Ernie 2.0: A Continual Pre-Training Framework for Language Understanding
Sun Y, Wang S, Li Y, et al. Ernie 2.0: A continual pre-training framework for language understanding[C]//Proceedings of the AAAI * Conference on Artificial Intelligence. 2020, 34(05): 8968-8975.
关键词:Continual Multi-task Learning
概括:加入更多的预训练任务,为了有效的训练采用了连续训练的方法。
以前的模型只能学到简单的共现信息,其他有价值的信息,比如lexical\syntactic\semantic information都没有被提取出来。
因此本文Continual Multi-task Learning、不同层次的预训练任务能够提取lexical\syntactic\semantic information。
1、Continual Multi-task Learning
连续的多任务学习能记住之前学习到的信息。
上游的预训练任务和下游特定任务的Fine-tuning的闭环。
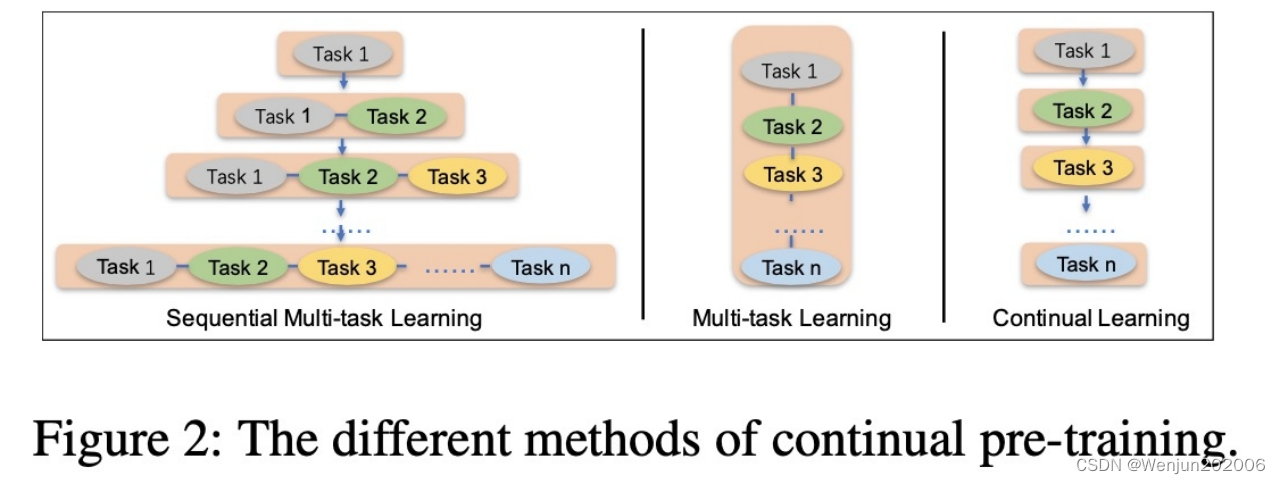
2、Pre-training Tasks
三个层次的预训练任务:
(1)Word-aware task: capture the lexical information
(2)Structure-aware task: capture the syntactic information
(3)Semantic-aware task: semantic information
2.1、Word-aware
- Knowledge Masking:同ERNIE 1.0的实体/短语 masking
- Capitalization Prediction:token大小写预测的任务
- Token-Document Relation Prediction:预测句子中的词是否出现在了segment原始文档中,约等于预测token是否为关键词
2.2、Structure-aware
- Sentence Reordering(语序关系):打乱k个句子,预测原始顺序(给每个句子做k分类)
- Sentence Distance(语义距离):3分类任务,预测两个句子是相连、出现在同一个文档还是在不同文档
2.3、Semantic-aware
- Discourse Relation:判断句子的语义关系,例如logical relationship (is a, has a, contract)
- IR Relevance Task: