2024/11/5-2024/11/7:
前置知识:
[译] 理解 LSTM(Long Short-Term Memory, LSTM) 网络 - wangduo - 博客园
【官方双语】LSTM(长短期记忆神经网络)StatQuest_哔哩哔哩_bilibili
大部分思路来自于:
部分常量设置与官方api使用:
其实在实现RNN之后可以发现,lstm基本是同样的套路。 在看完上面的前置知识之后,理解三个门的作用即可对lstm有一个具体的认识,这里不再赘述。
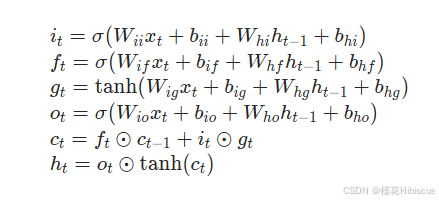
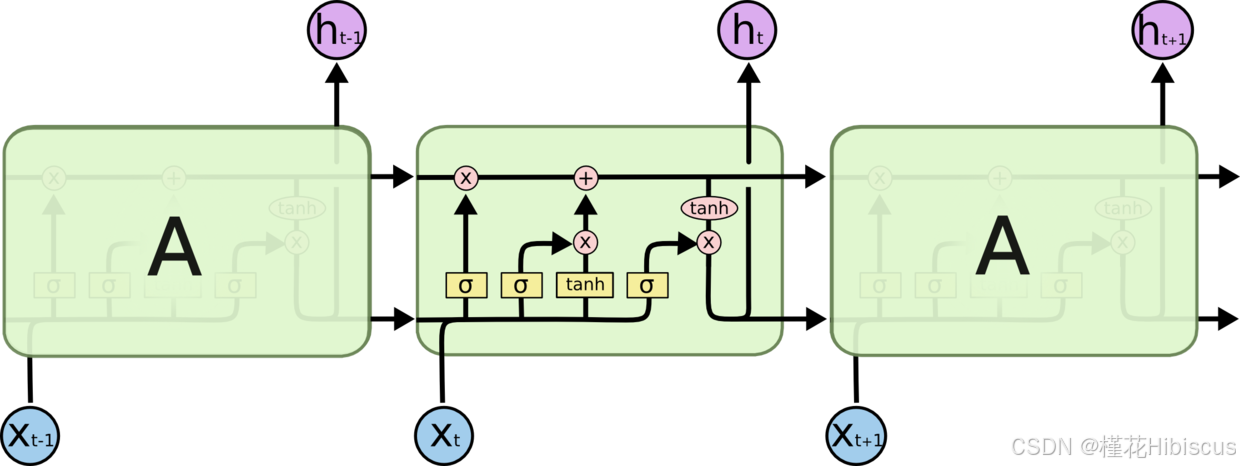
关于输入设置这方面,参考如下:
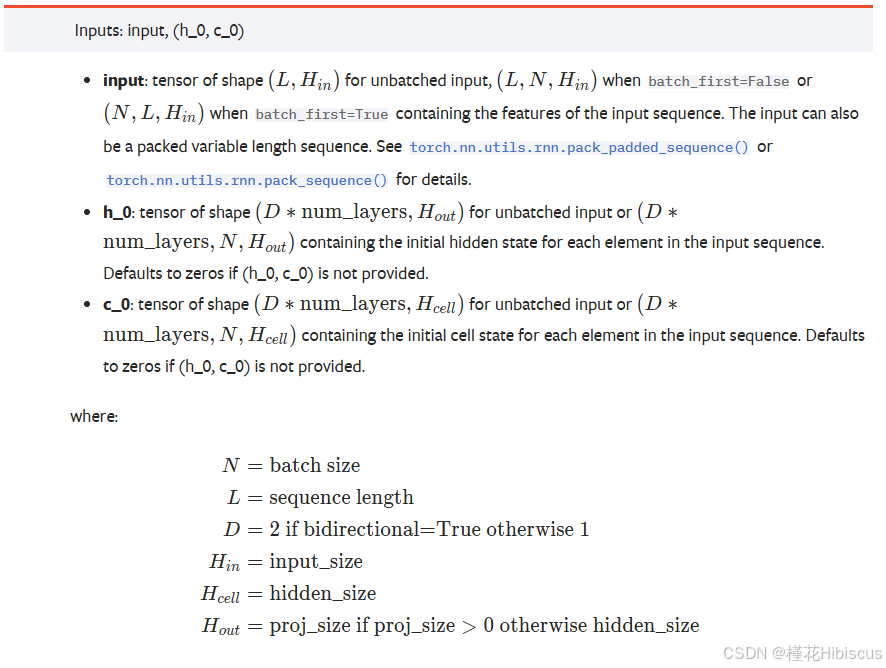
python
# 定义常量
bs, T, i_size, h_size = 2, 3, 4, 5
# 输入序列
input = torch.randn(bs, T, i_size)
# 初始值,不需要训练
c0 = torch.randn(bs, h_size)
h0 = torch.randn(bs, h_size)
将定义的常量输入官方api:
python
# 调用官方api
lstm_layer = nn.LSTM(i_size, h_size, batch_first=True)
# 单层单项lstm,h0与c0的第0维度为 D(是否双向)*num_layers 故增加0维,维度为1
output, (h_final, c_final) = lstm_layer(input, (h0.unsqueeze(0), c0.unsqueeze(0)))
print(output.shape)
print(output)
for k, v in lstm_layer.named_parameters():
print(k)
print(v.shape)
输出如下:
torch.Size([2, 3, 5])
tensor([[[ 0.1134, -0.1032, 0.1496, 0.1853, -0.3758],
[ 0.1831, 0.0223, 0.0377, 0.0867, -0.1090],
[ 0.1233, 0.1121, 0.0574, -0.0401, -0.1576]],
[[-0.2761, 0.3259, 0.1687, -0.0632, 0.2046],
[ 0.1796, 0.3110, 0.0974, 0.0294, 0.0220],
[ 0.1205, 0.1815, 0.0840, -0.1714, -0.1216]]],
grad_fn=<TransposeBackward0>)
weight_ih_l0
torch.Size([20, 4])
weight_hh_l0
torch.Size([20, 5])
bias_ih_l0
torch.Size([20])
bias_hh_l0
torch.Size([20])
可以看到LSTM的内置参数有 weight_ih_l0、weight_hh_l0、bias_ih_l0、bias_hh_l0,将关于三个门的知识结合在一起看差不多就明白接下来应该怎么做了:
h和c都是统一经过*weight+bias的操作,加在一起后经过tahn或者sigmoid激活函数,最后或点乘或加在h或者c上进行对参数的更新。只要不把维度的对应关系搞混还是比较好复现的。
需要注意的是:三个门中的四个weight和bias(遗忘门一个,输入门两个,输出门一个)全部都按照第0维度拼在了一起方便同时进行矩阵运算,所以我们可以看到这些权重和偏置的第0维度的大小为4*h_size。一开始这一点也带给了我比较大的困惑。
代码复现与验证:
代码较为简单,跟上次实现RNN的思路也差不多,基本是照着官方api那给的公式一步一步来的:
python
# 代码复现
def lstm_forward(input, initial_states, w_ih, w_hh, b_ih, b_hh):
h0, c0 = initial_states
bs, T, i_size = input.shape
h_size = w_ih.shape[0] // 4
prev_h = h0 # [bs, h_size]
prev_c = c0 # [bs, h_size]
"""
w_ih # 4*h_size, i_size
w_hh # 4*h_size, h_size
"""
# 输出序列
output_size = h_size
output = torch.zeros(bs, T, output_size)
for t in range(T):
x = input[:, t, :] # 当前时刻输入向量, [bs, i_size]
w_times_x = torch.matmul(w_ih, x.unsqueeze(-1)).squeeze(-1) # [bs, 4*h_size]
w_times_h_prev = torch.matmul(w_hh, prev_h.unsqueeze(-1)).squeeze(-1) # [bs, 4*h_size]
# 分别计算输入门(i),遗忘门(f),输出门(o),cell(g)
i_t = torch.sigmoid(w_times_x[:, : h_size] + w_times_h_prev[:, : h_size] + b_ih[: h_size] + b_hh[: h_size])
f_t = torch.sigmoid(w_times_x[:, h_size: 2*h_size] + w_times_h_prev[:, h_size: 2*h_size] +
b_ih[h_size: 2*h_size] + b_hh[h_size: 2*h_size])
g_t = torch.tanh(w_times_x[:, 2*h_size: 3*h_size] + w_times_h_prev[:, 2*h_size: 3*h_size] +
b_ih[2*h_size: 3*h_size] + b_hh[2*h_size: 3*h_size])
o_t = torch.sigmoid(w_times_x[:, 3*h_size:] + w_times_h_prev[:, 3*h_size:] + b_ih[3*h_size:] + b_hh[3*h_size:])
# 更新流
prev_c = f_t * prev_c + i_t * g_t
prev_h = o_t * torch.tanh(prev_c)
output[:, t, :] = prev_h
return output, (prev_h, prev_c)
输出结果对比验证:
python
# 调用官方api
lstm_layer = nn.LSTM(i_size, h_size, batch_first=True)
# 单层单项lstm,h0与c0的第0维度为 D(是否双向)*num_layers 故增加0维,维度为1
output, (h_final, c_final) = lstm_layer(input, (h0.unsqueeze(0), c0.unsqueeze(0)))
print(output.shape)
print(output)
for k, v in lstm_layer.named_parameters():
print(k, v.shape)
output, (h_final, c_final) = lstm_forward(input, (h0, c0), lstm_layer.weight_ih_l0,
lstm_layer.weight_hh_l0, lstm_layer.bias_ih_l0, lstm_layer.bias_hh_l0)
print(output)
结果如下:
torch.Size([2, 3, 5])
tensor([[[-0.6394, -0.1796, 0.0831, 0.0816, -0.0620],
[-0.5798, -0.2235, 0.0539, -0.0120, -0.0272],
[-0.4229, -0.0798, -0.0762, -0.0030, -0.0668]],
[[ 0.0294, 0.3240, -0.4318, 0.5005, -0.0223],
[-0.1458, 0.0472, -0.1115, 0.3445, 0.3558],
[-0.2922, -0.1013, -0.1755, 0.3065, 0.1130]]],
grad_fn=<TransposeBackward0>)
weight_ih_l0 torch.Size([20, 4])
weight_hh_l0 torch.Size([20, 5])
bias_ih_l0 torch.Size([20])
bias_hh_l0 torch.Size([20])
tensor([[[-0.6394, -0.1796, 0.0831, 0.0816, -0.0620],
[-0.5798, -0.2235, 0.0539, -0.0120, -0.0272],
[-0.4229, -0.0798, -0.0762, -0.0030, -0.0668]],
[[ 0.0294, 0.3240, -0.4318, 0.5005, -0.0223],
[-0.1458, 0.0472, -0.1115, 0.3445, 0.3558],
[-0.2922, -0.1013, -0.1755, 0.3065, 0.1130]]], grad_fn=<CopySlices>)
复现成功。
appendix:
这里放下LSTMP的参数设置:
python
# lstmp对h_size进行压缩
proj_size = 3
# h0的h_size也改为proj_size,而c0不变
h0 = torch.randn(bs, proj_size)
# 调用官方api
lstmp_layer = nn.LSTM(i_size, h_size, batch_first=True, proj_size=proj_size)
# 单层单项lstm,h0与c0的第0维度为 D(是否双向)*num_layers 故增加0维,维度为1
output, (h, c) = lstmp_layer(input, (h0.unsqueeze(0), c0.unsqueeze(0)), )
print(output)
print(output.shape)
print(h.shape)
print(c.shape)
for k, v in lstmp_layer.named_parameters():
print(k, v.shape)
tensor([[[-0.0492, 0.0265, 0.0883],
[-0.1028, -0.0327, -0.0542],
[ 0.0250, -0.0231, -0.1199]],
[[-0.2417, -0.1737, -0.0755],
[-0.2351, -0.0837, -0.0376],
[-0.2527, -0.0258, -0.0236]]], grad_fn=<TransposeBackward0>)
torch.Size([2, 3, 3])
torch.Size([1, 2, 3])
torch.Size([1, 2, 5])
weight_ih_l0 torch.Size([20, 4])
weight_hh_l0 torch.Size([20, 3])
bias_ih_l0 torch.Size([20])
bias_hh_l0 torch.Size([20])
weight_hr_l0 torch.Size([3, 5])
其实LSTMP就多出了个weight_hr_l0对h进行压缩,但是不对cell压缩,目的是减少lstm的参数量,在小一点的sequence上基本没啥区别。若要支持lstmp,在前面的代码上改动几行即可:
python
def lstm_forward(input, initial_states, w_ih, w_hh, b_ih, b_hh, w_hr=None):
h0, c0 = initial_states
bs, T, i_size = input.shape
h_size = w_ih.shape[0] // 4
prev_h = h0 # [bs, h_size]
prev_c = c0 # [bs, h_size]
"""
w_ih # 4*h_size, i_size
w_hh # 4*h_size, h_size
"""
if w_hr is not None:
# 输出压缩至p_size
p_size = w_hr.shape[0]
output_size = p_size
else:
output_size = h_size
output = torch.zeros(bs, T, output_size)
for t in range(T):
x = input[:, t, :] # 当前时刻输入向量, [bs, i_size]
w_times_x = torch.matmul(w_ih, x.unsqueeze(-1)).squeeze(-1) # [bs, 4*h_size]
w_times_h_prev = torch.matmul(w_hh, prev_h.unsqueeze(-1)).squeeze(-1) # [bs, 4*h_size]
# 分别计算输入门(i),遗忘门(f),输出门(o),cell(g)
i_t = torch.sigmoid(w_times_x[:, : h_size] + w_times_h_prev[:, : h_size] + b_ih[: h_size] + b_hh[: h_size])
f_t = torch.sigmoid(w_times_x[:, h_size: 2*h_size] + w_times_h_prev[:, h_size: 2*h_size] +
b_ih[h_size: 2*h_size] + b_hh[h_size: 2*h_size])
g_t = torch.tanh(w_times_x[:, 2*h_size: 3*h_size] + w_times_h_prev[:, 2*h_size: 3*h_size] +
b_ih[2*h_size: 3*h_size] + b_hh[2*h_size: 3*h_size])
o_t = torch.sigmoid(w_times_x[:, 3*h_size:] + w_times_h_prev[:, 3*h_size:] + b_ih[3*h_size:] + b_hh[3*h_size:])
# 更新流
prev_c = f_t * prev_c + i_t * g_t
prev_h = o_t * torch.tanh(prev_c) # [bs, h_size]
if w_hr is not None:
prev_h = torch.matmul(w_hr, prev_h.unsqueeze(-1)).squeeze(-1) # [bs, p_size]
output[:, t, :] = prev_h
return output, (prev_h, prev_c)
经过验证,复现成功。