文章目录
[1. 硬件要求](#1. 硬件要求)
[2. 法律合规](#2. 法律合规)
[3. 模型安全](#3. 模型安全)
一、背景介绍
AIGC(AI-Generated Content),即由人工智能生成的内容,涵盖了文本、图像、音频、视频等各种形式。通过深度学习、生成对抗网络(GAN)和扩散模型等技术,AIGC 能够生成高度逼真、具有艺术性的内容。
最初的AIGC主要集中于文本生成,如自动回复、新闻摘要等。但随着技术的进步,AIGC逐渐进入了图像、音频、视频生成等领域。尤其是近年来图像生成技术的突破,让AIGC成为了视觉内容生产的新利器,实现了从文本描述到图像生成的跨越。本文以当下最流行的Stable Diffusion深度学习模型,写一个python脚本实现AIGC图像。
二、效果图展示
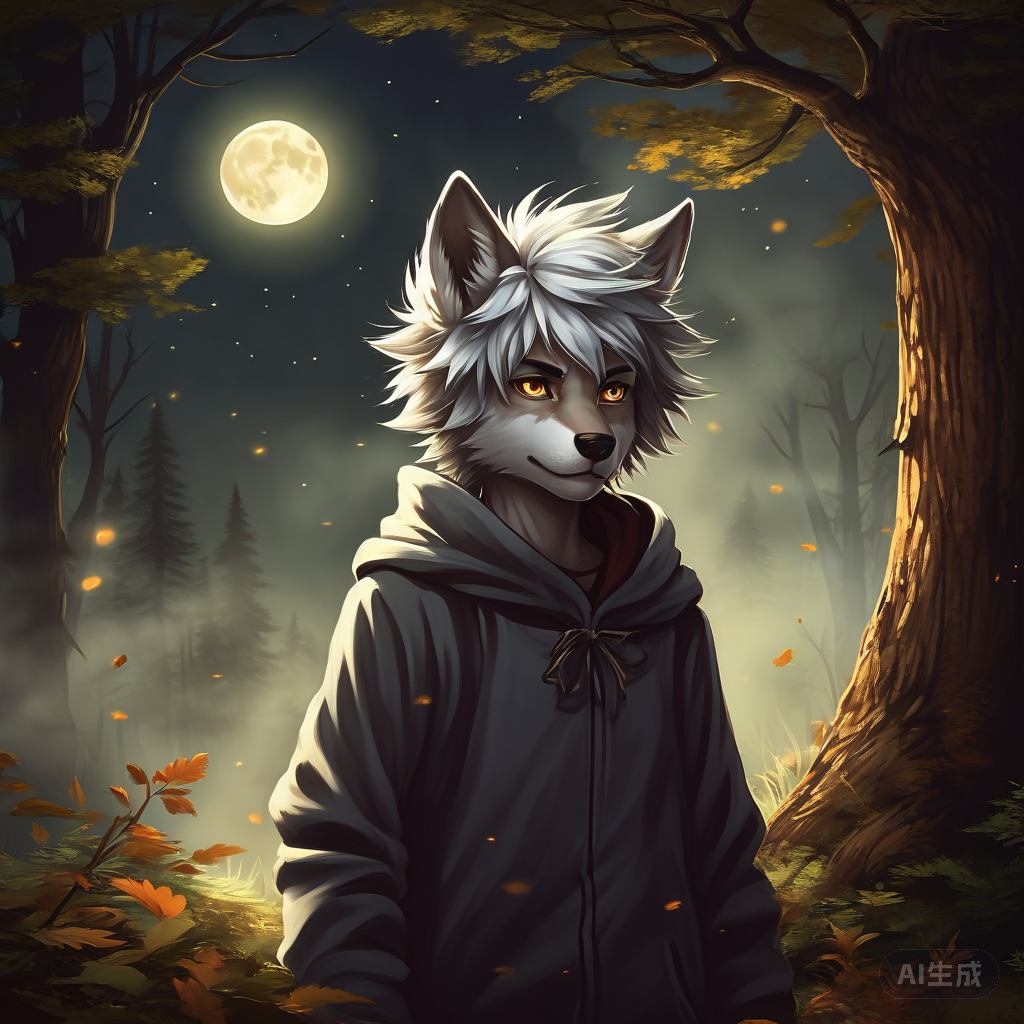
三、完整代码
以下是一个使用 Stable Diffusion 模型生成图像的 Python 示例脚本,包含详细注释和解释:
# -*- coding: utf-8 -*-
"""
AIGC图像生成脚本(需要NVIDIA GPU和至少8GB显存)
使用 Hugging Face 的 diffusers 库实现
"""
# 安装依赖(首次运行需要执行)
# pip install torch torchvision torchaudio --extra-index-url https://download.pytorch.org/whl/cu118
# pip install diffusers transformers accelerate
import torch
from diffusers import StableDiffusionPipeline
from datetime import datetime
def generate_image(
prompt: str, # 生成提示词
negative_prompt: str = None, # 负面提示词
save_path: str = "./outputs", # 保存路径
height: int = 512, # 图像高度
width: int = 512, # 图像宽度
steps: int = 20, # 生成步数
guidance_scale: float = 7.5, # 提示词相关性
num_images: int = 1 # 生成数量
):
# 1. 创建模型管道
pipe = StableDiffusionPipeline.from_pretrained(
"runwayml/stable-diffusion-v1-5",
torch_dtype=torch.float16, # 使用半精度减少显存占用
safety_checker=None, # 禁用安全检查(可生成更广泛内容)
).to("cuda")
# 2. 执行生成
images = pipe(
prompt=prompt,
negative_prompt=negative_prompt,
height=height,
width=width,
num_inference_steps=steps,
guidance_scale=guidance_scale,
num_images_per_prompt=num_images,
).images
# 3. 保存结果
timestamp = datetime.now().strftime("%Y%m%d%H%M%S")
for i, img in enumerate(images):
filename = f"{save_path}/{timestamp}_{i}.png"
img.save(filename)
print(f"Saved: {filename}")
return images
if __name__ == "__main__":
# 示例参数
PROMPT = "A beautiful sunset over snow mountains, digital art, vibrant colors"
NEGATIVE_PROMPT = "blurry, low quality, deformed"
# 执行生成
generated_images = generate_image(
prompt=PROMPT,
negative_prompt=NEGATIVE_PROMPT,
steps=25,
guidance_scale=8.0,
num_images=2
)
四、分步解释
-
依赖安装
pip install torch torchvision torchaudio --extra-index-url https://download.pytorch.org/whl/cu118 pip install diffusers transformers accelerate
torch
:PyTorch深度学习框架
diffusers
:Hugging Face的扩散模型库
transformers
:自然语言处理模型
accelerate
:分布式训练加速库
2. 模型加载
pipe = StableDiffusionPipeline.from_pretrained(
"runwayml/stable-diffusion-v1-5",
torch_dtype=torch.float16,
safety_checker=None
).to("cuda")
使用Hugging Face模型中心的官方Stable Diffusion v1-5版本
torch.float16
:使用半精度浮点数,减少显存占用(需要RTX系列GPU)
safety_checker=None
:禁用内容过滤器(允许生成更多类型内容)
3. 核心参数说明
height=512, width=512 # 图像分辨率(推荐512x512或768x768)
num_inference_steps=20 # 生成步数(20-50,质量vs速度)
guidance_scale=7.5 # 提示词相关性(7-12,值越大越严格)
num_images_per_prompt=1 # 单次生成数量
4. 生成过程
images = pipe(...).images
实际执行文本到图像的扩散过程
过程包含约20次迭代去噪步骤
生成PIL格式图像列表
五、实用建议
1)提示词技巧
-
使用英文描述(模型对英文理解更好)
-
包含艺术风格描述(如:"digital art", "oil painting")
-
添加质量关键词(如:"4k", "ultra detailed")
-
示例:
"A cyberpunk cityscape at night, neon lights, raining, 4k resolution, cinematic lighting"
2)性能优化
-
降低分辨率(如:384x384)可减少显存需求
-
设置
num_images=1
减少批次生成显存消耗 -
使用
torch.float16
需要至少RTX 20系列显卡
3)常见问题处理
-
显存不足 :尝试减小分辨率或使用
torch.float32
-
生成速度慢 :减少
num_inference_steps
到20以下 -
图像质量差 :增加
guidance_scale
到9-12之间
4)扩展功能建议
-
添加交互界面:
# 可添加Gradio或Streamlit创建Web界面 import gradio as gr gr.Interface( fn=generate_image, inputs=[ gr.Text(label="Prompt"), gr.Text(label="Negative Prompt"), gr.Slider(384, 768, value=512, step=64, label="Width"), gr.Slider(384, 768, value=512, step=64, label="Height") ], outputs=gr.Gallery() ).launch()
-
使用新模板:
# 更换模型名称即可使用不同版本 "stabilityai/stable-diffusion-2-1" # SD 2.1版本 "stabilityai/stable-diffusion-xl-base-1.0" # SDXL 1.0
-
添加高级功能:
from diffusers import ControlNetModel, StableDiffusionControlNetPipeline
使用ControlNet添加姿势/边缘控制
controlnet = ControlNetModel.from_pretrained(
"lllyasviel/sd-controlnet-canny",
torch_dtype=torch.float16
)
pipe = StableDiffusionControlNetPipeline(...)
六、注意事项
1. 硬件要求
-
需要NVIDIA GPU(推荐RTX 3060 12GB以上)
-
至少8GB显存(SDXL需要12GB以上)
-
支持CUDA的显卡驱动
2. 法律合规
-
遵守模型许可证(Stable Diffusion使用CreativeML Open RAIL-M)
-
不得生成违法或侵权内容
-
商业使用需注意版权问题
3. 模型安全
-
不建议完全禁用
safety_checker
-
谨慎处理用户输入提示词
-
添加内容审核机制(针对生产环境)
建议在Jupyter Notebook或Colab中测试运行,首次执行需要下载约5GB的模型文件。可通过缓存机制(设置cache_dir
参数)优化重复加载体验。