一、定义
UserWarning: Provided key output for dynamic axes is not a valid input/output name warnings.warn(
案例
实体识别bert 案例
转transformers 模型到onnx 接口解读
二、实现
https://huggingface.co/docs/transformers/main_classes/onnx#transformers.onnx.FeaturesManager
UserWarning: Provided key output for dynamic axes is not a valid input/output name warnings.warn(
代码:
python
with torch.no_grad():
symbolic_names = {0: 'batch_size', 1: 'max_seq_len'}
torch.onnx.export(model,
(inputs["input_ids"], inputs["token_type_ids"], inputs["attention_mask"]),
"./saves/bertclassify.onnx",
opset_version=14,
input_names=["input_ids", "token_type_ids", "attention_mask"],
output_names=["logits"],
dynamic_axes = {'input_ids': symbolic_names,
'attention_mask': symbolic_names,
'token_type_ids': symbolic_names,
'logits': symbolic_names
}
)
改正后:原因: input_names 名字顺序与模型定义不一致导致。为了避免错误产生,应该标准化。如下2所示。
python
tokenizer = AutoTokenizer.from_pretrained(model_path)
model = BertForSequenceClassification.from_pretrained(model_path)
model.eval()
onnx_config = FeaturesManager._SUPPORTED_MODEL_TYPE['bert']['sequence-classification']("./saves")
dummy_inputs = onnx_config.generate_dummy_inputs(tokenizer, framework='pt')
from itertools import chain
with torch.no_grad():
symbolic_names = {0: 'batch_size', 1: 'max_seq_len'}
torch.onnx.export(model,
(inputs["input_ids"],inputs["attention_mask"], inputs["token_type_ids"]),
"./saves/bertclassify.onnx",
opset_version=14,
input_names=["input_ids", "attention_mask", "token_type_ids"],
output_names=["logits"],
dynamic_axes = {
name: axes for name, axes in chain(onnx_config.inputs.items(), onnx_config.outputs.items())
}
)
# #验证是否成功
import onnx
onnx_model=onnx.load("./saves/bertclassify.onnx")
onnx.checker.check_model(onnx_model)
print("无报错,转换成功")
# #推理
import onnxruntime
ort_session=onnxruntime.InferenceSession("./saves/bertclassify.onnx", providers=['CPUExecutionProvider']) #加载模型
ort_input={"input_ids":inputs["input_ids"].cpu().numpy(),"token_type_ids":inputs["token_type_ids"].cpu().numpy(),
"attention_mask":inputs["attention_mask"].cpu().numpy()}
output_on = ort_session.run(["logits"], ort_input)[0] #推理
print(output_org.detach().numpy())
print(output_on)
assert np.allclose(output_org.detach().numpy(), output_on, 10-5) #无报错
标准化:
python
output_onnx_path = "./saves/bertclassify.onnx"
from itertools import chain
dummy_inputs = onnx_config.generate_dummy_inputs(tokenizer, framework='pt')
torch.onnx.export(
model,
(dummy_inputs,),
f=output_onnx_path,
input_names=list(onnx_config.inputs.keys()),
output_names=list(onnx_config.outputs.keys()),
dynamic_axes={
name: axes for name, axes in chain(onnx_config.inputs.items(), onnx_config.outputs.items())
},
do_constant_folding=True,
opset_version=14,
)
全部:
python
import torch
devices=torch.device("cpu")
from transformers.onnx.features import FeaturesManager
import torch
from transformers import AutoTokenizer, BertForSequenceClassification
import numpy as np
model_path = "./saves"
tokenizer = AutoTokenizer.from_pretrained(model_path)
model = BertForSequenceClassification.from_pretrained(model_path)
words = ["你叫什么名字"]
inputs = tokenizer(words, return_tensors='pt', padding=True)
model.eval()
onnx_config = FeaturesManager._SUPPORTED_MODEL_TYPE['bert']['sequence-classification']("./saves")
dummy_inputs = onnx_config.generate_dummy_inputs(tokenizer, framework='pt')
from itertools import chain
output_org = model(**inputs).logits
torch.onnx.export(
model,
(dummy_inputs,),
f=output_onnx_path,
input_names=list(onnx_config.inputs.keys()),
output_names=list(onnx_config.outputs.keys()),
dynamic_axes={
name: axes for name, axes in chain(onnx_config.inputs.items(), onnx_config.outputs.items())
},
do_constant_folding=True,
opset_version=14,
)
# #验证是否成功
import onnx
onnx_model=onnx.load("./saves/bertclassify.onnx")
onnx.checker.check_model(onnx_model)
print("无报错,转换成功")
# #推理
import onnxruntime
ort_session=onnxruntime.InferenceSession("./saves/bertclassify.onnx", providers=['CPUExecutionProvider']) #加载模型
ort_input={"input_ids":inputs["input_ids"].cpu().numpy(),"token_type_ids":inputs["token_type_ids"].cpu().numpy(),
"attention_mask":inputs["attention_mask"].cpu().numpy()}
output_on = ort_session.run(["logits"], ort_input)[0] #推理
print(output_org.detach().numpy())
print(output_on)
assert np.allclose(output_org.detach().numpy(), output_on, 10-5) #无报错
无任何警告产生
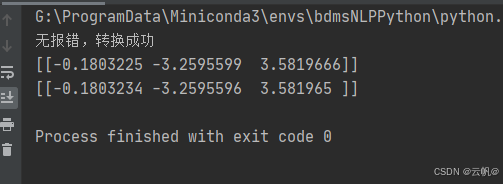
实体识别案例
python
import onnxruntime
from itertools import chain
from transformers.onnx.features import FeaturesManager
config = ner_config
tokenizer = ner_tokenizer
model = ner_model
output_onnx_path = "bert-ner.onnx"
onnx_config = FeaturesManager._SUPPORTED_MODEL_TYPE['bert']['sequence-classification'](config)
dummy_inputs = onnx_config.generate_dummy_inputs(tokenizer, framework='pt')
torch.onnx.export(
model,
(dummy_inputs,),
f=output_onnx_path,
input_names=list(onnx_config.inputs.keys()),
output_names=list(onnx_config.outputs.keys()),
dynamic_axes={
name: axes for name, axes in chain(onnx_config.inputs.items(), onnx_config.outputs.items())
},
do_constant_folding=True,
opset_version=onnx_config.default_onnx_opset, #默认,报错改为14
)