U-Net and (b) 3D CAN. The network 𝑆 𝑇 is a slight modification from 𝑆 (Fig. 3)where 5 more channels were added to the output layer. During training, the first two convolution blocks were frozen.
图A.10. 用于迁移学习的异常感知分割网络 𝑆 𝑇,基于 (a) 3D U-Net 和 (b) 3D CAN。网络 𝑆 𝑇 是对 𝑆(图3)进行的轻微修改,输出层增加了5个通道。在训练过程中,前两个卷积块被冻结。
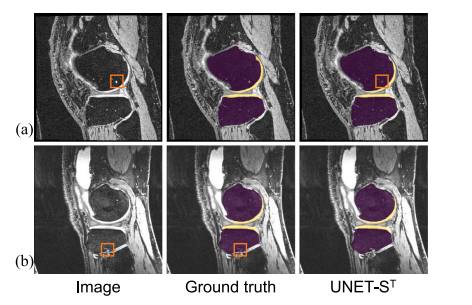
Fig. C.11. Example of (a) ''false positive'' and (b) ''false negative'' case, which mostlyoccurred with very small lesions. The orange boxes highlight the small lesions.
图C.11. (a)"假阳性"和 (b)"假阴性"案例的示例,主要发生在非常小的病变中。橙色框突出显示了小病变。
Table
表
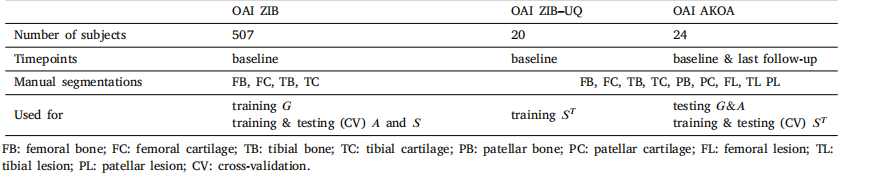
Table 1Summary of the datasets used in the current study.
表1本研究中使用的数据集摘要。
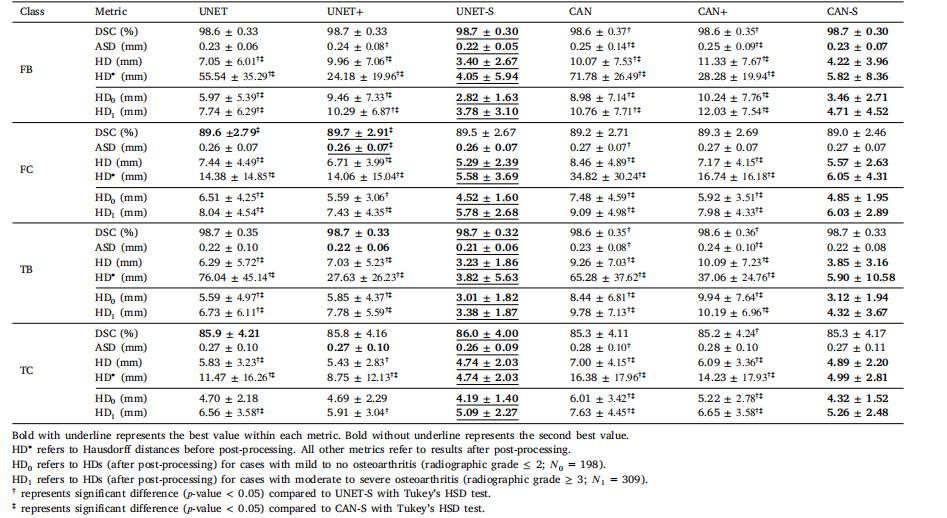
Table 2Mean DSC, ASD, and HD values for segmentations of the femoral and tibial bone and cartilage volumes from the proposed anomaly-aware method (𝑈𝑁𝐸𝑇 -𝑆 and 𝐶𝐴𝑁-𝑆) withtheir baseline networks, evaluated using 5-fold cross-validation on the OAI ZIB dataset (𝑁 = 507).
表2使用5折交叉验证在 OAI ZIB 数据集(𝑁 = 507)上评估的股骨和胫骨骨骼及软骨体积的分割中,基于提出的异常感知方法(𝑈𝑁𝐸𝑇-𝑆 和 𝐶𝐴𝑁-𝑆)与其基线网络的平均Dice相似系数(DSC)、平均表面距离(ASD)和Hausdorff距离(HD)值。
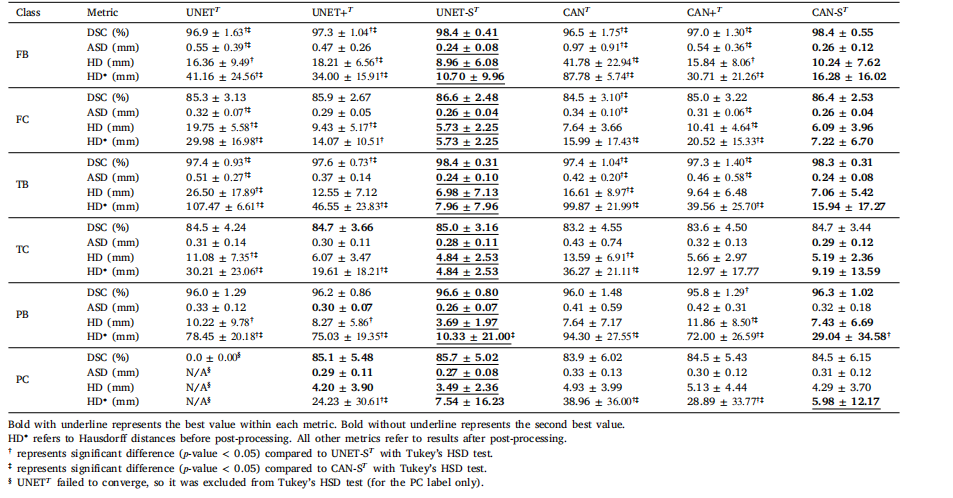
Table 3Mean DSC, ASD, and HD values for segmentation of the femoral, tibial, and patellar bone and cartilage volumes from the proposed anomaly-aware method (𝑈𝑁𝐸𝑇 -𝑆 𝑇 and𝐶𝐴𝑁-𝑆* 𝑇 ) with their baseline networks with transfer learning, evaluated using 5-fold cross-validation on the OAI AKOA dataset (𝑁 = 24 × 2).
表3使用迁移学习的基于异常感知方法(𝑈𝑁𝐸𝑇-𝑆 𝑇 和 𝐶𝐴𝑁-𝑆 𝑇)及其基线网络对股骨、胫骨和髌骨的骨骼及软骨体积进行分割的平均Dice相似系数(DSC)、平均表面距离(ASD)和Hausdorff距离(HD)值,使用 OAI AKOA 数据集(𝑁 = 24 × 2)通过5折交叉验证进行评估。
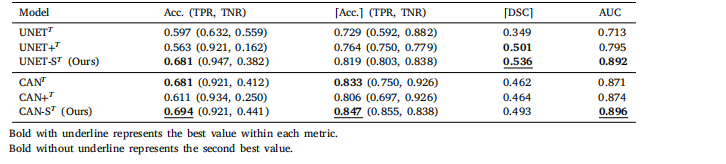
Table 4Bone lesion detection and segmentation performance on the OAI AKOA dataset in terms of accuracy and mean DSC. Here,Acc. refers to the accuracy with no post-processing while ⌈Acc.⌉ refers to the highest accuracy achieved with post-processing.Both are reported with the corresponding sensitivity (TPR) and specificity (TNR). ⌈DSC⌉ is the highest mean DSC (averagedover all bone lesions) achieved with post-processing. AUC is the area under the receiver operating characteristic (ROC) curve.Note that 𝑈𝑁𝐸𝑇 𝑇 failed to converge for the patellar lesion label. Results for each bone can be found in the supplementarymaterial.
表4OAI AKOA 数据集上骨损伤检测和分割性能的准确率和平均DSC。这里,Acc. 表示无后处理的准确率,⌈Acc.⌉ 表示通过后处理达到的最高准确率。两者都报告了相应的敏感性(TPR)和特异性(TNR)。⌈DSC⌉ 是通过后处理达到的最高平均DSC(在所有骨损伤上取平均)。AUC 是受试者工作特征(ROC)曲线下面积。请注意,𝑈𝑁𝐸𝑇 𝑇 在髌骨损伤标签上未能收敛。每个骨的结果可以在补充材料中找到。