大家好,我是Edison。
上周水了一篇 Microsoft.Extensions.AI 的介绍文章,很多读者反馈想要了解更多。很多时候,除了集成LLM实现聊天对话,还会有很多语义搜索和RAG的使用场景,那么今天就给大家介绍一下如何完成语义搜索。
Microsoft.Extensions.VectorData介绍
语义搜索正在改变应用程序查找和解释数据的方式,它专注于语义关联,而不仅仅是关键字匹配。
Microsoft.Extensions.VectorData 是一组 .NET代码库,旨在管理 .NET 应用程序中基于向量的数据。这些库为与向量存储交互提供了一个统一的 C# 抽象层,使开发人员能够有效地处理嵌入并执行向量相似性查询。
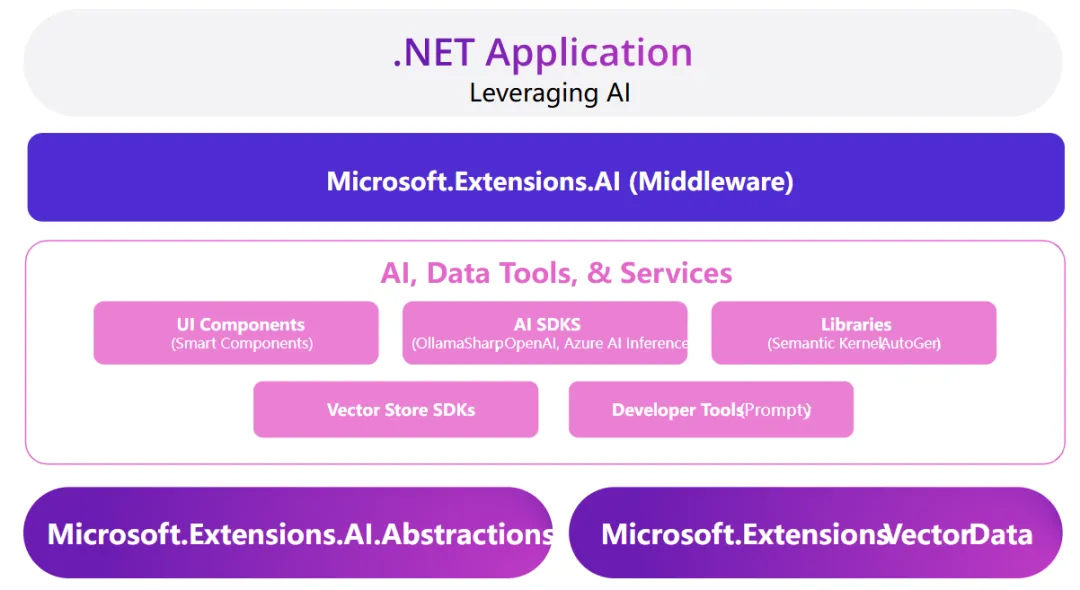
更多该代码库的内容请参考:Luis 《Introducting Microsoft.Extensions.VectorData》
在接下来的demo中,我们会使用以下工具:
(1) Qdrant 作为 VectorStore
(2) Ollama 运行 all-minilm 模型 作为 Emedding生成器
ollama pull all-minilm
Qdrant向量搜索引擎
Qdrant是一个向量相似性搜索引擎,它提供了一个生产就绪的服务,拥有便捷的 API来存储、搜索和管理带有额外负载的点(即向量)。它非常适合需要高效相似性搜索的应用程序。我们可以在 Docker 容器中运行 它,这也使它成为对开发人员友好的选择。
容器运行Qdrant:
docker run -p 6333:6333 -p 6334:6334 \
-v $(pwd)/qdrant_storage:/qdrant/storage \
qdrant/qdrant
验证Qdrant运行:访问 server:6333/dashboard
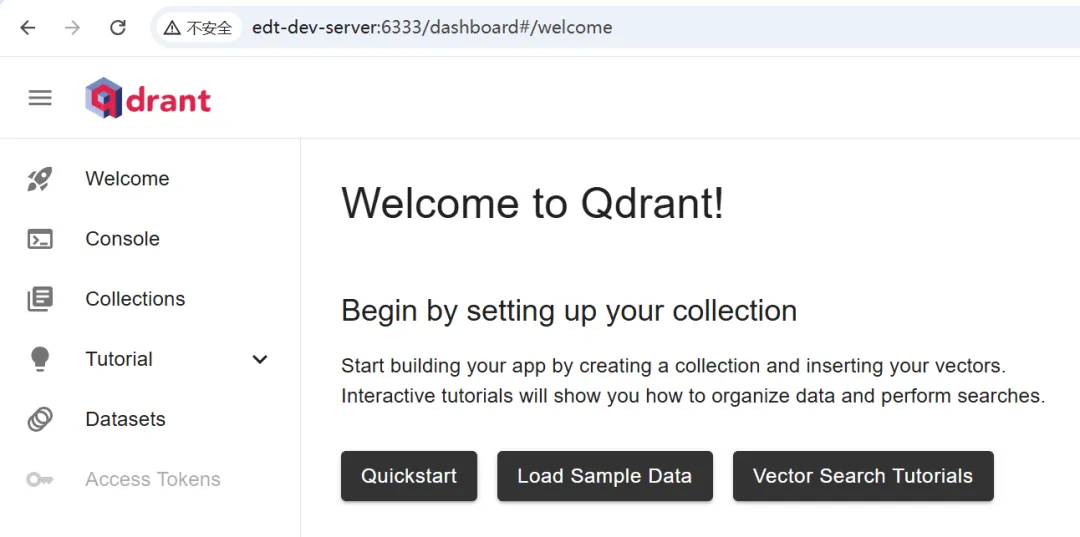
开始DEMO案例
安装NuGet包:
Microsoft.Extensions.AI (preview)
Microsoft.Extensions.Ollama (preivew)
Microsoft.Extensions.AI.OpenAI (preivew)
Microsoft.Extensions.VectorData.Abstractions (preivew)
Microsoft.SemanticKernel.Connectors.Qdrant (preivew)
这里我们假设做一个CloudService的语义搜索,分下面一些步骤来实现它。
Step1. 配置文件appsettings.json:
{
"Embedding": {
"EndPoint": "http://localhost:11434",
"Model": "all-minilm"
},
"Qdrant": {
"Host": "edt-dev-server",
"Port": 6334
}
}
Step2. 加载配置:
var config = new ConfigurationBuilder()
.AddJsonFile($"appsettings.json")
.Build();
Step3. 初始化Embedding生成器:这里我们使用的是本地的Ollama运行all-minilm模型来做。
var generator =
new OllamaEmbeddingGenerator(new Uri(config["Embedding:EndPoint"]), config["Embedding:Model"]);
此外,我们也可以使用OpenAI的Embedding服务:
var generator = new OpenAIClient(new ApiKeyCredential(config["OneAPI:ApiKey"]), new OpenAIClientOptions() { Endpoint = new Uri(config["OneAPI:EndPoint"]) })
.AsEmbeddingGenerator(modelId: config["Embedding:ModelId"]);
Step4. 初始化Qdrant向量存储:
var vectorStore = new QdrantVectorStore(new QdrantClient(config["Qdrant:Host"], int.Parse(config["Qdrant:Port"])));
// Get the collection if it exist in qdrant
var cloudServicesStore = vectorStore.GetCollection<ulong, CloudService>("cloudServices");
// Create the collection if it doesn't exist yet.
await cloudServicesStore.CreateCollectionIfNotExistsAsync();
Step5. 插入测试数据:
// Define the test data
var cloudServices = new List<CloudService>()
{
new CloudService
{
Key=1,
Name="Azure App Service",
Description="Host .NET, Java, Node.js, and Python web applications and APIs in a fully managed Azure service. You only need to deploy your code to Azure. Azure takes care of all the infrastructure management like high availability, load balancing, and autoscaling."
},
new CloudService
{
Key=2,
Name="Azure Service Bus",
Description="A fully managed enterprise message broker supporting both point to point and publish-subscribe integrations. It's ideal for building decoupled applications, queue-based load leveling, or facilitating communication between microservices."
},
new CloudService
{
Key=3,
Name="Azure Blob Storage",
Description="Azure Blob Storage allows your applications to store and retrieve files in the cloud. Azure Storage is highly scalable to store massive amounts of data and data is stored redundantly to ensure high availability."
},
new CloudService
{
Key=4,
Name="Microsoft Entra ID",
Description="Manage user identities and control access to your apps, data, and resources.."
},
new CloudService
{
Key=5,
Name="Azure Key Vault",
Description="Store and access application secrets like connection strings and API keys in an encrypted vault with restricted access to make sure your secrets and your application aren't compromised."
},
new CloudService
{
Key=6,
Name="Azure AI Search",
Description="Information retrieval at scale for traditional and conversational search applications, with security and options for AI enrichment and vectorization."
}
};
// Insert test data into the collection in qdrant
foreach (var service in cloudServices)
{
service.Vector = await generator.GenerateEmbeddingVectorAsync(service.Description);
await cloudServicesStore.UpsertAsync(service);
}
其中,CloudService的定义如下:
public class CloudService
{
[VectorStoreRecordKey]
public ulong Key { get; set; }
[VectorStoreRecordData]
public string Name { get; set; }
[VectorStoreRecordData]
public string Description { get; set; }
[VectorStoreRecordVector(384, DistanceFunction.CosineSimilarity)]
public ReadOnlyMemory<float> Vector { get; set; }
}
Step6. 生成查询Emedding并从Qdrant中执行查询:
// Generate query embedding
var query = "Which Azure service should I use to store my Word documents?";
var queryEmbedding = await generator.GenerateEmbeddingVectorAsync(query);
// Query from vector data store
var searchOptions = new VectorSearchOptions()
{
Top = 1, // Only return the Top 1 record from Qdrant
VectorPropertyName = "Vector"
};
var results = await cloudServicesStore.VectorizedSearchAsync(queryEmbedding, searchOptions);
await foreach (var result in results.Results)
{
Console.WriteLine($"Name: {result.Record.Name}");
Console.WriteLine($"Description: {result.Record.Description}");
Console.WriteLine($"Vector match score: {result.Score}");
Console.WriteLine();
}
首先,验证下Qdrant中是否新增了数据:
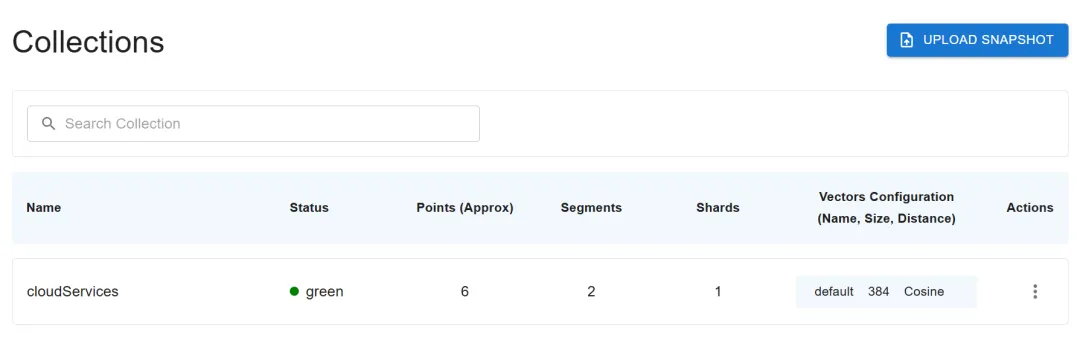
其次,查看运行结果显示:返回最匹配的一个数据返回,因为我们设置的Top1记录。
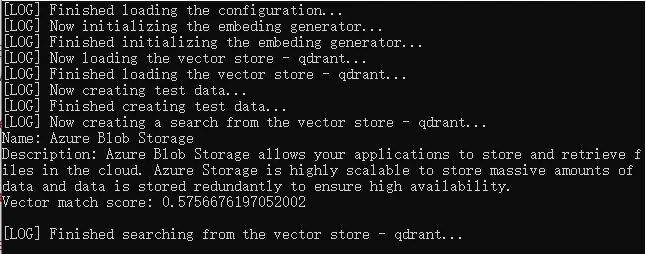
完整的代码示例请参考该示例代码的GitHub仓库。
小结
本文介绍了Microsoft.Extensions.Vector的基本概念 和 基本使用,结合Embedding Model(如all-minilm) 和 VectorStore(如Qdrant),我们可以快速实现语义搜索,而不仅仅是关键字匹配。
如果你也是.NET程序员希望参与AI应用的开发,那就快快了解和使用基于Microsoft.Extensioins.AI的生态组件库吧。
参考内容
Eddie Chen,《探索Microsoft.Extensions.VectorData与Qdrant和Azure AI搜索的使用》
Luis,《Introducting Microsoft.Extensions.VectorData》
路边石,《Microsoft.Extensions.AI.OpenAI官方代码示例》
推荐内容
作者:周旭龙
出处:https://edisonchou.cnblogs.com
本文版权归作者和博客园共有,欢迎转载,但未经作者同意必须保留此段声明,且在文章页面明显位置给出原文链接。