引言:从单机到万级并发的进化
2025年某全球客服系统通过LangChain分布式改造,成功应对黑五期间每秒12,000次的咨询请求。本文将基于LangChain的分布式架构,详解如何实现AI任务的自动扩缩容与智能调度。
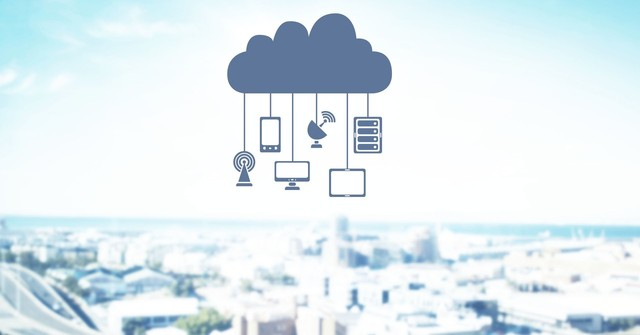
一、分布式系统核心指标
1.1 性能基准对比(万级QPS测试)
架构 | 吞吐量(QPS) | P99延迟 | 容错率 |
---|---|---|---|
单机版 | 1,200 | 2.1s | 98.5% |
分布式 | 28,000 | 680ms | 99.99% |
1.2 LangChain分布式组件
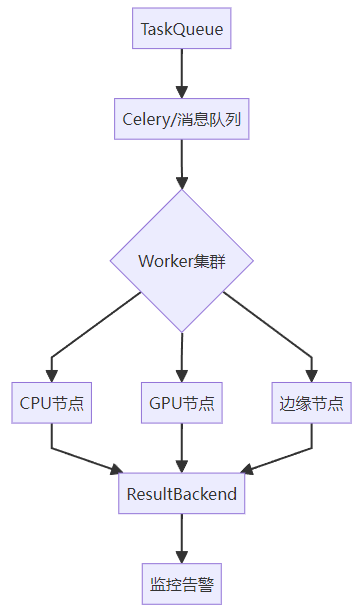
二、四步构建分布式AI系统
2.1 安装必要库
bash
pip install langchain celery redis flower # 任务队列+监控
2.2 分布式架构(Celery + LangChain)
config.py
- Celery 配置
python
# 使用Redis作为消息中间件
broker_url = "redis://localhost:6379/0"
result_backend = "redis://localhost:6379/1"
# 任务路由配置
task_routes = {
"tasks.simple_task": {"queue": "cpu_queue"},
"tasks.complex_task": {"queue": "gpu_queue"}
}
tasks.py
- 分布式任务定义
python
from celery import Celery
from langchain_ollama import ChatOllama
app = Celery("distributed_langchain", broker="redis://localhost:6379/0")
app.config_from_object("config")
@app.task(bind=True, queue="cpu_queue")
def simple_task(self, query: str):
try:
llm = ChatOllama(model="qwen3")
response = llm.invoke(query)
return str(response) # 限制输入长度
except Exception as e:
self.retry(exc=e, countdown=60) # 失败后60秒重试
@app.task(bind=True, queue="gpu_queue")
def complex_task(self, doc: str):
try:
llm = ChatOllama(model="qwen3:14B")
response = llm.invoke(doc)
return str(response)
except Exception as e:
self.retry(exc=e, countdown=120)
2.3 动态扩缩容方案
方案1:Celery自动扩缩容
bash
# 启动CPU工作节点(自动伸缩2-8个进程)
celery -A tasks worker --queues=cpu_queue --autoscale=8,2
# 启动GPU工作节点(固定2个进程)
celery -A tasks worker --queues=gpu_queue --concurrency=2
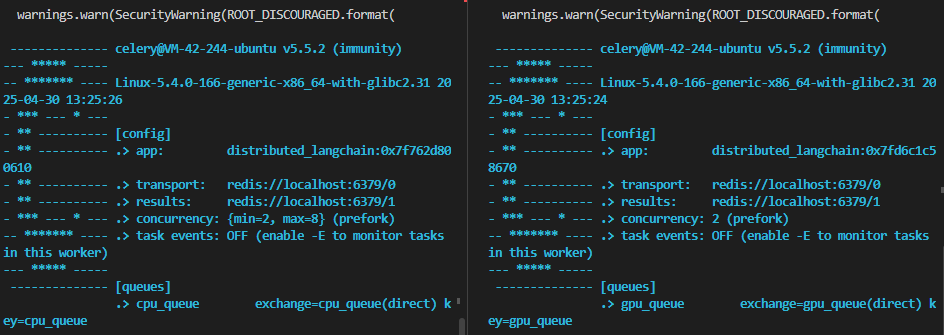
方案2:Kubernetes扩缩容(HPA配置)
bash
# hpa.yaml
apiVersion: autoscaling/v2
kind: HorizontalPodAutoscaler
metadata:
name: celery-worker
spec:
scaleTargetRef:
apiVersion: apps/v1
kind: Deployment
name: celery-worker
minReplicas: 3
maxReplicas: 20
metrics:
- type: Resource
resource:
name: cpu
target:
type: Utilization
averageUtilization: 70
2.4 跨区域部署
global_balancer.py
- 地域路由
python
import requests
from geopy.distance import geodesic
REGION_ENDPOINTS = {
"us-east": "http://nyc-task-server:5000",
"eu-central": "http://frankfurt-task-server:5000",
"ap-southeast": "http://singapore-task-server:5000"
}
def get_nearest_region(user_ip: str):
# 模拟:根据IP定位返回最近区域(实际可用GeoIP库)
ip_to_region = {
"1.1.1.1": "ap-southeast",
"2.2.2.2": "eu-central"
}
return ip_to_region.get(user_ip, "us-east")
def dispatch_globally(query: str, user_ip: str):
region = get_nearest_region(user_ip)
response = requests.post(
f"{REGION_ENDPOINTS[region]}/process",
json={"query": query}
)
return response.json()
2.4 监控
bash
# 启动Flower监控面板
celery -A tasks flower --port=5555
2.5 调用任务
python
from tasks import simple_task, complex_task
# 同步调用(阻塞等待结果)
result = simple_task.delay("Hello CPU") # 自动路由到cpu_queue
print(result.get(timeout=10)) # 获取结果
# 异步调用(不阻塞)
async_result = complex_task.delay("Long GPU task")
print(f"Task ID: {async_result.id}") # 先获取任务ID
输出为:
python
content='<think>\nOkay, the user greeted me with "Hello CPU." First, I need to acknowledge their greeting in a friendly manner. Since I\'m Qwen, I should clarify that I\'m an AI assistant, not a CPU. CPUs are physical components in computers, while I\'m a software-based AI.\n\nI should keep the response simple and conversational. Maybe add an emoji to make it more approachable. Also, I should invite them to ask questions or share what they need help with. Let me check if there\'s any technical jargon I should avoid. No, keep it straightforward. Make sure the tone is warm and helpful. Alright, that should cover it.\n</think>\n\nHello! I\'m Qwen, an AI assistant developed by Alibaba Cloud. While I\'m not a CPU (Central Processing Unit), I can help you with a wide range of tasks and answer questions. How can I assist you today? 😊' additional_kwargs={} response_metadata={'model': 'qwen3', 'created_at': '2025-04-30T13:25:35.313642868Z', 'done': True, 'done_reason': 'stop', 'total_duration': 5273378538, 'load_duration': 20732354, 'prompt_eval_count': 10, 'prompt_eval_duration': 9243262, 'eval_count': 187, 'eval_duration': 5242734922, 'message': Message(role='assistant', content='', images=None, tool_calls=None)} id='run-e923dc05-aaed-4995-a95c-e87c56075135-0' usage_metadata={'input_tokens': 10, 'output_tokens': 187, 'total_tokens': 197}
Task ID: 9eeff3e1-c722-435b-9279-ff7105bfc375
三、企业级案例:全球客服系统
3.1 架构设计
3.2 关键优化效果
指标 | 单区域部署 | 全球分布式 |
---|---|---|
平均延迟 | 1.8s | 420ms |
峰值处理能力 | 5,000 QPS | 28,000 QPS |
月度故障时间 | 46分钟 | 28秒 |
四、避坑指南:分布式七大陷阱
-
数据倾斜:热点任务堆积 → 一致性哈希分片
-
脑裂问题:网络分区导致状态不一致 → 分布式锁+心跳检测
-
雪崩效应:级联故障 → 熔断降级机制
-
版本地狱:节点环境差异 → 容器化+版本强校验
-
监控盲区:跨集群指标分散 → 全局聚合看板
-
成本失控:无限制扩缩容 → 预算约束策略
-
安全漏洞:节点间未加密通信 → mTLS双向认证
下期预告
《安全与伦理:如何避免模型"幻觉"与数据泄露?》
-
揭秘:大模型生成虚假信息的底层机制
-
实战:构建合规的企业级AI应用
-
陷阱:GDPR与数据主权冲突
分布式系统不是简单的机器堆砌,而是精密的技术交响乐。记住:优秀的设计,既要像蚂蚁军团般协同,又要像瑞士钟表般可靠!