连续登录问题是经典问题,今天做下总结。首先对原数据进行处理成客户和日期是不重复的,且日期是 yyyy-MM-dd 格式,这样好使用日期相关的函数。
本文参考在文末,增加了图表,更加容易理解。
表:temp01_cust_logon。
表字段和数据如下图的 A 和 B 列。
方法1: 利用窗口函数。
我们先对每个客户的登录日期做排序( 临时表:temp02_cust_logon2),然后对日期与排序的值进行相减得到 date_line( 临时表:temp03_cust_logon3)。因为如果是连续登录日期,那么减去连续的排序值就是相同的日期,再对相同的日期进行统计,超过3就是连续登录三天。
sql
-- 利用窗口函数
with temp02_cust_logon2 as
(
select
t1.kehu_id
,t1.date
,row_number () over (partition by t1.kehu_id order by t1.date) as rn
from
temp01_cust_logon as t1
)
,temp03_cust_logon3 as
(
select
t1.kehu_id
,t1.date
,t1.rn
,date_sub(t1.date,t1.rn) as date_line
from
temp02_cust_logon2 as t1
)
-- select * from temp03_cust_logon3
select
t1.kehu_id
,t1.date_line
,count(1) as cnt
from
temp03_cust_logon3 as t1
group by
t1.kehu_id
,t1.date_line
having
count(1) >= 3
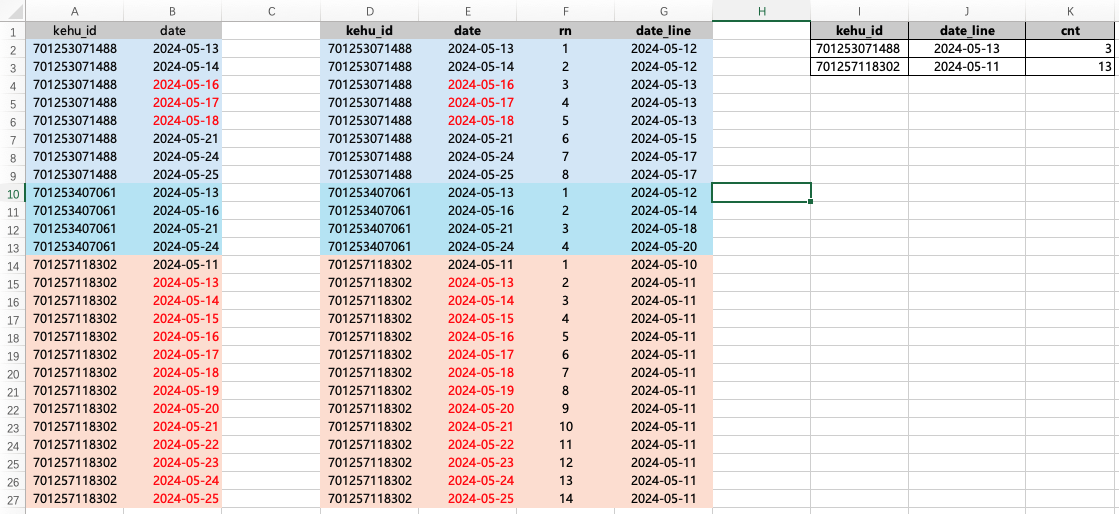
方法2:使用 lag (lead) 函数
首先看看这个函数如何使用。我本身数据是从20240510-20240525分区取的,所以使用这两个时间点来向前向后填充。
sql
select
t1.kehu_id
,t1.date
,row_number () over (partition by t1.kehu_id order by t1.date) as rn
,lead(t1.date,2) over (partition by t1.kehu_id order by t1.date asc) as lead_date1
,coalesce(lead(t1.date,2) over (partition by t1.kehu_id order by t1.date asc),'2024-05-25') as lead_date2
,coalesce(lead(t1.date,3) over (partition by t1.kehu_id order by t1.date asc),'2024-05-25') as lead_date3
,lag(t1.date,2) over (partition by t1.kehu_id order by t1.date asc) as lag_date1
,coalesce(lag(t1.date,2) over (partition by t1.kehu_id order by t1.date asc),'2024-05-10') as lag_date2
,coalesce(lag(t1.date,3) over (partition by t1.kehu_id order by t1.date asc),'2024-05-10') as lag_date3
from
temp01_cust_logon as t1
lead 函数是想后面的数据向前位移,最后的位移的位置出现 NULL,可以用 coalesce 填充。我用相同的颜色表示位移的数据,这样就很好理解了。同样,lag 函数是将最前面的数据空出来,出现 NULL。还有一种写法,将出现NULL的位置填充自己想写的内容,不需要 coalesce 。
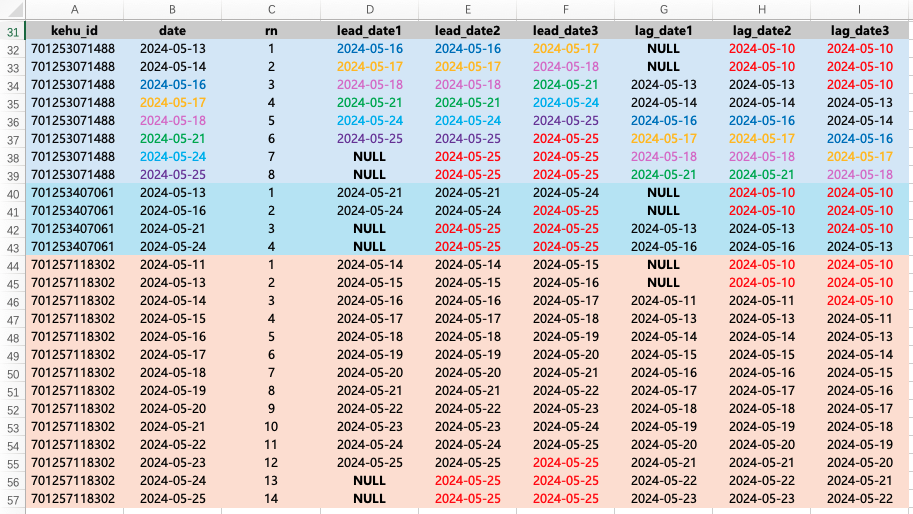
但是实际上我想用客户本身最早和最近登录时间来填充,就得先建立临时表。注意标记红色的数据,和上面的数据做对比。
sql
with temp01_cust_logon_minmax as
(
select
t1.kehu_id
,max(t1.date) as max_date
,min(t1.date) as min_date
from
temp01_cust_logon as t1
group by
t1.kehu_id
)
select
t1.kehu_id
,t1.date
,row_number () over (partition by t1.kehu_id order by t1.date) as rn
,lead(t1.date,2) over (partition by t1.kehu_id order by t1.date asc) as lead_date1
,coalesce(lead(t1.date,2) over (partition by t1.kehu_id order by t1.date asc),t2.max_date) as lead_date2
,coalesce(lead(t1.date,3) over (partition by t1.kehu_id order by t1.date asc),t2.max_date) as lead_date3
,lag(t1.date,2) over (partition by t1.kehu_id order by t1.date asc) as lag_date1
,coalesce(lag(t1.date,2) over (partition by t1.kehu_id order by t1.date asc),t2.min_date) as lag_date2
,coalesce(lag(t1.date,3) over (partition by t1.kehu_id order by t1.date asc),t2.min_date) as lag_date3
from
temp01_cust_logon as t1
left join
temp01_cust_logon_minmax as t2
on t1.kehu_id = t2.kehu_id
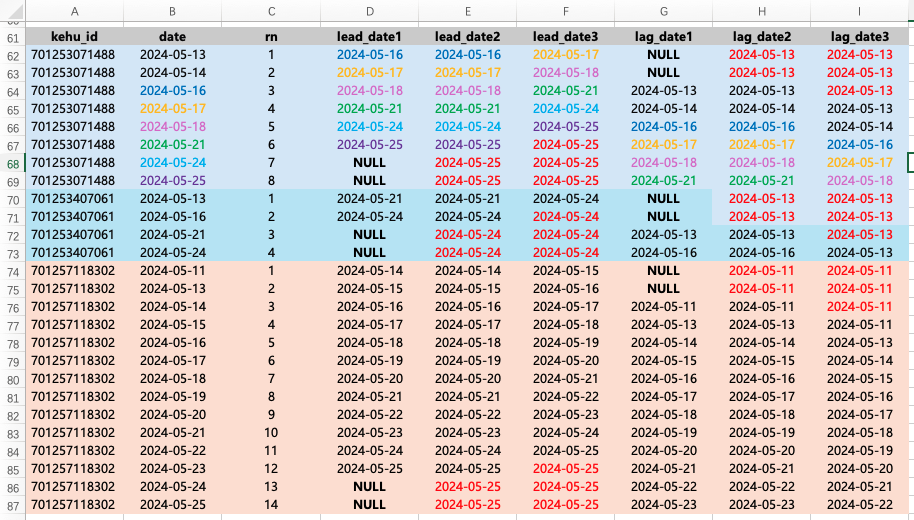
这是完整代码:我们对客户日期排序后,使用 lag 函数,这样就可以使用时间差函数计算。如果是连续登录,那么时间差是一样的。我们找的是连续登录三天,则找到出现 2 的时间差。然后再对时间差打标签,最后进行统计。
但是这里我们可以发现,20240513 这个最早登录日期被我人为填充后,时间差出现了异常,所以还是保留 NULL。我写了 date_diff2 ,date_line3 是我想要的标签字段,根据这个字段进行统计去重客户数。
sql
with temp01_cust_logon_minmax as
(
select
kehu_id
,max(date) as max_date
,min(date) as min_date
from
temp01_cust_logon
group by
kehu_id
)
,temp02_cust_logon2 as
(
select
t1.kehu_id
,t1.date
,row_number () over (partition by t1.kehu_id order by t1.date) as rn
,lag(t1.date,2,t2.min_date) over (partition by t1.kehu_id order by t1.date asc) as lag_date
,lag(t1.date,2,'0000-00-00') over (partition by t1.kehu_id order by t1.date asc) as lag_date2
from
temp01_cust_logon as t1
left join
temp01_cust_logon_minmax as t2
on t1.kehu_id = t2.kehu_id
)
-- select * from temp02_cust_logon2
,temp03_cust_logon3 as
(
select
t2.kehu_id
,t2.date
,t2.rn
,t2.lag_date
,date_diff(t2.date,t2.lag_date) as date_fiff
,case when date_diff(t2.date,t2.lag_date) = 2 then 1 else 0 end as date_line1
,if (date_diff(t2.date,t2.lag_date) = 2,1,0) as date_line2
,date_diff(t2.date,t2.lag_date2) as date_fiff2
,if (date_diff(t2.date,t2.lag_date2) = 2,1,0) as date_line3
from
temp02_cust_logon2 as t2
)
select * from temp03_cust_logon3
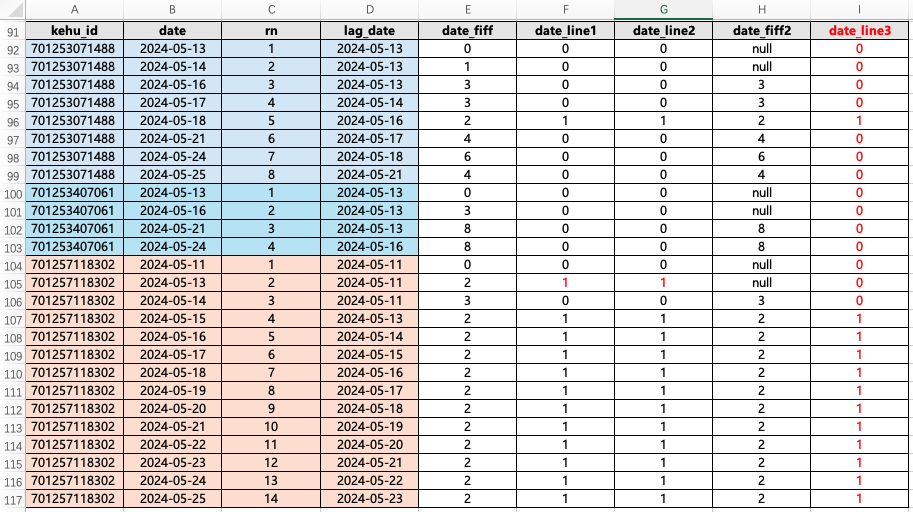
方法三:lag 和 max 开窗函数
我使用 '0000-00-00' 填充 NULL,lag 之后一个日期。再计算日期差,出现 NULL正好,不参与计算加减和判断。然后对日期差 date_diff 进行判断,是等于1,则判断成 0 ,如果不是1,则是登录日期 date ,为下一步做准备。最后使用 max() 开窗函数,逐项判断登录的最近(最大)日期。
"max(t1.date_line) over (partition by t1.kehu_id order by t1.date) as max_line" 意思是对 date_line 取最大值,按照客户号分区,登录日期 date 生序排序。
sql
with temp02_cust_logon2 as
(
select
t1.kehu_id
,t1.date
,row_number () over (partition by t1.kehu_id order by t1.date) as rn
,lag(t1.date,1,'0000-00-00') over (partition by t1.kehu_id order by t1.date asc) as lag_date
from
temp01_cust_logon as t1
)
-- select * from temp02_cust_logon2
,temp03_cust_logon3 as
(
select
t2.kehu_id
,t2.date
,t2.rn
,t2.lag_date
,date_diff(t2.date,t2.lag_date) as date_fiff
,if (date_diff(t2.date,t2.lag_date) = 1,'0',t2.date) as date_line
from
temp02_cust_logon2 as t2
)
select
t1.kehu_id
,t1.date
,t1.lag_date
,t1.date_fiff
,t1.date_line
,max(t1.date_line) over (partition by t1.kehu_id order by t1.date) as max_line
from
temp03_cust_logon3 as t1
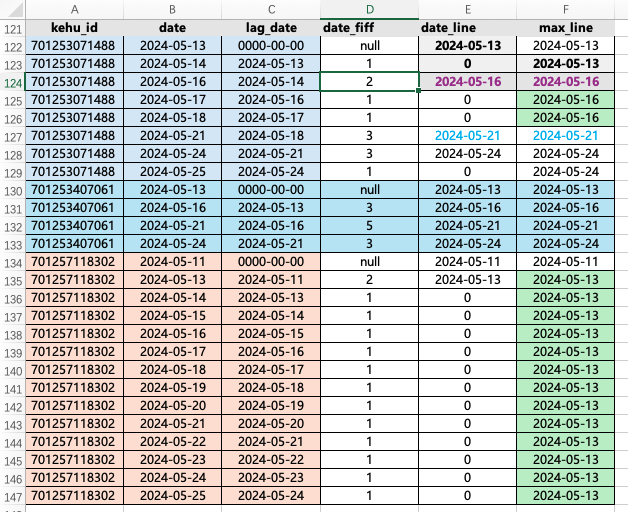
方法四:自相关
自相关理解相对容易,但是数据量大的话,产生的笛卡尔积,数据会爆炸性的增加,查询时间很久,不推荐数据量大的情况。截图数据不全。
使用客户号关联,第一个客户有8个日期,自关联后 2024-05-13 就会和自己另外的 8个日期关联到。这样是三个客户,分别有 8、4、14 个日期,那自相关后产生多行数据?276。是 8 * 8 + 4 * 4 + 14 * 14 = 276。
sql
select
t1.kehu_id
,t1.date
,t2.date as date2
,t2.kehu_id as kehu_id2
,date_sub(t1.date,2) as date_sub
from
temp01_cust_logon as t1
inner join
temp01_cust_logon as t2
on t1.kehu_id = t2.kehu_id
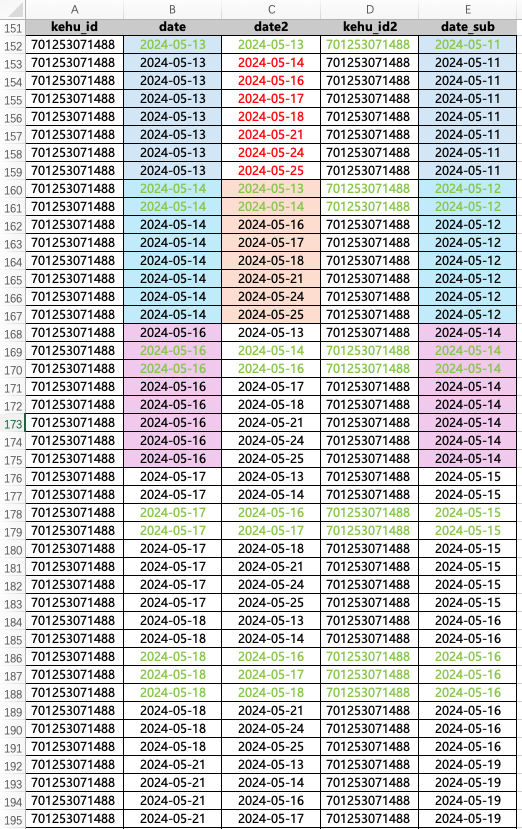
sql
select
t1.kehu_id
,t1.date
,t2.date as date2
,t2.kehu_id as kehu_id2
,date_sub(t1.date,2) as date_sub
from
temp01_cust_logon as t1
inner join
temp01_cust_logon as t2
on t1.kehu_id = t2. kehu_id
where
t2.date between date_sub(t1.date,2) and t1.date
date2 在 date_sub 和 date 之间。between and 是 >= and <= 。
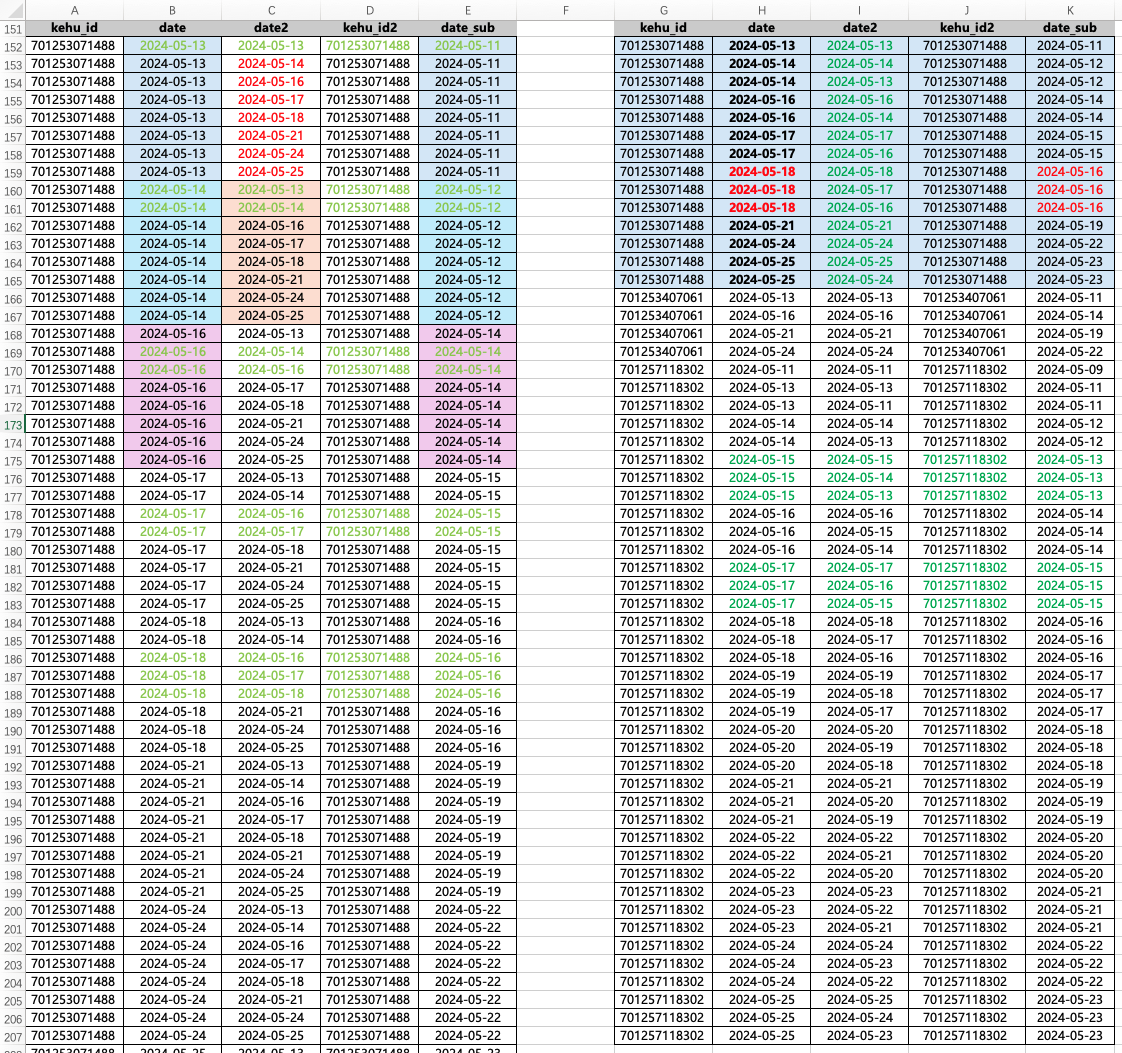
然后再统计。
sql
with temp02_cust_logon2 as
(
select
t1.kehu_id
,t1.date
,t2.date as date2
,t2.kehu_id as kehu_id2
,date_sub(t1.date,2) as date_sub
from
temp01_cust_logon as t1
inner join
temp01_cust_logon as t2
on t1.kehu_id = t2. kehu_id
where
t2.date between date_sub(t1.date,2) and t1.date
)
select
t1.kehu_id
,t1.date
, count(1) as cnt
from temp02_cust_logon2 as t1
group by
t1.kehu_id
,t1.date
having
count(1) >= 3
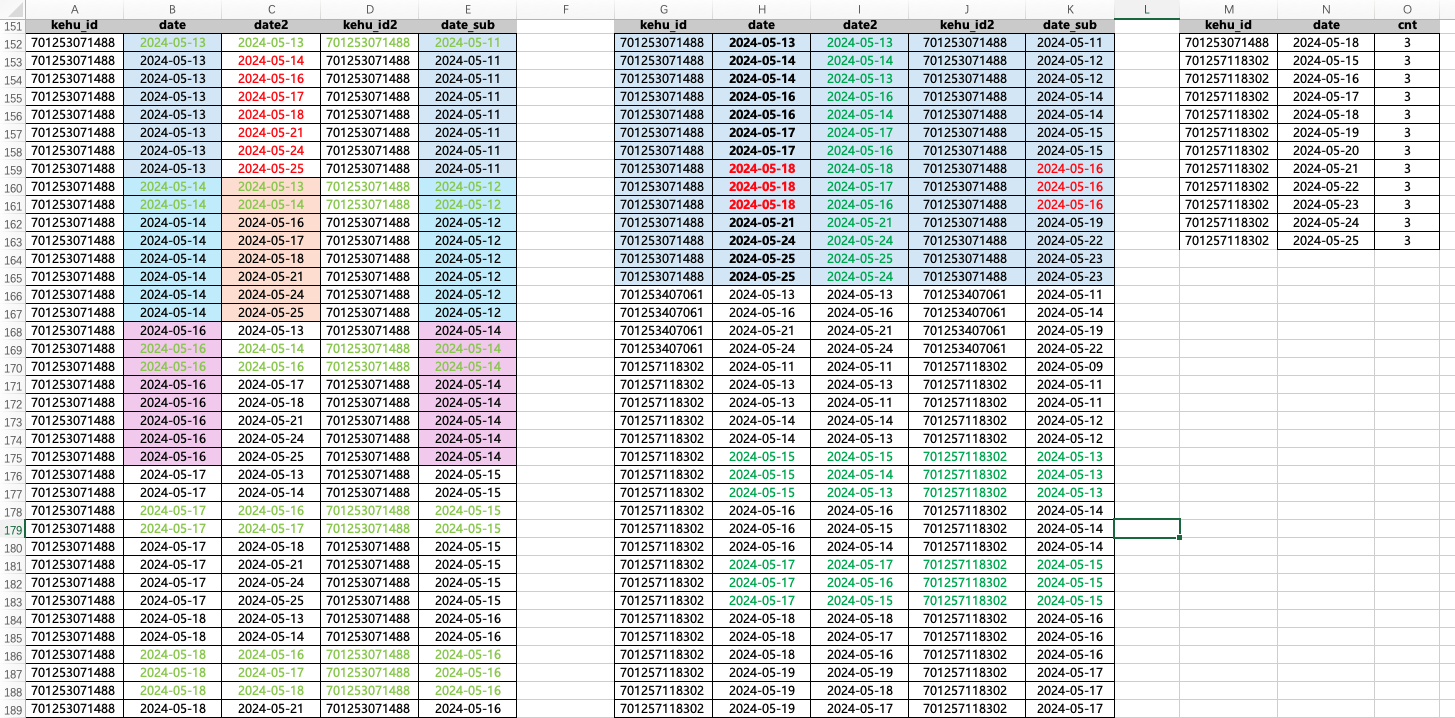
小提示:Mac 操作excel重复上一步是 command + Y 。替换的快捷键是command+shift+H ,查找是 command + F 。
参考:
数仓面试------连续登录问题:https://mp.weixin.qq.com/s/W81ivF0uPWsVZP28IEhFvQ