正解/逆解
求正解/逆解有现成的库,参考https://github.com/petercorke/robotics-toolbox-python,代码如下:
import roboticstoolbox as rtb
import numpy as np
np.set_printoptions(precision=6, suppress=True)
robot = rtb.models.Panda()
qr = np.array([0, -0.3, 0, -2.2, 0, 2.0, np.pi / 4])
qz = np.zeros(7)
print("正解")
te = robot.fkine(qr)
print(te.data[0])
print("逆解")
# 可能存在多个逆解,若不设置seed, 多次执行返回的结果可能不一样
# q1 = robot.ikine_LM(te.data[0], q0=qz).q
q1 = robot.ikine_LM(te.data[0], q0=qz, seed=1234).q
print(q1)
# 检查逆解是否正确
assert np.allclose(te.data[0], robot.fkine(q1).data[0])
输出:
正解
[[ 0.995004 0. 0.099833 0.484047]
[ 0. -1. -0. -0. ]
[ 0.099833 0. -0.995004 0.41263 ]
[ 0. 0. 0. 1. ]]
逆解
[ 2.684527 0.329245 -2.734035 -2.197693 0.147658 1.990311 0.668895]
可视化也很方便
robot.plot(qr, backend="swift", block=True)
输出:
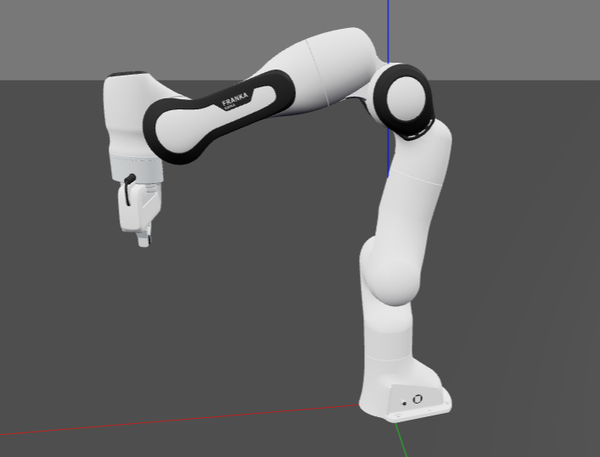
STEP格式文件
可以将STEP格式转换为GLB格式,参考https://github.com/trimesh/cascadio,代码如下:
import cascadio
cascadio.step_to_glb("wrist_mount.step", "wrist_mount.glb", 0.1, 0.5)
import trimesh
trimesh.load("wrist_mount.glb").show()
摄像头基础知识
焦点/焦距/视场角/光圈/景深/光学畸变,参考https://www.optmv.com/content/details113_4276.html