NSGA-II(非支配排序遗传算法II)详解与实现
1. 算法简介
NSGA-II(Non-dominated Sorting Genetic Algorithm II)是一种高效的多目标优化算法,由Deb等人在2002年提出。它主要解决多个目标之间相互冲突的优化问题。
1.1 核心特点
-
快速非支配排序
- 时间复杂度:O(MN²)
- M为目标数量,N为种群大小
- 比NSGA的O(MN³)更高效
-
拥挤度距离
- 保持种群多样性
- 不需要用户定义分享参数
- 避免使用小生境技术
-
精英策略
- 保留父代和子代中的优秀个体
- 确保最优解不会丢失
- 加快收敛速度
1.2 基本概念
-
帕累托支配关系
对于最小化问题,解x支配解y,需满足:
- ∀ i : f i ( x ) ≤ f i ( y ) \forall i: f_i(x) \leq f_i(y) ∀i:fi(x)≤fi(y)
- ∃ j : f j ( x ) < f j ( y ) \exists j: f_j(x) < f_j(y) ∃j:fj(x)<fj(y)
-
帕累托前沿
- 由非支配解构成
- 表示目标之间的最优折中
- 为决策者提供选择空间
2. 算法流程
2.1 主要步骤
- 初始化种群P(t)
- 生成子代Q(t)
- 合并R(t) = P(t) ∪ Q(t)
- 对R(t)进行非支配排序
- 根据排序和拥挤度选择新种群P(t+1)
- 重复步骤2-5直到满足终止条件
2.2 关键组件
- 快速非支配排序
python
def fast_non_dominated_sort(population, objectives):
"""
快速非支配排序
返回:各个等级的解集合
"""
n = len(population)
domination_count = np.zeros(n) # 被支配次数
dominated_solutions = [[] for _ in range(n)] # 支配的解
fronts = [[]] # 非支配等级
# 计算支配关系
for i in range(n):
for j in range(i + 1, n):
if dominates(objectives[i], objectives[j]):
dominated_solutions[i].append(j)
domination_count[j] += 1
elif dominates(objectives[j], objectives[i]):
dominated_solutions[j].append(i)
domination_count[i] += 1
# 找出第一个非支配前沿
for i in range(n):
if domination_count[i] == 0:
fronts[0].append(i)
# 生成其他等级的前沿
i = 0
while fronts[i]:
next_front = []
for j in fronts[i]:
for k in dominated_solutions[j]:
domination_count[k] -= 1
if domination_count[k] == 0:
next_front.append(k)
i += 1
fronts.append(next_front)
return fronts[:-1] # 去掉最后一个空集
- 拥挤度距离计算
python
def crowding_distance(objectives, front):
"""
计算一个前沿中各个解的拥挤度距离
"""
n = len(front)
if n <= 2:
return [float('inf')] * n
distances = [0] * n
m = objectives.shape[1] # 目标数量
for i in range(m):
# 对每个目标进行排序
sorted_idx = sorted(range(n), key=lambda k: objectives[front[k], i])
# 端点设为无穷大
distances[sorted_idx[0]] = float('inf')
distances[sorted_idx[-1]] = float('inf')
# 计算中间点的距离
obj_range = objectives[front[sorted_idx[-1]], i] - objectives[front[sorted_idx[0]], i]
if obj_range == 0:
continue
for j in range(1, n-1):
distances[sorted_idx[j]] += (
objectives[front[sorted_idx[j+1]], i] -
objectives[front[sorted_idx[j-1]], i]
) / obj_range
return distances
3. 完整实现
python
import numpy as np
from typing import List, Tuple, Union
class NSGA2:
def __init__(
self,
pop_size: int = 100,
n_generations: int = 100,
n_objectives: int = 2,
n_variables: int = 30,
mutation_rate: float = 0.01,
crossover_rate: float = 0.9,
variable_bounds: Union[List[Tuple[float, float]], None] = None
):
self.pop_size = pop_size
self.n_generations = n_generations
self.n_objectives = n_objectives
self.n_variables = n_variables
self.mutation_rate = mutation_rate
self.crossover_rate = crossover_rate
# 如果没有指定变量范围,默认为[0,1]
if variable_bounds is None:
self.variable_bounds = [(0, 1)] * n_variables
else:
self.variable_bounds = variable_bounds
def create_offspring(self, population: np.ndarray) -> np.ndarray:
"""生成子代"""
offspring = np.zeros_like(population)
for i in range(0, self.pop_size, 2):
# 选择父代
parent1_idx = self.tournament_selection(population)
parent2_idx = self.tournament_selection(population)
# 交叉
if np.random.random() < self.crossover_rate:
child1, child2 = self.simulated_binary_crossover(
population[parent1_idx],
population[parent2_idx]
)
else:
child1 = population[parent1_idx].copy()
child2 = population[parent2_idx].copy()
# 变异
child1 = self.polynomial_mutation(child1)
child2 = self.polynomial_mutation(child2)
offspring[i] = child1
offspring[i+1] = child2
return offspring
def tournament_selection(self, population: np.ndarray, tournament_size: int = 2) -> int:
"""锦标赛选择"""
idx = np.random.randint(0, self.pop_size, tournament_size)
return idx[0] # 简化版本,实际应该比较个体的非支配等级和拥挤度
def simulated_binary_crossover(self, parent1: np.ndarray, parent2: np.ndarray,
eta: float = 20) -> Tuple[np.ndarray, np.ndarray]:
"""模拟二进制交叉"""
child1 = np.zeros_like(parent1)
child2 = np.zeros_like(parent2)
for i in range(self.n_variables):
if np.random.random() < 0.5:
child1[i] = parent1[i]
child2[i] = parent2[i]
continue
# 模拟二进制交叉
beta = self._get_beta(eta)
child1[i] = 0.5 * ((1 + beta) * parent1[i] + (1 - beta) * parent2[i])
child2[i] = 0.5 * ((1 - beta) * parent1[i] + (1 + beta) * parent2[i])
# 边界处理
lower, upper = self.variable_bounds[i]
child1[i] = np.clip(child1[i], lower, upper)
child2[i] = np.clip(child2[i], lower, upper)
return child1, child2
def _get_beta(self, eta: float) -> float:
"""获取交叉参数beta"""
u = np.random.random()
if u <= 0.5:
return (2 * u) ** (1 / (eta + 1))
return (1 / (2 * (1 - u))) ** (1 / (eta + 1))
def polynomial_mutation(self, individual: np.ndarray, eta: float = 20) -> np.ndarray:
"""多项式变异"""
child = individual.copy()
for i in range(self.n_variables):
if np.random.random() > self.mutation_rate:
continue
lower, upper = self.variable_bounds[i]
delta = upper - lower
# 多项式变异
r = np.random.random()
if r < 0.5:
delta_l = (2 * r) ** (1 / (eta + 1)) - 1
else:
delta_l = 1 - (2 * (1 - r)) ** (1 / (eta + 1))
child[i] += delta_l * delta
child[i] = np.clip(child[i], lower, upper)
return child
def calculate_objectives(self, population: np.ndarray) -> np.ndarray:
"""计算目标函数值"""
return np.array([zdt1(x) for x in population])
def select_by_crowding(self, front: List[int], crowding_dist: List[float],
n_select: int) -> List[int]:
"""根据拥挤度距离选择个体"""
sorted_idx = sorted(range(len(front)),
key=lambda k: crowding_dist[k],
reverse=True)
return [front[i] for i in sorted_idx[:n_select]]
def evolve(self, initial_population: np.ndarray) -> np.ndarray:
"""执行进化过程"""
population = initial_population
for gen in range(self.n_generations):
# 生成子代
offspring = self.create_offspring(population)
# 合并父代和子代
combined_pop = np.vstack([population, offspring])
# 计算目标值
objectives = self.calculate_objectives(combined_pop)
# 非支配排序
fronts = fast_non_dominated_sort(combined_pop, objectives)
# 选择新种群
new_population = []
for front in fronts:
if len(new_population) + len(front) <= self.pop_size:
new_population.extend(front)
else:
# 计算拥挤度距离
crowding_dist = crowding_distance(objectives, front)
# 根据拥挤度选择剩余个体
remaining = self.pop_size - len(new_population)
selected = self.select_by_crowding(front, crowding_dist, remaining)
new_population.extend(selected)
break
population = combined_pop[new_population]
if gen % 10 == 0:
print(f"Generation {gen}: Population size = {len(population)}")
return population
def dominates(obj1: np.ndarray, obj2: np.ndarray) -> bool:
"""判断obj1是否支配obj2"""
return np.all(obj1 <= obj2) and np.any(obj1 < obj2)
def fast_non_dominated_sort(population: np.ndarray, objectives: np.ndarray) -> List[List[int]]:
"""快速非支配排序"""
n = len(population)
domination_count = np.zeros(n)
dominated_solutions = [[] for _ in range(n)]
fronts = [[]]
for i in range(n):
for j in range(i + 1, n):
if dominates(objectives[i], objectives[j]):
dominated_solutions[i].append(j)
domination_count[j] += 1
elif dominates(objectives[j], objectives[i]):
dominated_solutions[j].append(i)
domination_count[i] += 1
for i in range(n):
if domination_count[i] == 0:
fronts[0].append(i)
i = 0
while fronts[i]:
next_front = []
for j in fronts[i]:
for k in dominated_solutions[j]:
domination_count[k] -= 1
if domination_count[k] == 0:
next_front.append(k)
i += 1
fronts.append(next_front)
return fronts[:-1]
def crowding_distance(objectives: np.ndarray, front: List[int]) -> List[float]:
"""计算拥挤度距离"""
n = len(front)
if n <= 2:
return [float('inf')] * n
distances = [0] * n
m = objectives.shape[1]
for i in range(m):
sorted_idx = sorted(range(n), key=lambda k: objectives[front[k], i])
distances[sorted_idx[0]] = float('inf')
distances[sorted_idx[-1]] = float('inf')
obj_range = objectives[front[sorted_idx[-1]], i] - objectives[front[sorted_idx[0]], i]
if obj_range == 0:
continue
for j in range(1, n-1):
distances[sorted_idx[j]] += (
objectives[front[sorted_idx[j+1]], i] -
objectives[front[sorted_idx[j-1]], i]
) / obj_range
return distances
4. 应用示例
让我们以一个经典的多目标优化问题ZDT1为例:
python
def zdt1(x: np.ndarray) -> np.ndarray:
"""ZDT1测试函数"""
f1 = x[0]
g = 1 + 9 * np.mean(x[1:])
f2 = g * (1 - np.sqrt(f1/g))
return np.array([f1, f2])
# 运行示例
if __name__ == "__main__":
nsga2 = NSGA2(pop_size=100, n_generations=100, n_variables=30)
initial_pop = np.random.random((100, 30))
final_pop = nsga2.evolve(initial_pop)
# 计算最终种群的目标值
final_objectives = nsga2.calculate_objectives(final_pop)
# 绘制帕累托前沿
import matplotlib.pyplot as plt
plt.figure(figsize=(10, 6))
plt.scatter(final_objectives[:, 0], final_objectives[:, 1])
plt.xlabel('f1')
plt.ylabel('f2')
plt.title('Pareto Front')
plt.grid(True)
plt.show()
5. 完整实现与解析
让我们逐块解析NSGA-II的完整实现,理解每个部分的作用和原理。
5.1 类的初始化
python
def __init__(
self,
pop_size: int = 100,
n_generations: int = 100,
n_objectives: int = 2,
n_variables: int = 30,
mutation_rate: float = 0.01,
crossover_rate: float = 0.9,
variable_bounds: Union[List[Tuple[float, float]], None] = None
):
这部分就像是在准备一场比赛:
pop_size
:决定有多少选手参加(种群大小)n_generations
:比赛要进行多少轮(迭代次数)n_objectives
:评判标准有几个(目标函数数量)n_variables
:每个选手有多少个可调整的参数mutation_rate
:选手自我调整的概率crossover_rate
:选手互相学习的概率variable_bounds
:每个参数的取值范围
5.2 生成子代
python
def create_offspring(self, population: np.ndarray) -> np.ndarray:
"""生成子代"""
offspring = np.zeros_like(population)
for i in range(0, self.pop_size, 2):
# 选择父代
parent1_idx = self.tournament_selection(population)
parent2_idx = self.tournament_selection(population)
# 交叉和变异
if np.random.random() < self.crossover_rate:
child1, child2 = self.simulated_binary_crossover(
population[parent1_idx],
population[parent2_idx]
)
else:
child1 = population[parent1_idx].copy()
child2 = population[parent2_idx].copy()
这就像是在进行一场配对比赛:
- 每次选两个选手(父代)
- 让他们互相学习(交叉)
- 产生两个新选手(子代)
- 新选手可能会有一些自己的创新(变异)
5.3 模拟二进制交叉
python
def simulated_binary_crossover(self, parent1: np.ndarray, parent2: np.ndarray,
eta: float = 20) -> Tuple[np.ndarray, np.ndarray]:
这就像两个选手互相学习的过程:
eta
参数控制学习程度:- 值越大,子代越像父代
- 值越小,变化越大
- 每个参数都有机会被交换或混合
- 确保新的参数不会超出范围
举个例子:
- 父代1的某个参数是0.3
- 父代2的某个参数是0.7
- 交叉后可能得到0.4和0.6两个新值
5.4 多项式变异
python
def polynomial_mutation(self, individual: np.ndarray, eta: float = 20) -> np.ndarray:
这就像选手在尝试新东西:
- 每个参数都有小概率发生改变
- 改变的幅度由
eta
控制:- 值越大,改变越小
- 值越小,改变越大
- 确保改变后的值仍在允许范围内
比如:
- 原来的参数值是0.5
- 变异后可能变成0.48或0.52
5.5 非支配排序
python
def fast_non_dominated_sort(population: np.ndarray, objectives: np.ndarray):
这就像对选手进行分级:
- 第一级:没有人比他们更好的选手
- 第二级:除去第一级后,没有人比他们更好的选手
- 以此类推...
举例:
- A选手:速度快,耐力好 → 第一级
- B选手:速度快,耐力一般 → 第二级
- C选手:速度一般,耐力好 → 第二级
- D选手:速度慢,耐力差 → 第三级
5.6 拥挤度距离
python
def crowding_distance(objectives: np.ndarray, front: List[int]):
这就像测量选手之间的独特性:
- 在同一级别中
- 看看每个选手与周围选手的不同程度
- 不同程度越大,得分越高
比如在第一级中:
- A选手:与其他人很不同 → 高分
- B选手:与C选手很相似 → 低分
- C选手:与B选手很相似 → 低分
5.7 进化主循环
python
def evolve(self, initial_population: np.ndarray):
整个过程就像一个循环的比赛:
- 现有选手比赛
- 产生新选手
- 所有选手一起评级
- 选出最好的一批进入下一轮
- 重复这个过程
每一代都会:
- 保留最优秀的选手
- 淘汰表现差的选手
- 产生新的选手
5.8 测试函数
python
def zdt1(x: np.ndarray) -> np.ndarray:
这是一个测试案例,就像是给选手设置的考试:
- 有两个目标:
- 第一个目标:x[0]越小越好
- 第二个目标:一个复杂的计算结果越小越好
- 这两个目标是相互冲突的
- 需要找到多个平衡的解
6. 结果分析
6.1 帕累托前沿
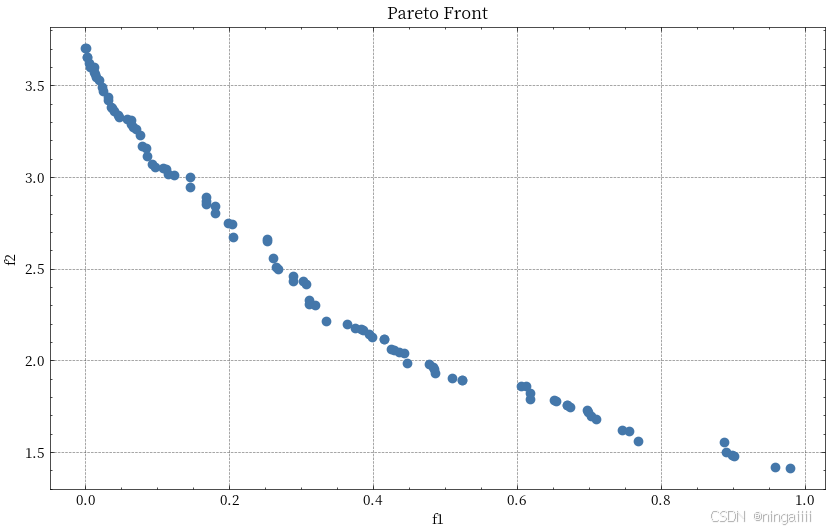
从上图的帕累托前沿我们可以观察到以下特点:
-
前沿形状
- 呈现出平滑的凹形曲线
- f1从0到1分布
- f2从约1.4到3.8分布
- 这是典型的ZDT1测试问题的理论前沿形状
-
解的分布
- 点的分布较为均匀
- 覆盖了整个决策空间
- 没有明显的间隙
- 在两端的解密度略低
-
权衡关系
- 清晰展示了f1和f2之间的此消彼长关系
- 当f1接近0时,f2达到最大值(约3.8)
- 当f1接近1时,f2达到最小值(约1.4)
- 中间区域展示了两个目标的平衡点
-
算法性能表现
-
收敛性:
- 解都落在一条光滑曲线上
- 没有明显的离群点
- 说明算法收敛性良好
-
多样性:
- 解在整个前沿上分布均匀
- 端点解被很好地保留
- 说明拥挤度距离机制效果良好
-
前沿完整性:
- 覆盖了整个帕累托前沿
- 没有明显的空缺区域
- 说明算法的搜索能力强
-
6.2 实际应用意义
这个结果在实际应用中意味着:
-
决策支持
- 为决策者提供了一系列可选的折中方案
- 每个点代表一个不同的权衡方案
- 决策者可以根据实际需求选择合适的解
-
方案选择
- 如果更重视f1(第一个目标),可以选择右侧的解
- 如果更重视f2(第二个目标),可以选择左侧的解
- 如果需要平衡,可以选择中间区域的解
-
解的特点
- 左上角的解:f1较小,f2较大
- 右下角的解:f1较大,f2较小
- 中间的解:两个目标都处于中等水平
总结
NSGA-II算法通过其高效的非支配排序和新颖的拥挤度计算机制,成功解决了多目标优化问题中的三个关键问题:
- 如何引导种群向帕累托前沿收敛
- 如何保持解的多样性
- 如何保留精英个体
这使得NSGA-II成为了多目标优化领域最受欢迎的算法之一。