Numpy
创建数组
import numpy as np
创建数组
arr1=np.array([1,2,3])
arr2=np.array([[3,4,5],[2,4,1]])
print(arr1)
print(arr2)
print(type(arr1))
1 2 3
\[3 4 5
2 4 1\]
<class 'numpy.ndarray'>
查看数组的基础属性
print(arr1.shape)
print(arr1.ndim)
print(arr1.dtype)
print(arr2.shape)
print(arr2.ndim)
print(arr2.dtype)
(3,)
1
int32
(2, 3)
2
int32
初识数组特点
list1=([0.3,0.5,4.2])
arr1=np.array([0.3,0.5,4.2])
print(list1)
print(arr1)
print(list1 ** 2)
print([i**2 for i in list1])
print(arr1 ** 2)
0.3, 0.5, 4.2
0.3 0.5 4.2
0.09, 0.25, 17.64
0.09 0.25 17.64
创建常见数组
arr3=np.arange(0,10)
arr4=np.arange(10)
arr5=np.arange(0,1,0.1)
print(arr3)
print(arr4)
print(arr5)
0 1 2 3 4 5 6 7 8 9
0 1 2 3 4 5 6 7 8 9
0. 0.1 0.2 0.3 0.4 0.5 0.6 0.7 0.8 0.9
arr6=np.linspace(0,1,10)
print(arr6)
0. 0.1 0.2 0.3 0.4 0.5 0.6 0.7 0.8 0.9 1.
arr7=np.zeros([3,4,5])
print(arr7)
\[\[ 0. 0. 0. 0. 0.
0. 0. 0. 0. 0.
0. 0. 0. 0. 0.
0. 0. 0. 0. 0.\]
\[ 0. 0. 0. 0. 0.
0. 0. 0. 0. 0.
0. 0. 0. 0. 0.
0. 0. 0. 0. 0.\]
\[ 0. 0. 0. 0. 0.
0. 0. 0. 0. 0.
0. 0. 0. 0. 0.
0. 0. 0. 0. 0.\]\]
arr8=np.ones([3,4,5])
print(arr8)
\[\[ 1. 1. 1. 1. 1.
1. 1. 1. 1. 1.
1. 1. 1. 1. 1.
1. 1. 1. 1. 1.\]
\[ 1. 1. 1. 1. 1.
1. 1. 1. 1. 1.
1. 1. 1. 1. 1.
1. 1. 1. 1. 1.\]
\[ 1. 1. 1. 1. 1.
1. 1. 1. 1. 1.
1. 1. 1. 1. 1.
1. 1. 1. 1. 1.\]\]
数组的数据类型
arr8=np.array([3,4,5],dtype=np.float) # 声明数组的数据类型
print(arr8)
print(arr8.dtype)
arr8[0]=1.2
print(arr8)
print(np.int32(arr8)) # 转换数组的数据类型
3. 4. 5.
float64
1.2 4. 5.
1 4 5
生成随机数
print(np.random.random(10))
0.73744669 0.80080002 0.69015703 0.51743988 0.05570415 0.34901843 0.0734643 0.86541768 0.18830607 0.07036505
print(np.random.rand(10))
0.27580848 0.69278954 0.12912278 0.53180506 0.21617686 0.4357371 0.92604774 0.19278177 0.81396217 0.15550441
print(np.random.rand(3,4))
print(np.random.randn(3,4))
\[ 0.03654586 0.23675581 0.35543946 0.31476077
0.12875907 0.78566879 0.87653987 0.78687239
0.79805071 0.46032083 0.08375826 0.60476404\]
\[ 0.22623064 -0.34199973 -0.50766523 0.01726679
0.71462127 -1.19509683 -0.3916739 0.67375221
-1.02150652 0.98995901 1.64691806 0.81784057\]
数组的索引
arr1=np.array([0.3,0.78,0.24,5,3.2])
print(arr1)
print(arr1[0])
print(arr1[-5])
print(arr1[1:2])
print(arr1[-4:-2])
0.3 0.78 0.24 5. 3.2
0.3
0.3
0.78
0.78 0.24
逻辑型索引
arr2=np.array([2.3,1.8,4.5])
print(arr2)
print(arr2[[False,False,True]])
index=arr2>2
print(arr2[index])
2.3 1.8 4.5
4.5
2.3 4.5
多维数组的索引
arr3=np.arange(1,13).reshape([3,4])
print(arr3)
print(arr3[2,3])
print(arr3[2,0:])
print(arr3[:,3])
print(arr3[1:,1:3])
\[ 1 2 3 4
5 6 7 8
9 10 11 12\]
12
9 10 11 12
4 8 12
\[ 6 7
10 11\]
\[ 5 6 7 8
9 10 11 12\]
False True True
修改数组中的元素
arr3=np.arange(1,13).reshape([3,4])
print(arr3)
arr3[0,0]=15
print(arr3)
\[ 1 2 3 4
5 6 7 8
9 10 11 12\]
\[15 2 3 4
5 6 7 8
9 10 11 12\]
求解距离矩阵
n=10 # 样本个数
x=np.linspace(1,100,n) # 样本的横坐标
y=np.linspace(1,100,n) # 样本的纵坐标
dist=np.sqrt((x[0]-x[1])**2+(y[0]-y[1])**2)
dist = np.zeros([n, n]) # 初始距离矩阵
for i in range(n):
for j in range(n):
dist[i, j] = np.sqrt((x[i] - x[j])**2 + (y[i] - y[j])**2) # 计算欧式距离
print(x)
print(y)
print(dist)
1. 12. 23. 34. 45. 56. 67. 78. 89. 100.
1. 12. 23. 34. 45. 56. 67. 78. 89. 100.
\[ 0. 15.55634919 31.11269837 46.66904756 62.22539674 77.78174593 93.33809512 108.8944443 124.45079349 140.00714267
15.55634919 0. 15.55634919 31.11269837 46.66904756 62.22539674 77.78174593 93.33809512 108.8944443 124.45079349
31.11269837 15.55634919 0. 15.55634919 31.11269837 46.66904756 62.22539674 77.78174593 93.33809512 108.8944443
46.66904756 31.11269837 15.55634919 0. 15.55634919 31.11269837 46.66904756 62.22539674 77.78174593 93.33809512
62.22539674 46.66904756 31.11269837 15.55634919 0. 15.55634919 31.11269837 46.66904756 62.22539674 77.78174593
77.78174593 62.22539674 46.66904756 31.11269837 15.55634919 0. 15.55634919 31.11269837 46.66904756 62.22539674
93.33809512 77.78174593 62.22539674 46.66904756 31.11269837 15.55634919 0. 15.55634919 31.11269837 46.66904756
108.8944443 93.33809512 77.78174593 62.22539674 46.66904756 31.11269837 15.55634919 0. 15.55634919 31.11269837
124.45079349 108.8944443 93.33809512 77.78174593 62.22539674 46.66904756 31.11269837 15.55634919 0. 15.55634919
140.00714267 124.45079349 108.8944443 93.33809512 77.78174593 62.22539674 46.66904756 31.11269837 15.55634919 0. \]
数组形态变化
arr4=np.arange(1,13)
print(arr4)
print(arr4.reshape([3,4]))
arr5=arr4.reshape([3,4])
print(arr5.ravel()) # 数组的展平
print(arr5.flatten('F')) #数组的纵向展平
arr6=arr4.reshape([3,4])
print(np.hstack((arr5,arr6))) # 数组的横向拼接
print(np.vstack((arr5,arr6))) # 数组的纵向拼接
1 2 3 4 5 6 7 8 9 10 11 12
\[ 1 2 3 4
5 6 7 8
9 10 11 12\]
1 2 3 4 5 6 7 8 9 10 11 12
1 5 9 2 6 10 3 7 11 4 8 12
\[ 1 2 3 4 1 2 3 4
5 6 7 8 5 6 7 8
9 10 11 12 9 10 11 12\]
\[ 1 2 3 4
5 6 7 8
9 10 11 12
1 2 3 4
5 6 7 8
9 10 11 12\]
掌握 NumPy 矩阵与通用函数
import numpy as np
matr1 = np.mat("1 2 3;4 5 6;7 8 9")
print(type(matr1))
matr2 = np.matrix([[1, 2, 3],[4, 5, 6],[7, 8, 9]])
np.bmat('matr1 matr2; matr1, matr2')
<class 'numpy.matrix'>
matrix([[1, 2, 3, 1, 2, 3],
4, 5, 6, 4, 5, 6\], \[7, 8, 9, 7, 8, 9\], \[1, 2, 3, 1, 2, 3\], \[4, 5, 6, 4, 5, 6\], \[7, 8, 9, 7, 8, 9\]\]) # 通用函数 arr1 = np.array(\[0.2, 0.4, 0.6\]) arr2=np.array(\[0.2,0.6,0.78\]) list1 = \[0.2, 0.4, 0.6
print(arr1+1)
print(arr1-1)
print(arr1*2)
print(arr1/2)
print(list1*2)
print(arr1+arr2)
print(arr1>0)
print(arr1<arr2)
print(np.any(arr1==0.2))
print(np.all(arr2==0.2))
1.2 1.4 1.6
-0.8 -0.6 -0.4
0.4 0.8 1.2
0.1 0.2 0.3
0.2, 0.4, 0.6, 0.2, 0.4, 0.6
0.4 1. 1.38
True True True
False True True
True
False
广播机制
arr3 = np.arange(1, 13).reshape([4, 3])
arr4 = np.array([1, 2, 3])
arr5 = np.array([[1], [2], [3], [4]])
print(arr3)
print(arr4)
print(arr5)
print(arr3+arr4)
print(arr3+arr5)
\[ 1 2 3
4 5 6
7 8 9
10 11 12\]
1 2 3
\[1
2
3
4\]
\[ 2 4 6
5 7 9
8 10 12
11 13 15\]
\[ 2 3 4
6 7 8
10 11 12
14 15 16\]
利用 NumPy 进行统计分析
import numpy as np
读写二进制文件
arr1 = np.arange(1, 13).reshape([4, 3])
arr2 = np.arange(1, 13).reshape([3, 4])
print(arr1)
print(arr2)
\[ 1 2 3
4 5 6
7 8 9
10 11 12\]
\[ 1 2 3 4
5 6 7 8
9 10 11 12\]
读写txt文件
np.savetxt('tmp/arr1.txt', arr1, delimiter=',') # 保存数据
np.loadtxt('tmp/arr1.txt', delimiter=',') # 读取数据
array([[ 1., 2., 3.],
4., 5., 6.\], \[ 7., 8., 9.\], \[10., 11., 12.\]\]) # 使用数组进行简单统计分析 arr3 = np.random.randint(1, 10, (3, 4)) print(arr3) arr3.sort(axis=0) print(arr3) print(arr3.argsort(axis=0)) print(np.tile(arr3, 2)) print(np.repeat(arr3, 2, axis=1)) print(arr3.mean()) print(arr3.mean(axis=0)) print(arr3.max(axis=0)) print(arr3.argmax(axis=0)) \[\[8 6 7 3
2 9 9 4
2 5 2 3\]
\[2 5 2 3
2 6 7 3
8 9 9 4\]
\[0 0 0 0
1 1 1 1
2 2 2 2\]
\[2 5 2 3 2 5 2 3
2 6 7 3 2 6 7 3
8 9 9 4 8 9 9 4\]
\[2 2 5 5 2 2 3 3
2 2 6 6 7 7 3 3
8 8 9 9 9 9 4 4\]
5.0
4. 6.66666667 6. 3.33333333
8 9 9 4
2 2 2 2
Pandas
import pandas as pd
读取文本数据
pd.read_csv?
data_txt = pd.read_csv('data/meal_order_info.txt',sep=' ')
data_csv = pd.read_csv('data/meal_order_info.csv', encoding='gbk', header=0)
data_csv
将数据框存储为文本文件数据
data_csv.to_csv('tmp/data_csv.csv',index=None, encoding='gbk')
data_csv
读取Excel文件
data_excel = pd.read_excel('data/meal_order_detail.xlsx',sheet_name='meal_order_detail2')
data_excel
data_excel.to_excel('tmp/data_excel.xlsx', index=None, sheet_name='test1')
掌握DataFrame的常用操作
import pandas as pd
Series系列
ser1 = pd.Series([1,2,'a'],index=['a','b','c'])
print(ser1)
ser2 = pd.Series({'a':[1,2,3],'b':['1','2','3']})
print(ser2)
a 1
b 2
c a
dtype: object
a [1, 2, 3]
b [1, 2, 3]
dtype: object
构造数据框(DataFrame)
d=[[1.3,2.0,3,4],[2,4,1,4],[2,5,1.9,7],[3,1,0,11]]
print(d)
df = pd.DataFrame(d, index=['a', 'b', 'c', 'd'], columns=['A', 'B', 'C', 'D'])
print(df)
d={'color':['blue','green','yellow','red','white'],
'object':['ball','pen','pencil','paper','mug'],
'price':[1.2,1.0,0.6,0.9,1.7]}
frame = pd.DataFrame(d,index=['a','b','c','d','e'])
print(frame)
print(pd.DataFrame(index=[1, 2], columns=[1, 2]))
print(pd.DataFrame(1, index=[1, 2], columns=[1, 2]))
\[1.3, 2.0, 3, 4\], \[2, 4, 1, 4\], \[2, 5, 1.9, 7\], \[3, 1, 0, 11\]
A B C D
a 1.3 2.0 3.0 4
b 2.0 4.0 1.0 4
c 2.0 5.0 1.9 7
d 3.0 1.0 0.0 11
color object price
a blue ball 1.2
b green pen 1.0
c yellow pencil 0.6
d red paper 0.9
e white mug 1.7
1 2
1 NaN NaN
2 NaN NaN
1 2
1 1 1
2 1 1
数据框的常用属性
d=[[1.3,2.0,3,4],[2,4,1,4],[2,5,1.9,7],[3,1,0,11]]
df = pd.DataFrame(d, index=['a', 'b', 'c', 'd'], columns=['A', 'B', 'C', 'D'])
print(df)
print(df.values)
print(df.index)
print(df.shape)
print(df.dtypes)
A B C D
a 1.3 2.0 3.0 4
b 2.0 4.0 1.0 4
c 2.0 5.0 1.9 7
d 3.0 1.0 0.0 11
\[ 1.3 2. 3. 4.
2. 4. 1. 4.
2. 5. 1.9 7.
3. 1. 0. 11. \]
Index(['a', 'b', 'c', 'd'], dtype='object')
(4, 4)
A float64
B float64
C float64
D int64
dtype: object
数据框的查改增删操作
import pandas as pd
访问数据框中的元素
d=[[1.3,2.0,3,4],[2,4,1,4],[2,5,1.9,7],[3,1,0,11]]
df = pd.DataFrame(d, index=['a', 'b', 'c', 'd'], columns=['A', 'B', 'C', 'D'])
print(df)
print(df['A']) # 单列数据访问
print(df[['A', 'C']]) # 多列数据访问
A B C D
a 1.3 2.0 3.0 4
b 2.0 4.0 1.0 4
c 2.0 5.0 1.9 7
d 3.0 1.0 0.0 11
a 1.3
b 2.0
c 2.0
d 3.0
Name: A, dtype: float64
A C
a 1.3 3.0
b 2.0 1.0
c 2.0 1.9
d 3.0 0.0
print(df.head(3)) # 访问某几行数据
print(df.tail(3))
A B C D
a 1.3 2.0 3.0 4
b 2.0 4.0 1.0 4
c 2.0 5.0 1.9 7
A B C D
b 2.0 4.0 1.0 4
c 2.0 5.0 1.9 7
d 3.0 1.0 0.0 11
print(df)
print(df.iloc[0, 0]) # 按照行列顺序进行数据访问
print(df.iloc[0:3, 0])
print(df.iloc[:, 0])
print(df.iloc[0, :])
print(df.iloc[1:3, 1:3])
A B C D
a 1.3 2.0 3.0 4
b 2.0 4.0 1.0 4
c 2.0 5.0 1.9 7
d 3.0 1.0 0.0 11
1.3
a 1.3
b 2.0
c 2.0
Name: A, dtype: float64
a 1.3
b 2.0
c 2.0
d 3.0
Name: A, dtype: float64
A 1.3
B 2.0
C 3.0
D 4.0
Name: a, dtype: float64
B C
b 4.0 1.0
c 5.0 1.9
<class 'pandas.core.series.Series'>
print(df.loc['a', 'A']) # 按照行列名称进行数据访问
print(df.loc['a':'c', 'A'])
print(df.loc[:, 'A'])
print(df.loc['a', :])
print(df.loc[['b','c'], ['B', 'C']])
1.3
a 1.3
b 2.0
c 2.0
Name: A, dtype: float64
a 1.3
b 2.0
c 2.0
d 3.0
Name: A, dtype: float64
A 1.3
B 2.0
C 3.0
D 4.0
Name: a, dtype: float64
B C
b 4.0 1.0
c 5.0 1.9
注意如下方式返回值的区别
print(df.iloc[:, 0])
print(df.iloc[:, 0:1])
print(type(df.iloc[:, 0]))
print(type(df.iloc[:, 0:1]))
a 1.3
b 2.0
c 2.0
d 3.0
Name: A, dtype: float64
A
a 1.3
b 2.0
c 2.0
d 3.0
<class 'pandas.core.series.Series'>
<class 'pandas.core.frame.DataFrame'>
修改数据框中的元素
d=[[1.3,2.0,3,4],[2,4,1,4],[2,5,1.9,7],[3,1,0,11]]
df = pd.DataFrame(d, index=['a', 'b', 'c', 'd'], columns=['A', 'B', 'C', 'D'])
print(df)
df.loc['a', 'A'] = 101
df.loc[:, 'B'] = 0.25
df.loc[:, 'C'] = [1, 2, 3, 4]
print(df)
A B C D
a 1.3 2.0 3.0 4
b 2.0 4.0 1.0 4
c 2.0 5.0 1.9 7
d 3.0 1.0 0.0 11
A B C D
a 101.0 0.25 1 4
b 2.0 0.25 2 4
c 2.0 0.25 3 7
d 3.0 0.25 4 11
C:\Users\Administrator\AppData\Local\Temp\ipykernel_70148\2679651701.py:7: DeprecationWarning: In a future version, `df.iloc[:, i] = newvals` will attempt to set the values inplace instead of always setting a new array. To retain the old behavior, use either `df[df.columns[i]] = newvals` or, if columns are non-unique, `df.isetitem(i, newvals)`
df.loc[:, 'C'] = [1, 2, 3, 4]
为数据框增添数据
d=[[1.3,2.0,3,4],[2,4,1,4],[2,5,1.9,7],[3,1,0,11]]
df = pd.DataFrame(d, index=['a', 'b', 'c', 'd'], columns=['A', 'B', 'C', 'D'])
print(df)
df['E'] = 5
df['F'] = [1, 2, 3, 4]
print(df)
A B C D
a 1.3 2.0 3.0 4
b 2.0 4.0 1.0 4
c 2.0 5.0 1.9 7
d 3.0 1.0 0.0 11
A B C D E F
a 1.3 2.0 3.0 4 5 1
b 2.0 4.0 1.0 4 5 2
c 2.0 5.0 1.9 7 5 3
d 3.0 1.0 0.0 11 5 4
import pandas as pd
删除数据框中的元素
d=[[1.3,2.0,3,4],[2,4,1,4],[2,5,1.9,7],[3,1,0,11]]
df = pd.DataFrame(d, index=['a', 'b', 'c', 'd'], columns=['A', 'B', 'C', 'D'])
print(df)
print(df.drop('D'))
print(df.drop('D', axis=1, inplace=False)) # 删除数据框的列元素
print(df)
print(df.drop(['a', 'c'], axis=0)) # 输出数据框的行元素
A B C D
a 1.3 2.0 3.0 4
b 2.0 4.0 1.0 4
c 2.0 5.0 1.9 7
d 3.0 1.0 0.0 11
A B C
a 1.3 2.0 3.0
b 2.0 4.0 1.0
c 2.0 5.0 1.9
d 3.0 1.0 0.0
A B C D
a 1.3 2.0 3.0 4
b 2.0 4.0 1.0 4
c 2.0 5.0 1.9 7
d 3.0 1.0 0.0 11
A B C D
b 2.0 4.0 1.0 4
d 3.0 1.0 0.0 11
描述分析DataFrame数据
import numpy as np
import pandas as pd
d=[[1.3,2.0,3,4],[2,4,1,4],[2,5,1.9,7],[3,1,0,11]]
df = pd.DataFrame(d, index=['a', 'b', 'c', 'd'], columns=['A', 'B', 'C', 'D'])
print(df)
print(np.mean(df, axis=1))
print(df.mean(axis=1))
print(df.std())
print(df.describe())
print(df.T.describe())
df['A'].value_counts()
转换与处理时间序列数据
import pandas as pd
order = pd.read_csv('data/meal_order_info.csv', encoding='gbk')
print(order)
print(order['lock_time'].dtypes)
order['lock_time'] = pd.to_datetime(order['lock_time'])
print(order['lock_time'].dtypes)
print(pd.DatetimeIndex(order['lock_time']))
print(pd.PeriodIndex(order['lock_time'], freq='H'))
order['lock_time']
print(order['lock_time'][0].year) # 获取数据年份信息
print(order['lock_time'].dt.year) # 获取数据年份信息
print(order['lock_time'].dt.month) # 获取数据月份信息
print(order['lock_time'].dt.week) # 获取数据周次信息
print(order['lock_time'] + pd.Timedelta(days=1)) # 时间平移
print(order['lock_time'][1] - order['lock_time'][0]) # 求时间差别
使用分组聚合进行组内计算
import pandas as pd
detail = pd.read_excel('data/meal_order_detail.xlsx')
detail.head()
detail_group = detail[['order_id', 'counts', 'amounts']].groupby(by='order_id') # 分组操作
detail_group.agg('mean').head(3) # 对分组数据的所有列都执行mean操作
detail_group.agg(['mean', 'sum']).head(3) # 对分组数据的所有列都执行mean和sum操作
detail_group.agg({'counts': ['mean', np.max], 'amounts': 'std'}).head(3) # 对分组数据的不同列执行不同操作
detail_group.agg({'counts': lambda x: sum(x)**2}).head(3) # 将自定义函数放入聚合操作中
创建透视表与交叉表
import pandas as pd
detail = pd.read_excel('data/meal_order_detail.xlsx')
detail.head()
pd.pivot_table(detail[['order_id', 'counts', 'amounts']], index='order_id', aggfunc='sum').head(3)
pd.pivot_table(detail[['order_id', 'dishes_name', 'counts']], index='order_id', columns='dishes_name',aggfunc='sum').head(3)
pd.pivot_table(detail[['order_id', 'dishes_name', 'counts']], index='order_id', columns='dishes_name',values='counts', fill_value=0).head()
pd.crosstab(index=detail['order_id'], columns=detail['dishes_name']).head(3)
pd.crosstab(index=detail['order_id'], columns=detail['dishes_name'], values=detail['counts'], aggfunc='sum').fillna(0).head(3)
Matplotlib
python
import numpy as np
import matplotlib.pyplot as plt
import matplotlib
x = np.arange(0, 1.1, 0.1)
print(x)
plt.figure() # 第一环节,创建画布
plt.plot(x, x**2) # 第二环节,绘制图形
plt.plot(x, x**4)
plt.show() # 第三环节,显示图形
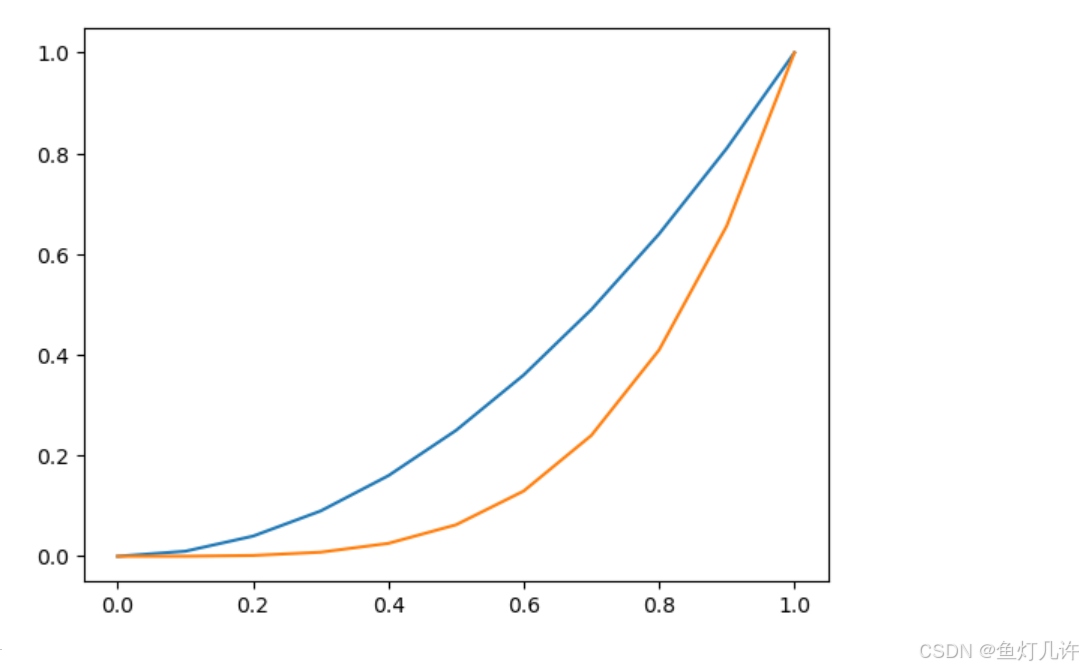
python
plt.figure
x = np.arange(0, 1.1, 0.1)
print(x)
plt.figure() # 第一环节,创建画布
plt.plot(x, x**2) # 第二环节,绘制图形
plt.plot(x, x**4)
plt.xlim(0, 1)
plt.ylim(0, 1)
plt.title('lines')
plt.xlabel('x')
plt.ylabel('y')
plt.legend(['y=x^2', 'y=x^4'])
plt.savefig('tmp/examplt.png')
plt.show() # 第三环节,显示图形
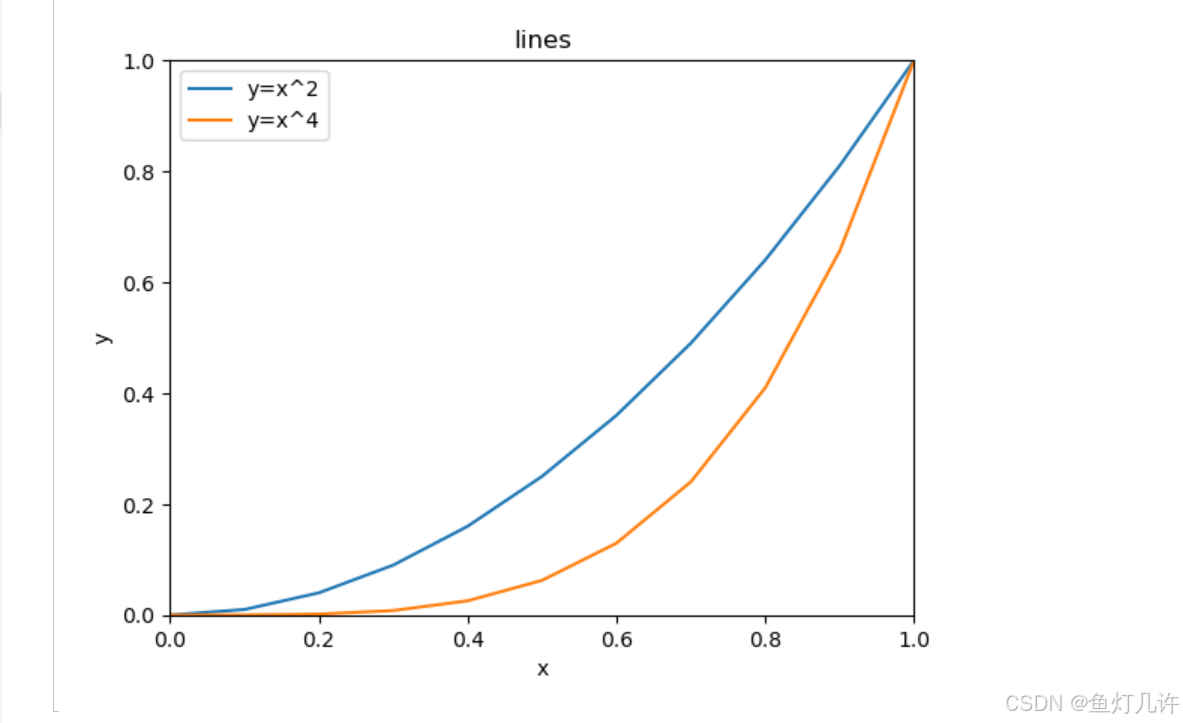
python
import numpy as np
import matplotlib.pyplot as plt
data = np.load('国民经济核算季度数据.npz', allow_pickle=True)
columns = data['columns']
values = data['values']
print(columns)
print(values)
data['values'].shape
# 绘制散点图
plt.figure(figsize=(8, 6))
plt.rcParams['font.sans-serif'] = 'SimHei' # 设置中文显示
plt.rcParams['axes.unicode_minus'] = False
plt.scatter(values[:, 1], values[:, 3], marker='o')
plt.scatter(values[:, 1], values[:, 4], marker='*')
plt.scatter(values[:, 1], values[:, 5], marker='D')
plt.xticks(range(0, 70, 4), values[range(0, 70, 4), 1], rotation=45)
plt.legend(['第一产业生产总值', '第二产业生产总值', '第三产业生产总值'])
plt.title('2000-2017年各产业生产总值散点图')
plt.ylabel('生产总值(亿元)')
plt.savefig('tmp/2000-2017年各产业生产总值散点图.png')
plt.show()
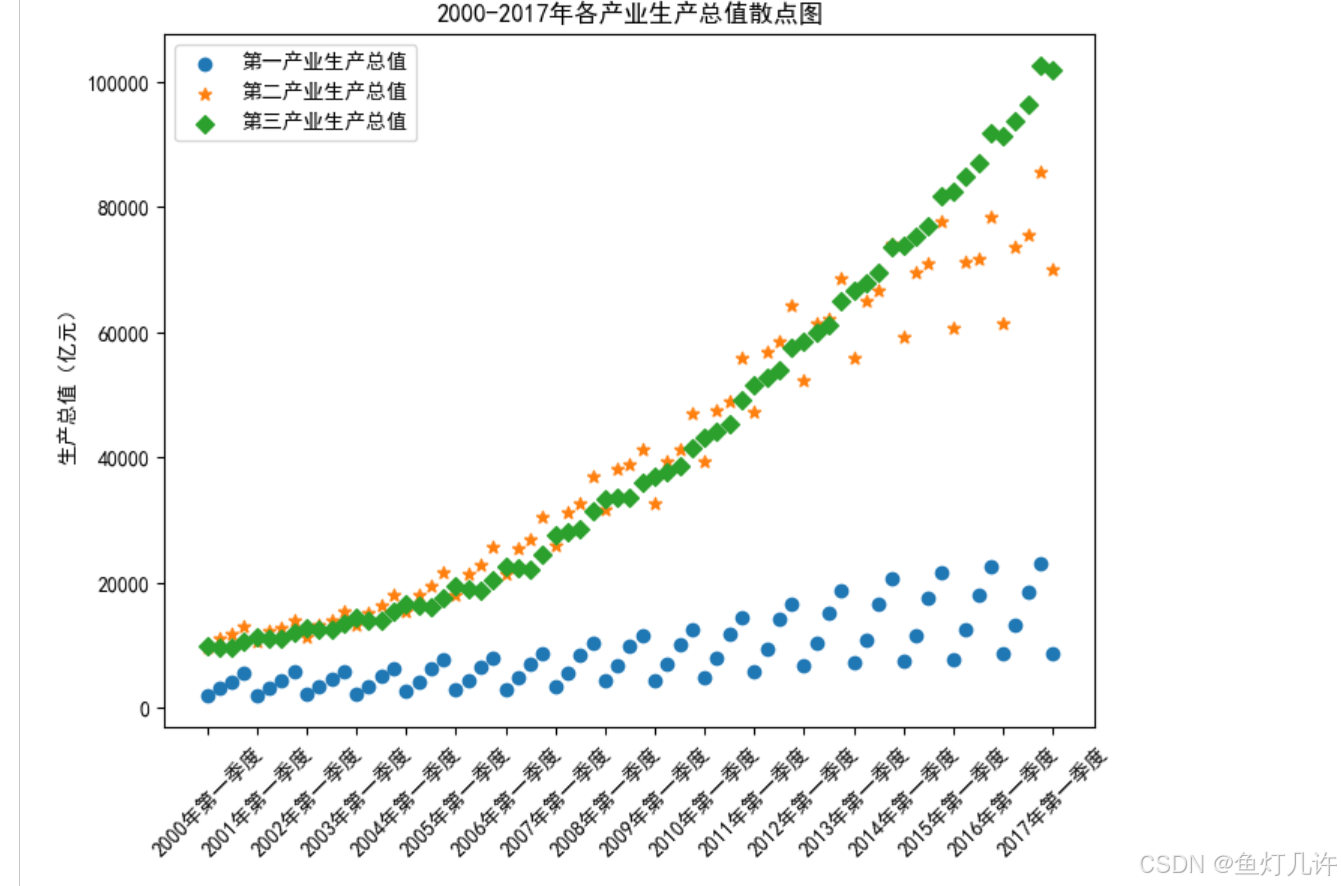
python
# 绘制折线图
plt.figure(figsize=(8, 6))
plt.rcParams['font.sans-serif'] = 'SimHei' # 设置中文显示
plt.rcParams['axes.unicode_minus'] = False
plt.plot(values[:, 1], values[:, 3], linestyle='solid')
plt.plot(values[:, 1], values[:, 4], marker='*')
plt.plot(values[:, 1], values[:, 5], marker='D')
plt.xticks(range(0, 70, 4), values[range(0, 70, 4), 1], rotation=45)
plt.legend(['第一产业生产总值', '第二产业生产总值', '第三产业生产总值'])
plt.title('2000-2017年各产业生产总值折线图')
plt.ylabel('生产总值(亿元)')
plt.savefig('tmp/2000-2017年各产业生产总值折线图.png')
plt.show()
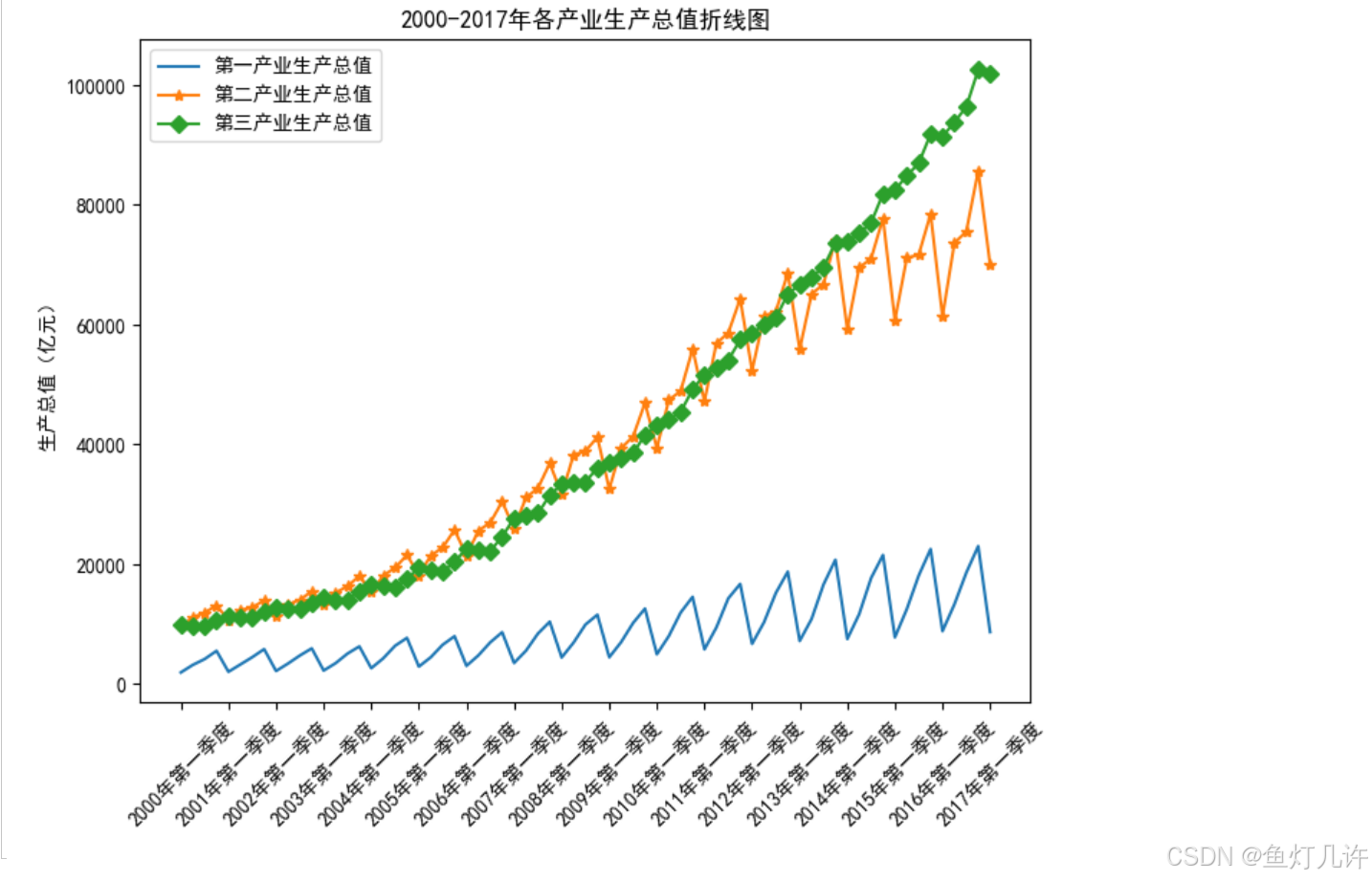
python
# 绘制直方图
plt.figure(figsize=(8, 6))
plt.rcParams['font.sans-serif'] = 'SimHei' # 设置中文显示
plt.rcParams['axes.unicode_minus'] = False
plt.title('2017年第一季度各产业生产总值直方图')
plt.ylabel('生产总值(亿元)')
plt.bar(columns[3:6], values[-1, 3:6])
my_height = values[-1, 3:6]
for i in range(len(my_height)):
plt.text(i, my_height[i]+1000, my_height[i], va='bottom', ha='center')
plt.show()
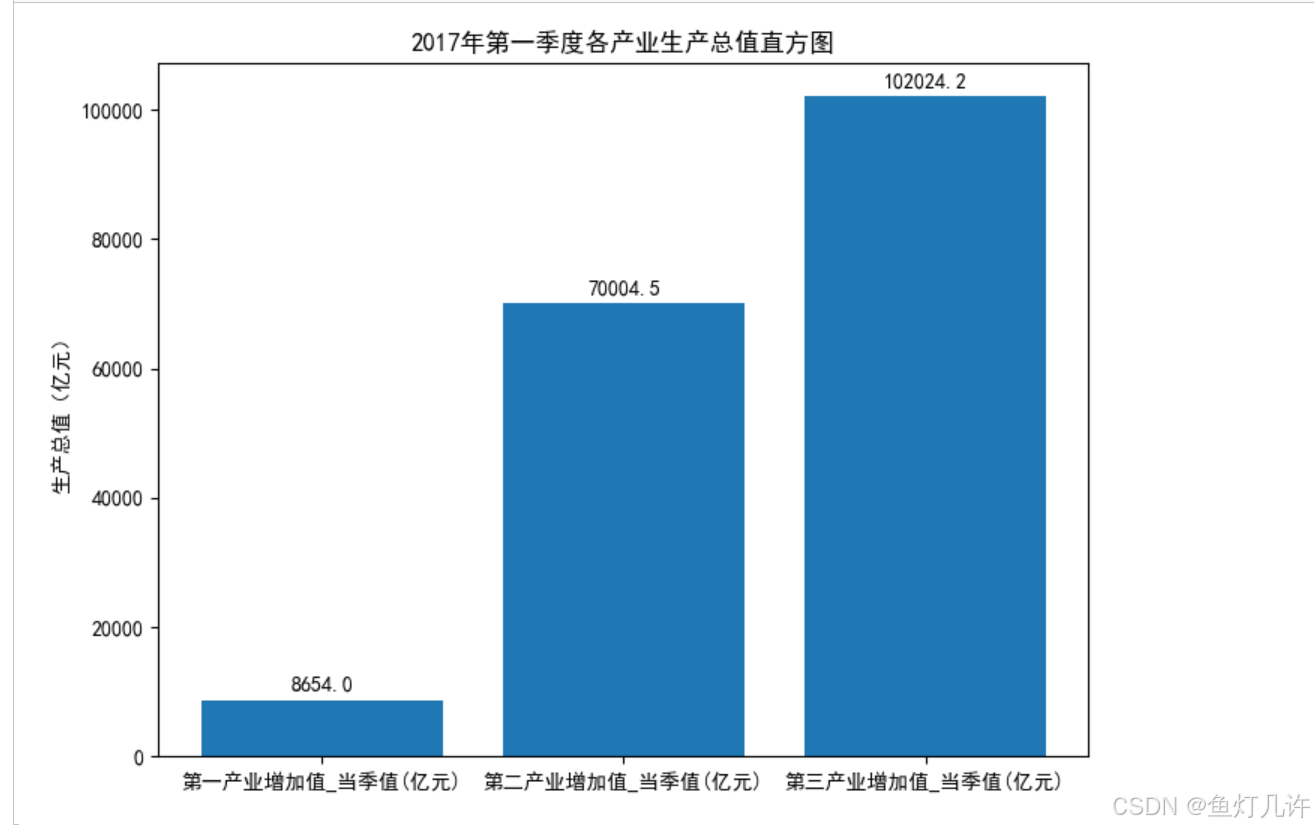
python
# 绘制饼图
plt.figure(figsize=(6, 6))
plt.rcParams['font.sans-serif'] = 'SimHei' # 设置中文显示
plt.rcParams['axes.unicode_minus'] = False
labels = ['第一产业', '第二产业', '第三产业']
plt.pie(values[-1, 3:6], explode=[0.01, 0.01, 0.01], labels=labels, autopct='%1.1f%%')
plt.title('2017年第一季度各产业生产总值饼图')
plt.show()
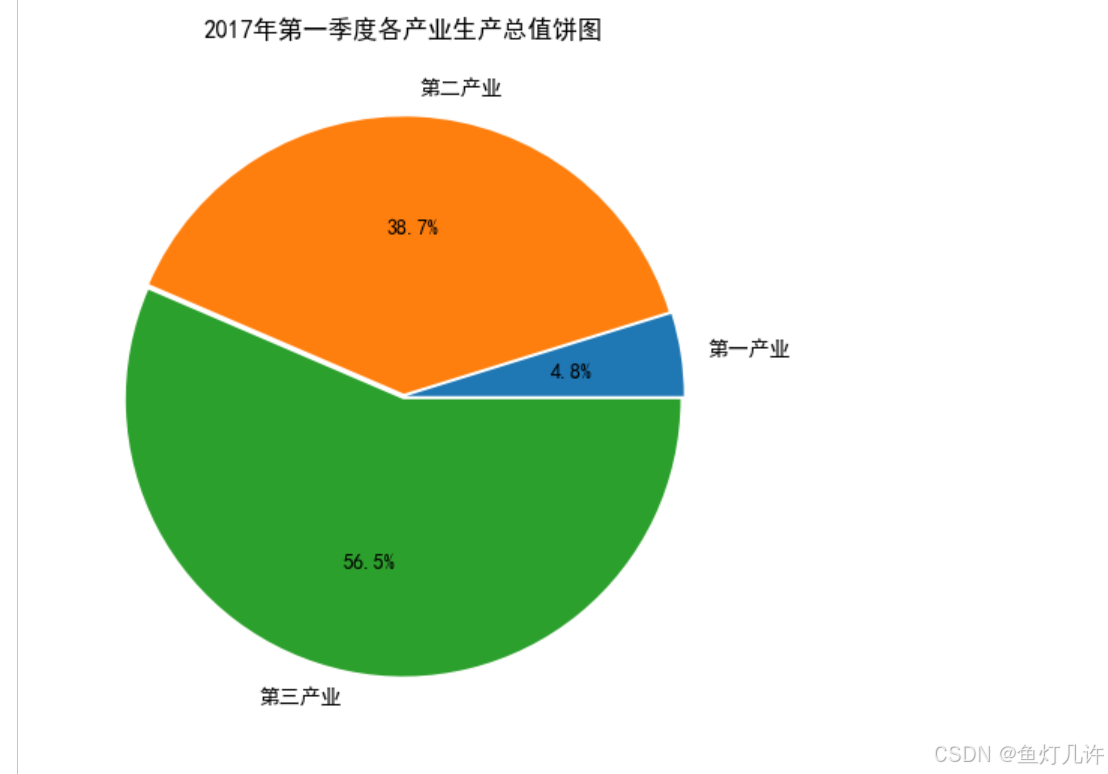
python
# 绘制箱线图
plt.figure(figsize=(6, 6))
plt.rcParams['font.sans-serif'] = 'SimHei' # 设置中文显示
plt.rcParams['axes.unicode_minus'] = False
labels = ['第一产业', '第二产业', '第三产业']
plt.boxplot(values[:, 3:6], notch=True, labels=labels)
plt.show()
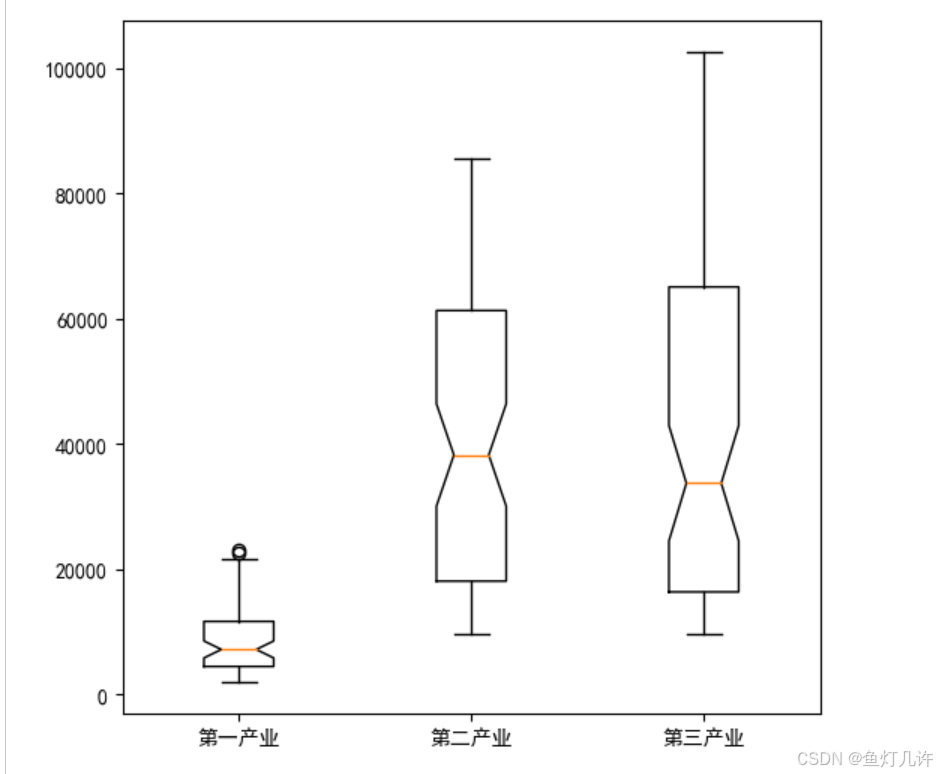
python
plt.figure(figsize=(6, 6))
plt.rcParams['font.sans-serif'] = 'SimHei' # 设置中文显示
plt.rcParams['axes.unicode_minus'] = False
labels = ['第一产业', '第二产业', '第三产业']
plt.boxplot(values[:, 3], notch=True)
plt.show()
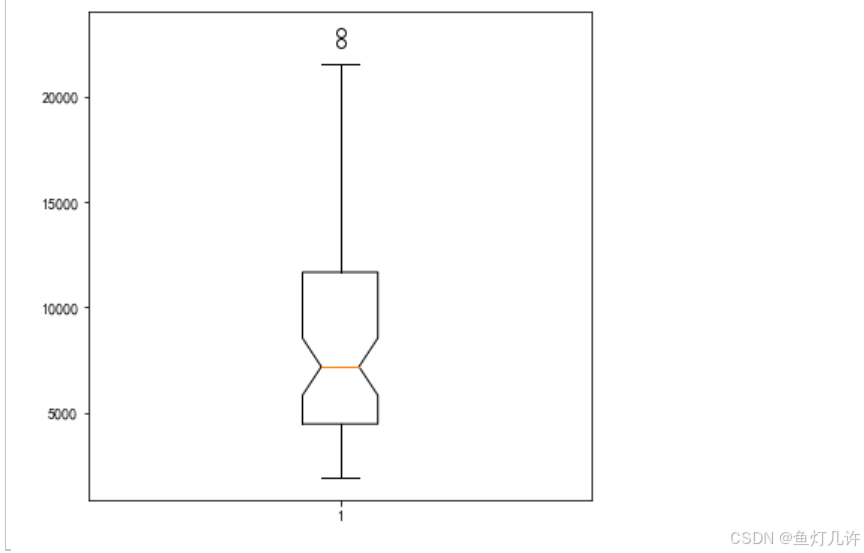
Requests库
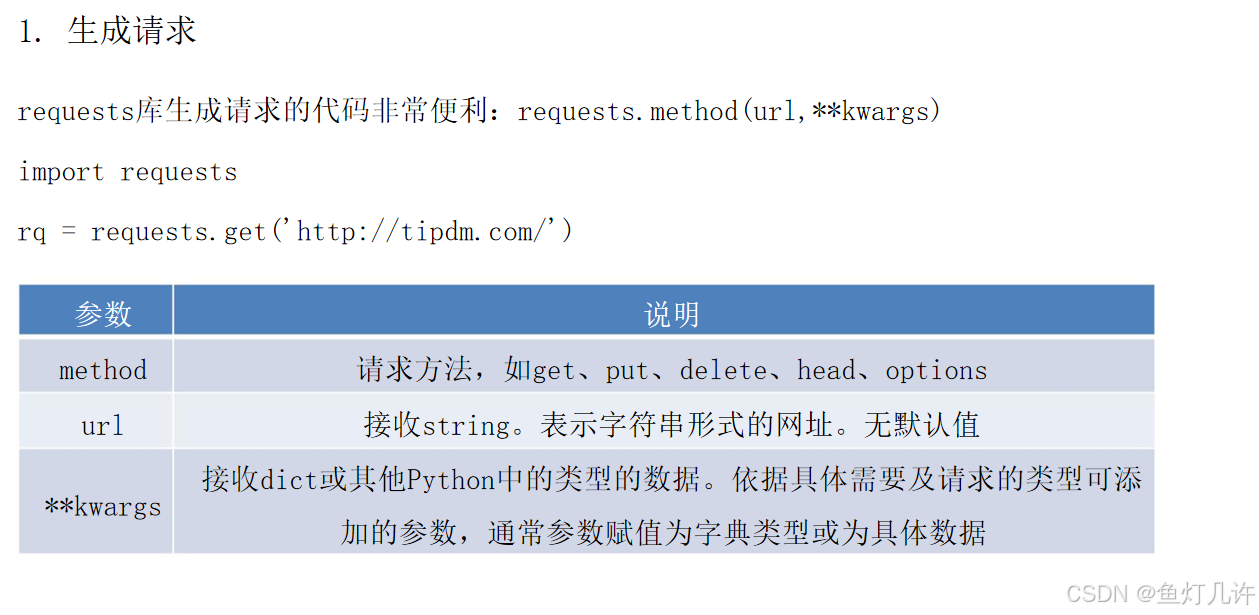
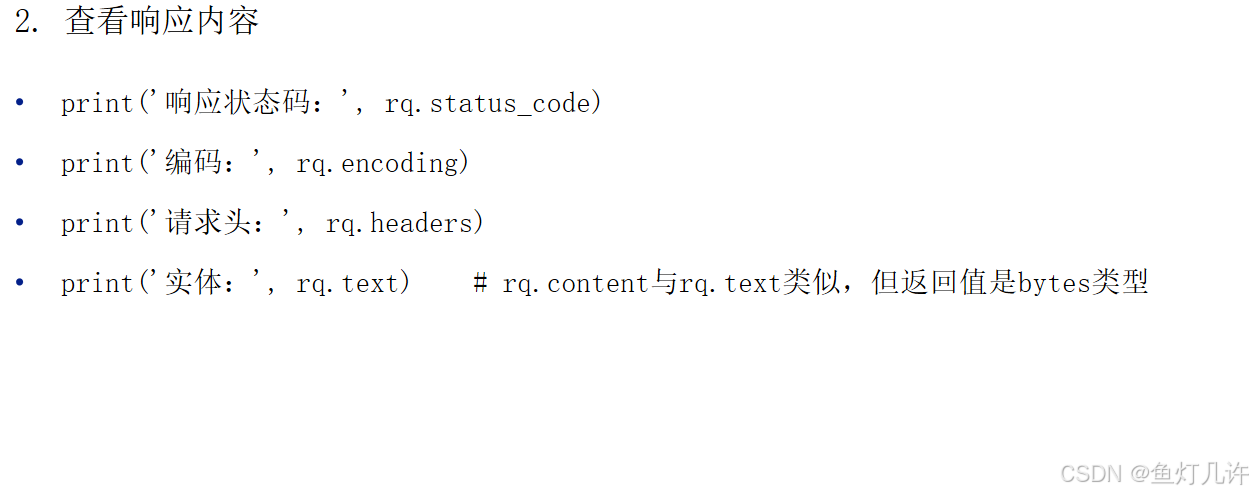
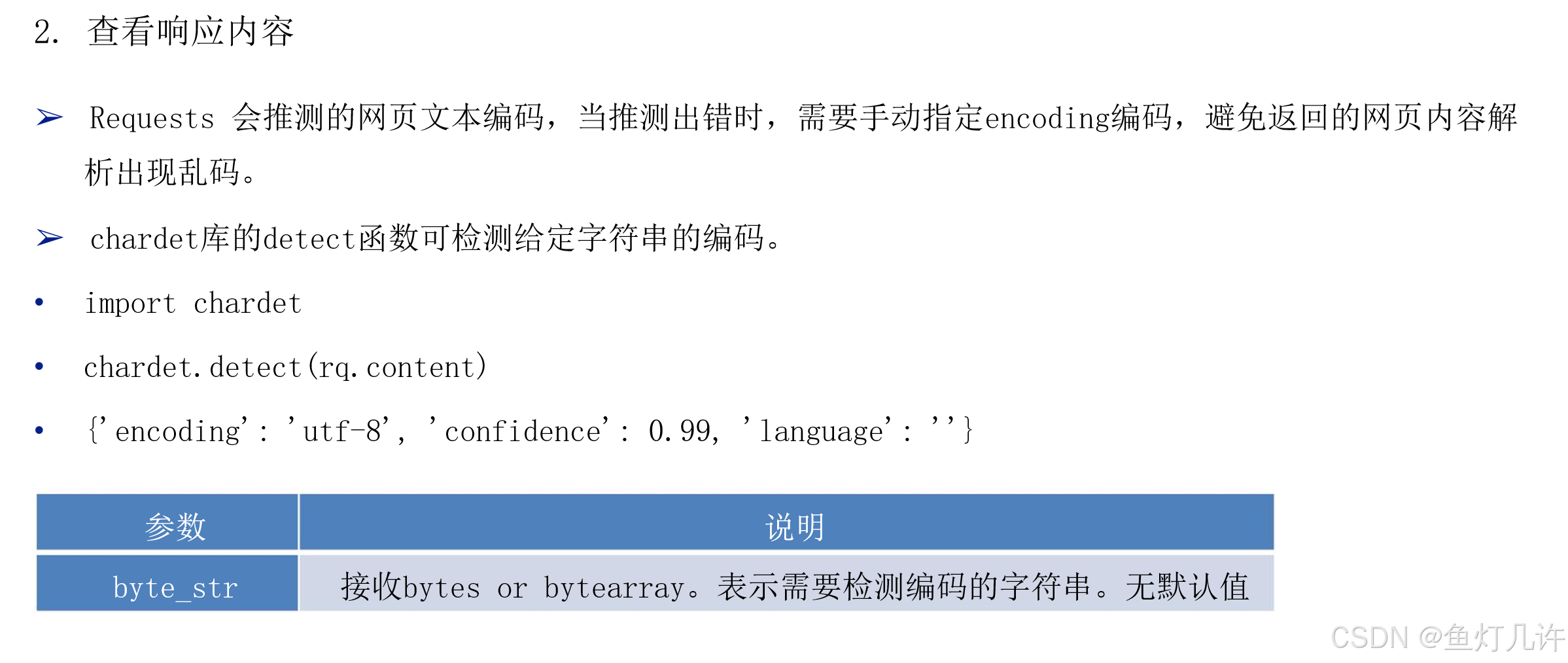
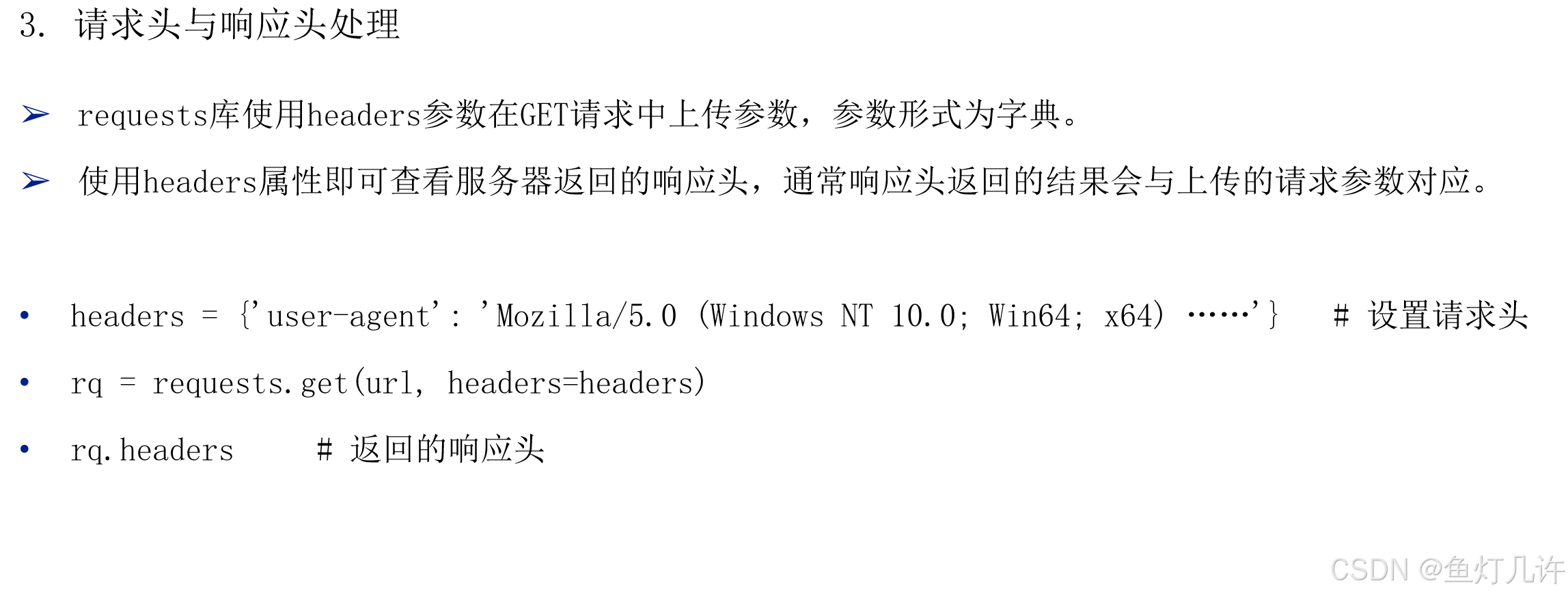
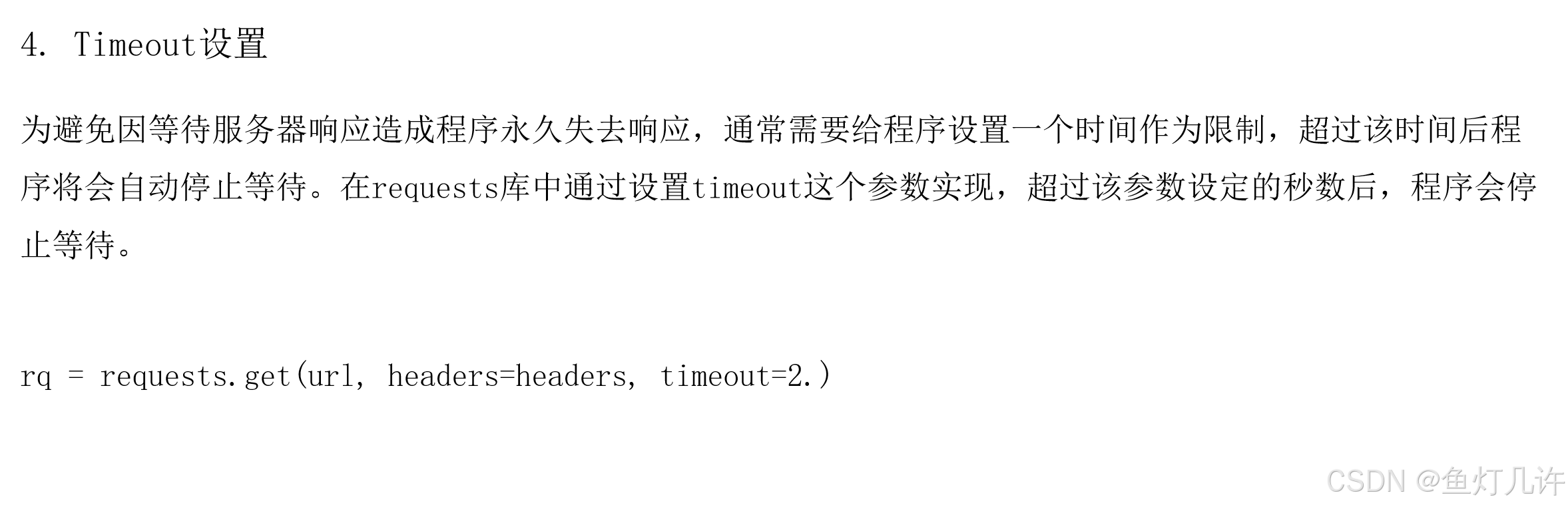
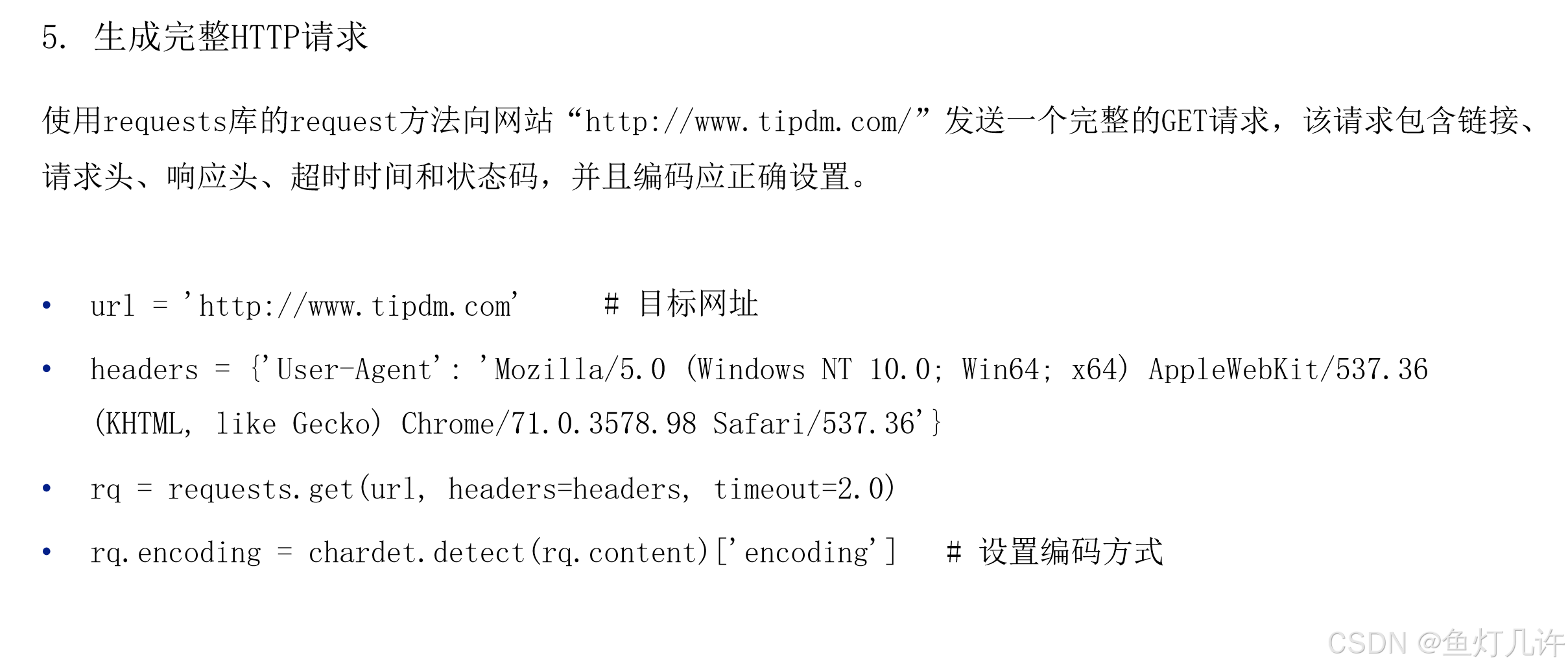