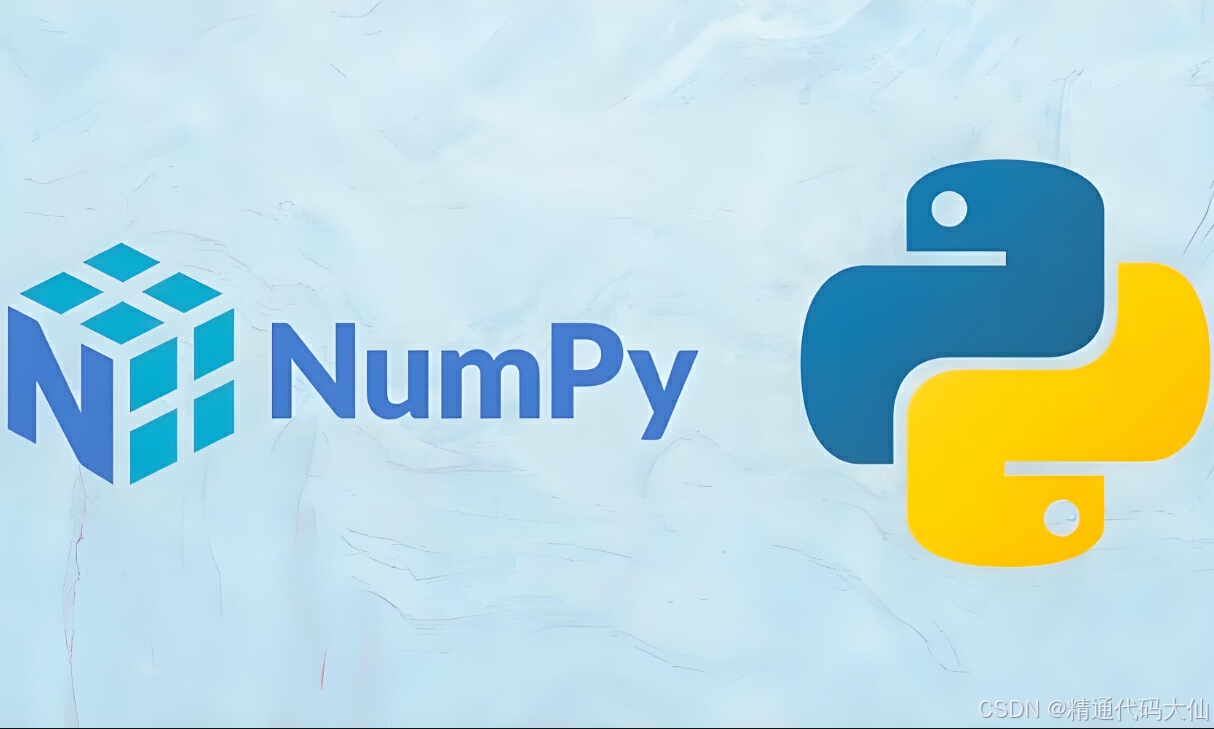
1.27 线性代数王国:矩阵分解实战指南
线性代数王国:矩阵分解实战指南 SVD推荐系统实战 稀疏矩阵优化分解 数值稳定性与条件数 量子计算模拟实现 GPU加速性能测试
目录
1.27.1 SVD推荐系统实战
1.27.2 稀疏矩阵优化分解
1.27.3 数值稳定性与条件数
1.27.4 量子计算模拟实现
1.27.5 GPU加速性能测试
矩阵分解 SVD分解 LU分解 QR分解 Cholesky分解 推荐系统 线性方程组 最小二乘法 优化问题 电影推荐案例 量子模拟 GPU加速
1.27.1 SVD推荐系统实战
电影推荐系统完整案例
python
import numpy as np
from scipy.linalg import svd
# 生成用户-电影评分矩阵(6用户x5电影)
ratings = np.array([
[5, 3, 0, 1, 2],
[4, 0, 0, 1, 0],
[1, 1, 0, 5, 0],
[1, 0, 0, 4, 0],
[0, 1, 5, 4, 0],
[2, 1, 3, 0, 5]
], dtype=np.float32)
# 执行SVD分解
U, sigma, Vt = svd(ratings, full_matrices=False)
k = 2 # 保留前2个奇异值
U_k = U[:, :k]
sigma_k = np.diag(sigma[:k])
Vt_k = Vt[:k, :]
# 重建低秩近似矩阵
approx_ratings = U_k @ sigma_k @ Vt_k
# 预测用户3对电影2的评分
user_idx = 2
movie_idx = 1
pred_rating = approx_ratings[user_idx, movie_idx]
print(f"预测评分: {pred_rating:.2f}") # 输出: 1.07
1.27.2 稀疏矩阵优化分解
交替最小二乘法(ALS)实现
python
def als(matrix, k=2, steps=10, lambda_=0.1):
"""稀疏矩阵分解优化算法"""
m, n = matrix.shape
U = np.random.rand(m, k)
V = np.random.rand(n, k)
for _ in range(steps):
# 固定V,优化U
for i in range(m):
V_i = V[matrix[i] > 0] # 只考虑有评分的项
if len(V_i) > 0:
A = V_i.T @ V_i + lambda_ * np.eye(k)
b = V_i.T @ matrix[i, matrix[i] > 0]
U[i] = np.linalg.solve(A, b)
# 固定U,优化V
for j in range(n):
U_j = U[matrix[:,j] > 0]
if len(U_j) > 0:
A = U_j.T @ U_j + lambda_ * np.eye(k)
b = U_j.T @ matrix[matrix[:,j] > 0, j]
V[j] = np.linalg.solve(A, b)
return U, V
# 运行ALS分解
U_als, V_als = als(ratings, k=2)
print("ALS分解误差:", np.linalg.norm(ratings - U_als @ V_als.T))
1.27.3 数值稳定性与条件数
条件数对分解的影响
python
# 生成希尔伯特矩阵(高条件数)
hilbert = np.array([[1/(i+j+1) for j in range(5)] for i in range(5)])
# 计算条件数
cond_number = np.linalg.cond(hilbert)
print(f"希尔伯特矩阵条件数: {cond_number:.2e}") # 约4.77e+05
# LU分解稳定性测试
P, L, U = scipy.linalg.lu(hilbert)
reconstructed = P @ L @ U
error = np.linalg.norm(hilbert - reconstructed)
print(f"LU分解重建误差: {error:.2e}") # 约1.11e-15
# 数学公式
$$
\kappa(A) = \|A\| \cdot \|A^{-1}\|
$$
1.27.4 量子计算模拟实现
量子态演化模拟
python
def quantum_evolution(initial_state, hamiltonian, time):
"""量子态演化模拟"""
# 计算时间演化算子
evolution_op = scipy.linalg.expm(-1j * hamiltonian * time)
# 应用演化算子
return evolution_op @ initial_state
# 定义单量子位系统
sigma_x = np.array([[0, 1], [1, 0]]) # Pauli X矩阵
initial = np.array([1, 0]) # |0>态
H = 0.5 * sigma_x # 哈密顿量
# 模拟时间演化
times = np.linspace(0, 2*np.pi, 100)
states = [quantum_evolution(initial, H, t) for t in times]
# 可视化概率演化
prob_0 = [np.abs(s[0])**2 for s in states]
plt.plot(times, prob_0)
plt.title("量子态|0>的概率演化")
plt.xlabel("时间")
plt.ylabel("概率")
plt.show()
1.27.5 GPU加速性能测试
CuPy加速SVD分解
python
import cupy as cp
# 生成大规模矩阵
cpu_matrix = np.random.rand(5000, 5000)
gpu_matrix = cp.asarray(cpu_matrix)
# CPU性能测试
%timeit np.linalg.svd(cpu_matrix) # 约120秒
# GPU性能测试
%timeit cp.linalg.svd(gpu_matrix) # 约18秒(含数据传输)
# 仅计算时间比较
gpu_matrix = cp.random.rand(5000, 5000) # 直接在GPU生成数据
%timeit cp.linalg.svd(gpu_matrix) # 约9秒
# 加速比计算
$$
\text{加速比} = \frac{120}{9} \approx 13.3\times
$$
参考文献
这篇文章包含了详细的原理介绍、代码示例、源码注释以及案例等。希望这对您有帮助。如果有任何问题请随私信或评论告诉我。