Modulation Classification
Using CNN to classify RF modulation data.
Dataset is from: DATA LINK
paper: Over the Air Deep Learning Based Radio Signal Classification
Data Preprocessing
Data is processed. Column data are a two variable label composed of the Modulation and SNR, Row 0 is the binary encoded version of the Modulation and SNR, Row 1 is the actual data, each column is a 2, 128 array of I and Q data for the specified Modulation and SNR in the column label.
Build the CNN
python
from keras.models import Sequential
from keras.layers import Dense, Activation, Dropout, Conv1D, MaxPooling1D, GlobalAveragePooling1D, Flatten
verbose, epochs, batch_size = 1, 256, 1024
n_timesteps, n_features, n_outputs = xTrain.shape[1], xTrain.shape[2], yTrain.shape[1]
print('timesteps=', n_timesteps, 'features=', n_features, 'outputs=', n_outputs)
model = Sequential()
model.add(Conv1D(filters=64, kernel_size=3, activation='relu', input_shape=(n_timesteps, n_features)))
model.add(Conv1D(filters=64, kernel_size=3, activation='relu'))
model.add(Dropout(0.5))
model.add(MaxPooling1D(pool_size=2))
model.add(Flatten())
model.add(Dense(100, activation='relu'))
model.add(Dense(n_outputs, activation='softmax'))
model.compile(loss='categorical_crossentropy', optimizer='adam', metrics=['accuracy'])
#model.compile(RAdam(), loss='categorical_crossentropy', metrics=['accuracy'])
print(model.summary())
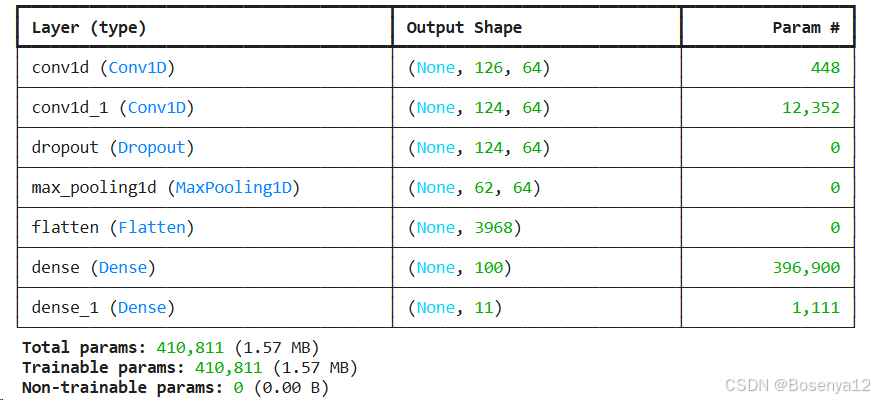