使用Hadoop MapReduce分析邮件日志提取 id、状态 和 目标邮箱
在大数据处理和分析的场景中,Hadoop MapReduce是一种常见且高效的工具。本文将展示如何使用Hadoop MapReduce来分析邮件日志,提取邮件的发送状态(成功、失败或退回)和目标邮箱。
项目结构
我们将创建一个Java项目,该项目包含三个主要部分:
**Mapper类:**解析邮件日志,提取ID、状态和目标邮箱。
**Reducer类:**汇总Mapper输出的数据,生成最终结果。
**Driver类:**配置和运行MapReduce作业。
数据格式
我们将处理的邮件日志示例如下:
在这些日志中,我们需要提取邮件的ID、发送状态(成功、失败或退回)和目标邮箱。
代码实现
以下是完整的Java代码,包含Mapper、Reducer和Driver类:
java
package org.example.mapReduce;
import java.io.IOException;
import org.apache.hadoop.conf.Configuration;
import org.apache.hadoop.fs.Path;
import org.apache.hadoop.io.LongWritable;
import org.apache.hadoop.io.Text;
import org.apache.hadoop.mapreduce.Job;
import org.apache.hadoop.mapreduce.Mapper;
import org.apache.hadoop.mapreduce.Reducer;
import org.apache.hadoop.mapreduce.lib.input.FileInputFormat;
import org.apache.hadoop.mapreduce.lib.output.FileOutputFormat;
public class MailLogAnalysis {
public static class MailLogMapper extends Mapper<LongWritable, Text, Text, Text> {
@Override
protected void map(LongWritable key, Text value, Context context) throws IOException, InterruptedException {
String line = value.toString();
if (line.contains("starting delivery")) {
String[] parts = line.split(" ");
String id = parts[3].replace(":", "");
String targetEmail = parts[8];
context.write(new Text(id), new Text("email," + targetEmail));
}
if (line.contains("success") || line.contains("failure") || line.contains("bounce")) {
String status = "success";
if (line.contains("failure")) {
status = "failure";
}
if (line.contains("bounce")) {
status = "bounce";
}
String[] parts = line.split(" ");
String id = parts[2].replace(":", "");
context.write(new Text(id), new Text("status," + status));
}
}
}
public static class MailLogReducer extends Reducer<Text, Text, Text, Text> {
@Override
protected void reduce(Text key, Iterable<Text> values, Context context) throws IOException, InterruptedException {
String email = "";
String status = "failure";
for (Text val : values) {
String[] parts = val.toString().split(",", 2);
if (parts[0].equals("email")) {
email = parts[1];
} else if (parts[0].equals("status")) {
status = parts[1];
}
}
context.write(key, new Text(status + "," + email));
}
}
public static void main(String[] args) throws Exception {
Configuration conf = new Configuration();
Job job = Job.getInstance(conf, "Mail Log Analysis");
job.setJarByClass(MailLogAnalysis.class);
job.setMapperClass(MailLogMapper.class);
job.setReducerClass(MailLogReducer.class);
job.setOutputKeyClass(Text.class);
job.setOutputValueClass(Text.class);
FileInputFormat.addInputPath(job, new Path(args[0]));
FileOutputFormat.setOutputPath(job, new Path(args[1]));
System.exit(job.waitForCompletion(true) ? 0 : 1);
}
}
使用Hadoop MapReduce分析邮件日志
在大数据处理和分析的场景中,Hadoop MapReduce是一种常见且高效的工具。本文将展示如何使用Hadoop MapReduce来分析邮件日志,提取邮件的发送状态(成功、失败或退回)和目标邮箱。我们将通过一个具体的例子来实现这一目标。
项目结构
我们将创建一个Java项目,该项目包含三个主要部分:
Mapper类:解析邮件日志,提取ID、状态和目标邮箱。
Reducer类:汇总Mapper输出的数据,生成最终结果。
Driver类:配置和运行MapReduce作业。
数据格式
我们将处理的邮件日志示例如下:
less
复制代码
@400000004faa61e21e8e3e24 starting delivery 1820: msg 850901 to remote sunkang@189.cn
@400000004faa61e536864a44 delivery 1820: success: 121.14.53.136_accepted_message./Remote_host_said:_250_Ok:_queued_as_43A2222C006/
@400000004faa61e70a73c60c delivery 1823: deferral: 210.32.157.174_failed_after_I_sent_the_message./Remote_host_said:_450_Requested_action_not_taken:_AQAAf5CrT+qlYqpPamRUAA--.7571S2,_please_try_again/
@400000004faa61e70a73c60c bounce 1824: 550 Mailbox not found
在这些日志中,我们需要提取邮件的ID、发送状态(成功、失败或退回)和目标邮箱。
代码实现
以下是完整的Java代码,包含Mapper、Reducer和Driver类:
java
复制代码
package org.example.mapReduce;
import java.io.IOException;
import org.apache.hadoop.conf.Configuration;
import org.apache.hadoop.fs.Path;
import org.apache.hadoop.io.LongWritable;
import org.apache.hadoop.io.Text;
import org.apache.hadoop.mapreduce.Job;
import org.apache.hadoop.mapreduce.Mapper;
import org.apache.hadoop.mapreduce.Reducer;
import org.apache.hadoop.mapreduce.lib.input.FileInputFormat;
import org.apache.hadoop.mapreduce.lib.output.FileOutputFormat;
public class MailLogAnalysis {
public static class MailLogMapper extends Mapper<LongWritable, Text, Text, Text> {
@Override
protected void map(LongWritable key, Text value, Context context) throws IOException, InterruptedException {
String line = value.toString();
if (line.contains("starting delivery")) {
String[] parts = line.split(" ");
String id = parts[3].replace(":", "");
String targetEmail = parts[8];
context.write(new Text(id), new Text("email," + targetEmail));
}
if (line.contains("success") || line.contains("failure") || line.contains("bounce")) {
String status = "success";
if (line.contains("failure")) {
status = "failure";
}
if (line.contains("bounce")) {
status = "bounce";
}
String[] parts = line.split(" ");
String id = parts[2].replace(":", "");
context.write(new Text(id), new Text("status," + status));
}
}
}
public static class MailLogReducer extends Reducer<Text, Text, Text, Text> {
@Override
protected void reduce(Text key, Iterable<Text> values, Context context) throws IOException, InterruptedException {
String email = "";
String status = "failure";
for (Text val : values) {
String[] parts = val.toString().split(",", 2);
if (parts[0].equals("email")) {
email = parts[1];
} else if (parts[0].equals("status")) {
status = parts[1];
}
}
context.write(key, new Text(status + "," + email));
}
}
public static void main(String[] args) throws Exception {
Configuration conf = new Configuration();
Job job = Job.getInstance(conf, "Mail Log Analysis");
job.setJarByClass(MailLogAnalysis.class);
job.setMapperClass(MailLogMapper.class);
job.setReducerClass(MailLogReducer.class);
job.setOutputKeyClass(Text.class);
job.setOutputValueClass(Text.class);
FileInputFormat.addInputPath(job, new Path(args[0]));
FileOutputFormat.setOutputPath(job, new Path(args[1]));
System.exit(job.waitForCompletion(true) ? 0 : 1);
}
}
代码解释
Mapper类
MailLogMapper类从日志中提取邮件的ID、目标邮箱和发送状态,并将这些信息作为键值对输出:
如果行包含"starting delivery",则提取邮件的ID和目标邮箱,并输出键值对<ID, email, 目标邮箱>。
如果行包含"success"、"failure"或"bounce",则提取邮件的ID和发送状态,并输出键值对<ID, status, 发送状态>。
Reducer类
MailLogReducer类汇总Mapper输出的数据,生成最终的结果:
对于每个邮件ID,汇总对应的目标邮箱和发送状态。
输出包含ID、发送状态和目标邮箱的最终结果。
Driver类
MailLogAnalysis类配置和运行MapReduce作业:
设置作业名称、Mapper类和Reducer类。
设置输入路径和输出路径。
提交作业并等待完成。
MapReduce运行结果
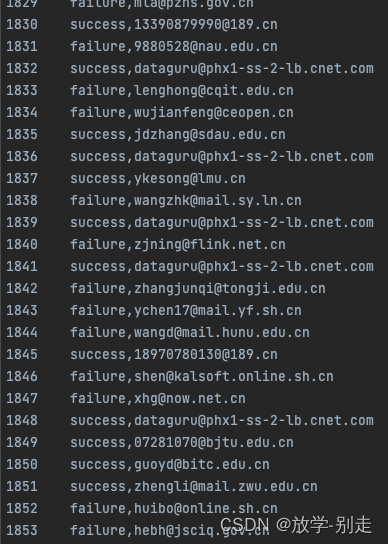
总结
通过本文的示例,我们展示了如何使用Hadoop MapReduce来分析邮件日志,提取邮件的发送状态和目标邮箱。希望本文能为您的大数据处理和分析工作提供一些帮助。
如有遇到问题可以找小编沟通交流哦。另外小编帮忙辅导大课作业,学生毕设等。不限于MapReduce, MySQL, python,java,大数据,模型训练等。 hadoop hdfs yarn spark Django flask flink kafka flume datax sqoop seatunnel echart可视化 机器学习等