下面的例子是简单的使用opencv 实现的模板匹配流程,其中时间性能和精确度还需要调整,如果直接使用会出问题,所以这个只是例子,根据代码原理可以实现尺度变化的模板匹配和旋转尺度变化同时,具体根据实现的旋转代码进一步实现,但是就结果而言和halcon的模板匹配差距较大,性能更不行,因此仅供参考
cpp
struct MatchResult
{
std::vector<cv::Point> points;
double angle;
double score;
MatchResult(std::vector<cv::Point> points, double angle, double score) :points(points), angle(angle), score(score) {};
};
//旋转图像
cv::Mat ImageRotate(cv::Mat image, double angle)
{
cv::Mat newImg;
cv::Point2f pt = cv::Point2f((float)image.cols / 2, (float)image.rows / 2);
cv::Mat M = cv::getRotationMatrix2D(pt, angle, 1.0);
cv::warpAffine(image, newImg, M, image.size());
return newImg;
}
std::vector<cv::Point> GetRotatePoints(cv::Size size, double angle) {
// 定义模板图像的中心点
cv::Point2f center(size.width / 2.0, size.height / 2.0);
// 计算旋转矩阵
cv::Mat rotationMatrix = cv::getRotationMatrix2D(center, angle, 1.0);
// 定义模板图像的四个顶点
std::vector<cv::Point2f> srcPoints = {
cv::Point2f(0, 0),
cv::Point2f(size.width, 0),
cv::Point2f(size.width, size.height),
cv::Point2f(0, size.height)
};
// 存储旋转后的四个顶点
std::vector<cv::Point2f> dstPoints(4);
// 进行仿射变换
cv::transform(srcPoints, dstPoints, rotationMatrix);
// 将结果转换为cv::Point类型并返回
std::vector<cv::Point> resultPoints(4);
for (int i = 0; i < 4; ++i) {
resultPoints[i] = cv::Point(cvRound(dstPoints[i].x), cvRound(dstPoints[i].y));
}
return resultPoints;
}
/*
旋转模板匹配函数(通过图像金字塔、增大旋转步长来提升匹配速度)
Mat src:原图像
Mat model:模板图
double startAngle:旋转的最小角
double endAngle:旋转的最大角
double firstStep:角度旋转时的最大步长
double secondStep:角度旋转时的最小步长
int numLevels = 0:图像金字塔缩放次数
*/
MatchResult rotateMatch(cv::Mat src, cv::Mat model, double startAngle, double endAngle, double firstStep, double secondStep, int numLevels = 0) {
//对模板图像和待检测图像分别进行图像金字塔下采样
for (int i = 0; i < numLevels; i++) {
cv::pyrDown(src, src, cv::Size(src.cols / 2, src.rows / 2));
cv::pyrDown(model, model, cv::Size(model.cols / 2, model.rows / 2));
}
cv::Mat rotatedImg, result;
double score = -1;
cv::Point location;
double angle;
bool isSecond = false;
while (true) {
for (double curAngle = startAngle; curAngle <= endAngle; curAngle += firstStep) {
rotatedImg = ImageRotate(model, curAngle);
//imshow("rotated", rotatedImg);
//imshow("src-pyrDown", src);
//waitKey();
matchTemplate(src, rotatedImg, result, cv::TM_CCOEFF_NORMED);
double minval, maxval;
cv::Point minloc, maxloc;
cv::minMaxLoc(result, &minval, &maxval, &minloc, &maxloc);
if (maxval > score)
{
location = maxloc;
score = maxval;
angle = curAngle;
}
}
if (isSecond && firstStep<= secondStep) break;
startAngle = angle - firstStep;
endAngle = angle + firstStep;
if ((endAngle - startAngle) / 5 > secondStep) {
firstStep = (endAngle - startAngle) / 5;
}
else {
firstStep = secondStep;
isSecond = true;
}
}
cv::Point finalPoint = cv::Point(location.x * pow(2, numLevels), location.y * pow(2, numLevels));
std::vector<cv::Point> points = GetRotatePoints(cv::Size(model.cols * pow(2, numLevels), model.rows * pow(2, numLevels)), angle);
for (int j = 0; j < points.size(); j++)
{
points[j].x += finalPoint.x;
points[j].y += finalPoint.y;
}
return MatchResult(points, angle, score);
}
int main() {
//读取所有图像
std::vector<cv::Mat> imgs;
std::string imageName;
std::string path = "E:\\prj\\shape_based_matching-master\\test\\board\\test";
std::vector<std::string> img_paths;
cv::glob(path, img_paths);
for (auto& p : img_paths)
{
cv::Mat img = cv::imread(p);
imgs.push_back(img);
}
cv::Mat templateImg = cv::imread("E:\\prj\\shape_based_matching-master\\test\\board\\train.png");
cv::Rect box(cv::Point(135, 120), cv::Point(470, 365));
//cv::rectangle(drawFrame, box, cv::Scalar(0, 255, 0), 2);
templateImg = templateImg(box).clone();
int i = 0;
for (cv::Mat img : imgs)
{
i += 1;
MatchResult matchResult = rotateMatch(img, templateImg, 0, 360, 30, 0.1, 0);
std::vector<cv::Point> points = matchResult.points;
std::cout << i << "- 角度:" << matchResult.angle << std::endl;
std::cout << i << "- 得分:" << matchResult.score << std::endl;
cv::line(img, points[0], points[1], cv::Scalar(255, 0, 0), 2);
cv::line(img, points[1], points[2], cv::Scalar(255, 0, 0), 2);
cv::line(img, points[2], points[3], cv::Scalar(255, 0, 0), 2);
cv::line(img, points[3], points[0], cv::Scalar(255, 0, 0), 2);
cv::Point pt1 = cv::Point((points[0].x + points[3].x) / 2, (points[0].y + points[3].y) / 2);
cv::Point pt2 = cv::Point((points[1].x + points[2].x) / 2, (points[1].y + points[2].y) / 2);
cv::arrowedLine(img, pt2, pt1, cv::Scalar(0, 0, 255), 2);
cv::imshow("img_" + std::to_string(i), img);
cv::waitKey(0);
}
return 0;
}
结果如下:
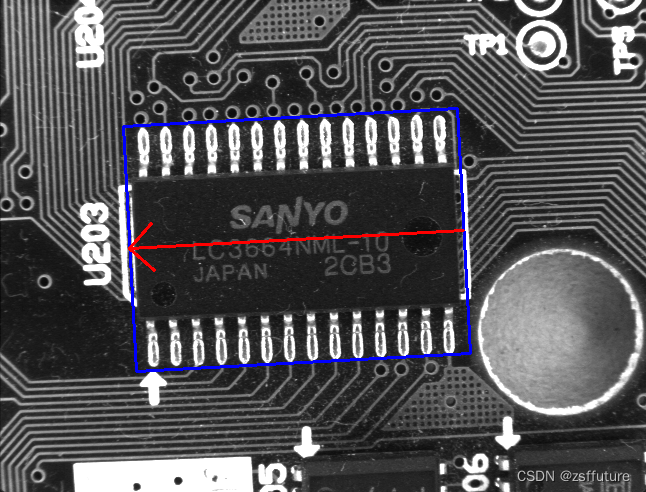
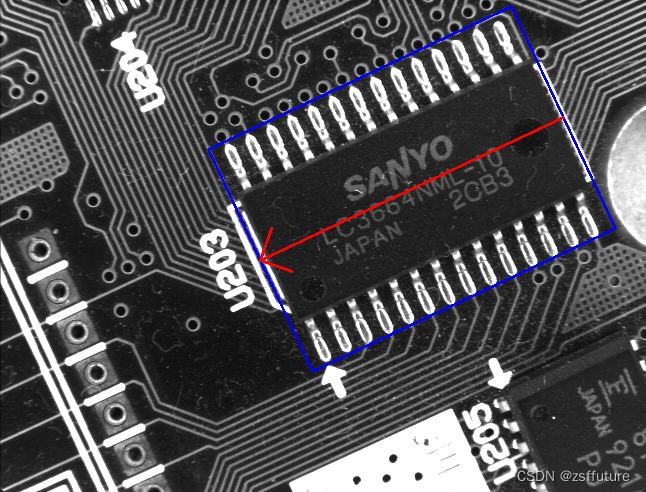
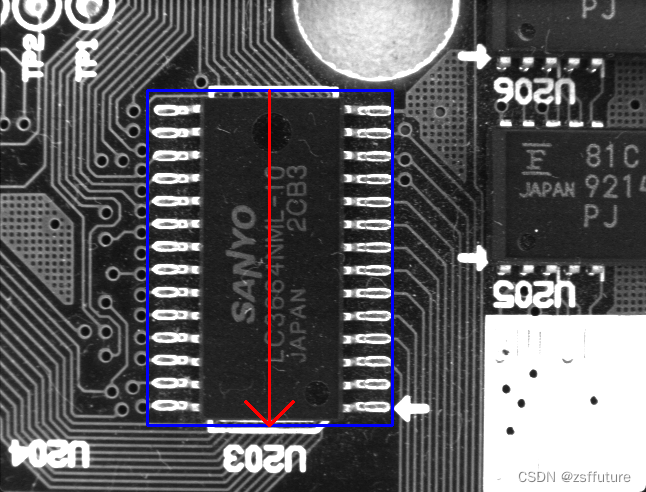
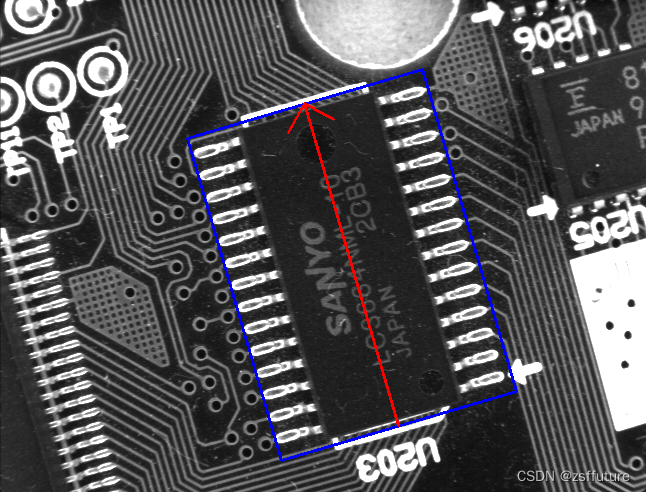
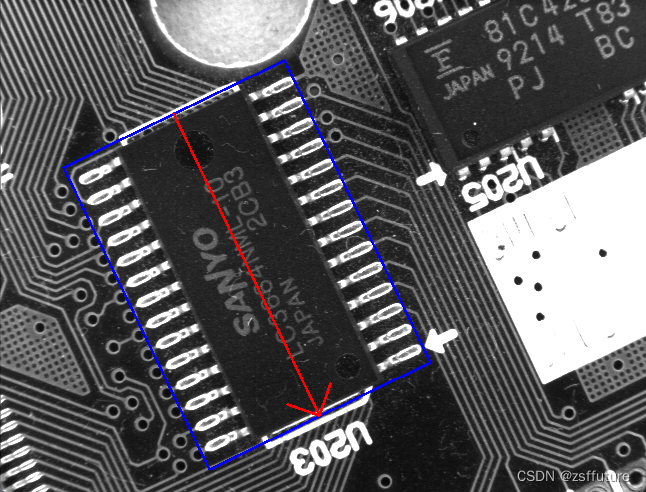
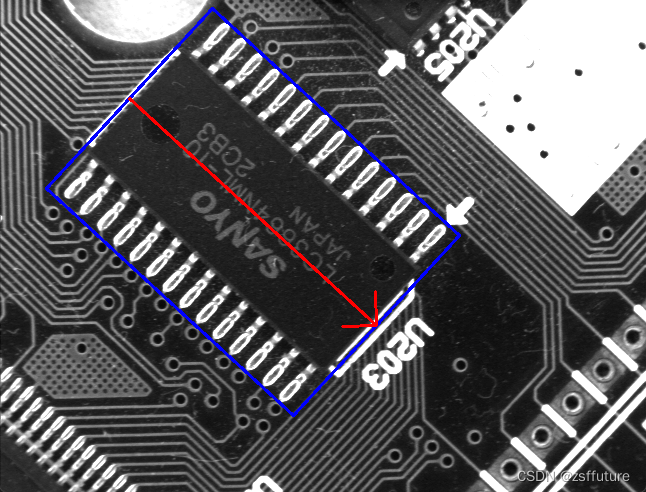