从零开始构建微博爬虫与数据分析系统
引言
社交媒体平台蕴含着海量的信息和数据,通过对这些数据的收集和分析,我们可以挖掘出有价值的见解。本文将详细介绍如何构建一个完整的微博爬虫和数据分析系统,从数据爬取、清洗、到多维度分析与可视化。
系统架构
整个系统分为两个主要模块:
- 微博爬虫模块:负责通过API获取微博数据并保存
- 数据分析模块:对获取的数据进行清洗和多维度分析
一、微博爬虫实现
1.1 爬虫设计思路
微博的数据爬取主要基于其Ajax接口,通过模拟浏览器请求获取JSON格式数据。主要挑战在于:
- 需要登录凭证(Cookie)才能访问完整内容
- 接口限制和反爬措施
- 数据格式的解析与清洗
1.2 核心代码实现
WeiboCrawler类是爬虫的核心,主要包含以下功能:
python
class WeiboCrawler:
def __init__(self, cookie=None):
# 初始化请求头和会话
self.headers = {...}
if cookie:
self.headers['Cookie'] = cookie
self.session = requests.Session()
self.session.headers.update(self.headers)
def get_user_info(self, user_id):
# 获取用户基本信息
url = f'https://weibo.com/ajax/profile/info?uid={user_id}'
# 实现...
def get_user_weibos(self, user_id, page=1, count=20):
# 获取用户微博列表
url = f'https://weibo.com/ajax/statuses/mymblog?uid={user_id}&page={page}&feature=0'
# 实现...
def crawl_user_weibos(self, user_id, max_pages=None):
# 爬取所有微博并返回结果
# 实现...
1.3 数据清洗与存储
爬取的原始数据需要进行清洗,主要包括:
- 去除HTML标签和特殊字符
- 提取时间、内容、图片链接等信息
- 识别转发内容并单独处理
清洗后的数据以结构化文本形式存储,便于后续分析:
python
def format_weibo(self, weibo):
# 格式化微博内容为易读格式
created_at = datetime.strptime(weibo['created_at'], '%a %b %d %H:%M:%S %z %Y')
text = self.clean_text(weibo.get('text', ''))
formatted = f"[{created_at.strftime('%Y-%m-%d %H:%M:%S')}]\n{text}\n"
# 处理转发内容、图片链接等
# ...
return formatted
二、数据分析模块
2.1 数据加载与预处理
WeiboAnalyzer类负责从文本文件加载微博数据,并转换为结构化形式:
python
def load_data(self):
# 从文件加载微博数据
with open(self.file_path, 'r', encoding='utf-8') as f:
lines = f.readlines()
# 提取用户信息和微博内容
# ...
print(f"成功加载 {len(self.weibos)} 条微博")
2.2 时间分布分析
分析微博发布的时间规律,包括日期、小时和星期分布:
python
def time_distribution_analysis(self):
# 提取日期和时间
dates = [weibo['date'].date() for weibo in self.weibos]
hours = [weibo['date'].hour for weibo in self.weibos]
weekdays = [weibo['date'].weekday() for weibo in self.weibos]
# 使用pandas和matplotlib进行统计和可视化
# ...
通过这一分析,我们可以了解用户在什么时间段最活跃,是否有固定的发布模式。
2.3 内容分析与关键词提取
使用jieba分词和TF-IDF算法提取微博内容的关键词:
python
def content_analysis(self):
# 合并所有微博内容
all_content = ' '.join([weibo['content'] for weibo in self.weibos])
# 使用jieba进行分词
jieba.analyse.set_stop_words('stopwords.txt')
words = jieba.cut(all_content)
# 过滤单个字符和数字
filtered_words = [word for word in words if len(word) > 1 and not word.isdigit()]
# 统计词频
word_counts = Counter(filtered_words)
# 提取关键词
keywords = jieba.analyse.extract_tags(all_content, topK=50, withWeight=True)
# 生成词云和关键词图表
# ...
词云能直观地展示内容主题,关键词分析则揭示了用户最关注的话题。
2.4 引用人物分析
分析微博中引用的名人或专家:
python
def quote_analysis(self):
# 定义可能被引用的人物列表
famous_people = [
'曾国藩', '尼采', '荣格', '苏格拉底', '马云', '武志红',
'阿德勒', '王安石', '苏东坡', '海德格尔', '左宗棠', '宗萨'
]
# 统计每个人物被引用的次数
quotes = {person: 0 for person in famous_people}
for weibo in self.weibos:
content = weibo['content']
for person in famous_people:
if person in content:
quotes[person] += 1
# 绘制引用人物条形图
# ...
这一分析可以揭示用户的思想倾向和崇拜的对象。
2.5 图片使用分析
分析微博中的图片使用情况:
python
def image_analysis(self):
# 统计带图片的微博数量
weibos_with_images = [weibo for weibo in self.weibos if weibo['images']]
image_counts = [len(weibo['images']) for weibo in weibos_with_images]
# 计算统计数据
total_weibos = len(self.weibos)
weibos_with_images_count = len(weibos_with_images)
percentage = weibos_with_images_count / total_weibos * 100 if total_weibos > 0 else 0
# 绘制饼图和分布图
# ...
三、可视化报告生成
最终,将所有分析结果整合为一个HTML报告:
python
def generate_report(self):
# 执行所有分析
self.time_distribution_analysis()
self.content_analysis()
self.quote_analysis()
self.image_analysis()
# 生成HTML报告
html_content = f"""
<!DOCTYPE html>
<html>
<head>
<meta charset="UTF-8">
<title>微博数据分析报告</title>
<style>
body {{ font-family: Arial, sans-serif; margin: 20px; }}
h1, h2 {{ color: #1DA1F2; }}
.section {{ margin-bottom: 30px; }}
img {{ max-width: 100%; border: 1px solid #ddd; }}
</style>
</head>
<body>
<h1>微博数据分析报告</h1>
<!-- 各部分分析结果 -->
<!-- ... -->
</body>
</html>
"""
with open('weibo_analysis_report.html', 'w', encoding='utf-8') as f:
f.write(html_content)
四、实际应用案例
以用户"侯小强"(ID: 1004524612)为例,我爬取了其全部1069条微博并进行分析。以下是一些关键发现:
- 时间分布:该用户主要在晚上8点至10点发布微博,周六和周日活跃度明显高于工作日
- 关键词分析:心理、生活、思考是最常出现的关键词,表明用户关注心理学和个人成长话题
- 引用分析:尼采、荣格、苏格拉底是被最多引用的人物,表明用户对西方哲学有较深兴趣
- 图片使用:约37%的微博包含图片,其中以单图发布为主
网页展示效果如下:
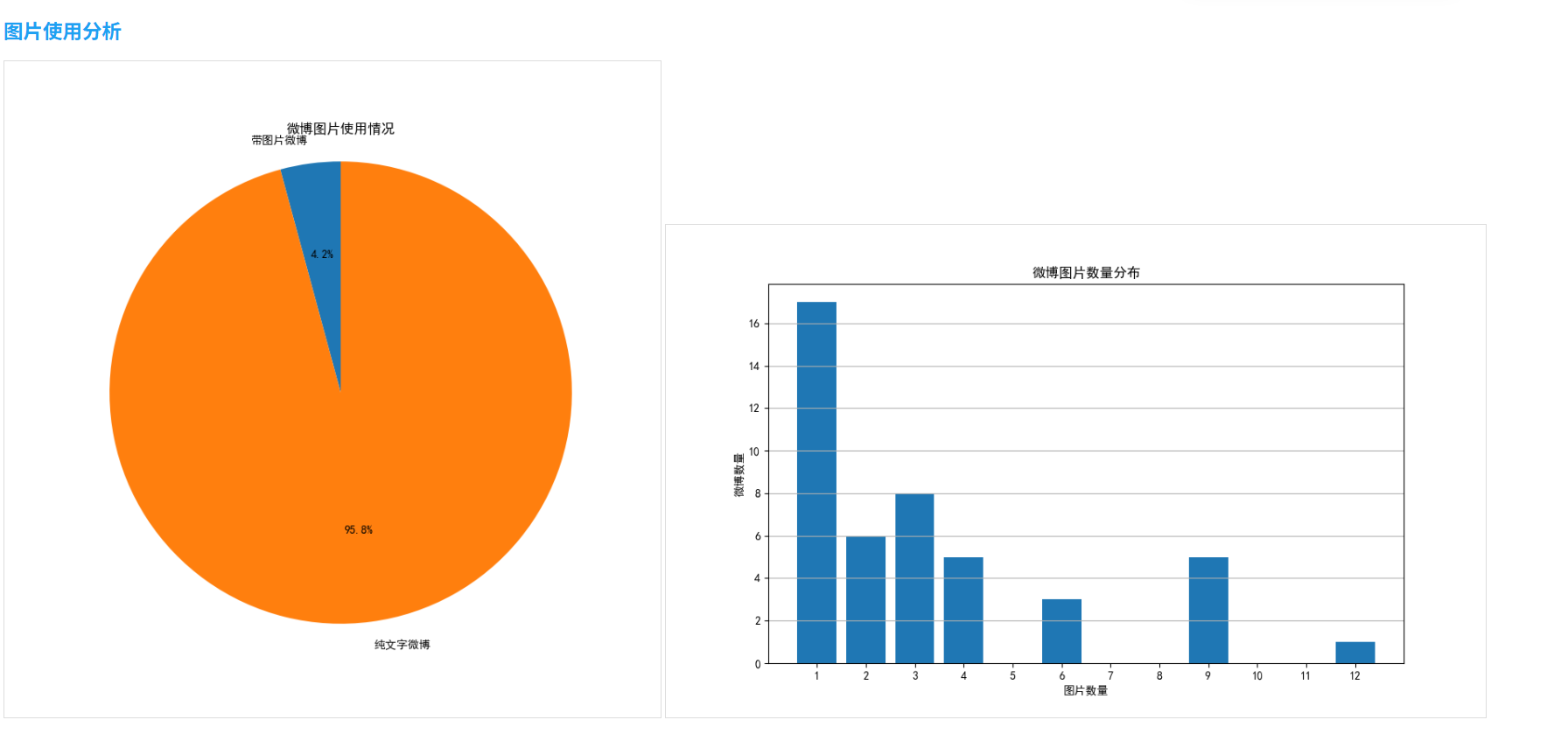
五、技术难点与解决方案
- 反爬虫机制:微博有严格的请求频率限制,我通过设置合理的请求间隔(1秒)和会话保持来解决
- 中文分词挑战:中文分词准确度对内容分析至关重要,使用jieba库并自定义停用词表提高分析质量
- 数据清洗:微博内容中包含大量HTML标签和特殊字符,需要精心设计正则表达式进行清洗
- 可视化定制:调整matplotlib的中文字体和样式设置,确保图表美观且信息丰富
六、总结与展望
本项目实现了一个完整的微博数据爬取和分析系统,可以帮助我们从用户的微博内容中挖掘出有价值的信息。未来的改进方向包括:
- 支持多用户批量爬取和对比分析
- 加入情感分析功能,评估微博的情感倾向
- 增加互动数据(点赞、评论、转发)的分析
- 开发时间序列分析,检测用户兴趣变化趋势
通过这个项目,我们不仅可以了解特定用户的发布规律和内容偏好,还能窥探社交媒体用户的思想动态和关注重点,为社会学和心理学研究提供数据支持。
完整代码:爬取数据代码-weibo_crawler.py
python
import requests
import json
import time
import os
import re
import argparse
from datetime import datetime
class WeiboCrawler:
def __init__(self, cookie=None):
"""
初始化微博爬虫
:param cookie: 用户登录的cookie字符串
"""
self.headers = {
'User-Agent': 'Mozilla/5.0 (Windows NT 10.0; Win64; x64) AppleWebKit/537.36 (KHTML, like Gecko) Chrome/91.0.4472.124 Safari/537.36',
'Accept': 'application/json, text/plain, */*',
'Accept-Language': 'zh-CN,zh;q=0.9',
'Referer': 'https://weibo.com/',
'Origin': 'https://weibo.com',
}
if cookie:
self.headers['Cookie'] = cookie
self.session = requests.Session()
self.session.headers.update(self.headers)
def get_user_info(self, user_id):
"""
获取用户基本信息
:param user_id: 用户ID
:return: 用户信息字典
"""
url = f'https://weibo.com/ajax/profile/info?uid={user_id}'
try:
response = self.session.get(url)
if response.status_code == 200:
data = response.json()
if data.get('ok') == 1 and 'data' in data:
return data['data']['user']
return None
except Exception as e:
print(f"获取用户信息失败: {e}")
return None
def get_user_weibos(self, user_id, page=1, count=20):
"""
获取用户的微博列表
:param user_id: 用户ID
:param page: 页码
:param count: 每页微博数量
:return: 微博列表
"""
url = f'https://weibo.com/ajax/statuses/mymblog?uid={user_id}&page={page}&feature=0'
try:
response = self.session.get(url)
if response.status_code == 200:
data = response.json()
if data.get('ok') == 1 and 'data' in data:
return data['data']['list'], data['data']['total']
return [], 0
except Exception as e:
print(f"获取微博列表失败: {e}")
return [], 0
def clean_text(self, text):
"""
清理文本内容,去除HTML标签等
:param text: 原始文本
:return: 清理后的文本
"""
if not text:
return ""
# 去除HTML标签
text = re.sub(r'<[^>]+>', '', text)
# 替换特殊字符
text = text.replace(' ', ' ')
text = text.replace('<', '<')
text = text.replace('>', '>')
text = text.replace('&', '&')
# 去除多余空格和换行
text = re.sub(r'\s+', ' ', text).strip()
return text
def format_weibo(self, weibo):
"""
格式化微博内容
:param weibo: 微博数据
:return: 格式化后的微博文本
"""
created_at = datetime.strptime(weibo['created_at'], '%a %b %d %H:%M:%S %z %Y').strftime('%Y-%m-%d %H:%M:%S')
text = self.clean_text(weibo.get('text', ''))
formatted = f"[{created_at}]\n"
formatted += f"{text}\n"
# 添加转发内容
if 'retweeted_status' in weibo and weibo['retweeted_status']:
retweeted = weibo['retweeted_status']
retweeted_user = retweeted.get('user', {}).get('screen_name', '未知用户')
retweeted_text = self.clean_text(retweeted.get('text', ''))
formatted += f"\n转发 @{retweeted_user}: {retweeted_text}\n"
# 添加图片链接
if 'pic_ids' in weibo and weibo['pic_ids']:
formatted += "\n图片链接:\n"
for pic_id in weibo['pic_ids']:
pic_url = f"https://wx1.sinaimg.cn/large/{pic_id}.jpg"
formatted += f"{pic_url}\n"
formatted += "-" * 50 + "\n"
return formatted
def crawl_user_weibos(self, user_id, max_pages=None):
"""
爬取用户的所有微博
:param user_id: 用户ID
:param max_pages: 最大爬取页数,None表示爬取全部
:return: 所有微博内容的列表
"""
user_info = self.get_user_info(user_id)
if not user_info:
print(f"未找到用户 {user_id} 的信息")
return []
screen_name = user_info.get('screen_name', user_id)
print(f"开始爬取用户 {screen_name} 的微博")
all_weibos = []
page = 1
total_pages = float('inf')
while (max_pages is None or page <= max_pages) and page <= total_pages:
print(f"正在爬取第 {page} 页...")
weibos, total = self.get_user_weibos(user_id, page)
if not weibos:
break
all_weibos.extend(weibos)
# 计算总页数
if total > 0:
total_pages = (total + 19) // 20 # 每页20条,向上取整
page += 1
# 防止请求过快
time.sleep(1)
print(f"共爬取到 {len(all_weibos)} 条微博")
return all_weibos, screen_name
def save_weibos_to_file(self, user_id, max_pages=None):
"""
爬取用户微博并保存到文件
:param user_id: 用户ID
:param max_pages: 最大爬取页数
:return: 保存的文件路径
"""
weibos, screen_name = self.crawl_user_weibos(user_id, max_pages)
if not weibos:
return None
# 创建文件名
filename = f"{user_id}_weibos.txt"
# 写入文件
with open(filename, 'w', encoding='utf-8') as f:
f.write(f"用户: {screen_name} (ID: {user_id})\n")
f.write(f"爬取时间: {datetime.now().strftime('%Y-%m-%d %H:%M:%S')}\n")
f.write(f"微博数量: {len(weibos)}\n")
f.write("=" * 50 + "\n\n")
for weibo in weibos:
formatted = self.format_weibo(weibo)
f.write(formatted)
print(f"微博内容已保存到文件: {filename}")
return filename
def main():
parser = argparse.ArgumentParser(description='微博爬虫 - 爬取指定用户的微博')
parser.add_argument('user_id', help='微博用户ID')
parser.add_argument('--cookie', help='登录cookie字符串', default=None)
parser.add_argument('--max-pages', type=int, help='最大爬取页数', default=None)
parser.add_argument('--cookie-file', help='包含cookie的文件路径', default=None)
args = parser.parse_args()
cookie = args.cookie
# 如果提供了cookie文件,从文件读取cookie
if args.cookie_file and not cookie:
try:
with open(args.cookie_file, 'r', encoding='utf-8') as f:
cookie = f.read().strip()
except Exception as e:
print(f"读取cookie文件失败: {e}")
crawler = WeiboCrawler(cookie=cookie)
crawler.save_weibos_to_file(args.user_id, args.max_pages)
if __name__ == "__main__":
main()
数据分析代码:weibo_analsis.py
python
import re
import os
import matplotlib.pyplot as plt
from datetime import datetime
import jieba
import jieba.analyse
from collections import Counter
import numpy as np
from wordcloud import WordCloud
import matplotlib.font_manager as fm
from matplotlib.font_manager import FontProperties
import pandas as pd
from matplotlib.dates import DateFormatter
import seaborn as sns
# 设置中文字体
plt.rcParams['font.sans-serif'] = ['SimHei'] # 用来正常显示中文标签
plt.rcParams['axes.unicode_minus'] = False # 用来正常显示负号
class WeiboAnalyzer:
def __init__(self, file_path):
"""
初始化微博分析器
:param file_path: 微博数据文件路径
"""
self.file_path = file_path
self.weibos = []
self.user_info = {}
self.load_data()
def load_data(self):
"""
加载微博数据
"""
with open(self.file_path, 'r', encoding='utf-8') as f:
lines = f.readlines()
# 提取用户信息
if lines and "用户:" in lines[0]:
user_info_match = re.match(r'用户: (.*) \(ID: (.*)\)', lines[0])
if user_info_match:
self.user_info['name'] = user_info_match.group(1)
self.user_info['id'] = user_info_match.group(2)
if len(lines) > 2 and "微博数量:" in lines[2]:
count_match = re.match(r'微博数量: (\d+)', lines[2])
if count_match:
self.user_info['count'] = int(count_match.group(1))
# 提取微博内容
current_weibo = None
for line in lines:
# 新微博的开始
if re.match(r'\[\d{4}-\d{2}-\d{2} \d{2}:\d{2}:\d{2}\]', line):
if current_weibo:
self.weibos.append(current_weibo)
date_match = re.match(r'\[(\d{4}-\d{2}-\d{2} \d{2}:\d{2}:\d{2})\]', line)
if date_match:
date_str = date_match.group(1)
content = line[len(date_str) + 3:].strip()
current_weibo = {
'date': datetime.strptime(date_str, '%Y-%m-%d %H:%M:%S'),
'content': content,
'images': [],
'is_retweet': False,
'retweet_content': '',
'retweet_user': ''
}
# 图片链接
elif line.strip().startswith('https://wx1.sinaimg.cn/'):
if current_weibo:
current_weibo['images'].append(line.strip())
# 转发内容
elif current_weibo and line.strip().startswith('转发 @'):
current_weibo['is_retweet'] = True
retweet_match = re.match(r'转发 @(.*): (.*)', line.strip())
if retweet_match:
current_weibo['retweet_user'] = retweet_match.group(1)
current_weibo['retweet_content'] = retweet_match.group(2)
# 继续添加内容
elif current_weibo and not line.strip() == '-' * 50 and not line.strip() == '=' * 50:
current_weibo['content'] += ' ' + line.strip()
# 添加最后一条微博
if current_weibo:
self.weibos.append(current_weibo)
print(f"成功加载 {len(self.weibos)} 条微博")
def time_distribution_analysis(self):
"""
分析微博发布时间分布
"""
if not self.weibos:
print("没有微博数据可分析")
return
# 提取日期和时间
dates = [weibo['date'].date() for weibo in self.weibos]
hours = [weibo['date'].hour for weibo in self.weibos]
weekdays = [weibo['date'].weekday() for weibo in self.weibos]
# 创建日期DataFrame
df = pd.DataFrame({
'date': dates,
'hour': hours,
'weekday': weekdays
})
# 按日期统计
date_counts = df['date'].value_counts().sort_index()
# 按小时统计
hour_counts = df['hour'].value_counts().sort_index()
# 按星期几统计
weekday_counts = df['weekday'].value_counts().sort_index()
weekday_names = ['周一', '周二', '周三', '周四', '周五', '周六', '周日']
# 创建图表
fig, axes = plt.subplots(3, 1, figsize=(12, 15))
# 日期分布图
axes[0].plot(date_counts.index, date_counts.values, marker='o')
axes[0].set_title('微博发布日期分布')
axes[0].set_xlabel('日期')
axes[0].set_ylabel('微博数量')
axes[0].grid(True)
# 小时分布图
axes[1].bar(hour_counts.index, hour_counts.values)
axes[1].set_title('微博发布时间段分布')
axes[1].set_xlabel('小时')
axes[1].set_ylabel('微博数量')
axes[1].set_xticks(range(0, 24))
axes[1].grid(True)
# 星期几分布图
axes[2].bar([weekday_names[i] for i in weekday_counts.index], weekday_counts.values)
axes[2].set_title('微博发布星期分布')
axes[2].set_xlabel('星期')
axes[2].set_ylabel('微博数量')
axes[2].grid(True)
plt.tight_layout()
plt.savefig('time_distribution.png')
plt.close()
print("时间分布分析完成,结果已保存为 time_distribution.png")
def content_analysis(self):
"""
分析微博内容
"""
if not self.weibos:
print("没有微博数据可分析")
return
# 合并所有微博内容
all_content = ' '.join([weibo['content'] for weibo in self.weibos])
# 使用jieba进行分词
jieba.analyse.set_stop_words('stopwords.txt') # 如果有停用词表
words = jieba.cut(all_content)
# 过滤掉单个字符和数字
filtered_words = [word for word in words if len(word) > 1 and not word.isdigit()]
# 统计词频
word_counts = Counter(filtered_words)
# 提取关键词
keywords = jieba.analyse.extract_tags(all_content, topK=50, withWeight=True)
# 创建词云
wordcloud = WordCloud(
font_path='simhei.ttf', # 设置中文字体
width=800,
height=400,
background_color='white'
).generate_from_frequencies(dict(word_counts))
# 绘制词云图
plt.figure(figsize=(10, 6))
plt.imshow(wordcloud, interpolation='bilinear')
plt.axis('off')
plt.title('微博内容词云')
plt.savefig('wordcloud.png')
plt.close()
# 绘制关键词条形图
plt.figure(figsize=(12, 8))
keywords_dict = dict(keywords[:20])
plt.barh(list(reversed(list(keywords_dict.keys()))),
list(reversed(list(keywords_dict.values()))))
plt.title('微博内容关键词TOP20')
plt.xlabel('权重')
plt.tight_layout()
plt.savefig('keywords.png')
plt.close()
print("内容分析完成,结果已保存为 wordcloud.png 和 keywords.png")
def quote_analysis(self):
"""
分析微博中引用的人物
"""
if not self.weibos:
print("没有微博数据可分析")
return
# 定义可能被引用的人物列表
famous_people = [
'曾国藩', '尼采', '荣格', '苏格拉底', '马云', '武志红',
'阿德勒', '王安石', '苏东坡', '海德格尔', '左宗棠', '宗萨'
]
# 统计每个人物被引用的次数
quotes = {person: 0 for person in famous_people}
for weibo in self.weibos:
content = weibo['content']
for person in famous_people:
if person in content:
quotes[person] += 1
# 过滤掉未被引用的人物
quotes = {k: v for k, v in quotes.items() if v > 0}
# 按引用次数排序
sorted_quotes = dict(sorted(quotes.items(), key=lambda item: item[1], reverse=True))
# 绘制引用人物条形图
plt.figure(figsize=(10, 6))
plt.bar(sorted_quotes.keys(), sorted_quotes.values())
plt.title('微博中引用人物统计')
plt.xlabel('人物')
plt.ylabel('引用次数')
plt.xticks(rotation=45)
plt.tight_layout()
plt.savefig('quotes.png')
plt.close()
print("引用人物分析完成,结果已保存为 quotes.png")
def image_analysis(self):
"""
分析微博中的图片使用情况
"""
if not self.weibos:
print("没有微博数据可分析")
return
# 统计带图片的微博数量
weibos_with_images = [weibo for weibo in self.weibos if weibo['images']]
image_counts = [len(weibo['images']) for weibo in weibos_with_images]
# 计算统计数据
total_weibos = len(self.weibos)
weibos_with_images_count = len(weibos_with_images)
percentage = weibos_with_images_count / total_weibos * 100 if total_weibos > 0 else 0
# 绘制饼图
plt.figure(figsize=(8, 8))
plt.pie([weibos_with_images_count, total_weibos - weibos_with_images_count],
labels=['带图片微博', '纯文字微博'],
autopct='%1.1f%%',
startangle=90)
plt.title('微博图片使用情况')
plt.axis('equal')
plt.savefig('image_usage.png')
plt.close()
# 绘制图片数量分布
if image_counts:
plt.figure(figsize=(10, 6))
counter = Counter(image_counts)
plt.bar(counter.keys(), counter.values())
plt.title('微博图片数量分布')
plt.xlabel('图片数量')
plt.ylabel('微博数量')
plt.xticks(range(1, max(image_counts) + 1))
plt.grid(axis='y')
plt.savefig('image_count.png')
plt.close()
print("图片使用分析完成,结果已保存为 image_usage.png 和 image_count.png")
def generate_report(self):
"""
生成分析报告
"""
# 执行所有分析
self.time_distribution_analysis()
self.content_analysis()
self.quote_analysis()
self.image_analysis()
# 生成HTML报告
html_content = f"""
<!DOCTYPE html>
<html>
<head>
<meta charset="UTF-8">
<title>微博数据分析报告</title>
<style>
body {{ font-family: Arial, sans-serif; margin: 20px; }}
h1, h2 {{ color: #1DA1F2; }}
.section {{ margin-bottom: 30px; }}
img {{ max-width: 100%; border: 1px solid #ddd; }}
</style>
</head>
<body>
<h1>微博数据分析报告</h1>
<div class="section">
<h2>用户信息</h2>
<p>用户名: {self.user_info.get('name', '未知')}</p>
<p>用户ID: {self.user_info.get('id', '未知')}</p>
<p>微博总数: {self.user_info.get('count', len(self.weibos))}</p>
<p>分析微博数: {len(self.weibos)}</p>
</div>
<div class="section">
<h2>时间分布分析</h2>
<img src="time_distribution.png" alt="时间分布分析">
</div>
<div class="section">
<h2>内容分析</h2>
<h3>词云</h3>
<img src="wordcloud.png" alt="词云">
<h3>关键词</h3>
<img src="keywords.png" alt="关键词">
</div>
<div class="section">
<h2>引用人物分析</h2>
<img src="quotes.png" alt="引用人物分析">
</div>
<div class="section">
<h2>图片使用分析</h2>
<img src="image_usage.png" alt="图片使用情况">
<img src="image_count.png" alt="图片数量分布">
</div>
</body>
</html>
"""
with open('weibo_analysis_report.html', 'w', encoding='utf-8') as f:
f.write(html_content)
print("分析报告已生成: weibo_analysis_report.html")
def main():
# 创建停用词文件(如果需要)
stopwords = [
'的', '了', '在', '是', '我', '有', '和', '就', '不', '人', '都',
'一', '一个', '上', '也', '很', '到', '说', '要', '去', '你', '会',
'着', '没有', '看', '好', '自己', '这', '那', '啊', '吧', '把', '给',
'但是', '但', '还', '可以', '这个', '这样', '这些', '因为', '所以',
'如果', '就是', '么', '什么', '只是', '只有', '这种', '那个', '他们'
]
with open('stopwords.txt', 'w', encoding='utf-8') as f:
f.write('\n'.join(stopwords))
# 分析微博数据
analyzer = WeiboAnalyzer('1004524612_weibos.txt')
analyzer.generate_report()
if __name__ == "__main__":
main()
所有数据以及代码也放在下面的仓库里了:源码链接
参考资料
- Python爬虫实战指南
- 《数据可视化之美》
- 自然语言处理与文本挖掘技术
- jieba中文分词官方文档