写在前面
最近,我在学习Transformer模型在图像领域的应用。图像处理任务一直以来都是深度学习领域的重要研究方向,而传统的卷积神经网络已在许多任务中取得了显著的成绩。然而,近年来,Transformer模型由于其在自然语言处理中的成功,逐渐被引入到计算机视觉领域。Vision Transformer(ViT)是应用Transformer架构于图像分类任务的一个重要突破,它证明了Transformer在视觉任务中的潜力。ViT通过将图像分割成若干固定大小的图块,并将每个图块视为一个序列输入到Transformer中进行处理。与传统的卷积神经网络不同,ViT摆脱了卷积操作,完全依赖自注意力机制来捕捉图像中的长距离依赖关系。
本篇文章将深入探讨Vision Transformer的原理、架构以及其在图像分类任务中的表现,并通过代码实现来帮助大家更好地理解其工作方式。
论文地址:https://arxiv.org/pdf/2010.11929
官方代码实现:vision_transformer/vit_jax/models_vit.py
VIT网络结构
Vision Transformer(ViT)是将Transformer架构应用于图像分类任务的一个创新模型。传统上,卷积神经网络(CNN)是图像处理任务的主流方法,而ViT提出了一种完全不同的视角:将图像分割成固定大小的图块,并将这些图块视为一维的序列来输入Transformer模型。ViT模型摒弃了卷积操作,完全依赖于Transformer的自注意力机制来捕捉图像中的长距离依赖。
下面的动态图是从网上找到的,展示也比较形象。
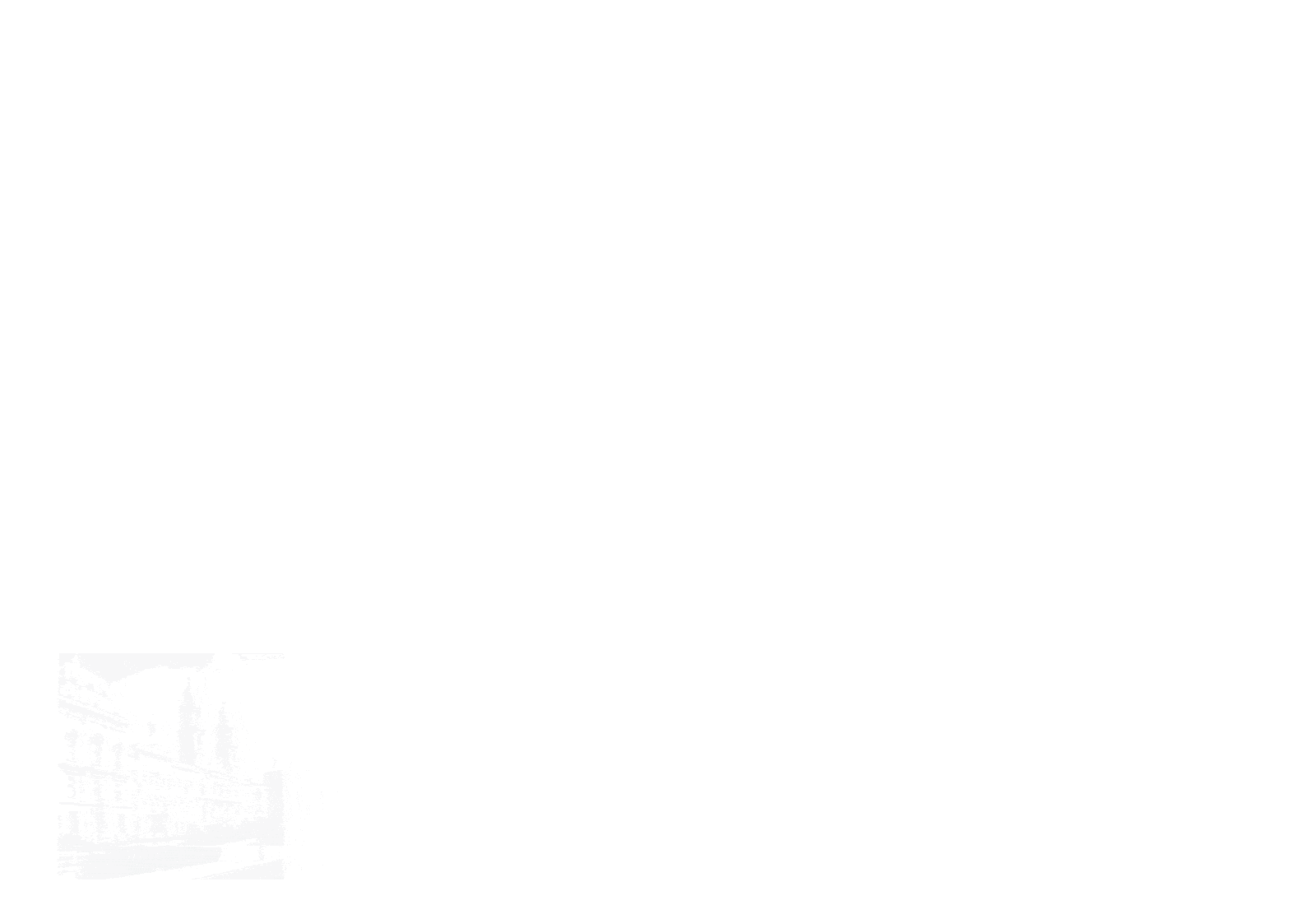
Patch Embedding结构
ViT的输入是一个大小为 H×W×C 的图像,其中 H 和 W 是图像的高和宽,C 是图像的通道数。ViT将图像分割成大小为 P×P 的小块,称为"patches"(图像块)。假设输入图像的大小是 H × W,通过将其切割成 P×P 的小块后,每个小块的大小为,并且总共有
个图块。每个图块的大小就是一个向量。每个图块被展平(flatten)并通过一个线性变换(即一个全连接层)映射到一个固定的维度 D,形成每个图块的嵌入(embedding)。该嵌入向量的维度就是Transformer的输入维度。
python
from functools import partial
import torch
import torch.nn as nn
from pyzjr.utils.FormatConver import to_2tuple
LayerNorm = partial(nn.LayerNorm, eps=1e-6)
class PatchEmbedding(nn.Module):
def __init__(self, img_size=224, patch_size=16, in_channels=3, embed_dim=768, norm_layer=None):
super().__init__()
self.img_size = to_2tuple(img_size)
self.patch_size = to_2tuple(patch_size)
self.embed_dim = embed_dim
# self.num_patches = (self.img_size[0] // self.patch_size[0]) * (self.img_size[1] // self.patch_size[1])
self.norm = norm_layer(self.embed_dim) if norm_layer else nn.Identity()
self.proj = nn.Conv2d(in_channels, embed_dim, kernel_size=patch_size, stride=patch_size, padding=0)
def forward(self, x):
x = self.proj(x) # 结果形状为 (batch_size, embed_dim, num_patches_H, num_patches_W)
x = x.flatten(2) # 将输出展平成 (batch_size, embed_dim, num_patches)
x = x.transpose(1, 2) # 转置为 (batch_size, num_patches, embed_dim)
x = self.norm(x)
return x
if __name__ == "__main__":
img_size = 224 # 图像大小
patch_size = 16 # 每个patch的大小
in_channels = 3 # 图像通道数
embed_dim = 768 # Patch嵌入维度
patch_embedding = PatchEmbedding(img_size=img_size, patch_size=patch_size, in_channels=in_channels,
embed_dim=embed_dim)
batch_size = 2
x = torch.randn(batch_size, in_channels, img_size, img_size)
output = patch_embedding(x)
print("Final output shape:", output.shape)
上面的实现其实就可以用一个卷积核就能实现patch的分割和嵌入,卷积核公式为:
代入计算刚好就是14。
在ViT中,输入到 Transformer Encoder 之前,需要添加两种类型的编码信息:类别编码 (Class Token) 和 位置编码 (Position Encoding)。这两种编码信息能够帮助 Transformer 更好地理解输入图像的全局信息和局部结构。下面分别介绍这两种编码。
类别编码
类别编码是一个用于表示图像整体的特殊标记符号,它的作用是让 Transformer 在整个图像的上下文中获取全局信息。Transformer 本身是基于序列模型的,它不像卷积神经网络 (CNN) 那样有局部感受野的结构,因此 Transformer 在处理图像时需要有一个机制来了解图像的全局信息。
类别编码就是一个类似于"占位符"的向量,表示图像的全局信息。它会与其他 patch 一同输入到 Transformer Encoder 中,最终模型会学习到类别编码的输出代表了整个图像的特征,最终用于分类或其他任务。
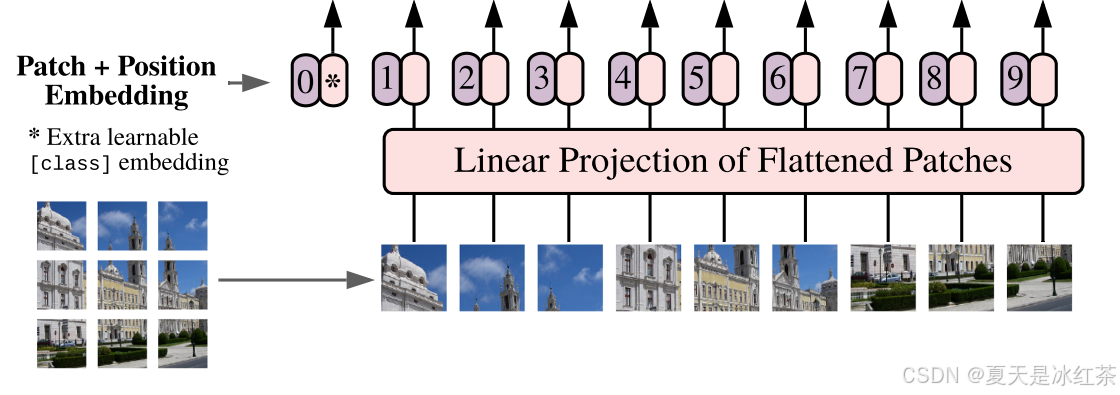
在上面的结构图中就是左侧的0,1,2,3...等等,它是一个与其他图像 patch 同样维度的向量,通常初始化为随机的可训练向量,会与 patch 嵌入向量进行拼接,从而形成一个包含图像所有局部特征和全局特征的输入序列。
位置编码
位置编码用于提供每个 patch 在图像中的相对位置信息。因为 Transformer 的注意力机制本身并不考虑输入的顺序,所以我们需要显式地为每个 patch 添加位置信息,来表示它们在原图中的空间布局。在 Transformer 中,输入的序列是无序的,模型并没有自动的空间位置信息。所以必须通过显式的方式引入每个 patch 的位置信息,才能让模型理解各个 patch 之间的空间关系。
对于图像任务,位置编码能够帮助模型保持空间结构信息,从而提高对图像内容的理解。
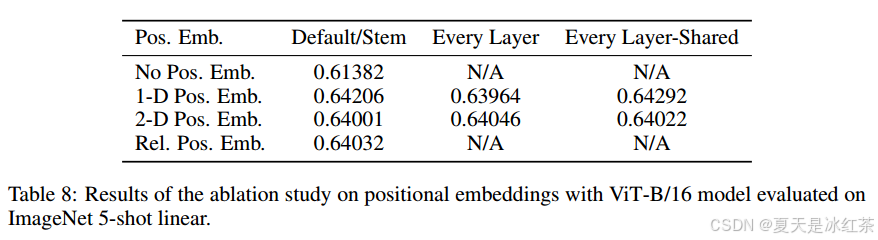
作者通过实验对比,发现加了位置编码的效果更好,而加几维的差别不大,关键是有没有。
位置编码通常是一个与图像的 patch 数量相匹配的向量,每个 patch 对应一个位置编码。通常有两种方式生成位置编码:一种是使用 固定的位置编码,另一种是使用 可学习的位置编码。ViT 中通常使用可学习的位置编码,允许模型根据数据学习每个位置的语义表示。
Transformer Encoder结构
LayerNorm
我想大家都知道常用的比较多的是 BatchNorm ,它依赖于批量数据(即通过计算整个 mini-batch 的均值和方差),而 LayerNorm 是针对每一个样本进行标准化的,它不依赖于 batch 的大小。
Transformer 是基于序列的模型,序列的长度可能变化很大。使用 LayerNorm 可以避免依赖 batch 的统计量,从而使模型能够在不同批次之间保持一致性,且更加稳定,特别是在处理变长序列时。
原理可以看看文档LayerNorm。
Multi-Head Attention
详细可以看我之前写的一篇博文Transformer中Self-Attention以及Multi-Head Attention模块详解。
这里参考的是其他博主(参考文章第一个)的写法,我觉得这里可以直接使用官方实现的torch.nn.MultiheadAttention。
python
class MultiheadAttention(nn.Module):
def __init__(
self,
embed_dim,
num_heads=8,
qkv_bias=False,
attn_drop=0.,
proj_drop=0.,
):
super(MultiheadAttention, self).__init__()
self.num_heads = num_heads
self.head_dim = embed_dim // num_heads
self.scale = self.head_dim ** -0.5
self.qkv = nn.Linear(embed_dim, embed_dim * 3, bias=qkv_bias)
self.attn_drop = nn.Dropout(attn_drop)
self.out_linear = nn.Linear(embed_dim, embed_dim)
self.out_linear_drop = nn.Dropout(proj_drop)
def forward(self, x):
B, N, C = x.shape
qkv = self.qkv(x).reshape(B, N, 3, self.num_heads, C // self.num_heads).permute(2, 0, 3, 1, 4)
q, k, v = qkv[:3]
attn = (q @ k.transpose(-2, -1)) * self.scale
attn = F.softmax(attn, dim=-1)
attn = self.attn_drop(attn)
x = (attn @ v).transpose(1, 2).reshape(B, N, C)
x = self.out_linear(x)
x = self.out_linear_drop(x)
return x
if __name__ == "__main__":
embed_dim = 64
num_heads = 8
batch_size = 2
seq_len = 10
# 随机生成输入数据 (batch_size, seq_len, embed_dim)
x = torch.rand(batch_size, seq_len, embed_dim)
attention_layer = MultiheadAttention(embed_dim, num_heads)
output = attention_layer(x)
print("输入形状:", x.shape)
print("输出形状:", output.shape)
MLP Head
在 ViT 中,MLP 被用来处理 Transformer Encoder 的每一层输出,结构上就是全连接+GELU激活函数+Dropout层。
python
class MLP(nn.Module):
def __init__(self, embed_dim, hidden_dim, drop_rate=0.1, act_layer=nn.GELU):
super(MLP, self).__init__()
self.fc1 = nn.Linear(embed_dim, hidden_dim)
self.act = act_layer()
self.fc2 = nn.Linear(hidden_dim, embed_dim)
self.dropout = nn.Dropout(drop_rate)
def forward(self, x):
x = self.fc1(x)
x = self.act(x)
x = self.dropout(x)
x = self.fc2(x)
x = self.dropout(x)
return x
Transformer Encoder Block
Transformer Encoder其实就是重复堆叠Encoder Block L次,下面是原论文当中给出的图形结构,在实际的代码实现当中,Encoder Block其实是由LayerNorm+Multi-Head Attention+Dropout和LayerNorm+MLP++Dropout实现,我看也有实现的时候使用的是DropPath。
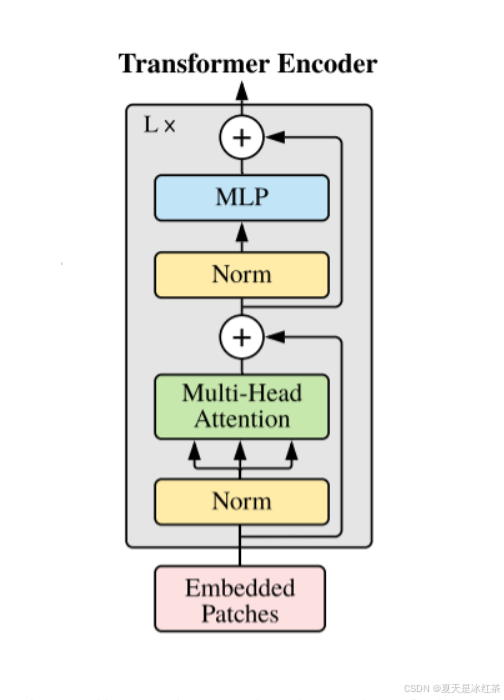
python
class EncoderBlock(nn.Module):
"""Transformer encoder block.
在 mlp block中, MLP 层的隐藏维度是输入的维度的4倍,
详见 Table 1: Details of Vision Transformer model variants
"""
mlp_ratio = 4
def __init__(
self,
dim,
num_heads,
drop_ratio=0.,
attention_dropout_ratio=0.,
drop_path_ratio=0.,
norm_layer=LayerNorm,
act_layer=nn.GELU
):
super(EncoderBlock, self).__init__()
self.num_heads = num_heads
# Attention block
self.norm1 = norm_layer(dim)
self.attention = MultiheadAttention(dim, num_heads, attn_drop=attention_dropout_ratio, proj_drop=drop_ratio)
self.drop_path = DropPath(drop_path_ratio) if drop_path_ratio > 0. else nn.Identity()
# MLP block
self.norm2 = norm_layer(dim)
mlp_hidden_dim = int(dim * self.mlp_ratio)
self.mlp = MLP(dim, mlp_hidden_dim, drop_ratio=drop_ratio, act_layer=act_layer)
def forward(self, x):
x = x + self.drop_path(self.attention(self.norm1(x)))
x = x + self.drop_path(self.mlp(self.norm2(x)))
return x
在mlp block中, MLP 层的隐藏维度是输入的维度的4倍,可以查看论文当中的Table 1。
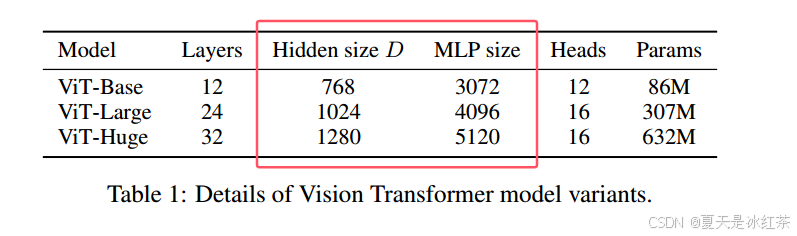
VIT模型实现
python
from functools import partial
import torch
import torch.nn as nn
import torch.nn.functional as F
from pyzjr.utils.FormatConver import to_2tuple
from pyzjr.nn.models.bricks.drop import DropPath
LayerNorm = partial(nn.LayerNorm, eps=1e-6)
class PatchEmbedding(nn.Module):
def __init__(self, img_size=224, patch_size=16, in_channels=3, embed_dim=768, norm_layer=None):
super().__init__()
self.img_size = to_2tuple(img_size)
self.patch_size = to_2tuple(patch_size)
self.embed_dim = embed_dim
self.norm = norm_layer(self.embed_dim) if norm_layer else nn.Identity()
self.proj = nn.Conv2d(in_channels, embed_dim, kernel_size=patch_size, stride=patch_size, padding=0)
def forward(self, x):
x = self.proj(x) # 结果形状为 (batch_size, embed_dim, num_patches_H, num_patches_W)
x = x.flatten(2) # 将输出展平成 (batch_size, embed_dim, num_patches)
x = x.transpose(1, 2) # 转置为 (batch_size, num_patches, embed_dim)
x = self.norm(x)
return x
class MultiheadAttention(nn.Module):
def __init__(
self,
embed_dim,
num_heads=8,
qkv_bias=False,
attention_dropout_ratio=0.,
proj_drop=0.,
):
super(MultiheadAttention, self).__init__()
self.num_heads = num_heads
self.head_dim = embed_dim // num_heads
self.scale = self.head_dim ** -0.5
self.qkv = nn.Linear(embed_dim, embed_dim * 3, bias=qkv_bias)
self.attn_drop = nn.Dropout(attention_dropout_ratio)
self.out_linear = nn.Linear(embed_dim, embed_dim)
self.out_linear_drop = nn.Dropout(proj_drop)
def forward(self, x):
B, N, C = x.shape
qkv = self.qkv(x).reshape(B, N, 3, self.num_heads, C // self.num_heads).permute(2, 0, 3, 1, 4)
q, k, v = qkv[:3]
attn = (q @ k.transpose(-2, -1)) * self.scale
attn = F.softmax(attn, dim=-1)
attn = self.attn_drop(attn)
x = (attn @ v).transpose(1, 2).reshape(B, N, C)
x = self.out_linear(x)
x = self.out_linear_drop(x)
return x
class MLP(nn.Module):
def __init__(self, in_features, hidden_features=None, out_features=None, act_layer=nn.GELU, drop_ratio=0.):
super().__init__()
out_features = out_features or in_features
hidden_features = hidden_features or in_features
self.fc1 = nn.Linear(in_features, hidden_features)
self.act = act_layer()
self.fc2 = nn.Linear(hidden_features, out_features)
self.drop = nn.Dropout(drop_ratio)
def forward(self, x):
x = self.fc1(x)
x = self.act(x)
x = self.drop(x)
x = self.fc2(x)
x = self.drop(x)
return x
class EncoderBlock(nn.Module):
"""Transformer encoder block.
在 mlp block中, MLP 层的隐藏维度是输入的维度的4倍,
详见 Table 1: Details of Vision Transformer model variants
"""
mlp_ratio = 4
def __init__(
self,
dim,
num_heads,
qkv_bias=False,
drop_ratio=0.,
attention_dropout_ratio=0.,
drop_path_ratio=0.,
norm_layer=LayerNorm,
act_layer=nn.GELU
):
super(EncoderBlock, self).__init__()
self.num_heads = num_heads
# Attention block
self.norm1 = norm_layer(dim)
self.attention = MultiheadAttention(dim, num_heads, qkv_bias=qkv_bias, attention_dropout_ratio=attention_dropout_ratio, proj_drop=drop_ratio)
self.drop_path = DropPath(drop_path_ratio) if drop_path_ratio > 0. else nn.Identity()
# MLP block
self.norm2 = norm_layer(dim)
mlp_hidden_dim = int(dim * self.mlp_ratio)
self.mlp = MLP(dim, mlp_hidden_dim, drop_ratio=drop_ratio, act_layer=act_layer)
def forward(self, x):
x = x + self.drop_path(self.attention(self.norm1(x)))
x = x + self.drop_path(self.mlp(self.norm2(x)))
return x
class TransformerEncoder(nn.Module):
"""堆叠 L 次 Transformer encoder block"""
def __init__(
self,
num_layers,
dim,
num_heads,
qkv_bias=False,
drop_ratio=0.,
attention_dropout_ratio=0.,
drop_path_ratio=0.,
norm_layer=LayerNorm,
act_layer=nn.GELU
):
super(TransformerEncoder, self).__init__()
dpr = [x.item() for x in torch.linspace(0, drop_path_ratio, num_layers)] # stochastic depth decay rule
self.layers = nn.ModuleList([
EncoderBlock(
dim=dim,
num_heads=num_heads,
qkv_bias=qkv_bias,
drop_ratio=drop_ratio,
attention_dropout_ratio=attention_dropout_ratio,
drop_path_ratio=dpr[_],
norm_layer=norm_layer,
act_layer=act_layer
)
for _ in range(num_layers)
])
self.norm = norm_layer(dim)
def forward(self, x):
for layer in self.layers:
x = layer(x)
x = self.norm(x)
return x
class VisionTransformer(nn.Module):
def __init__(
self,
img_size=224,
patch_size=16,
in_channels=3,
num_classes=1000,
hidden_dim=768,
num_heads=12,
num_layers=12,
qkv_bias=True,
drop_ratio=0.,
attention_dropout_ratio=0.,
drop_path_ratio=0.,
norm_layer=LayerNorm,
act_layer=nn.GELU
):
super(VisionTransformer, self).__init__()
assert img_size == 224, f"Image size must be 224, but got {img_size}"
assert img_size % patch_size == 0, f"Image size {img_size} must be divisible by patch size {patch_size}"
self.num_classes = num_classes
self.num_tokens = 1
self.patch_embed = PatchEmbedding(img_size=img_size, patch_size=patch_size, in_channels=in_channels,
embed_dim=hidden_dim, norm_layer=norm_layer)
num_patches = (img_size // patch_size) * (img_size // patch_size)
self.cls_token = nn.Parameter(torch.zeros(1, 1, hidden_dim))
self.pos_embed = nn.Parameter(torch.zeros(1, num_patches + self.num_tokens, hidden_dim))
self.pos_drop = nn.Dropout(p=drop_ratio)
self.blocks = TransformerEncoder(
num_layers=num_layers,
dim=hidden_dim,
num_heads=num_heads,
qkv_bias=qkv_bias,
drop_ratio=drop_ratio,
attention_dropout_ratio=attention_dropout_ratio,
drop_path_ratio=drop_path_ratio,
norm_layer=norm_layer,
act_layer=act_layer
)
self.norm = norm_layer(hidden_dim)
self.head = nn.Linear(hidden_dim, num_classes)
self._initialize_weights()
def _initialize_weights(self):
for m in self.modules():
if isinstance(m, nn.Linear):
nn.init.trunc_normal_(m.weight, std=.01)
if m.bias is not None:
nn.init.zeros_(m.bias)
elif isinstance(m, nn.Conv2d):
nn.init.kaiming_normal_(m.weight, mode="fan_out")
if m.bias is not None:
nn.init.zeros_(m.bias)
elif isinstance(m, nn.LayerNorm):
nn.init.zeros_(m.bias)
nn.init.ones_(m.weight)
def forward(self, x):
x = self.patch_embed(x) # [B, 196, 768]
cls_token = self.cls_token.expand(x.shape[0], -1, -1) # [B, 1, 768]
x = torch.cat((cls_token, x), dim=1) # [B, 196+1, 768]
x = self.pos_drop(x + self.pos_embed) # [B, 197, 768]
x = self.blocks(x) # [B, 197, 768]
x = x[:, 0] # [B, 768]
x = self.head(x) # [B, num_classes]
return x
def vit_b_16(num_classes=1000) -> VisionTransformer:
return VisionTransformer(
img_size=224,
patch_size=16,
num_classes=num_classes,
hidden_dim=768,
num_heads=12,
num_layers=12,
)
def vit_b_32(num_classes=1000) -> VisionTransformer:
return VisionTransformer(
img_size=224,
patch_size=32,
num_classes=num_classes,
hidden_dim=768,
num_heads=12,
num_layers=12,
)
def vit_l_16(num_classes=1000) -> VisionTransformer:
return VisionTransformer(
img_size=224,
patch_size=16,
num_classes=num_classes,
hidden_dim=1024,
num_heads=16,
num_layers=24,
)
def vit_l_32(num_classes=1000) -> VisionTransformer:
return VisionTransformer(
img_size=224,
patch_size=32,
num_classes=num_classes,
hidden_dim=1024,
num_heads=16,
num_layers=24,
)
def vit_h_14(num_classes=1000) -> VisionTransformer:
return VisionTransformer(
img_size=224,
patch_size=14,
num_classes=num_classes,
hidden_dim=1280,
num_heads=16,
num_layers=32,
)
if __name__=="__main__":
import torchsummary
device = 'cuda' if torch.cuda.is_available() else 'cpu'
input = torch.ones(2, 3, 224, 224).to(device)
net = vit_h_14(num_classes=4)
net = net.to(device)
out = net(input)
print(out)
print(out.shape)
torchsummary.summary(net, input_size=(3, 224, 224))
# vit_b_16 Total params: 85,651,204
# vit_b_32 Total params: 87,420,676
# vit_l_16 Total params: 303,105,028
# vit_l_32 Total params: 305,464,324
# vit_h_14 Total params: 630,442,244
虽然我这里实现的可以进行图像分类训练,但对于大多数实际应用,我还是推荐使用官方实现的代码模型,预训练模型进行迁移学习。这里仅作为学习参考。
参考文章
保姆级教学 ------ 手把手教你复现Vision Transformer_transformer输出特征图大小-CSDN博客
【Transformer系列】深入浅出理解ViT(Vision Transformer)模型-CSDN博客