Controlnet 介绍
- ControlNet论文:Adding Conditional Control to Text-to-Image Diffusion Models
- 项目地址:lllyasviel/ControlNet: Let us control diffusion models
- ControlNet 1.1项目地址:lllyasviel/ControlNet-v1-1-nightly
- diffusers框架的ControlNet训练脚本:diffusers/examples/controlnet
- ControlNet-G站教程:github.com/Mikubill/sd...
ControlNet是一项变革性技术,显著增强了文本到图像扩散模型的能力,实现了前所未有的图像生成空间控制。作为一个神经网络架构,ControlNet可以与规模庞大的预训练模型(如Stable Diffusion)无缝集成。它利用了这些在数十亿张图像上训练的模型 将空间条件引入图像创建过程。这些条件的范围很广,从边缘和人体姿势到深度和分割图,使用户能够以单纯文本提示无法实现的方式引导图像生成。
首先,它固定原始模型的参数,确保基础训练保持不变。随后,ControlNet引入了一个编码层的克隆模型用于训练,采用"零卷积"。这些特殊设计的卷积层以零权重开始,小心地集成新的空间条件。这种方法可以防止任何干扰噪声的介入,在保持模型原有能力的同时,启动新的学习轨迹。
模型
ControlNet模型:将 ControlNet 或 T2IAdaptor 模型纳入你的工作流,从而确保扩散模型受益于所选模型提供的特定指导。每个模型,无论是 ControlNet 还是 T2IAdaptor,都经过严格训练,以根据特定数据类型或风格偏好影响图像生成过程。许多 T2IAdaptor 模型的功能与 ControlNet 模型非常相似.
预处理器 :"image"输入必须连接到"ControlNet Preprocessor"节点,这对于使你的图像适应所使用的ControlNet 模型的特定需求至关重要。使用针对所选ControlNet模型定制的正确预处理器至关重要。此步骤可确保原始图像经过必要的修改---例如格式、大小、颜色的调整,或特定滤镜的应用---以优化它用于 ControlNet 的指导。经过这个预处理阶段后,原始图像被修改后的版本所取代,ControlNet 随后将其应用。这个过程可确保你的输入图像为 ControlNet 过程做好了精确准备
预处理器模型
lllyasviel 汇集了大部分预处理器模型,地址: lllyasviel/Annotators at main
ControlNet系列模型
ControlNet 1.0 模型权重
markdown
* **Author**: lllyasviel
* **GitHub Repository**:[https://github.com/lllyasviel/ControlNet(opens in a new tab)](https://github.com/lllyasviel/ControlNet)
* **Hugging Face Repository**:[https://huggingface.co/lllyasviel/ControlNet/tree/main(opens in a new tab)](https://huggingface.co/lllyasviel/ControlNet/tree/main)
* **Compatible Stable Diffusion Versions**: stable diffusion SD1.5,2.0
File Name | Size |
---|---|
control_sd15_canny.pth | 5.71 GB |
control_sd15_depth.pth | 5.71 GB |
control_sd15_hed.pth | 5.71 GB |
control_sd15_mlsd.pth | 5.71 GB |
control_sd15_normal.pth | 5.71 GB |
control_sd15_openpose.pth | 5.71 GB |
control_sd15_scribble.pth | 5.71 GB |
control_sd15_seg.pth | 5.71 GB |
ControlNet 1.1 模型权重(原生版本与剪枝版本,其中原生版本(1.35GB)后缀为pth,剪枝版本(689M)后缀为safetensors)
markdown
* 基于 sd v1.5 模型的 ControlNet v1.1 模型下载地址: [lllyasviel/ControlNet-v1-1 · HF Mirror (hf-mirror.com)](https://hf-mirror.com/lllyasviel/ControlNet-v1-1)
* 基于 sd v2.1 模型的 ControlNet v1.1 模型下载地址: [thibaud/controlnet-sd21 at main (hf-mirror.com)](https://hf-mirror.com/thibaud/controlnet-sd21/tree/main)
* 模型下载(700m版)[https://huggingface.co/webui/ControlNet-modules-safetensors](https://hf-mirror.com/webui/ControlNet-modules-safetensors/) 700m版为压缩过的16位浮点模型(16fp 表示范围更小,但文件更小,内存需求也更小,每个模型仅700MB)
* **Provider**:comfyanonymous **Hugging Face Repository**:[https://huggingface.co/comfyanonymous/ControlNet-v1-1_fp16_safetensors/tree/main(opens in a new tab)](https://huggingface.co/comfyanonymous/ControlNet-v1-1_fp16_safetensors/tree/main) **Size**: 723MB **Note**: Provided by the ComfyUI author's Hugging Face repository, it offers a better user experience in ComfyUI and is also compatible with other UIs.
* **Provider**:comfyanonymous **Hugging Face Repository**:[https://huggingface.co/comfyanonymous/ControlNet-v1-1_fp16_safetensors/tree/main(opens in a new tab)](https://huggingface.co/comfyanonymous/ControlNet-v1-1_fp16_safetensors/tree/main) **Size**: 136MB **Note**: Provided by the ComfyUI author's Hugging Face repository, it offers a better user experience in ComfyUI and is also compatible with other UIs.
ControlNet 1.1 与 ControlNet 1.0 具有完全相同的架构。ControlNet 1.1在ControlNet 1.0的基础上进行了优化,提高了鲁棒性和控制效果,同时添加了几个新的 ControlNet 模型。
从ControlNet 1.1开始,ControlNet 模型开始有标准的命名规则(SCNNR)来命名所有模型,这样我们在使用时也能更加方便。
具体的命名规则如下图所示: ControlNet 1.1一共发布了14个模型(11个成品模型和3 个实验模型):
control_v11p_sd15_canny
control_v11p_sd15_mlsd
control_v11f1p_sd15_depth
control_v11p_sd15_normalbae
control_v11p_sd15_seg
control_v11p_sd15_inpaint
control_v11p_sd15_lineart
control_v11p_sd15s2_lineart_anime
control_v11p_sd15_openpose
control_v11p_sd15_scribble
control_v11p_sd15_softedge
control_v11e_sd15_shuffle(实验模型)
control_v11e_sd15_ip2p(实验模型)
control_v11f1e_sd15_tile(实验模型)
ControlNet SDXL 模型权重
The official ControlNet has not provided any versions of the SDXL model. Therefore, this article primarily compiles ControlNet models provided by different authors. Due to time constraints, I am unable to test each model individually, so you can visit the links to the model repositories I provide to learn more about them.
For each model below, you'll find:
- Rank 256 files (reducing the original
4.7GB
ControlNet models down to~738MB
Control-LoRA models) and experimental- Rank 128 files (reducing to model down to
~377MB
)
Each Control-LoRA has been trained on a diverse range of image concepts and aspect ratios.
Others
- ControlNet第三方模型权重(包含二维码生成ControlNet、光影控制ControlNet、手部控制ControlNet等)
- ControlNet TheMistoAI 线控模型权重
T2I-Adapters 系列模型
- T2I-G站主页 SDXL :github.com/TencentARC/...
- T2I-G站介绍:github.com/TencentARC/...
- T2I-G SD : GitHub - TencentARC/T2I-Adapter at SD
T2I-Adapters比ControlNets高效得多。ControlNets会显著降低生成速度,而T2I-Adapters对生成速度几乎没有负面影响。
在ControlNets中,ControlNet模型每次迭代运行一次。对于T2I-Adapter,模型总共只运行一次。
T2I-Adapters在ComfyUI中的使用方式与ControlNets相同
- SD Model: TencentARC/T2I-Adapter · HF Mirror (hf-mirror.com)
- SDXL Model:
- Sketch: huggingface.co/TencentARC/...
- Canny: huggingface.co/TencentARC/...
- Lineart: huggingface.co/TencentARC/...
- Openpose: huggingface.co/TencentARC/...
- Depth-mid: huggingface.co/TencentARC/...
- Depth-zoe: huggingface.co/TencentARC/...
模型分类说明
目前 ControlNet 有 18 种 Control Type,分别是 Canny,Depth,NormalMap,OpenPose,MLSD,Lineart,SoftEdge,Scribble/Sketch,Segmentation,Shuffle,Tile/Blur,Inpaint,InstructP2P,Reference,Recolor,Revision,T2I-Adapter,IP-Adapter。
Line Extractors
硬边缘 Canny
Canny边缘检测算法 能够检测出原始图片中各对象的边缘轮廓特征 ,提取生成线稿图,作为SD模型生成时的条件。
接着再设置不同的提示词,让SD模型生成构图相同但是内容不同的画面,也可以用来给线稿图重新上色。
Canny算法中一共有2种Preprocessor,分别是Canny和invert (from white bg & black line) 。
预处理器 Node | 模型 | 场景 |
---|---|---|
Canny Edge | coadapter-canny-sd15v1,control_any3_canny,control_sd15_canny,control_canny-fp16,control_v11p_sd15_canny,controlnet-canny-sdxl-1.0,diff_control_sd15_canny_fp16,sdxl_canny_fp16,t2iadapter_canny_sd14v1,t2iadapter_canny_sd15v2 |
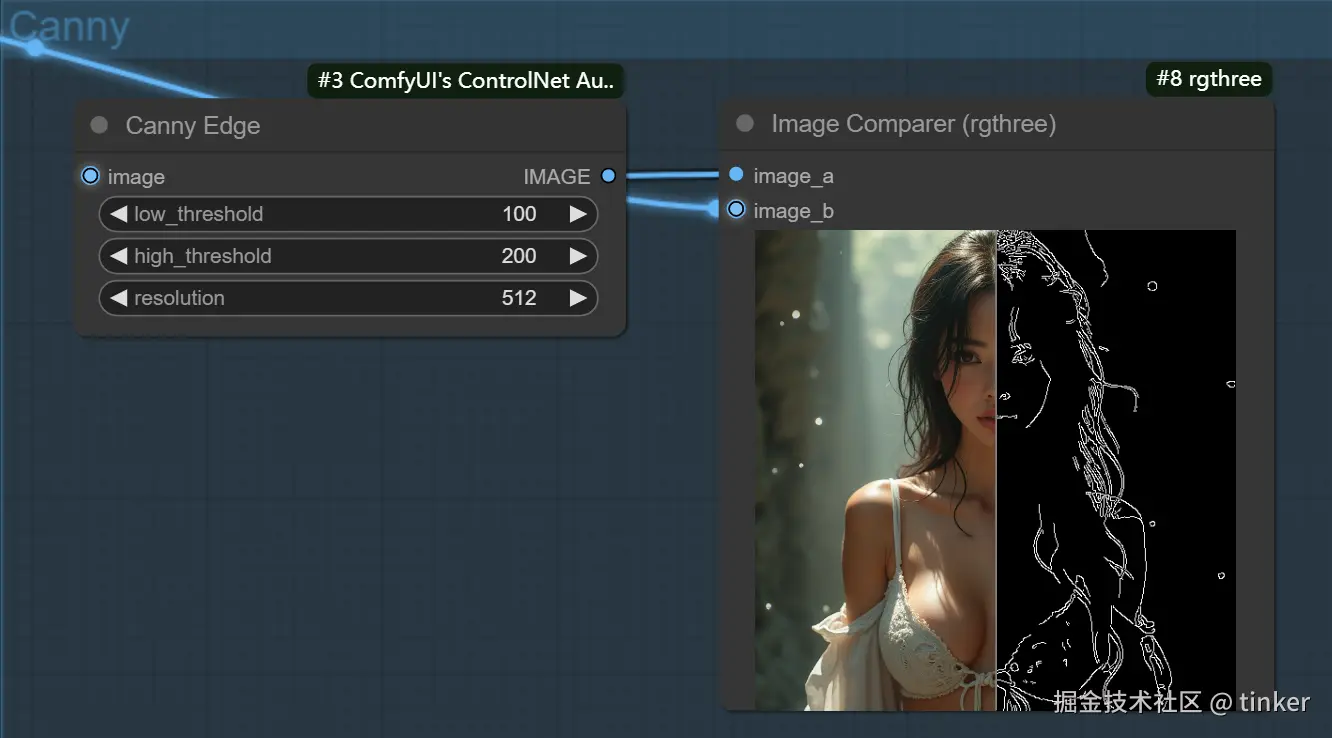
线稿 lineart
Lineart算法(线稿模型)与Canny算法大同小异,可以检测出输入图像中的线稿信息。
Lineart算法一共有五种预处理器,分别是lineart_anime预处理器、lineart_anime_denoise预处理器、lineart_coarse预处理器、lineart_realistic预处理器和lineart_standard 预处理器。
下面是Lineart算法各预处理器的具体效果:
- Lineart_anime预处理器:用于生成动漫风格的线稿/素描信息。
- Lineart_anime_denoise预处理器:Lineart_anime预处理器的优化版,在提取动漫风格线稿/素描信息的同时进行降噪处理。
- Lineart_coarse预处理器:用于生成粗糙线稿/素描,线条相比较于其它预处理器,的确更粗一些,效果也很不错,生成的图像则趋于真实。
- Lineart_realistic预处理器:能较好地提取人物线稿部分。
- Lineart_standard(from white bg&black line)预处理器:是一种特殊模式,将白色背景和黑色线条的图像转换为线稿或素描,能较好的还原场景中的线条,跟原图较为相似。
预处理器 Node | 模型 | 场景 |
---|---|---|
Realistic Lineart, Standard Lineart | control_v11p_sd15_lineart | |
Anime Lineart, Manga Lineart | control_v11p_sd15s2_lineart_anime |
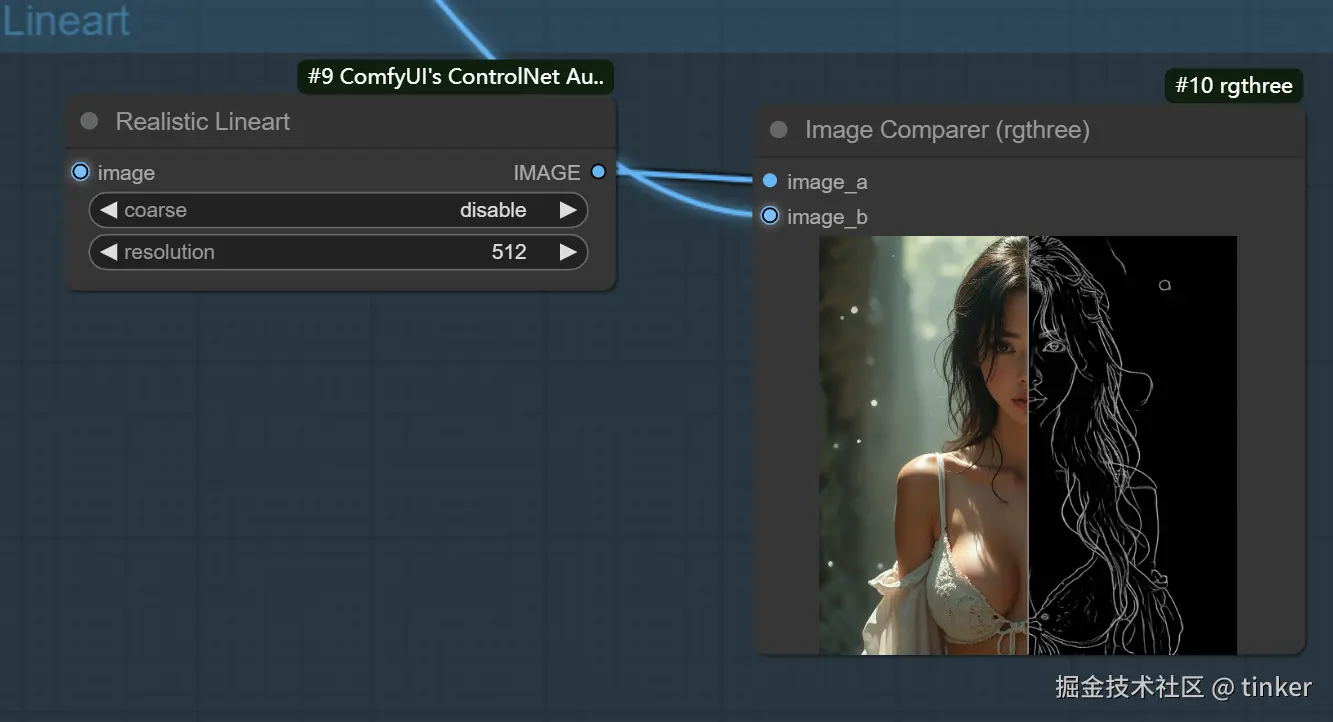
直线边缘 M-LSD
MLSD是一种线条检测算法,通过分析图片的线条结构和几何形状来构建出建筑外框(直线) ,它对于提取具有直边的轮廓非常有用,例如室内设计、建筑物、街景、相框和纸张边缘,但是对人或其它有弧度的物体边缘提取效果很差。
如果我们想要对室内、建筑等输入图片进行重构,原图环境中有人物出现,但是新生成的图片中不希望有人物,那么使用MLSD算法就可以很好的避开人物线条的检测,从而能够生成纯建筑的新图片。
预处理器 Node | 模型 | 场景 |
---|---|---|
M-LSD Lines | control_v11p_sd15_mlsd | MLSD算法非常适合用于室内设计、建筑设计等场景 |
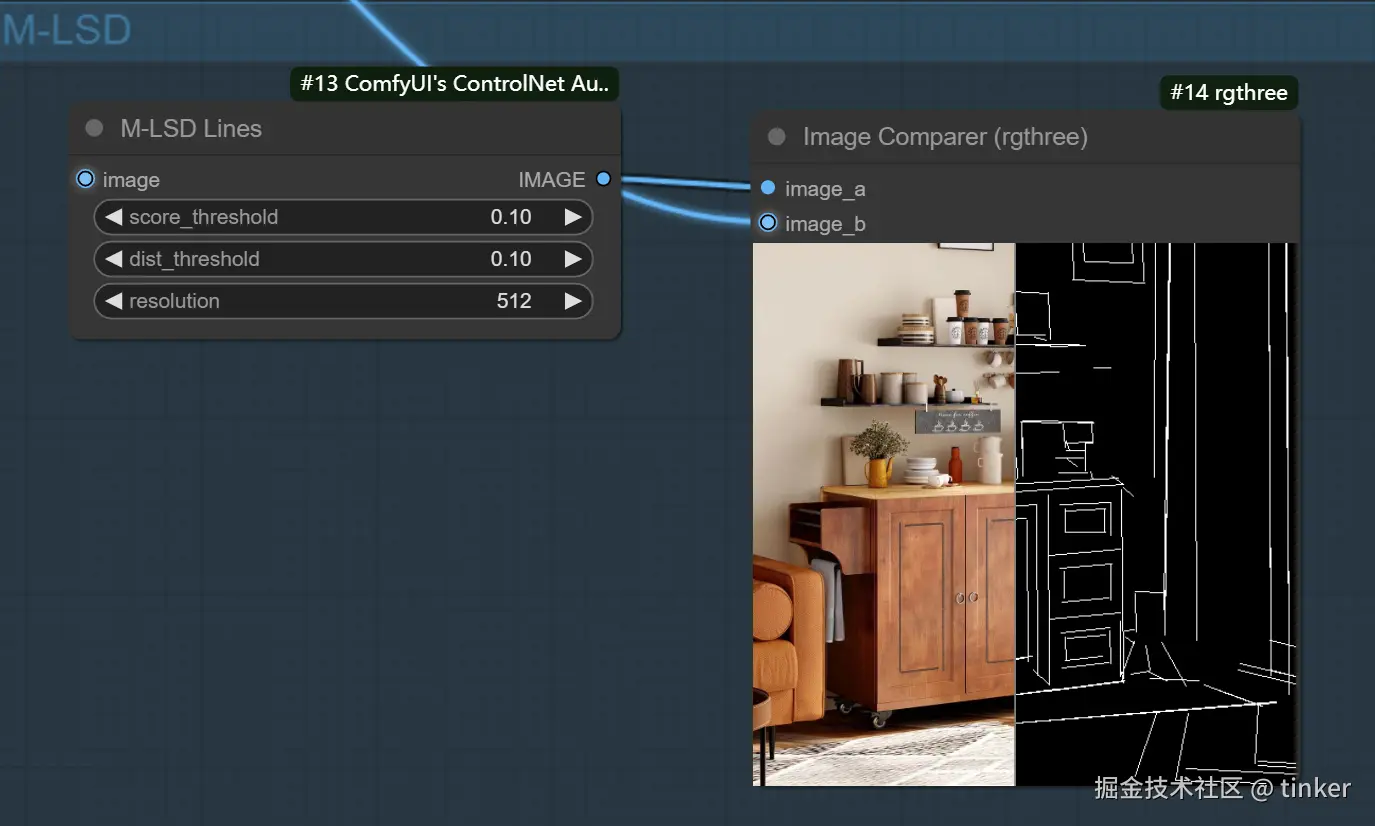
软边缘 Soft Edge
SoftEdge算法的主要作用是检测图像的软边缘轮廓,与Canny算法相比检测出的边缘轮廓没有那么细致和严格,相对比较宽松和柔性,让我们在AI绘画过程中有更大的灵活性与更多的创意空间。在传统图像处理领域,图像边缘指的是图像中颜色或亮度变化显著的地方,对应着物体与背景之间、或者物体与物体之间的交界。通过检测出图像边缘信息,我们可以提取出图像中的形状、纹理、结构等重要信息,进一步用于AI绘画、AIGC、传统深度学习、智能驾驶等领域的后续任务。
SoftEdge算法的 Preprocessor,分别是softedge_hed预处理器、softedge_hedsafe预处理器、softedge_pidinet预处理器以及softedge_pidinetsafe预处理器,其中带有"safe"字样的表示精简版。
softedge_hed预处理器跟Canny算法类似,也是一种边缘检测算法,可以把Canny算法理解为用铅笔提取边缘,而softedge_hed预处理器则是换用毛笔,被提取的图像边缘将会非常柔和,细节也会更加丰富,绘制的人物明暗对比明显,轮廓感更强,适合在保持原来构图的基础上重新着色和对画面风格进行改变。
如果是生成棱角分明或者机甲一类的图像,我们推荐使用Canny算法。如果是想要生成人物和动物等图像,使用softedge_hed预处理器效果会更好。
同样的,softedge_pidinet预处理器也是一种边缘检测算法,比起softedge_hed预处理器,它的泛化性与鲁棒性更强。
预处理器 | 预处理器 Node | 模型 | 场景 |
---|---|---|---|
softedge_hed(custom_nodes\comfyui_controlnet_aux\ckpts\lllyasviel/Annotators\ControlNetHED.pth.) | HED Soft-Edge Lines, PiDiNet Soft-Edge Lines | control_v11p_sd15_softedge | |
TEED Preprocessor | controlnet-sd-xl-1.0-softedge-dexined |
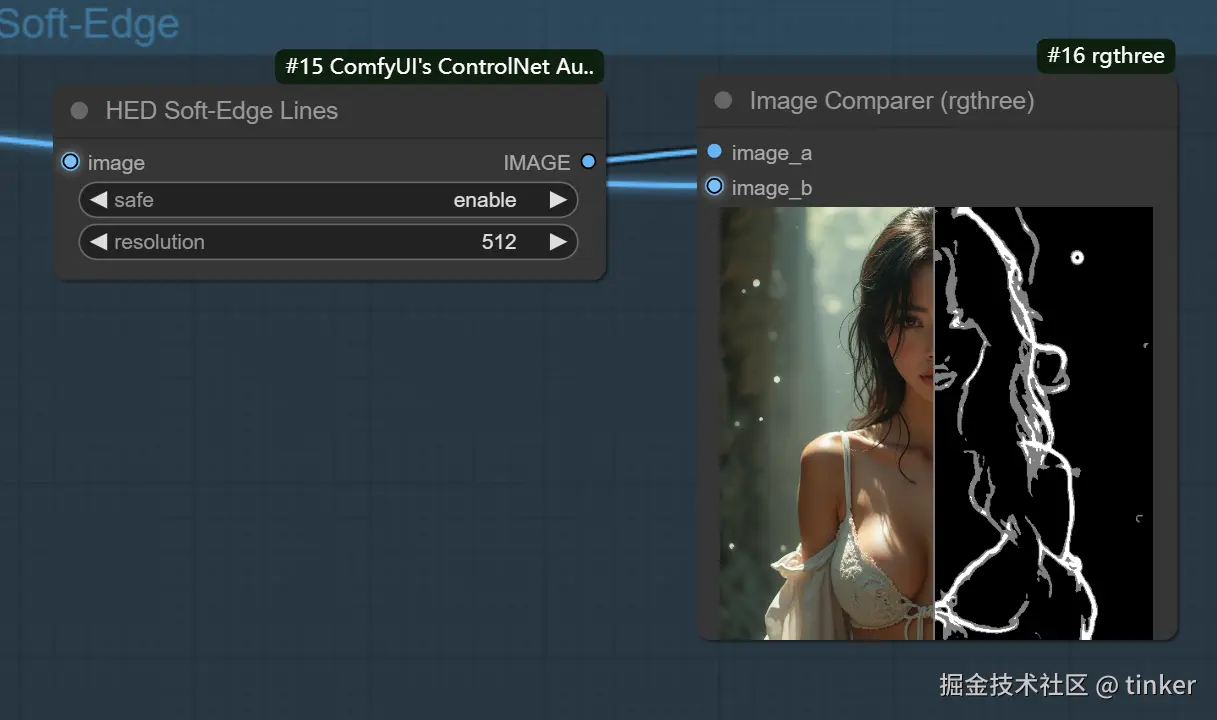
涂鸦 Scribble
Scribble/Sketch算法能够提取图片中曝光对比度比较明显的区域,生成黑白稿,涂鸦成图,其比Canny算法的自由度更高,也可以用于对手绘线稿进行着色处理。
从下图可以看到提取的涂鸦,不但保留了曝光度对比较大的部分,而且细节保留的也很不错。细节保留的越多,那么SD重新生成图片时所能更改的部分就越少。
当然的,我们也可以直接上传涂鸦,然后通过Scribble/Sketch算法进行补充绘图。
ControlNet Scribble/Sketch算法一共有四种预处理器,分别是:scribble_hed预处理器、scribble_pidinet预处理器、scribble_xdog预处理器以及t2ia_sketch_pidi预处理器。
- Scribble:旨在将图像转换为详细的艺术作品,模拟手绘涂鸦或草图。
- scribble_hed预处理器:由Holistically-Nested Edge Detection(HED) 边缘检测器构成,擅长生成像真人一样的轮廓,能够配合SD系列模型进行图像进行重新着色和重新设计样式等任务。
- scribble_pidinet预处理器:由Pixel Difference network(Pidinet) 网络构成,能够检测图像中曲线和直线边缘等特征。其结果与scribble_hed预处理器类似,但通常会产生更清晰的线条和更少的细节。
- scribble_xdog 预处理器:由EXtendedDifferenceofGaussian(XDoG)技术构成,同样是一种图像边缘检测算法。与其他预处理器不同的是,scribble_xdog预处理器附带一个XDoG Threshold参数可供我们调整阈值,这让我们的控制效果更加精细化。
- t2ia_sketch_pidi预处理器:t2ia_sketch_pidi预处理器在处理涂鸦图像时考虑一些特定的因素,例如涂鸦的形状、颜色、纹理等,以帮助算法更好地理解和利用图像中的信息(待确认补充)。
预处理器 | 预处理器 Node | 模型 | 场景 |
---|---|---|---|
scribble_pidinet | Scribble Lines | control_v11p_sd15_scribble, control_scribble, t2iadapter_sketch_sd14v1.pth | |
scribble_xdog | Scribble XDoG Lines | control_v11p_sd15_scribble, control_scribble | |
scribble_hed | Fake Scribble Lines | control_v11p_sd15_scribble, control_scribble, t2iadapter_sketch_sd14v1.pth | |
Scribble | Binary Lines | control_scribble |
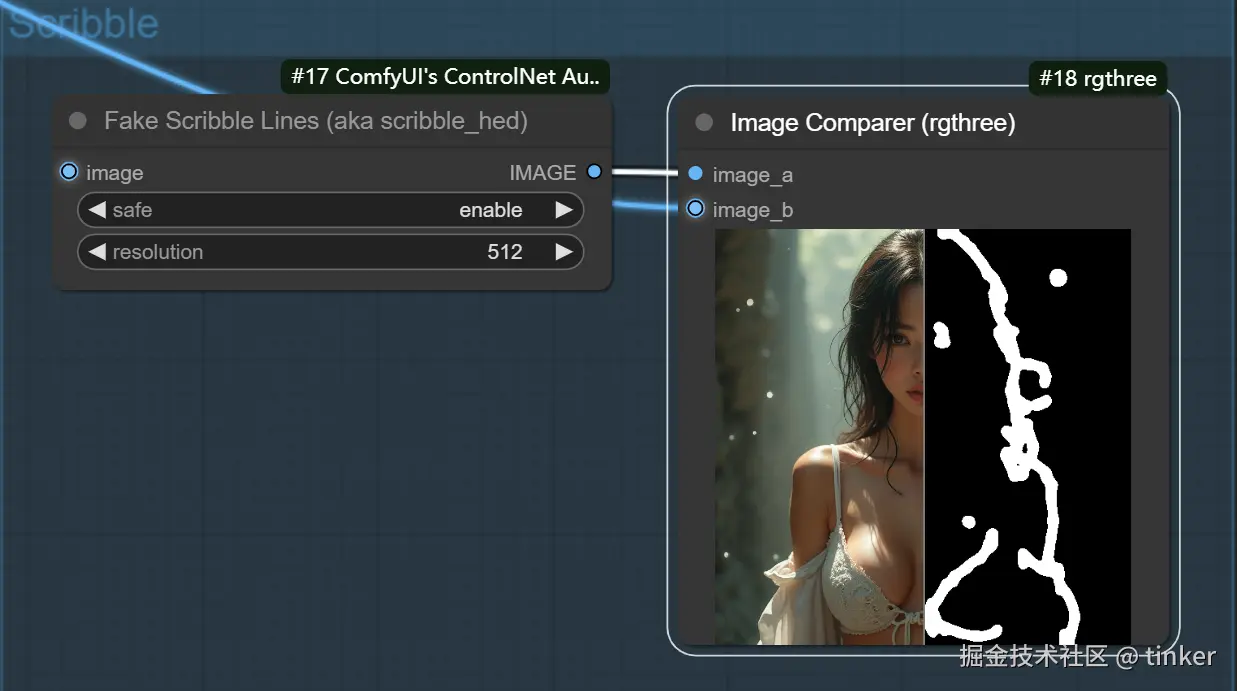
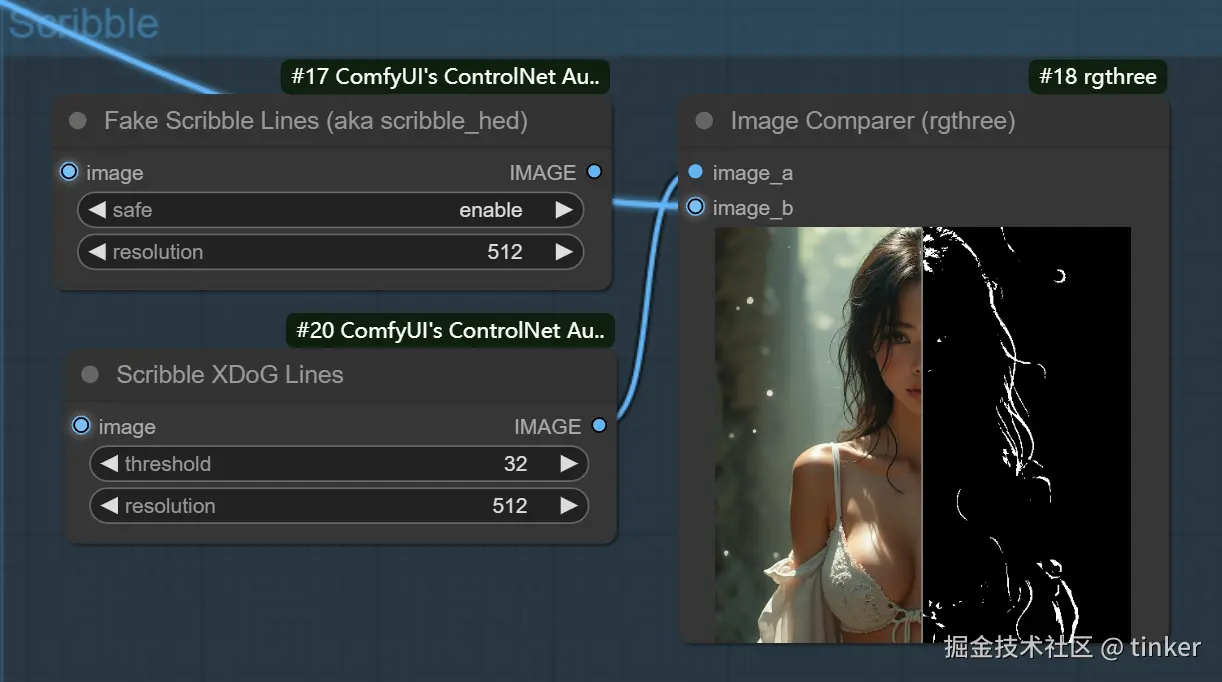
MistoLine+AnyLine 组建一个基于 SDXL 版本模型的线条处理
MistoLine 是一个可以适配任意类型线稿,准确性高,稳定性优秀的SDXL-ControlnetNet模型。它可以基于用户输入的任意类型的线稿图(手绘、各类controlnet-line preprocessor、模型线框轮廓等)作为条件,生成高质量图像(短边大于1024px),无需再根据不同线预处理器选择不同的controlnet模型,MistoLine在各类线稿条件下都有较好的泛化能力。 MistoLine是使用全新的线预处理算法(Anyline) ,并基于stabilityai/stable-diffusion-xl-base-1.0的Unet以及大模型训练工程上的创新,重新进行训练的Controlnet模型 。MistoLine对于不同类型的线稿输入都有较好的表现,在更加复杂的场景下对于细节还原,prompt对齐,稳定性方面对比现有的Controlnet模型都有更好的表现结果
- 预处理器
- AnyLine
- Anyline 模型地址:hf-mirror.com/TheMistoAI/...
- 中国(大陆地区)便捷下载地址 (MTEED.pth):链接:pan.baidu.com/s/1ik11P_u1... 提取码:v8f1
- 模型
- MistoLine 模型下载地址:TheMistoAI/MistoLine · HF Mirror (hf-mirror.com)
- mistoLine_rank256.safetensors : General usage version, for ComfyUI and AUTOMATIC1111-WebUI.
- mistoLine_fp16.safetensors : FP16 weights, for ComfyUI and AUTOMATIC1111-WebUI.
- AnyLine
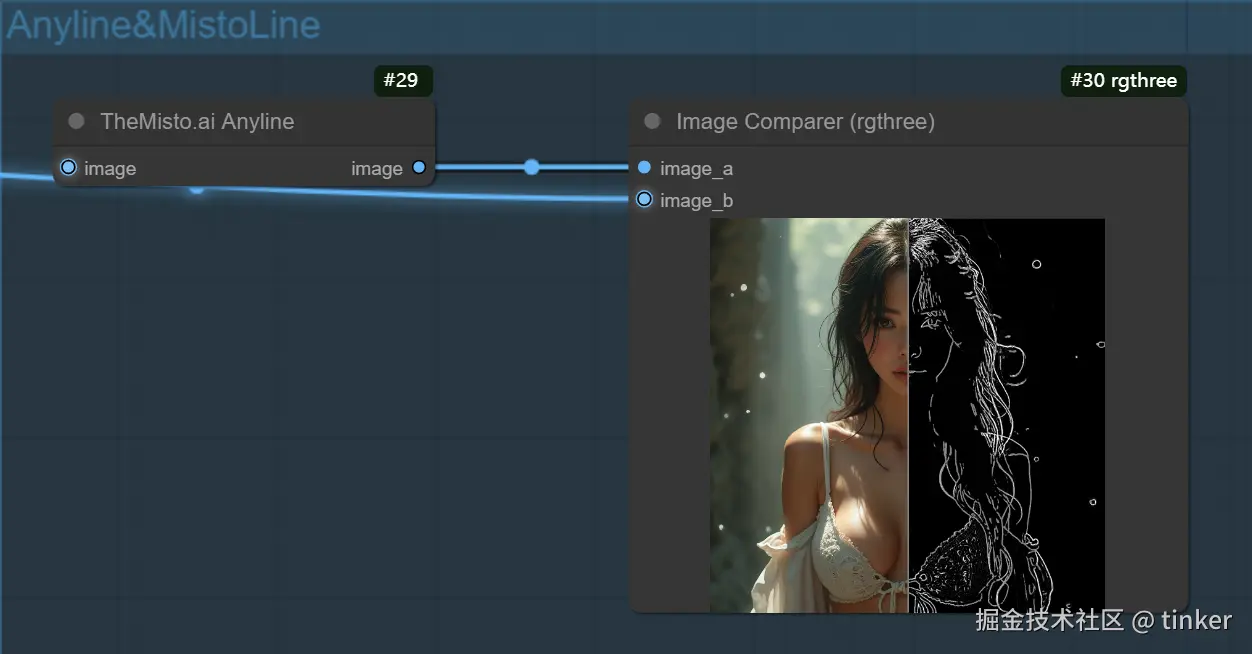
Normal and Depth Estimators
深度 depth
Depth算法通过提取原始图片中的深度信息 ,能够生成和原图一样深度结构的深度图。其中图片颜色越浅(白)的区域,代表距离镜头越近;越是偏深色(黑)的区域,则代表距离镜头越远。
Depth算法一共有四种预处理器,分别是depth_leres,depth_leres++,depth_midas和depth_zoe。
- depth_leres预处理器的成像焦点在中间景深层,这样的好处是能有更远的景深,且中距离物品边缘成像会更清晰,但近景图像的边缘会比较模糊
LeReS 深度信息估算比 MiDaS 深度信息估算方法的成像焦点在中间景深层,这样的好处是能有更远的景深,且中距离物品边缘成像会更清晰,但近景图像的边缘会比较模糊,具体实战中需用哪个估算方法可根据需要灵活选择。
- depth_leres++预处理器在depth_leres预处理器的基础上做了优化,能够有更多的细节
- depth_midas预处理器是经典的深度估计器,也是最常用的深度估计器。
MiDaS 深度信息估算,是用来控制空间距离的,类似生成一张深度图。一般用在较大纵深的风景,可以更好表示纵深的远近关系。
- depth_zoe预处理效果在细节层次方面在Midas和Leres模型之间取得平衡。
- Depth Anything:一种新的、改进的深度估计模型,专为各种场景而设计。
- Depth Hand Refiner:专门设计用于改善深度图中的手部细节,适用于手部定位至关重要的场景。
预处理器 | 预处理器 Node | 模型 | 场景 |
---|---|---|---|
depth_midas(\custom_nodes\comfyui_controlnet_aux\ckpts\lllyasviel/Annotators\dpt_hybrid-midas-501f0c75.pt) | MiDaS Depth Map | control_v11f1p_sd15_depth, control_depth, t2iadapter_depth_sd15v,t2iadapter_depth_sd14v, coadapter-depth-sd15v1 | |
depth_zoe | Zoe Depth Map | control_v11f1p_sd15_depth, control_depth, t2iadapter_depth_sd15v,t2iadapter_depth_sd14v, coadapter-depth-sd15v1 | |
depth_leres, depth_leres++ | LeReS Depth Map | control_v11f1p_sd15_depth, control_depth, t2iadapter_depth_sd15v,t2iadapter_depth_sd14v, coadapter-depth-sd15v1 | |
depth anything | Depth Anything, Zoe Depth Anything | Depth-Anything | |
Metric3D Depth | control_v11f1p_sd15_depth, control_depth, t2iadapter_depth_sd15v,t2iadapter_depth_sd14v, coadapter-depth-sd15v1 | ||
Depth Hand Refiner | MeshGraphormer_Hand_Refiner |
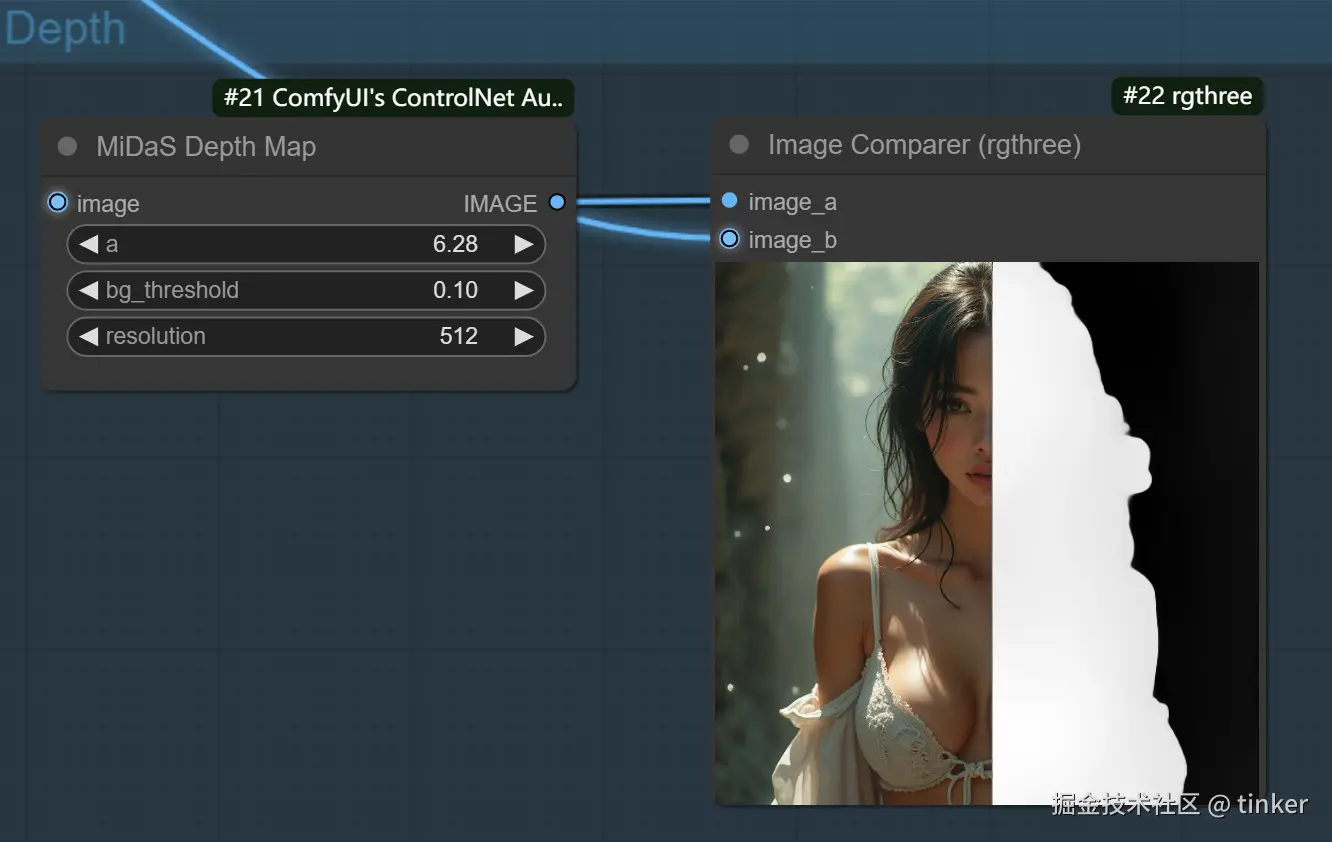
法线 Normal
NormalMap算法根据输入图片生成一张记录凹凸纹理信息的法线贴图,通过提取输入图片中的3D法线向量,并以法线为参考重新生成一张新图,同时给图片内容进行更好的光影处理。法线向量图指定了一个表面的方向。在ControlNet中,它是一张指定每个像素所在表面方向的图像,法线向量图像素代表的不是颜色值,而是表面的朝向。
NormalMap算法的用法与Depth算法类似,都是用于传递参考图像的三维结构特征。NormalMap算法与Depth算法相比在保持几何形状方面有更好的效果,在深度图中很多细微细节并不突出,但在法线图中则比较明显。法线贴图在游戏制作领域用的较多,常用于贴在低模上模拟高模的复杂光影效果,非常适合CG建模师。
NormalMap算法一共有两种预处理器,分别是normal_bae预处理器和normal_midas预处理器,
- Normal Bae:这种方法通过利用法线不确定性方法生成法线贴图。它提供了一种创新的技术来描绘表面的方向,增强了基于场景的物理几何而不是传统的基于颜色的方法的光照效果模拟。
- Normal Midas:利用Midas模型生成的深度图,Normal Midas可以准确估计法线贴图。这种方法允许基于场景的深度信息对表面纹理和光照进行细微模拟,从而丰富3D模型的视觉复杂性。
预处理器 | 预处理器 Node | 模型 | 场景 |
---|---|---|---|
normal_bae | BAE Normal Map | control_v11p_sd15_normalbase | |
normal_midas | MiDaS Normal Map | control_normal | |
DSINE Normal Map | control_v11p_sd15_normalbase, control_normal | ||
Metric3D Normal Map | control_v11p_sd15_normalbase |
手部深度 MeshGraphormer
- 预处理
- MeshGraphormer Hand Refiner(HandRefinder)
- 模型
Faces and Poses Estimators
姿态 Pose
OpenPose算法包含了实时的人体关键点检测模型,通过姿势识别,能够提取人体姿态,如人脸、手、腿和身体等位置关键点信息,从而达到精准控制人体动作 。除了生成单人的姿势,它还可以生成多人的姿势,此外还有手部骨骼模型,解决手部绘图不精准问题。
OpenPose算法一共有六种预处理器,分别是OpenPose,OpenPose_face,OpenPose_faceonly,OpenPose_full,openpose_hand,dw_openpose_full。
每种OpenPose预处理器的具体效果如下:
- OpenPose预处理器是OpenPose算法中最基础的预处理器,能够识别图像中人物的整体骨架(眼睛、鼻子、眼睛、脖子、肩膀、肘部、腕部、膝盖和脚踝等)
- OpenPose_face预处理器效果是在OpenPose预处理器的基础上增加脸部关键点的检测与识别
- OpenPose_faceonly预处理器仅检测脸部的关键点信息,如果我们想要固定脸部,改变其他部位的特征的话,可以使用此预处理器
- openpose_full预处理器能够识别图像中人物的整体骨架+脸部关键点+手部关键点,是一个非常全面的预处理器
- openpose_hand预处理器能够识别图像中人物的整体骨架+手部关键点
- dw_openpose_full预处理器是目前OpenPose算法中最强的预处理器,是OpenPose_full预处理器的增强版,使用了传统深度学习中的王牌检测模型yolox_l作为人体关键点的检测base模型,其不但能够人物的整体骨架+脸部关键点+手部关键点,而且精细程度也比openpose_full预处理器更好
DensePose 支持自动下载模型,也可以手动下载模型文件,放置下面目录
shell
Failed to find E:\vendor\cv\stablediffusion\ComfyUI-master\ComfyUI-master\custom_nodes\comfyui_controlnet_aux\ckpts\LayerNorm/DensePose-TorchScript-with-hint-image\densepose_r50_fpn_dl.torchscript.
Downloading from huggingface.co
DW 支持自动下载模型,也可以手动下载模型文件,放置下面目录
- custom_nodes\comfyui_controlnet_aux\ckpts\yzd-v/DWPose\yolox_l.onnx
- custom_nodes\comfyui_controlnet_aux\ckpts\hr16/DWPose-TorchScript-BatchSize5\dw-ll_ucoco_384_bs5.torchscript.pt
shell
Failed to find E:\vendor\cv\stablediffusion\ComfyUI-master\ComfyUI-master\custom_nodes\comfyui_controlnet_aux\ckpts\yzd-v/DWPose\yolox_l.onnx.
Downloading from huggingface.co
Failed to find E:\vendor\cv\stablediffusion\ComfyUI-master\ComfyUI-master\custom_nodes\comfyui_controlnet_aux\ckpts\hr16/DWPose-TorchScript-BatchSize5\dw-ll_ucoco_384_bs5.torchscript.pt.
Downloading from huggingface.co
cacher folder is C:\Users\leoli\AppData\Local\Temp, you can change it by custom_tmp_path in config.yaml
预处理器 | 预处理器 Node | 模型 | 场景 |
---|---|---|---|
dw_openpose_full | DWPose Estimator | control_v11p_sd15_openpose, control_openpose, t2iadapter_openpose_sd14v1 | |
openpose (detect_body) openpose_hand (detect_body + detect_hand) openpose_faceonly (detect_face) openpose_full (detect_hand + detect_body + detect_face)(custom_nodes\comfyui_controlnet_aux\ckpts\lllyasviel/Annotators\body_pose_model.pth, custom_nodes\comfyui_controlnet_aux\ckpts\lllyasviel/Annotators\hand_pose_model.pth, ustom_nodes\comfyui_controlnet_aux\ckpts\lllyasviel/Annotators\facenet.pth) | OpenPose Estimator | control_v11p_sd15_openpose, control_openpose, t2iadapter_openpose_sd14v1 | |
animal_openpose | Animal Estimator | control_sd15_animal_openpose_fp16 | |
mediapipe_face | MediaPipe Face Mesh | controlnet_sd21_laion_face_v2 | |
DensePose Estimator |
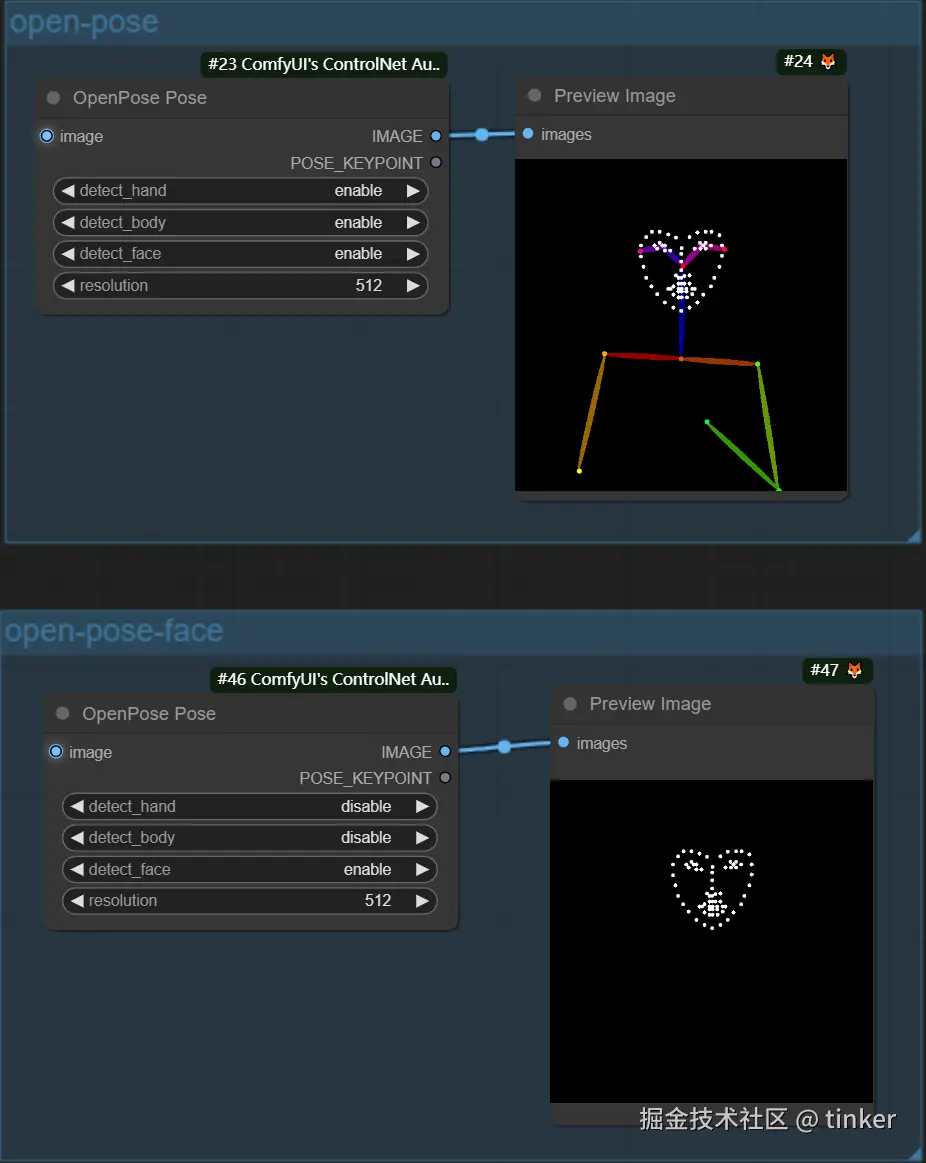
Semantic Segmentation
分割 Segmentor
Segmentation算法是传统深度学习三大支柱(分类,分割,检测)核心之一 ,主要通过对图片内容(人物、背景、建筑等)进行语义分割,可以区分画面色块,适用于大场景的画风更改。
但是输入图像的所有精细细节和深度特征都会丢失,与此同时会生成多个与输入图像中的物体的形状基本保持一致的mask(掩膜)。ControlNet中的Segmentation算法天然地能够与SD系列模型的inpatinting相结合使用,后者需要输入mask,并对mask部分进行局部重绘,而Segmentation算法就能够自动提供相应的mask部分。
Segmentation 预处理器,分别是 seg_ofade20k 预处理器、seg_ofcoco 预处理器和seg_ufade20k 预处理器
- Seg:旨在通过颜色区分图像中的对象,有效地将这些区别转化为输出中的不同元素。例如,它可以分离房间布局中的家具,这使其对于需要精确控制图像构图和编辑的项目特别有价值。
- ufade20k:利用在ADE20K数据集上训练的UniFormer分割模型,能够以高精度区分广泛的对象类型。
- ofade20k:采用OneFormer分割模型,也在ADE20K上训练,以其独特的分割能力提供另一种对象区分方法。
- ofcoco:利用在COCO数据集上训练的OneFormer分割,针对COCO数据集参数内分类的对象图像进行定制,有助于精确的对象识别和操作。
预处理器 | 预处理器 Node | 模型 | 场景 |
---|---|---|---|
seg_ofade20k, | OneFormer ADE20K Segmentor(custom_nodes\comfyui_controlnet_aux\ckpts\lllyasviel/Annotators\250_16_swin_l_oneformer_ade20k_160k.pth) | control_v11p_sd15_seg | |
seg_ofcoco | OneFormer COCO Segmentor(custom_nodes\comfyui_controlnet_aux\ckpts\lllyasviel/Annotators\150_16_swin_l_oneformer_coco_100ep.pth) | control_v11p_sd15_seg | |
Seg, seg_ufade20k | UniFormer Segmentor(custom_nodes\comfyui_controlnet_aux\ckpts\lllyasviel/Annotators\upernet_global_small.pth) | control_v11p_sd15_seg, control_sd15_seg | |
Anime Face Segmentor(\custom_nodes\comfyui_controlnet_aux\ckpts\bdsqlsz\qinglong_controlnet-lllite\Annotators\UNet.pth,\custom_nodes\comfyui_controlnet_aux\ckpts\skytnt/anime-seg\isnetis.ckpt, \custom_nodes\comfyui_controlnet_aux\ckpts\torch\mobilenet_v2-b0353104.pth) |
shell
Downloading from huggingface.co cacher folder is C:\Users\leoli\AppData\Local\Temp, you can change it by custom_tmp_path in config.yaml
mobile_sam.pt: custom_nodes\comfyui_controlnet_aux\ckpts\dhkim2810/MobileSAM\mobile_sam.pt
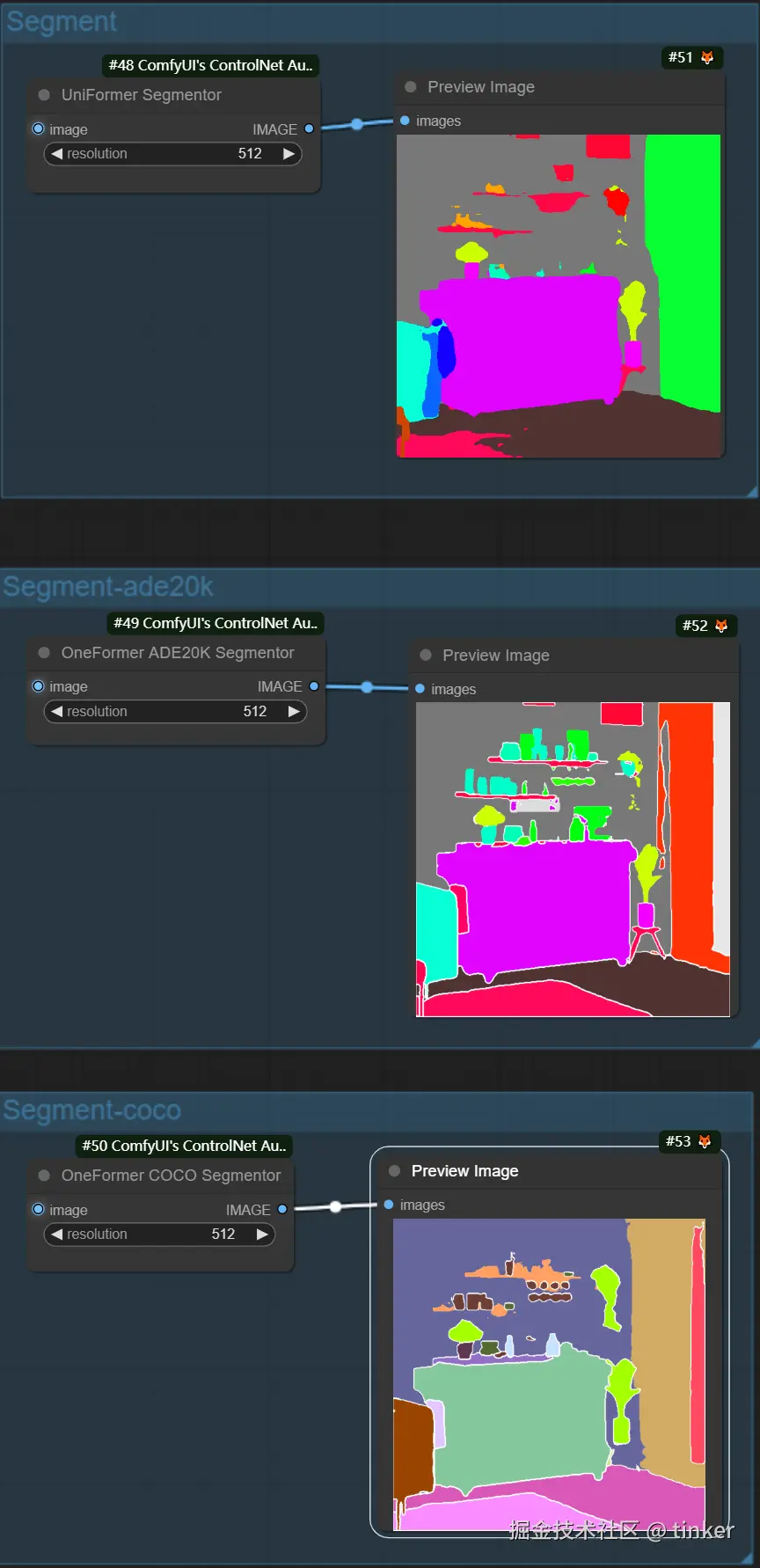
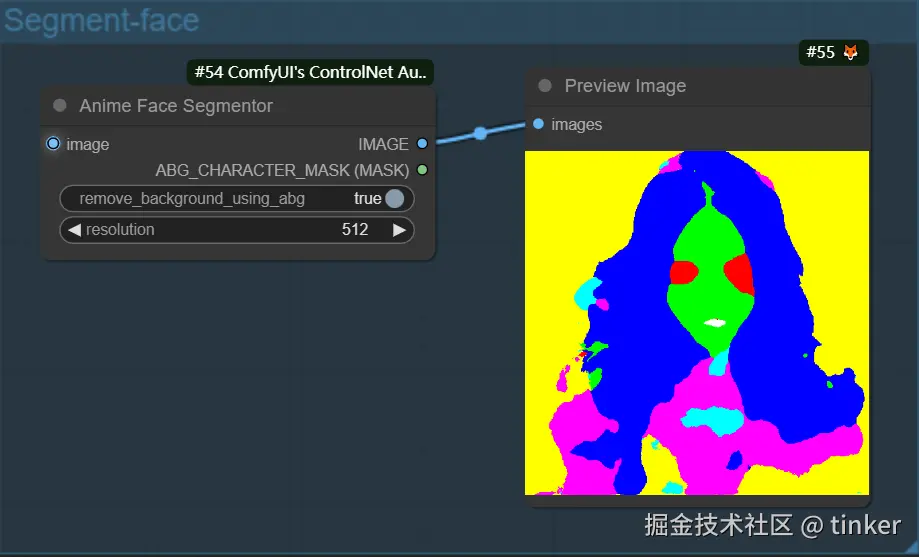
图生图
Inpaint 局部重绘
ControlNet Inpaint算法与Stable DIffusion系列模型原生的Inapinting操作一样,使用mask对需要重绘的部分进行遮盖,然后进行局部的图像重新生成。
ControlNet Inpaint模型是用50%随机mask和50%随机光流mask共同训练的。这意味着模型不仅支持常规的图像重绘应用,还可以处理视频光流变形任务。
与此同时,ControlNet Inpaint算法也可以进行扩充重绘(outpainting),比如说将人物半身图片扩充重绘成全身图片,将风景画的内容扩展补充,得到一个更大尺寸的图像。社交平台上时不时火一阵的AI扩图,其核心技术就是通过ControlNet Inpaint来实现。
目前ControlNet Inpaint算法中包含了三个预处理器,分别是 inpaint_global_harmonious 预处理器,inpaint_only 预处理器以及 inpaint_only+lama 预处理器。
ControlNet Inpaint模型目前只有 control_v11p_sd15_inpaint 一种,其对应的配置文件为control_v11p_sd15_inpaint.yaml。
预处理器 | 预处理器 Node | 模型 | 场景 |
---|---|---|---|
inpaint_global_harmonious, inpaint_only | Inpaint Preprocessor | control_v11p_sd15_inpaint |
Tile 高清修复,细节修复
ControlNet中的Tile算法和超分算法部分类似,能够增大图像的分辨率。但不同的是,ControlNet Tile算法在增加图像分辨率的同时,还能生成大量的细节特征而不是简单地进行插值。
总的来说,ControlNet中Tile算法有两种使用方法:
- 在图片尺寸不变的情况下,优化生成图片的细节。
- 在对图片尺寸进行超分的同时,生成相应的细节,完善超分后的图片效果。
由于Tile算法可以生成新的细节,因此我们可以使用该算法去除不良细节并添加更精致的细节。例如,消除因图像超分或者尺寸变化而导致的图像细节模糊的问题。
目前Tile算法一共有三种预处理器,分别是 tile_resample 预处理器、tile_colorfix+sharp预处理器以及 tile_colorfix 预处理器。
目前Tile算法一共有两个模型,分别是 control_v11u_sd15_tile 模型和 control_v11f1e_sd15_tile 模型。
预处理器 | 预处理器 Node | 模型 | 场景 |
---|---|---|---|
tile_resample, tile_colorfix | Tile | control_v11u_sd15_tile, control_v11f1e_sd15_tile |
InstryctP2P
ControlNet InstryctP2P 算法是一种通过提示词编辑图像的算法,在传统深度学习时代,著名的以GAN为核心的Pix2Pix模型就是图像编辑算法的代表。ControlNet InstryctP2P模型是在Instruct Pix2Pix数据集上进行训练的。ControlNet InstryctP2P算法不包含预处理器,目前只有一个control_v11e_sd15_ip2p模型。整体控制精度一般,适合抽空,偏娱乐。
- 模型
- control_v11e_sd15_ip2p
Recolor
Recolor算法主要起到对输入图像进行重新上色的效果。目前Recolor算法有两个预处理器,分别是recolor_intensity预处理器和recolor_luminance预处理器。
recolor_intensity预处理器在提取图像特征时更注重颜色的饱和度。而recolor_luminance预处理器在提取图像特征时更注重颜色的亮度,通常情况下选用recolor_intensity预处理器效果更好。
目前Recolor算法一共包含了三个ControlNet模型,分别是 ioclab_sd15_recolor,sai_xl_recolor_128lora 和 sai_xl_recolor_256lora。
其中 sai_xl_recolor_128lora 和 sai_xl_recolor_256lora 模型是两个匹配 SDXL 的 control-LoRA 模型。通过将低秩参数高效微调加入到ControlNet中,训练了Control-LoRAs模型。Control-LoRAs模型比起原生的ControlNet模型推理速度更快。
同时Recolor算法还有一个关键参数Gamma Correction(伽玛校正)。Gamma Correction(伽马校正)是一种在成像系统中用于校正或调整图像亮度或颜色的非线性操作。其主要目的是优化图像数据的使用,以符合人类对亮度和颜色的感知,确保图像在不同显示设备上的准确呈现。Gamma Correction默认设置为1,如果感觉生成的图像较暗就调小一点,如果感觉生成的图像过亮,就调大一点。
预处理器 | 预处理器 Node | 模型 | 场景 |
---|---|---|---|
recolor_intensity | Image Intensity | ioclab_sd15_recolor, bdsqlsz_controlllite_xl_recolor_luminance | |
recolor_luminance | Image Luminance | ioclab_sd15_recolor,bdsqlsz_controlllite_xl_recolor_luminance |
T2IAdapter-only
T2I-Adapter算法是由腾讯发布,和ControlNet模型一样,能够作为控制条件控制SD模型生成图片的过程。
color
预处理器 | 预处理器 Node | 模型 | 场景 |
---|---|---|---|
Color Pallete | t2iadapter_color_sd14v1, coadapter-color-sd15v1 |
Shuffle
预处理器 | 预处理器 Node | 模型 | 场景 |
---|---|---|---|
Content Shuffle | t2iadapter_style_sd14v1, coadapter-style-sd15v1 |
Optical Flow Estimators
预处理器 | 预处理器 Node | 模型 | 场景 |
---|---|---|---|
Unimatch Optical Flow | DragNUWA |
Reference-only使用详解
Reference-only算法可以说是CotrolNet系列算法中的一个"异类",它只有预处理器,没有对应的ControlNet模型,也就是说它不需要进行训练,而是一个纯算法。
Reference-only算法的预处理器能够直接使用输入的图像作为参考图 (图像提示词) 来控制SD模型的生成过程,类似于inpainting操作,从而可以生成与参考图像相似的图像。与此同时,SD模型在图像生成过程中仍会受到Prompt的约束与引导。
Reference-only算法的实现原理是通过将SD U-Net的自注意力模块与参考图像进行映射来实现的,我们先将参考图像加噪声送入U-Net中,提取在SelfAttention模块中的keys和values并与SD模型原本的特征叠加,这样一来就结合了自身特征和参考图特征,从而实现了无训练的参考图像特征作为图像提示词功能。
Reference-only算法一共包含三种预处理器,分别是reference_adain预处理器、reference_adain+attn预处理器和reference_only预处理器。
需要注意的是:Reference-only算法兼容Stable Diffusion 1.x-2.x和Stable Diffusion XL模型。
目前Reference-only算法能够完美兼容Stable Diffusion 1.x-2.x和Stable Diffusion XL模型。对于Stable Diffusion 3和FLUX.1系列模型,由于是基于Transformer架构,所以暂时还不适用,不过相信未来开源社区会及时跟进适配。
更多关于Reference-only算法的干货知识,Rocky为大家进行了汇总梳理,大家可以直接阅读:
- Reference-only/discussions
- github.com/huggingface...
- [New Preprocessor] The "reference_adain" and "reference_adain+attn" are added · Mikubill/sd-webui-controlnet · Discussion #1280
在ControlNet的Reference-only算法开源后,AIGC时代的很多领域都借鉴了其"即插即用"的思想,比如说视频动作控制、语音驱动的人像视频生成、虚拟试装、保ID人像生成、人像多视角合成等,逐渐成为了AIGC时代可控生成方法中的基石思想。
Revision使用详解
ControlNet里的Revision算法主要是在控制的过程中加入的"底图",它用池化的CLIP Embedding来生成与输入"底图"概念相似的图像。 Revision算法可以单独使用于SD系列模型的生成,也可以与提示词Prompt组合使用。
需要注意的是:Revision算法兼容Stable Diffusion和Stable Diffusion XL模型。
目前Revision算法一共有两种预处理器,分别是revision_clipvision预处理器和revision_ignore_prompt预处理器。
与此同时,Revision算法并不需要对应的ControlNet模型,因为其主要是对输入的图像进行处理,提取Embedding特征。
IP-Adapter
Stable Diffusion系列模型在正常情况下是只支持文本提示词的输入,而IP-Adapter算法能够在SD模型的图像生成过程中引入图像提示词(Image Prompt) ,从而能够识别输入图像的风格和内容,然后控制SD模型生成相似风格或者内容的图片,同时也可以搭配其他类型的ControlNet一起使用。
可以说IP-Adapter能让SD模型临摹艺术大师的作品,并且用在我们生成的图片中,在AI绘画开源社区中,大家给IP-Adapter算法的功能起了一个形象的名字:"垫图" 。
那么IP-Adapter算法和Stable Diffusion模型结合主要能干哪些有价值的事情呢?
- IP-Adapter算法可以同时使用图像提示词和文本提示词,引导图像的生成。
- IP-Adapter算法可以用于图生图以及图像inpainting。
- IP-Adapter算法与Stable Diffusion和Stable Diffusion XL模型同时适配,并且可以与其他ControlNet模型组合使用(T2I-Adapter)。
IP-Adapter算法一共有两个预处理器,分别是ip-adapter_clip_sd15预处理器(用于SD模型)和ip-adapter_clip_sdxl预处理器(SDXL模型)。
IP-Adapter模型一共有三个,分别是:
-
ip-adapter_sd15:适用于Stable Diffusion 1.5模型。
-
ip-adapter_sd15_plus:适用于 Stable Diffusion 1.5模型,能够细节更丰富的图像提示词,生成的图片和图像提示词的内容和风格更相似。
-
ip-adapter_xl:适用于 Stable Diffusion XL模型。
ComfyUI 插件
Fannovel16/comyfui_controlnet_aux
GitHub - Fannovel16/comfyui_controlnet_aux: ComfyUI's ControlNet Auxiliary Preprocessors
Plug-and-play ComfyUI node sets for making ControlNet hint images
The code is copy-pasted from the respective folders in github.com/lllyasviel/... and connected to the 🤗 Hub.
Please note that this repo only supports preprocessors making hint images (e.g. stickman, canny edge, etc). All preprocessors except Inpaint are intergrated into AIO Aux Preprocessor
node. This node allow you to quickly get the preprocessor but a preprocessor's own threshold parameters won't be able to set. You need to use its node directly to set thresholds.
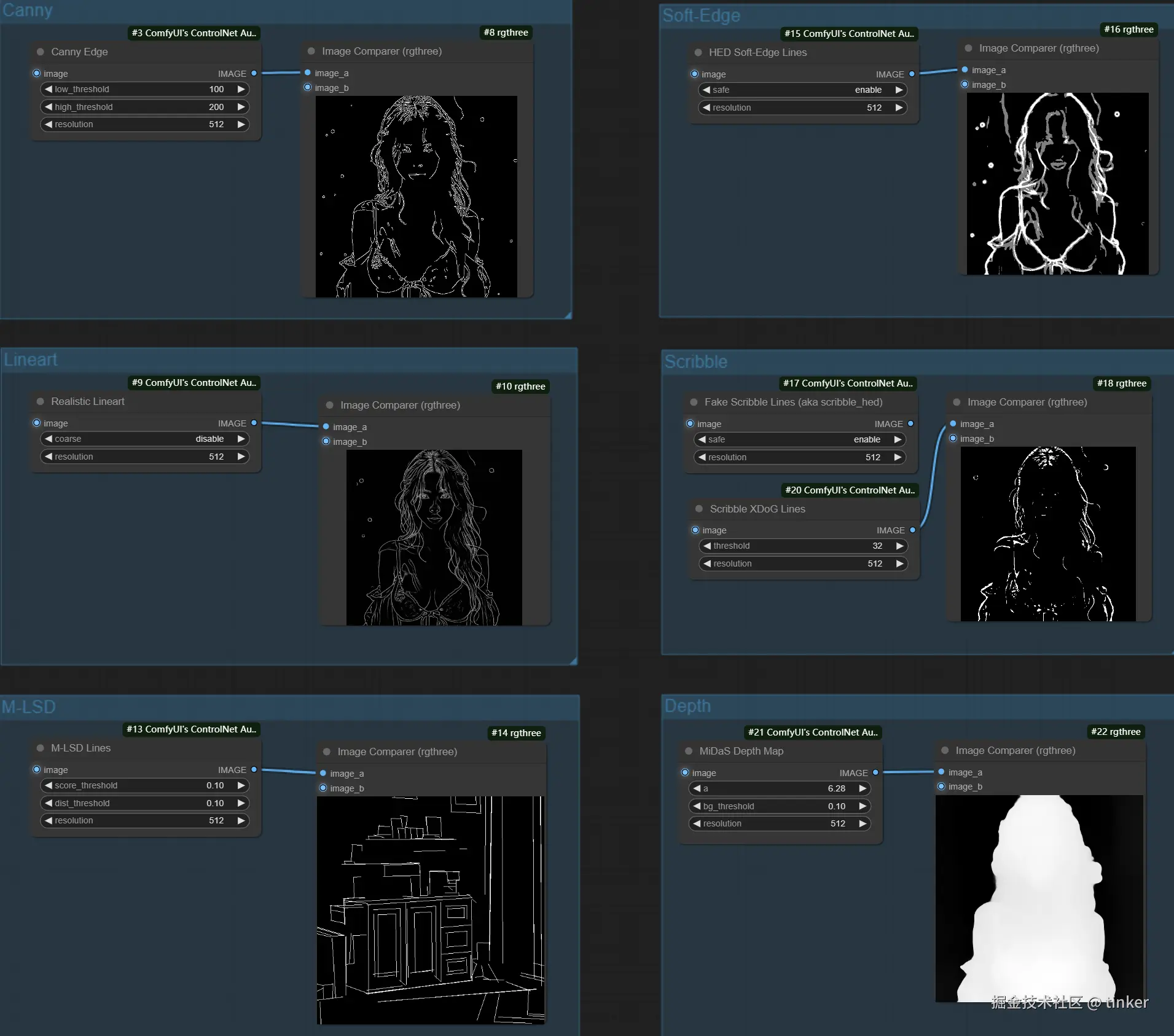
Kosinkadink/ComfyUI-Advanced-ControlNet
Nodes for scheduling ControlNet strength across timesteps and batched latents, as well as applying custom weights and attention masks. The ControlNet nodes here fully support sliding context sampling, like the one used in the ComfyUI-AnimateDiff-Evolved nodes. Currently supports ControlNets, T2IAdapters, ControlLoRAs, ControlLLLite, SparseCtrls, SVD-ControlNets, and Reference.
Custom weights allow replication of the "My prompt is more important" feature of Auto1111's sd-webui ControlNet extension.
ControlNet preprocessors are available through comfyui_controlnet_aux nodes
Features
- Timestep and latent strength scheduling
- Attention masks
- Soft weights to replicate "My prompt is more important" feature from sd-webui ControlNet extension, and also change the scaling
- ControlNet, T2IAdapter, and ControlLoRA support for sliding context windows
- ControlLLLite support (requires model_optional to be passed into and out of Apply Advanced ControlNet node)
- SparseCtrl support
- SVD-ControlNet support
- Reference support
- Supports
reference_attn
,reference_adain
, andrefrence_adain+attn
modes.style_fidelity
andref_weight
are equivalent to style_fidelity and control_weight in Auto1111, respectively, and strength of the Apply ControlNet is the balance between ref-influenced result and no-ref result. There is also a Reference ControlNet (Finetune) node that allows adjust the style_fidelity, weight, and strength of attn and adain separately.
- Supports
如何使用ComfyUI ControlNet:高级特性 - 时间步关键帧
ControlNet中的时间步关键帧在AI生成内容的行为控制方面提供了复杂的控制,特别是在时间和进展至关重要的情况下,如动画或不断发展的视觉效果。以下是关键参数的详细分解,以帮助你有效且直观地使用它们:
prev_timestep_kf:可以将prev_timestep_kf视为与序列中之前的关键帧连接。通过连接关键帧,你可以创建一个平滑过渡或分镜板,引导AI逐步完成生成过程,确保每个阶段都合乎逻辑地流入下一个阶段。
cn_weights:cn_weights有助于通过在生成过程的不同阶段调整ControlNet内的特定特征来微调输出。
latent_keyframe:latent_keyframe允许你在生成过程的特定阶段调整AI模型的每个部分对最终结果的影响强度。例如,如果你要生成一个图像,其中前景在过程演变中应变得更加细节化,你可以在后面的关键帧中增加负责前景细节的模型方面(潜在因素)的强度。相反,如果某些特征应随时间逐渐淡入背景,你可以在后续关键帧中降低它们的强度。这种控制级别在创建动态、不断发展的视觉效果或精确时间和进展至关重要的项目中特别有用。
mask_optional:使用注意力遮罩作为聚光灯,将ControlNet的影响集中在图像的特定区域。无论是突出场景中的角色还是强调背景元素,这些遮罩可以统一应用,也可以改变强度,精确地将AI的注意力引导到你想要的地方。
start_percent:start_percent标记你的关键帧开始发挥作用的时间点,以整个生成过程的百分比来衡量。设置这个就像安排演员在舞台上的登场时间,确保他们在表演中恰到好处地出现。
strength:strength提供对ControlNet整体影响的高级控制。
null_latent_kf_strength:对于在此场景(关键帧)中没有明确指示的任何演员(潜在因素),null_latent_kf_strength作为默认指令,告诉他们如何在背景中表现。它确保生成的任何部分都不会没有指导,即使在你没有具体提到的区域也能保持连贯的输出。
inherit_missing:激活inherit_missing允许你的当前关键帧采用其前身未指定的任何设置,就像弟弟妹妹继承衣服一样。它是一个有用的快捷方式,可以在不需要重复指令的情况下确保连续性和一致性。
guarantee_usage:guarantee_usage是你的保证,无论如何,当前关键帧在过程中都会有发挥作用的时刻,即使只是短暂的一瞬间。它确保你设置的每个关键帧都会产生影响,尊重你在引导AI创作过程中的详细规划。
时间步关键帧提供了精确引导AI创作过程所需的精度,使你能够完全按照自己的设想来编排视觉的演变,特别是在动画中,从开场到结尾。下面我们将更仔细地看时间步关键帧如何战略性地应用于管理动画的进展,确保从初始帧到最后一帧的无缝过渡,与你的艺术目标完美契合。
TheMistoAI/ComfyUI-Anyline
MistoLine: A Versatile and Robust SDXL-ControlNet Model for Adaptable Line Art Conditioning.
MistoLine is an SDXL-ControlNet model that can adapt to any type of line art input, demonstrating high accuracy and excellent stability. It can generate high-quality images (with a short side greater than 1024px) based on user-provided line art of various types, including hand-drawn sketches, different ControlNet line preprocessors, and model-generated outlines. MistoLine eliminates the need to select different ControlNet models for different line preprocessors, as it exhibits strong generalization capabilities across diverse line art conditions.
We developed MistoLine by employing a novel line preprocessing algorithm Anyline and retraining the ControlNet model based on the Unet of stabilityai/ stable-diffusion-xl-base-1.0, along with innovations in large model training engineering. MistoLine showcases superior performance across different types of line art inputs, surpassing existing ControlNet models in terms of detail restoration, prompt alignment, and stability, particularly in more complex scenarios.
The model is compatible with most SDXL models, except for PlaygroundV2.5, CosXL, and SDXL-Lightning(maybe). It can be used in conjunction with LCM and other ControlNet models.
A Fast, Accurate, and Detailed Line Detection Preprocessor Anyline is a ControlNet line preprocessor that accurately extracts object edges, image details, and textual content from most images. Users can input any type of image to quickly obtain line drawings with clear edges, sufficient detail preservation, and high fidelity text, which are then used as input for conditional generation in Stable Diffusion.
Upon installation, the Anyline preprocessor can be accessed in ComfyUI via search or right-click. The standard workflow using Anyline+Mistoline in SDXL is as follows
模型
- AnyLine * Anyline 模型地址:hf-mirror.com/TheMistoAI/... * 中国(大陆地区)便捷下载地址 (MTEED.pth):链接:pan.baidu.com/s/1ik11P_u1... 提取码:v8f1
- MistoLine * MistoLine 模型下载地址:TheMistoAI/MistoLine · HF Mirror (hf-mirror.com)
EeroHeikkinen/ComfyUI-eesahesNodes
Important update regarding InstantX Union Controlnet: The latest version of ComfyUI now includes native support for the InstantX/Shakkar Labs Union Controlnet Pro, which produces higher quality outputs than the alpha version this loader supports.
Flux Controlnet 模型
XLabs-AI
XLabs-AI/flux-controlnet-collections 是一个为 FLUX.1-dev 模型提供的 ControlNet 检查点集合。这个仓库由 Black Forest Labs 开发,旨在为 Flux 生态系统提供更多的控制选项。
使用 1024x1024 分辨率训练,支持三类模型,分别是 Canny ControlNet , Depth ControlNet , HED ControlNet for FLUX.1 [dev]
。已经迭代到 V3 版本,更好,更真实的版本。
下载地址:
All
Canny
Depth
Hed
InstantX&Shakker-Labs
InstantX Flux Union ControlNet 是一个多功能的 ControlNet 模型,专为 FLUX.1 开发版设计。这个模型整合了多种控制模式,使用户能够更灵活地控制图像生成过程。
主要特点:
- 多种控制模式:支持多种控制模式,包括 Canny 边缘检测、Tile(图块)、深度图、模糊、姿势控制等。
- 高性能:大多数控制模式都达到了较高的有效性,特别是 Canny、Tile、深度图、模糊和姿势控制等模式。
- 持续优化:开发团队正在不断改进模型,以提高其性能和稳定性。
- 兼容性:与 FLUX.1 开发版基础模型完全兼容,可以轻松集成到现有的 FLUX 工作流程中。
- 多控制推理:支持同时使用多个控制模式,为用户提供更精细的图像生成控制。
下载地址
- InstantX&Shakker-Labs 联合发布 ControlNet Union Pro 模型:Shakker-Labs/FLUX.1-dev-ControlNet-Union-Pro
- This checkpoint is a Pro version of FLUX.1-dev-Controlnet-Union trained with more steps and datasets.
- This model supports 7 control modes, including canny (0), tile (1), depth (2), blur (3), pose (4), gray (5), low quality (6).
- The recommended controlnet_conditioning_scale is 0.3-0.8.
- This model can be jointly used with other ControlNets.
- InstantX&Shakker-Labs 联合发布 ControlNet Depth 模型: Shakker-Labs/FLUX.1-dev-ControlNet-Depth · HF Mirror
- The model consists of 4 FluxTransformerBlock and 1 FluxSingleTransformerBlock.
- This checkpoint is trained on both real and generated image datasets, with 16A800 for 70K steps. The batch size 16 4=64 with resolution=1024. The learning rate is set to 5e-6. We use Depth-Anything-V2 to extract depth maps.
- The recommended controlnet_conditioning_scale is 0.3-0.7.
- InstantX 独立发布 beta 版本模型: InstantX/FLUX.1-dev-Controlnet-Union
- InstantX 发布抢先版本模型: InstantX/FLUX.1-dev-Controlnet-Canny
- 专注于 Canny 边缘检测:这个模型专门针对 Canny 边缘检测进行了优化,可以更好地处理和利用边缘信息。
- 高分辨率训练 :模型在总像素数为 10241024 的多尺度环境下进行训练,使用 88 的批量大小训练了 30k 步。
- 与 FLUX.1 兼容:专为 FLUX.1 开发版设计,可以无缝集成到 FLUX 工作流程中。
- 使用 bfloat16 精度:模型使用 bfloat16 精度,可以在保持精度的同时提高计算效率
jasperai/flux1-dev-controlnets
Jasperai 为 Flux.1-dev 开发了一系列 ControlNet 模型,这些模型旨在为 AI 图像生成提供更精确的控制。这个系列包括表面法线、深度图和超分辨率模型,为用户提供了多样化的创作工具
模型地址: hf-mirror.com/collections...
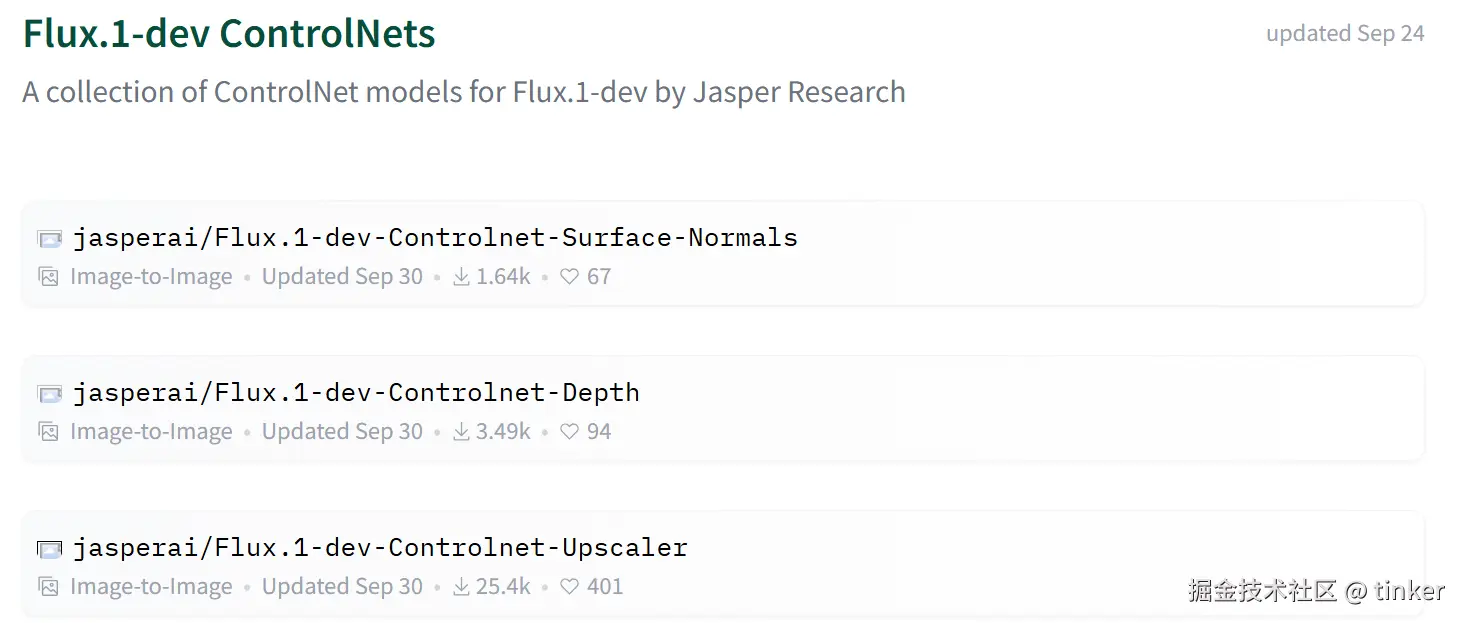
Flux MistoLine
模型下载: TheMistoAI/MistoLine_Flux.dev · HF Mirror
This is a ControlNet network designed for any lineart or outline sketches, compatible with Flux1.dev. The ControlNet model parameters are approximately 1.4B.
This model is not compatible with XLabs loaders and samplers. Please use TheMisto.ai Flux ControlNet ComfyUI suite. This is a Flow matching structure Flux-dev model, utilizing a scalable Transformer module as the backbone of this ControlNet.
We've implemented a dual-stream Transformer structure, which enhances alignment and expressiveness for various types of lineart and outline conditions without increasing inference time. The model has also been trained for alignment with both T5 and clip-l TextEncoders, ensuring balanced performance between conditioning images and text prompts.
For more details on the Flux.dev model structure, visit: hf-mirror.com/black-fores...
This ControlNet is compatible with Flux1.dev's fp16/fp8 and other models quantized with Flux1.dev. ByteDance 8/16-step distilled models have not been tested.
- The example workflow uses the flux1-dev-Q4_K_S.gguf quantized model.
- Generation quality: Flux1.dev(fp16)>>Flux1.dev(fp8)>>Other quantized models
- Generation speed: Flux1.dev(fp16)<<< Flux1.dev(fp8) <<< Other quantized models
Performance with Different Lineart or Scribble Preprocessors
Test Parameters:
- Prompt: "Hyper-realistic 3D render of a classic Formula 1 race car, bright red with Marlboro and Agip logos, number 1, large black tires, dramatic studio lighting, dark moody background, reflective floor, cinematic atmosphere, Octane render style, high detail"
- controlnet_strength: 0.65-0.8 (Recommended: Anyline with 0.6-0.7)
- steps: 30
- guidance: 4.0
- The quality of generated images is positively correlated with prompt quality. Controlnet_strength may vary for different types of lineart and outlines, so experiment with the settings!
Recommended Settings
- Image resolution: 720px or above on the short edge
- controlnet strength: 0.6 - 0.85 (adjust as needed)
- guidance: 3.0 - 5.0 (adjust as needed)
- steps: 30 or more
工作流
ComfyUI推荐参数:
- sampler steps:30
- CFG:7.0
- sampler_name:dpmpp_2m_sde
- scheduler:karras
- denoise:0.93
- controlnet_strength:1.0
- stargt_percent:0.0
- end_percent:0.9