文章目录
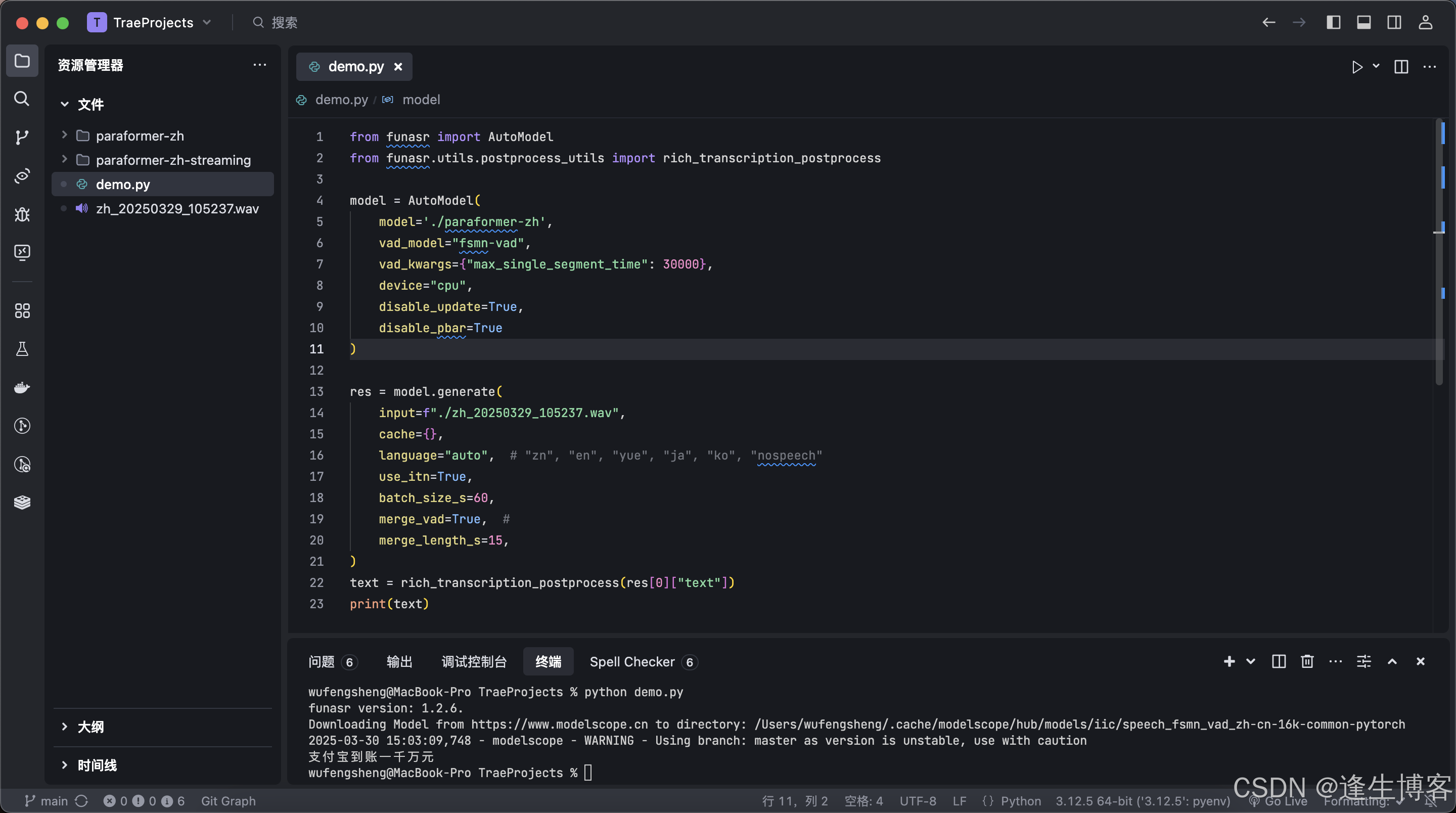
Github
官网
简介
FunASR是一个基础语音识别工具包,提供多种功能,包括语音识别(ASR)、语音端点检测(VAD)、标点恢复、语言模型、说话人验证、说话人分离和多人对话语音识别等。FunASR提供了便捷的脚本和教程,支持预训练好的模型的推理与微调。
我们在ModelScope与huggingface上发布了大量开源数据集或者海量工业数据训练的模型,可以通过我们的模型仓库了解模型的详细信息。代表性的Paraformer非自回归端到端语音识别模型具有高精度、高效率、便捷部署的优点,支持快速构建语音识别服务,详细信息可以阅读(服务部署文档)。
模型
安装
bash
pip3 install -U funasr
# 或从源码安装
git clone https://github.com/alibaba/FunASR.git && cd FunASR
pip3 install -e ./
非流式应用示例
bash
# 语音识别,带时间戳,非流式
git clone https://huggingface.co/funasr/paraformer-zh
python
from funasr import AutoModel
from funasr.utils.postprocess_utils import rich_transcription_postprocess
model = AutoModel(
model='./paraformer-zh',
vad_model="fsmn-vad",
vad_kwargs={"max_single_segment_time": 30000},
device="cpu",
disable_update=True,
disable_pbar=True
)
res = model.generate(
input=f"./zh_20250329_105237.wav",
cache={},
language="auto", # "zn", "en", "yue", "ja", "ko", "nospeech"
use_itn=True,
batch_size_s=60,
merge_vad=True, #
merge_length_s=15,
)
text = rich_transcription_postprocess(res[0]["text"])
print(text)
流式应用示例
bash
# 语音识别、流媒体
git clone https://huggingface.co/funasr/paraformer-zh-streaming
python
from funasr import AutoModel
chunk_size = [0, 10, 5] #[0, 10, 5] 600ms, [0, 8, 4] 480ms
encoder_chunk_look_back = 4 #number of chunks to lookback for encoder self-attention
decoder_chunk_look_back = 1 #number of encoder chunks to lookback for decoder cross-attention
model = AutoModel(model="./paraformer-zh-streaming", disable_update=True)
import soundfile
import os
speech, sample_rate = soundfile.read('./zh_20250329_105237.wav')
chunk_stride = chunk_size[1] * 960 # 600ms
cache = {}
# 计算总共需要处理的音频块数
total_chunk_num = int(len((speech)-1)/chunk_stride+1)
# 遍历所有音频块,逐块识别
for i in range(total_chunk_num):
speech_chunk = speech[i*chunk_stride:(i+1)*chunk_stride]
is_final = i == total_chunk_num - 1
res = model.generate(input=speech_chunk, cache=cache, is_final=is_final, chunk_size=chunk_size, encoder_chunk_look_back=encoder_chunk_look_back, decoder_chunk_look_back=decoder_chunk_look_back)
print(res)
注:chunk_size为流式传输延迟的配置。 [0,10,5]表示实时显示粒度为1060=600ms,前瞻信息为560=300ms。每次推理的输入为600ms(样本点为16000*0.6=960),输出为对应的文本。对于最后一段语音的输入,is_final=True需要设置 ,强制输出最后一个单词。
python
import sounddevice as sd
import numpy as np
import queue
from funasr import AutoModel
model = AutoModel(
model="./paraformer-zh-streaming",
disable_update=True,
disable_pbar=True
)
# 录音参数
SAMPLE_RATE = 16000 # FunASR 需要 16kHz 采样率
CHANNELS = 1
CHUNK_MS = 600 # 每个 chunk 600ms
CHUNK_SIZE = CHUNK_MS * 16 # 600ms 音频 = 9600 采样点
LOOK_BACK = 4 # Encoder 关注的 chunk 数量
# 音频缓存
audio_queue = queue.Queue()
cache = {} # 识别缓存
# 录音回调
def callback(indata, frames, time, status):
if status:
print(f"音频流错误: {status}")
audio_queue.put(indata.copy()) # 存入队列
# 开始监听麦克风
print("正在监听麦克风... (Ctrl+C 退出)")
try:
with sd.InputStream(samplerate=SAMPLE_RATE, channels=CHANNELS, callback=callback, blocksize=CHUNK_SIZE):
buffer = np.array([], dtype=np.float32) # 音频缓冲区
while True:
if not audio_queue.empty():
# 获取音频数据
audio_chunk = audio_queue.get().flatten()
buffer = np.append(buffer, audio_chunk) # 添加到缓冲区
# 每 600ms 进行一次识别
while len(buffer) >= CHUNK_SIZE:
chunk = buffer[:CHUNK_SIZE]
buffer = buffer[CHUNK_SIZE:] # 移除已处理部分
# 执行语音识别
res = model.generate(
input=chunk,
cache=cache,
is_final=False,
chunk_size=[0, 10, 5],
encoder_chunk_look_back=LOOK_BACK,
decoder_chunk_look_back=1
)
print("识别结果:", res[0]['text'])
except KeyboardInterrupt:
print("停止监听")