前言
关于LangGraph的简单介绍,请参考这篇博客:
LangGraph开发Agent智能体应用【基础聊天机器人】-CSDN博客
对比LangChain实现NL2SQL
关于用LangChain开发NL2SQL的Agent应用,在这篇博客提供了完整的代码实现:
LangChain开发LLM应用【入门指南】_langchain 开发社区-CSDN博客
我在这里赘述一下:
from sqlalchemy import create_engine
from langchain_community.utilities import SQLDatabase
# 数据库连接信息
username = 'root'
password = 'MyNewPass1!'
host = 'desk04v.mlprod.bjpdc.qihoo.net'
port = '3306'
database = 'test'
engine = create_engine(f'mysql+mysqlconnector://{username}:{password}@{host}:{port}/{database}')
db = SQLDatabase(engine)
result = db.run("select * FROM courses LIMIT 5;")
print(result)
from langchain_community.agent_toolkits import create_sql_agent
from langchain_openai import ChatOpenAI
llm = ChatOpenAI(model="gpt-4o", temperature=0)
agent_executor = create_sql_agent(llm, db=db, agent_type="openai-tools", verbose=True)
agent_executor.invoke(
"找到学分最高的课程"
)
效果如下:
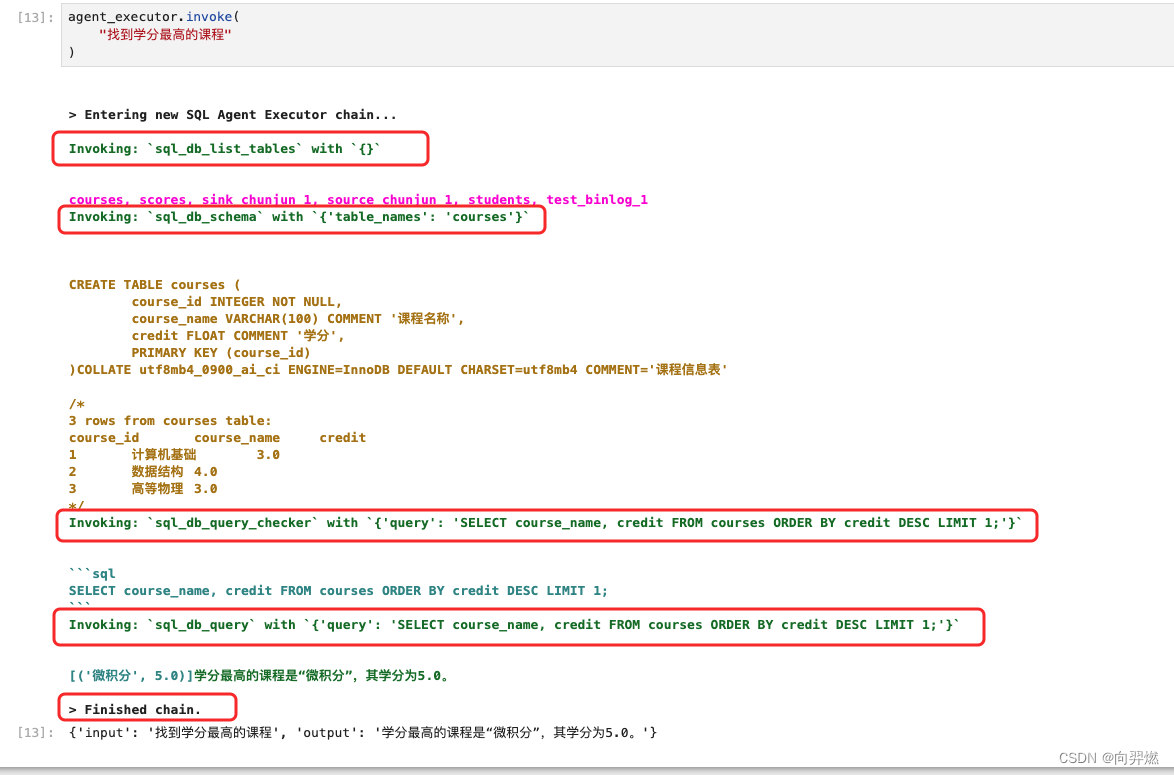
我并没有在代码中定义执行链,只是给LLM提供了一个工具集,让Agent自行决定如何使用。
可以看到,Agent,先查了一下数据库中有哪些表,找到看上去有用的表后,再查了一下表结构以及预览数据,再生成sql执行(先校验一遍再执行),最后整合结果告诉我结论。
可以说已经是非常智能了。
LangGraph实现实现NL2SQL
LangGraph的方式,就和LangChain不一样了,它的开发方式就是不断给图添加"节点"和"线",组成一个工作流。
注意:这里的工作流,并不是简单理解的操作流,LangGraph的工作流和LangChain的工作流不是是一个层面的东西,相信你看完这个例子就能感受到了。
还是先上代码!!!
第一步:定义工具集合
LangChain 和 LangGraph是打通的(准确的说,LangGraph是LangChain生态的高级框架)
所以我们可以直接使用LangChain的工具集 SQLDatabaseToolkit
如果你愿意深入看看源码,就知道这个工具集里有四个工具:
执行sql:QuerySQLDataBaseTool
查看表详情:InfoSQLDatabaseTool
sql语法检查:QuerySQLCheckerTool
查看所有表:ListSQLDatabaseTool
python
from langchain_community.agent_toolkits import SQLDatabaseToolkit
from langchain_openai import ChatOpenAI
from sqlalchemy import create_engine
from langchain_community.utilities import SQLDatabase
# 数据库连接信息
username = 'root'
password = 'MyNewPass1!'
host = 'desk04v.mlprod.bjpdc.qihoo.net'
port = '3306'
database = 'test'
engine = create_engine(f'mysql+mysqlconnector://{username}:{password}@{host}:{port}/{database}')
db = SQLDatabase(engine)
toolkit = SQLDatabaseToolkit(db=db, llm=ChatOpenAI(temperature=0))
context = toolkit.get_context()
tools = toolkit.get_tools()
第二步:定义LLM节点,并加入到图中
让LLM绑定工具,一定要绑定,就像你需要告诉LLM,可以使用哪些工具,LLM才会生成调用计划
python
from typing import Annotated
from langchain_openai import ChatOpenAI
from typing_extensions import TypedDict
from langgraph.graph import StateGraph
from langgraph.graph.message import add_messages
class State(TypedDict):
messages: Annotated[list, add_messages]
graph_builder = StateGraph(State)
# expt_llm = "gpt-4-1106-preview"
expt_llm = "gpt-4o"
llm = ChatOpenAI(temperature=0, model=expt_llm)
# Modification: tell the LLM which tools it can call
llm_with_tools = llm.bind_tools(tools)
def chatbot(state: State):
return {"messages": [llm_with_tools.invoke(state["messages"])]}
graph_builder.add_node("chatbot", chatbot)
第三步:定义工具节点,并加入到图中
python
import json
from langchain_core.messages import ToolMessage
class BasicToolNode:
"""运行最后一个AIMessage中请求的工具"""
def __init__(self, tools: list) -> None:
self.tools_by_name = {tool.name: tool for tool in tools}
def __call__(self, inputs: dict):
if messages := inputs.get("messages", []):
message = messages[-1]
else:
raise ValueError("No message found in input")
outputs = []
for tool_call in message.tool_calls:
print(tool_call["name"])
print(self.tools_by_name[tool_call["name"]])
tool_result = self.tools_by_name[tool_call["name"]].invoke(
tool_call["args"]
)
outputs.append(
ToolMessage(
content=json.dumps(tool_result),
name=tool_call["name"],
tool_call_id=tool_call["id"],
)
)
return {"messages": outputs}
tool_node = BasicToolNode(tools=tools)
graph_builder.add_node("tools", tool_node)
第四步:定义"边"
add_edge方法是直接定义"边",在例子中表示tools -> chatbot
add_conditional_edges方法是增加条件路由"边",在例子中表示chatbot根据情况 -> tools 或者 -> end
python
from typing import Literal
def route_tools(
state: State,
) -> Literal["tools", "__end__"]:
"""如果最后一条消息,在conditional_edge中使用路由到ToolNode,就调用工具。否则,路线到终点。"""
if isinstance(state, list):
ai_message = state[-1]
elif messages := state.get("messages", []):
ai_message = messages[-1]
else:
raise ValueError(f"在tool_edge的输入状态中没有找到消息: {state}")
if hasattr(ai_message, "tool_calls") and len(ai_message.tool_calls) > 0:
return "tools"
return "__end__"
# ' tools_condition '函数返回"tools",表示LLM要求使用工具,返回"__end__"直接结束。
graph_builder.add_conditional_edges(
"chatbot",
route_tools,
# The following dictionary lets you tell the graph to interpret the condition's outputs as a specific node
# It defaults to the identity function, but if you
# want to use a node named something else apart from "tools",
# You can update the value of the dictionary to something else
# e.g., "tools": "my_tools"
{"tools": "tools", "__end__": "__end__"},
)
# 任何时候调用一个工具,我们都会流转到聊天机器人
graph_builder.add_edge("tools", "chatbot")
graph_builder.set_entry_point("chatbot")
graph = graph_builder.compile()
第五步:把图画出来(非必需)
python
from IPython.display import Image, display
try:
display(Image(graph.get_graph().draw_mermaid_png()))
except:
# This requires some extra dependencies and is optional
pass
效果如下:
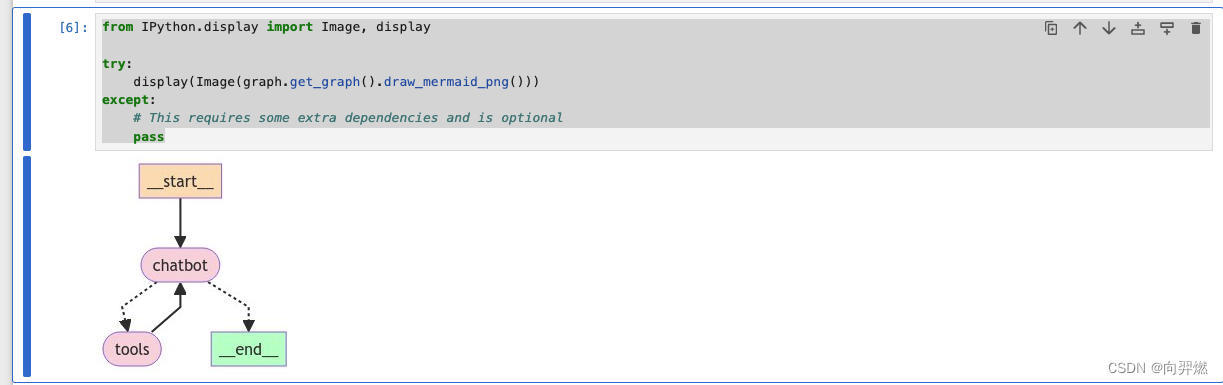
整个流程很简单,用大白话讲,就是:
把提问信息传给LLM,LLM决定用什么工具,然后graph就调用工具返回结果传给LLM,LLM拿到结果后有可能继续调用工具,也有可能直接输出答案,如此循环或者终止。
第六步:执行
通过流式调用,传入用户的提问
python
from langchain_core.messages import BaseMessage
while True:
user_input = input("User: ")
if user_input.lower() in ["quit", "exit", "q"]:
print("Goodbye!")
break
for event in graph.stream({"messages": [("user", user_input)]}):
for value in event.values():
if isinstance(value["messages"][-1], BaseMessage):
print("Assistant:", value["messages"][-1].content)
效果如下:
python
User: 找到5月各地区的运费最贵的用户
Assistant:
sql_db_list_tables
db=<langchain_community.utilities.sql_database.SQLDatabase object at 0x7fd64efb1f90>
Assistant: "arbitraments, courses, orders, scores, sink_chunjun_1, source_chunjun_1, students, test_binlog_1"
Assistant:
sql_db_schema
description='Input to this tool is a comma-separated list of tables, output is the schema and sample rows for those tables. Be sure that the tables actually exist by calling sql_db_list_tables first! Example Input: table1, table2, table3' db=<langchain_community.utilities.sql_database.SQLDatabase object at 0x7fd64efb1f90>
Assistant: "\nCREATE TABLE orders (\n\torder_id INTEGER NOT NULL COMMENT '\u8ba2\u5355ID', \n\tcustomer_id VARCHAR(255) COMMENT '\u5ba2\u6237ID', \n\temployee_id INTEGER COMMENT '\u5458\u5de5ID', \n\torder_date DATE COMMENT '\u8ba2\u5355\u65e5\u671f', \n\trequired_date DATE COMMENT '\u8981\u6c42\u4ea4\u8d27\u65e5\u671f', \n\tshipped_date DATE COMMENT '\u53d1\u8d27\u65e5\u671f', \n\tshipper_id INTEGER COMMENT '\u53d1\u8d27\u65b9\u5f0f', \n\tfreight DECIMAL(10, 2) COMMENT '\u8fd0\u8d39', \n\tship_name VARCHAR(255) COMMENT '\u6536\u8d27\u4eba\u540d\u79f0', \n\tship_address VARCHAR(255) COMMENT '\u6536\u8d27\u5730\u5740', \n\tship_city VARCHAR(255) COMMENT '\u6536\u8d27\u57ce\u5e02', \n\tship_region VARCHAR(255) COMMENT '\u6536\u8d27\u5730\u533a', \n\tship_postal_code VARCHAR(255) COMMENT '\u6536\u8d27\u90ae\u7f16', \n\torder_status VARCHAR(50) COMMENT '\u8ba2\u5355\u72b6\u6001', \n\tsnapshot_timestamp TIMESTAMP NULL COMMENT '\u5feb\u7167\u65f6\u95f4\u6233' DEFAULT CURRENT_TIMESTAMP, \n\tPRIMARY KEY (order_id)\n)DEFAULT CHARSET=utf8mb4 COLLATE utf8mb4_0900_ai_ci ENGINE=InnoDB\n\n/*\n3 rows from orders table:\norder_id\tcustomer_id\temployee_id\torder_date\trequired_date\tshipped_date\tshipper_id\tfreight\tship_name\tship_address\tship_city\tship_region\tship_postal_code\torder_status\tsnapshot_timestamp\n1\tCUST001\t1\t2024-05-01\t2024-05-05\t2024-05-03\t1\t100.50\t\u5f20\u4e09\t\u5317\u4eac\u5e02\u671d\u9633\u533a\u5efa\u56fd\u8def100\u53f7\t\u5317\u4eac\t\u534e\u5317\t100022\t\u5df2\u53d1\u8d27\t2024-06-04 17:05:11\n2\tCUST002\t2\t2024-05-02\t2024-05-06\t2024-05-04\t2\t200.75\t\u674e\u56db\t\u4e0a\u6d77\u5e02\u6d66\u4e1c\u65b0\u533a\u4e16\u7eaa\u5927\u9053200\u53f7\t\u4e0a\u6d77\t\u534e\u4e1c\t200120\t\u5df2\u53d1\u8d27\t2024-06-04 17:05:11\n3\tCUST003\t3\t2024-05-03\t2024-05-07\t2024-05-05\t3\t150.00\t\u738b\u4e94\t\u5e7f\u5dde\u5e02\u5929\u6cb3\u533a\u4f53\u80b2\u897f\u8def300\u53f7\t\u5e7f\u5dde\t\u534e\u5357\t510620\t\u5df2\u53d1\u8d27\t2024-06-04 17:05:11\n*/"
Assistant:
sql_db_query_checker
description='Use this tool to double check if your query is correct before executing it. Always use this tool before executing a query with sql_db_query!' db=<langchain_community.utilities.sql_database.SQLDatabase object at 0x7fd64efb1f90> llm=ChatOpenAI(client=<openai.resources.chat.completions.Completions object at 0x7fd64e081310>, async_client=<openai.resources.chat.completions.AsyncCompletions object at 0x7fd64e099350>, temperature=0.0, openai_api_key=SecretStr('**********'), openai_api_base='https://api.360.cn/v1', openai_proxy='') llm_chain=LLMChain(prompt=PromptTemplate(input_variables=['dialect', 'query'], template='\n{query}\nDouble check the {dialect} query above for common mistakes, including:\n- Using NOT IN with NULL values\n- Using UNION when UNION ALL should have been used\n- Using BETWEEN for exclusive ranges\n- Data type mismatch in predicates\n- Properly quoting identifiers\n- Using the correct number of arguments for functions\n- Casting to the correct data type\n- Using the proper columns for joins\n\nIf there are any of the above mistakes, rewrite the query. If there are no mistakes, just reproduce the original query.\n\nOutput the final SQL query only.\n\nSQL Query: '), llm=ChatOpenAI(client=<openai.resources.chat.completions.Completions object at 0x7fd64e081310>, async_client=<openai.resources.chat.completions.AsyncCompletions object at 0x7fd64e099350>, temperature=0.0, openai_api_key=SecretStr('**********'), openai_api_base='https://api.360.cn/v1', openai_proxy=''))
Assistant: "SELECT ship_region, customer_id, freight FROM orders \nWHERE MONTH(order_date) = 5 \nORDER BY freight DESC \nLIMIT 1;"
Assistant:
sql_db_query
description="Input to this tool is a detailed and correct SQL query, output is a result from the database. If the query is not correct, an error message will be returned. If an error is returned, rewrite the query, check the query, and try again. If you encounter an issue with Unknown column 'xxxx' in 'field list', use sql_db_schema to query the correct table fields." db=<langchain_community.utilities.sql_database.SQLDatabase object at 0x7fd64efb1f90>
Assistant: "[('\u534e\u4e1c', 'CUST008', Decimal('300.80'))]"
Assistant: 在5月份各地区中,运费最贵的用户信息如下:
- 地区:华东
- 用户ID:CUST008
- 运费:300.80元
User: 统计5月的每个地区的运费最贵的用户
Assistant:
sql_db_list_tables
db=<langchain_community.utilities.sql_database.SQLDatabase object at 0x7fd64efb1f90>
Assistant: "arbitraments, courses, orders, scores, sink_chunjun_1, source_chunjun_1, students, test_binlog_1"
Assistant:
sql_db_schema
description='Input to this tool is a comma-separated list of tables, output is the schema and sample rows for those tables. Be sure that the tables actually exist by calling sql_db_list_tables first! Example Input: table1, table2, table3' db=<langchain_community.utilities.sql_database.SQLDatabase object at 0x7fd64efb1f90>
Assistant: "\nCREATE TABLE orders (\n\torder_id INTEGER NOT NULL COMMENT '\u8ba2\u5355ID', \n\tcustomer_id VARCHAR(255) COMMENT '\u5ba2\u6237ID', \n\temployee_id INTEGER COMMENT '\u5458\u5de5ID', \n\torder_date DATE COMMENT '\u8ba2\u5355\u65e5\u671f', \n\trequired_date DATE COMMENT '\u8981\u6c42\u4ea4\u8d27\u65e5\u671f', \n\tshipped_date DATE COMMENT '\u53d1\u8d27\u65e5\u671f', \n\tshipper_id INTEGER COMMENT '\u53d1\u8d27\u65b9\u5f0f', \n\tfreight DECIMAL(10, 2) COMMENT '\u8fd0\u8d39', \n\tship_name VARCHAR(255) COMMENT '\u6536\u8d27\u4eba\u540d\u79f0', \n\tship_address VARCHAR(255) COMMENT '\u6536\u8d27\u5730\u5740', \n\tship_city VARCHAR(255) COMMENT '\u6536\u8d27\u57ce\u5e02', \n\tship_region VARCHAR(255) COMMENT '\u6536\u8d27\u5730\u533a', \n\tship_postal_code VARCHAR(255) COMMENT '\u6536\u8d27\u90ae\u7f16', \n\torder_status VARCHAR(50) COMMENT '\u8ba2\u5355\u72b6\u6001', \n\tsnapshot_timestamp TIMESTAMP NULL COMMENT '\u5feb\u7167\u65f6\u95f4\u6233' DEFAULT CURRENT_TIMESTAMP, \n\tPRIMARY KEY (order_id)\n)DEFAULT CHARSET=utf8mb4 COLLATE utf8mb4_0900_ai_ci ENGINE=InnoDB\n\n/*\n3 rows from orders table:\norder_id\tcustomer_id\temployee_id\torder_date\trequired_date\tshipped_date\tshipper_id\tfreight\tship_name\tship_address\tship_city\tship_region\tship_postal_code\torder_status\tsnapshot_timestamp\n1\tCUST001\t1\t2024-05-01\t2024-05-05\t2024-05-03\t1\t100.50\t\u5f20\u4e09\t\u5317\u4eac\u5e02\u671d\u9633\u533a\u5efa\u56fd\u8def100\u53f7\t\u5317\u4eac\t\u534e\u5317\t100022\t\u5df2\u53d1\u8d27\t2024-06-04 17:05:11\n2\tCUST002\t2\t2024-05-02\t2024-05-06\t2024-05-04\t2\t200.75\t\u674e\u56db\t\u4e0a\u6d77\u5e02\u6d66\u4e1c\u65b0\u533a\u4e16\u7eaa\u5927\u9053200\u53f7\t\u4e0a\u6d77\t\u534e\u4e1c\t200120\t\u5df2\u53d1\u8d27\t2024-06-04 17:05:11\n3\tCUST003\t3\t2024-05-03\t2024-05-07\t2024-05-05\t3\t150.00\t\u738b\u4e94\t\u5e7f\u5dde\u5e02\u5929\u6cb3\u533a\u4f53\u80b2\u897f\u8def300\u53f7\t\u5e7f\u5dde\t\u534e\u5357\t510620\t\u5df2\u53d1\u8d27\t2024-06-04 17:05:11\n*/"
Assistant:
sql_db_query_checker
description='Use this tool to double check if your query is correct before executing it. Always use this tool before executing a query with sql_db_query!' db=<langchain_community.utilities.sql_database.SQLDatabase object at 0x7fd64efb1f90> llm=ChatOpenAI(client=<openai.resources.chat.completions.Completions object at 0x7fd64e081310>, async_client=<openai.resources.chat.completions.AsyncCompletions object at 0x7fd64e099350>, temperature=0.0, openai_api_key=SecretStr('**********'), openai_api_base='https://api.360.cn/v1', openai_proxy='') llm_chain=LLMChain(prompt=PromptTemplate(input_variables=['dialect', 'query'], template='\n{query}\nDouble check the {dialect} query above for common mistakes, including:\n- Using NOT IN with NULL values\n- Using UNION when UNION ALL should have been used\n- Using BETWEEN for exclusive ranges\n- Data type mismatch in predicates\n- Properly quoting identifiers\n- Using the correct number of arguments for functions\n- Casting to the correct data type\n- Using the proper columns for joins\n\nIf there are any of the above mistakes, rewrite the query. If there are no mistakes, just reproduce the original query.\n\nOutput the final SQL query only.\n\nSQL Query: '), llm=ChatOpenAI(client=<openai.resources.chat.completions.Completions object at 0x7fd64e081310>, async_client=<openai.resources.chat.completions.AsyncCompletions object at 0x7fd64e099350>, temperature=0.0, openai_api_key=SecretStr('**********'), openai_api_base='https://api.360.cn/v1', openai_proxy=''))
Assistant: "SELECT ship_region, customer_id, MAX(freight) as max_freight\nFROM orders\nWHERE order_date BETWEEN '2024-05-01' AND '2024-05-31'\nGROUP BY ship_region, customer_id"
Assistant:
sql_db_query
description="Input to this tool is a detailed and correct SQL query, output is a result from the database. If the query is not correct, an error message will be returned. If an error is returned, rewrite the query, check the query, and try again. If you encounter an issue with Unknown column 'xxxx' in 'field list', use sql_db_schema to query the correct table fields." db=<langchain_community.utilities.sql_database.SQLDatabase object at 0x7fd64efb1f90>
Assistant: "[('\u534e\u5317', 'CUST001', Decimal('100.50')), ('\u534e\u4e1c', 'CUST002', Decimal('200.75')), ('\u534e\u5357', 'CUST003', Decimal('150.00')), ('\u534e\u5357', 'CUST004', Decimal('120.25')), ('\u897f\u5357', 'CUST005', Decimal('180.90')), ('\u897f\u5357', 'CUST006', Decimal('250.60')), ('\u534e\u4e1c', 'CUST007', Decimal('90.45')), ('\u534e\u4e1c', 'CUST008', Decimal('300.80')), ('\u534e\u4e2d', 'CUST009', Decimal('220.30')), ('\u897f\u5317', 'CUST010', Decimal('170.95'))]"
Assistant: 以下是5月每个地区运费最贵的用户:
| 地区 | 客户ID | 最大运费 (¥) |
|-------|--------|--------------|
| 华北 | CUST001 | 100.50 |
| 华东 | CUST008 | 300.80 |
| 华南 | CUST003 | 150.00 |
| 西南 | CUST006 | 250.60 |
| 华中 | CUST009 | 220.30 |
| 西北 | CUST010 | 170.95 |
可以看到,每个地区的运费最高的用户及其对应的运费如上所示。
User: q
Goodbye!
总结
不知道你有没有发现一个神奇的现象:
对于问题:找到5月各地区的运费最贵的用户
assistant生成的sql其实是错的:
SELECT ship_region, customer_id, MAX(freight) as max_freight
FROM orders
WHERE order_date BETWEEN '2024-05-01' AND '2024-05-31'
GROUP BY ship_region, customer_id
这是我在Leetcode上找的一个中等难度的sql题
assistant生成的这个sql,执行的结果,应该会出现同一个地区有多个用户的情况,但是最后给我的输出答案确实对的。。。
原因是:chatgpt4o 耍诈,它没有能力生成这么复杂sql,但是可以写一个中间结果的sql,然后自己把结果处理一下再返回给我
这只是测试数据集,数据量比较小,如果在生产环境上,那就有问题了。
如何解决?
可以使用few-shot的方式优化
关于few-shot的调优,我单独写了一个博客,请移步:LangGraph开发Agent智能体应用【NL2SQL】(few-shot优化)-CSDN博客
参考
LangChain开发LLM应用【入门指南】_langchain 开发社区-CSDN博客
LangGraph开发Agent智能体应用【基础聊天机器人】-CSDN博客
代码已上传,按需下载,谢谢大家