rm -r dp-203 -f
git clone https://github.com/MicrosoftLearning/dp-203-azure-data-engineer dp-203
cd dp-203/Allfiles/labs/07
./setup.ps1
python
%%pyspark
df = spark.read.load('abfss://files@datalakexxxxxxx.dfs.core.windows.net/products/products.csv', format='csv'
## If header exists uncomment line below
##, header=True
)
display(df.limit(10))
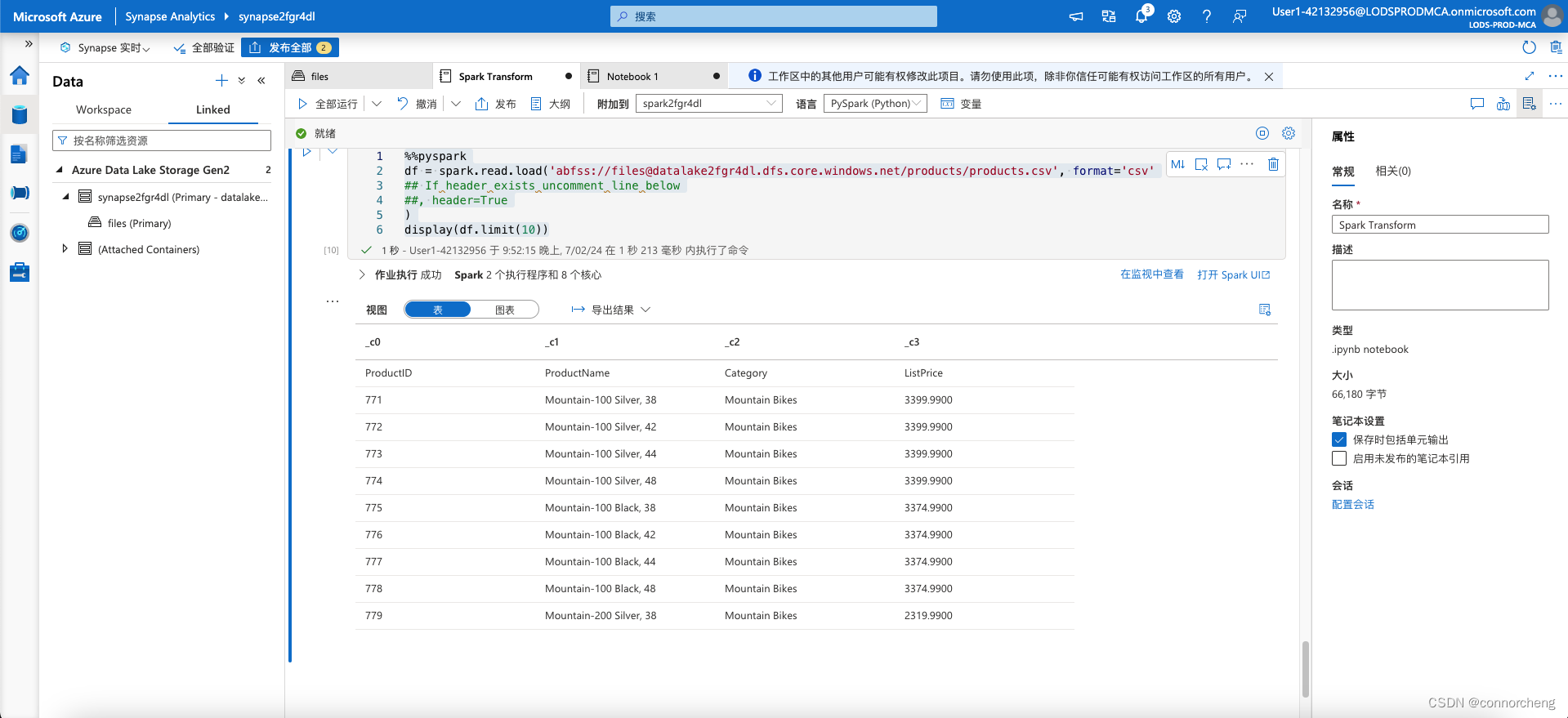
python
%%pyspark
df = spark.read.load('abfss://files@datalakexxxxxxx.dfs.core.windows.net/products/products.csv', format='csv'
## If header exists uncomment line below
, header=True
)
display(df.limit(10))
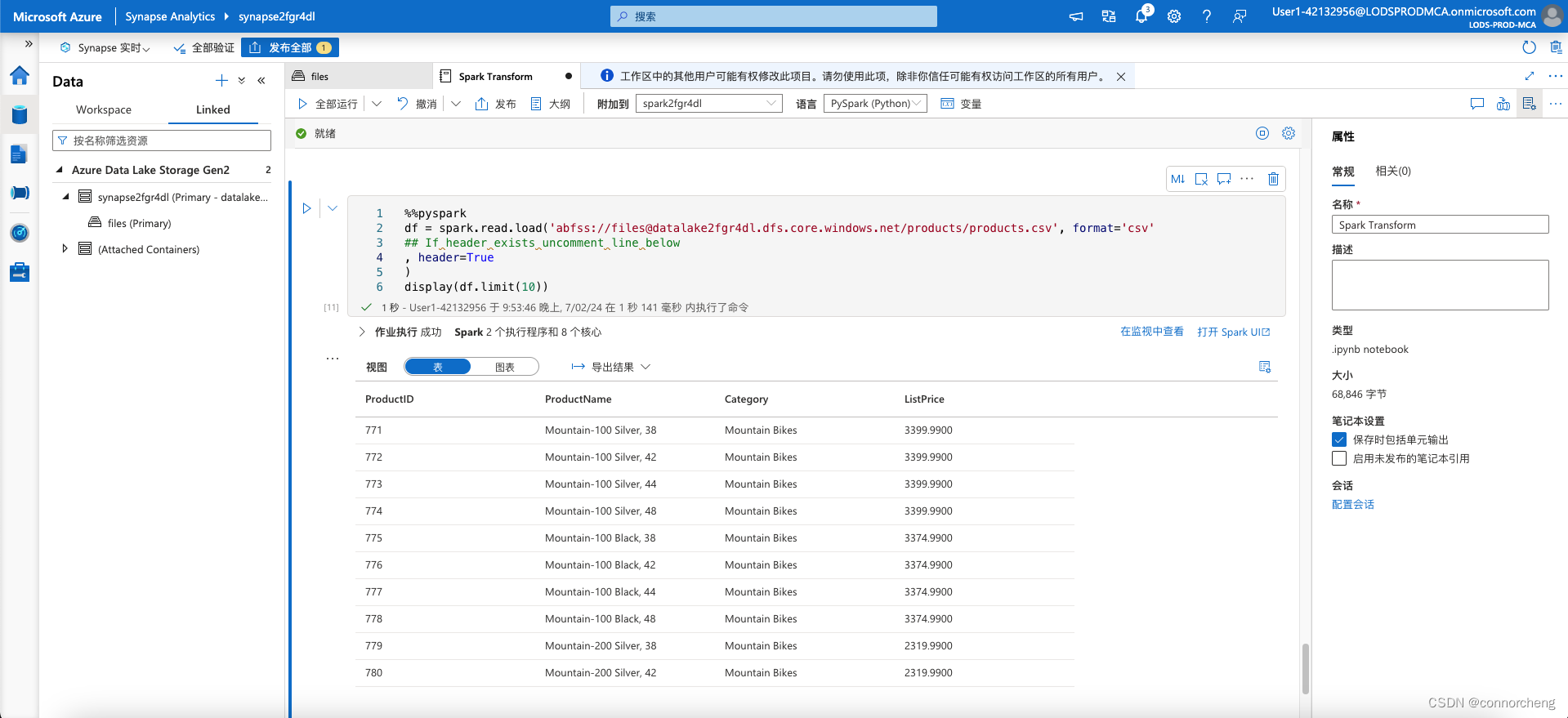
python
delta_table_path = "/delta/products-delta"
df.write.format("delta").save(delta_table_path)
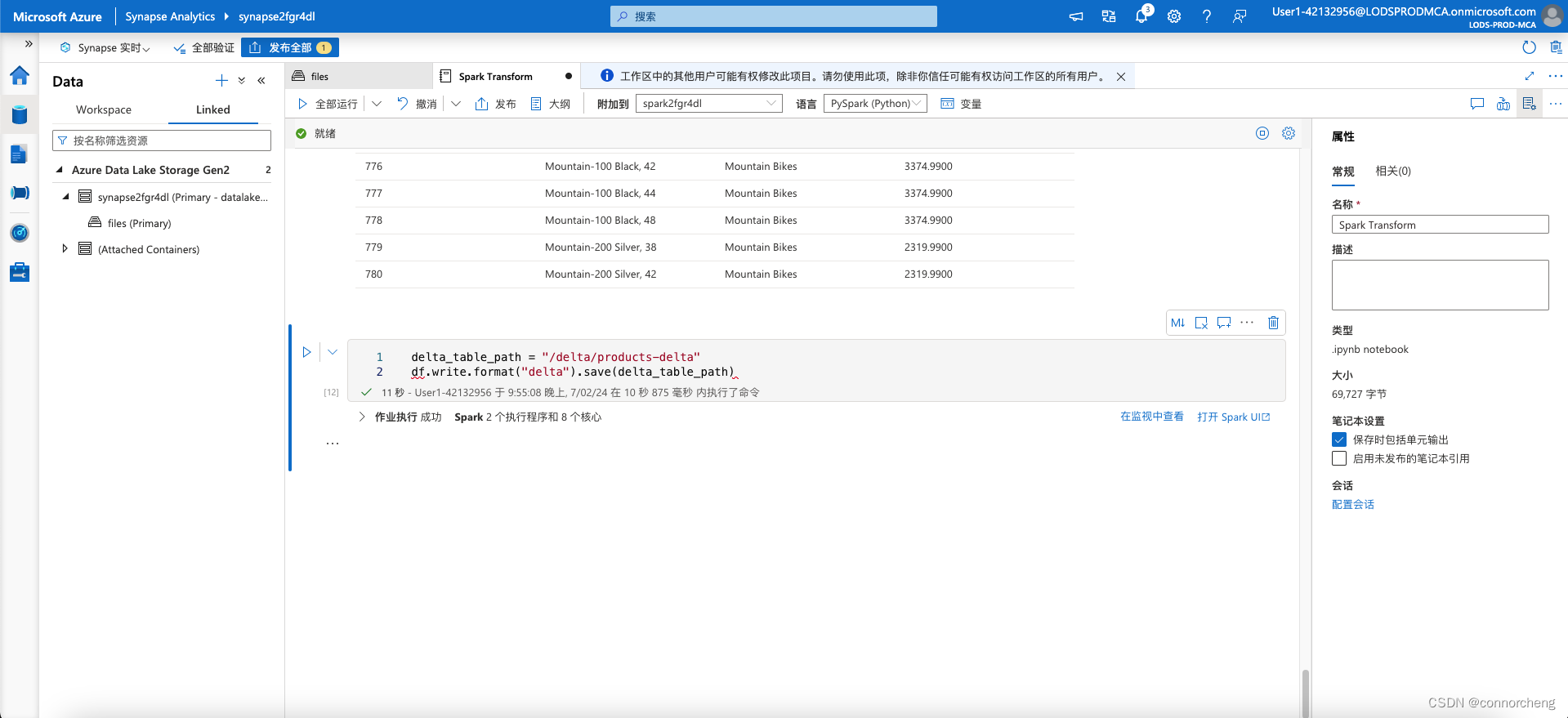
- On the files tab, use the ↑ icon in the toolbar to return to the root of the files container, and note that a new folder named delta has been created. Open this folder and the products-delta table it contains, where you should see the parquet format file(s) containing the data.
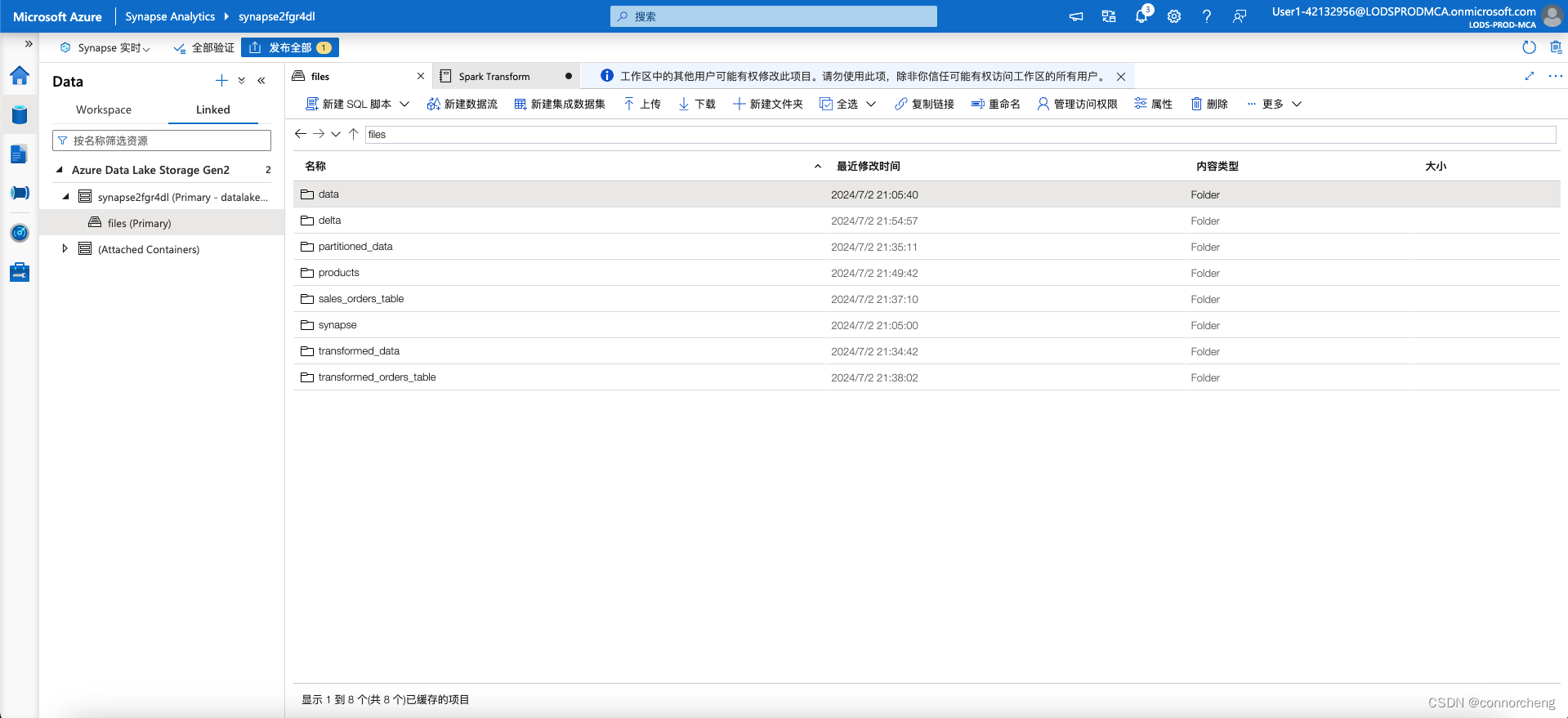
python
from delta.tables import *
from pyspark.sql.functions import *
# Create a deltaTable object
deltaTable = DeltaTable.forPath(spark, delta_table_path)
# Update the table (reduce price of product 771 by 10%)
deltaTable.update(
condition = "ProductID == 771",
set = { "ListPrice": "ListPrice * 0.9" })
# View the updated data as a dataframe
deltaTable.toDF().show(10)
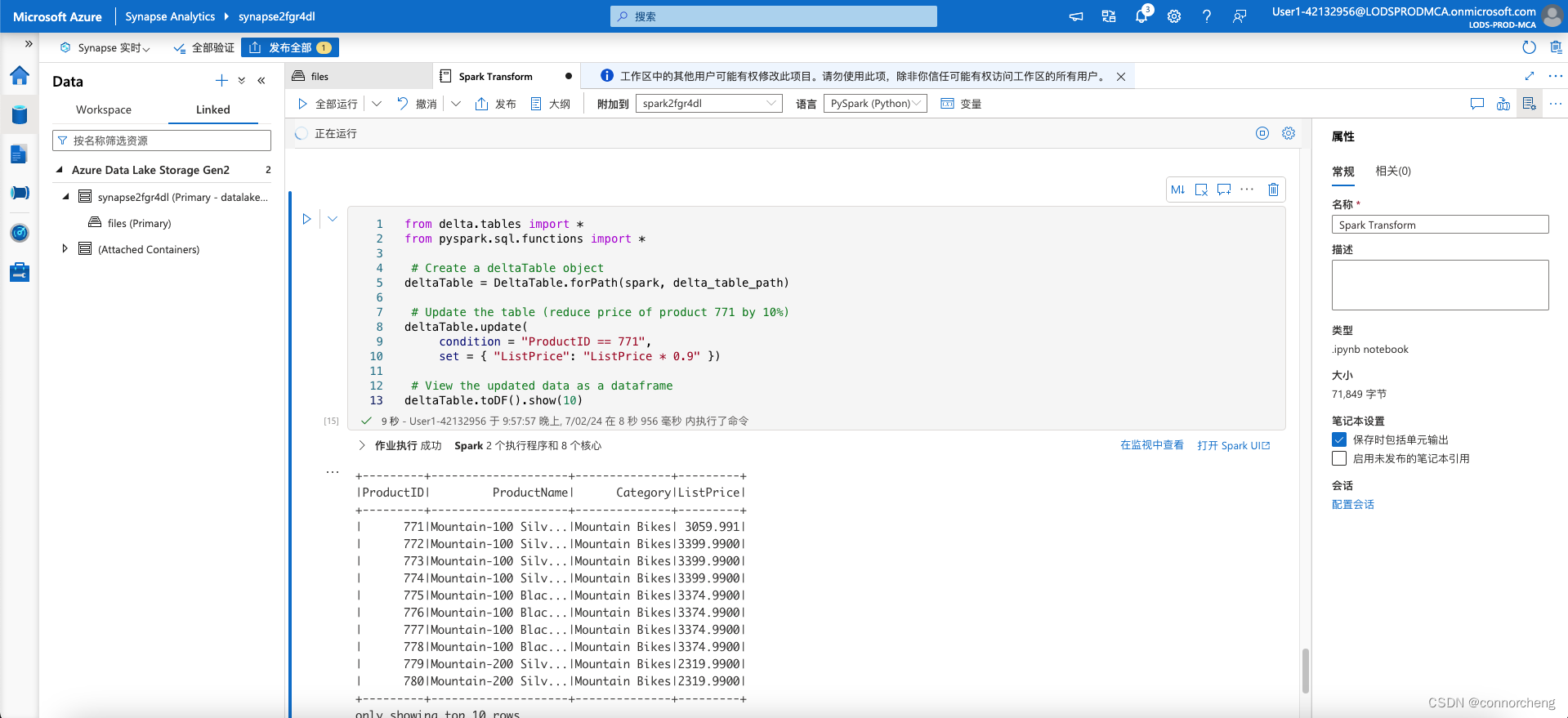
python
new_df = spark.read.format("delta").load(delta_table_path)
new_df.show(10)
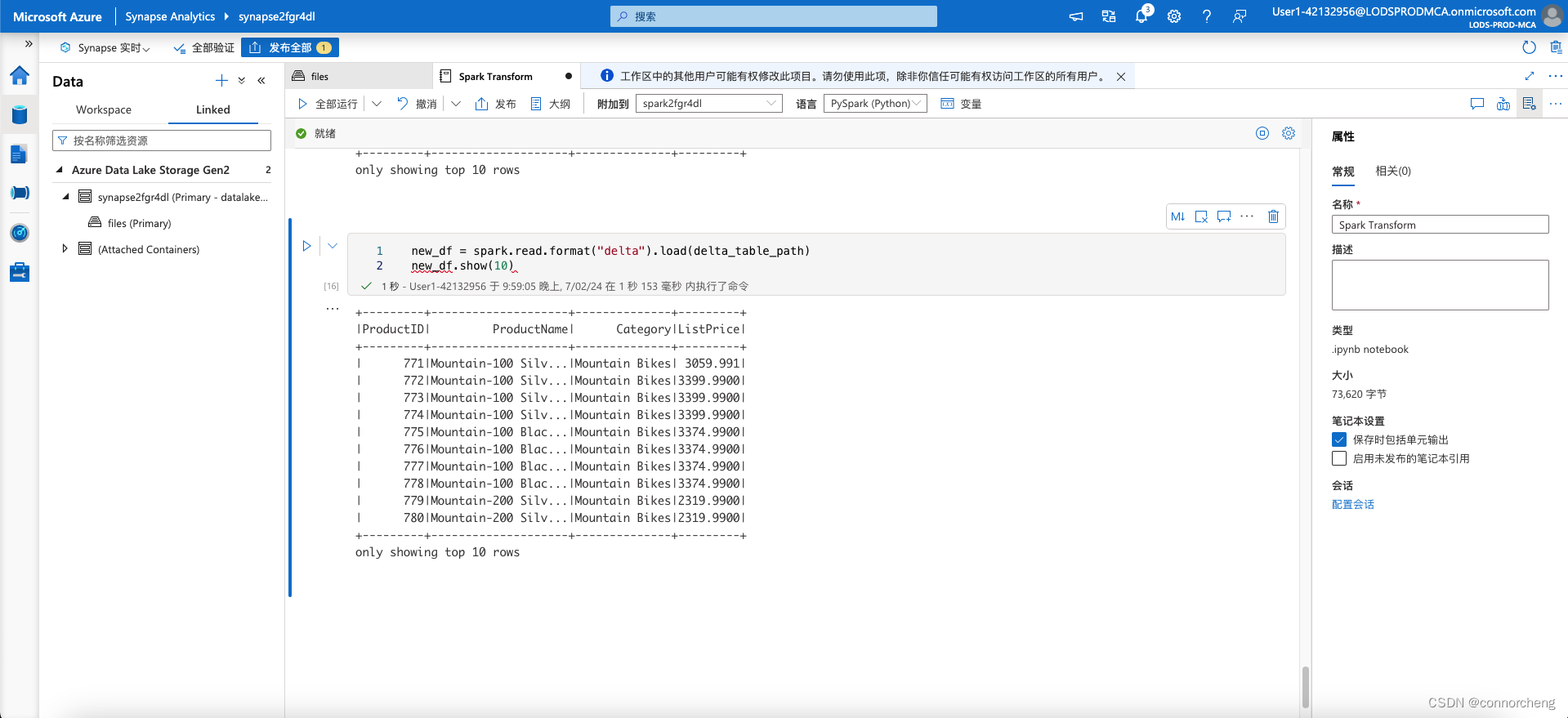
python
new_df = spark.read.format("delta").option("versionAsOf", 0).load(delta_table_path)
new_df.show(10)
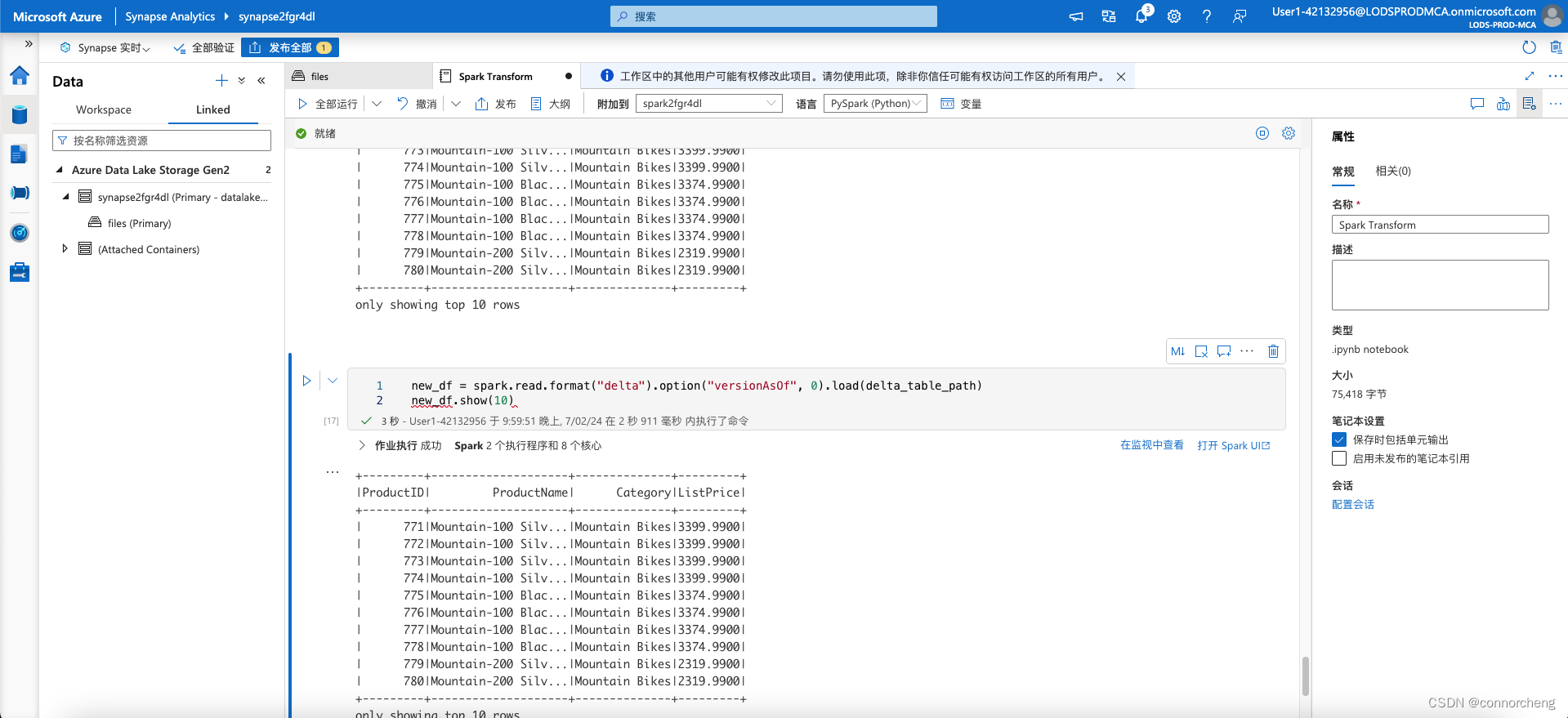
python
deltaTable.history(10).show(20, False, True)
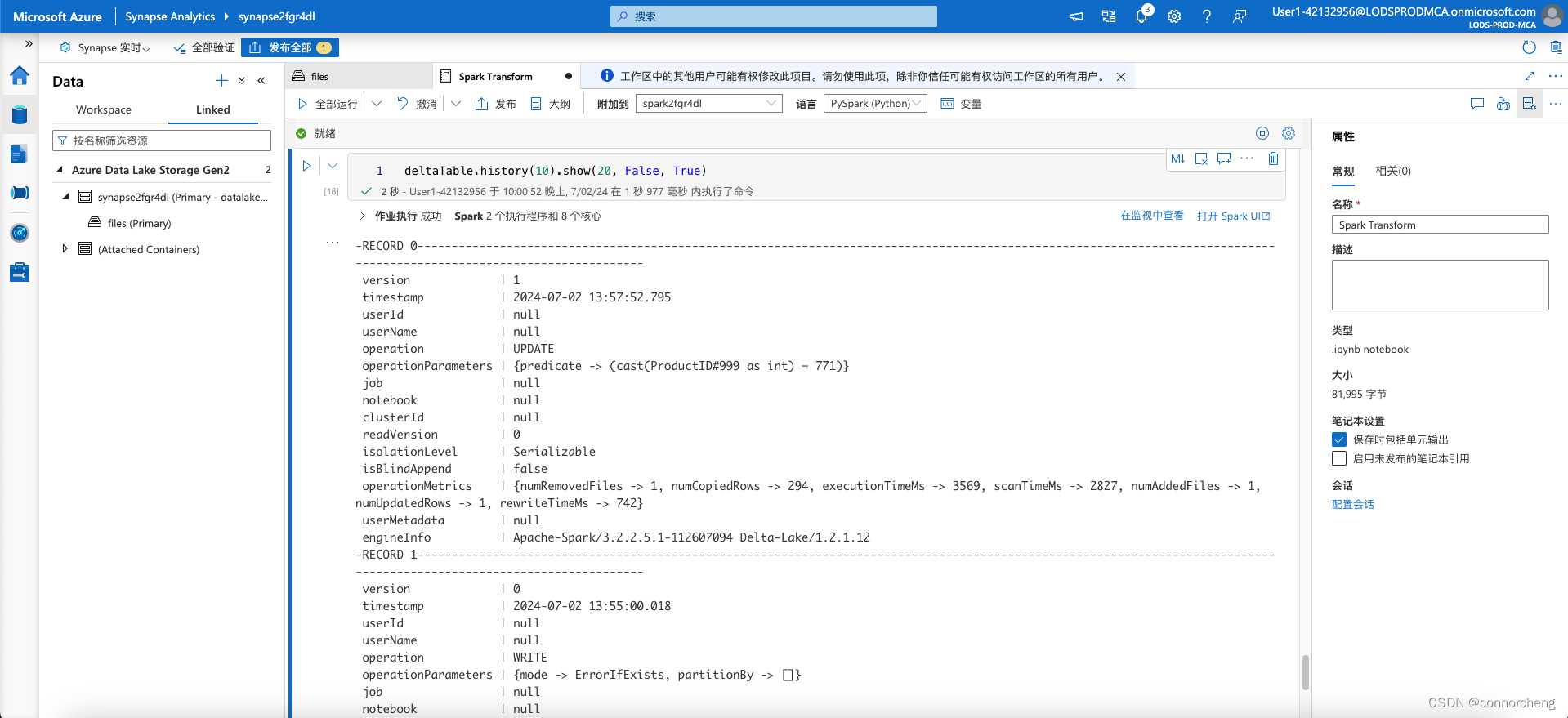
python
spark.sql("CREATE DATABASE AdventureWorks")
spark.sql("CREATE TABLE AdventureWorks.ProductsExternal USING DELTA LOCATION '{0}'".format(delta_table_path))
spark.sql("DESCRIBE EXTENDED AdventureWorks.ProductsExternal").show(truncate=False)
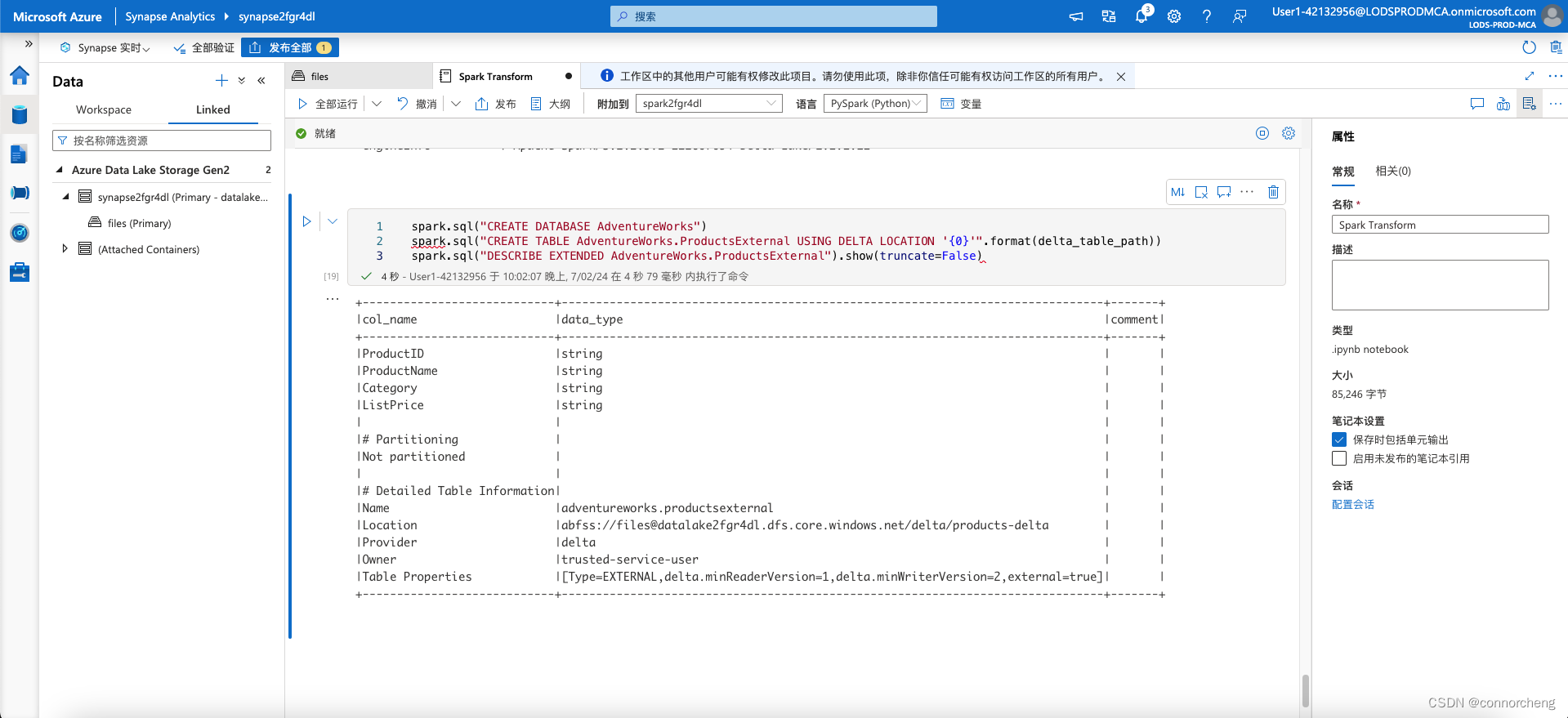
This code creates a new database named AdventureWorks and then creates an external tabled named ProductsExternal in that database based on the path to the parquet files you defined previously. It then displays a description of the table's properties. Note that the Location property is the path you specified.
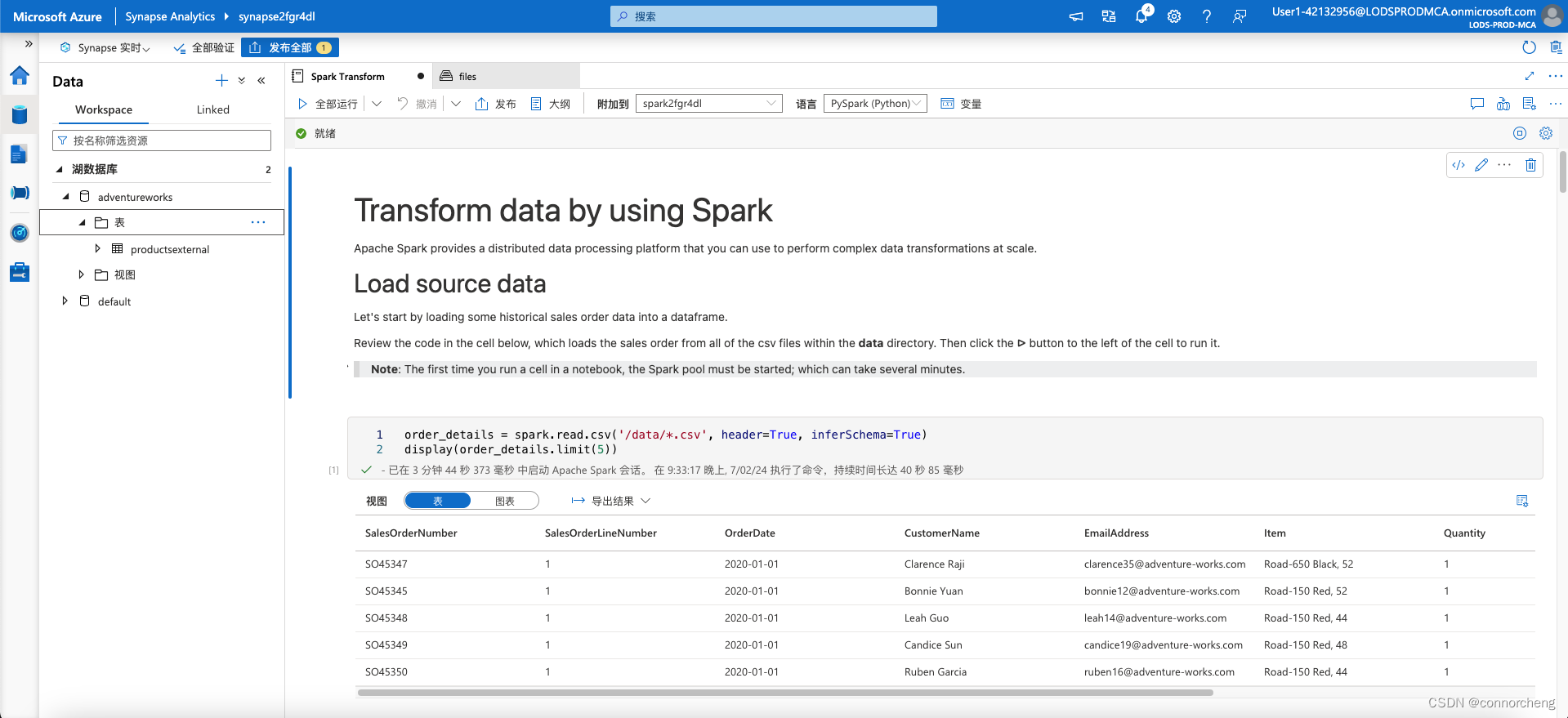
sql
%%sql
USE AdventureWorks;
SELECT * FROM ProductsExternal;
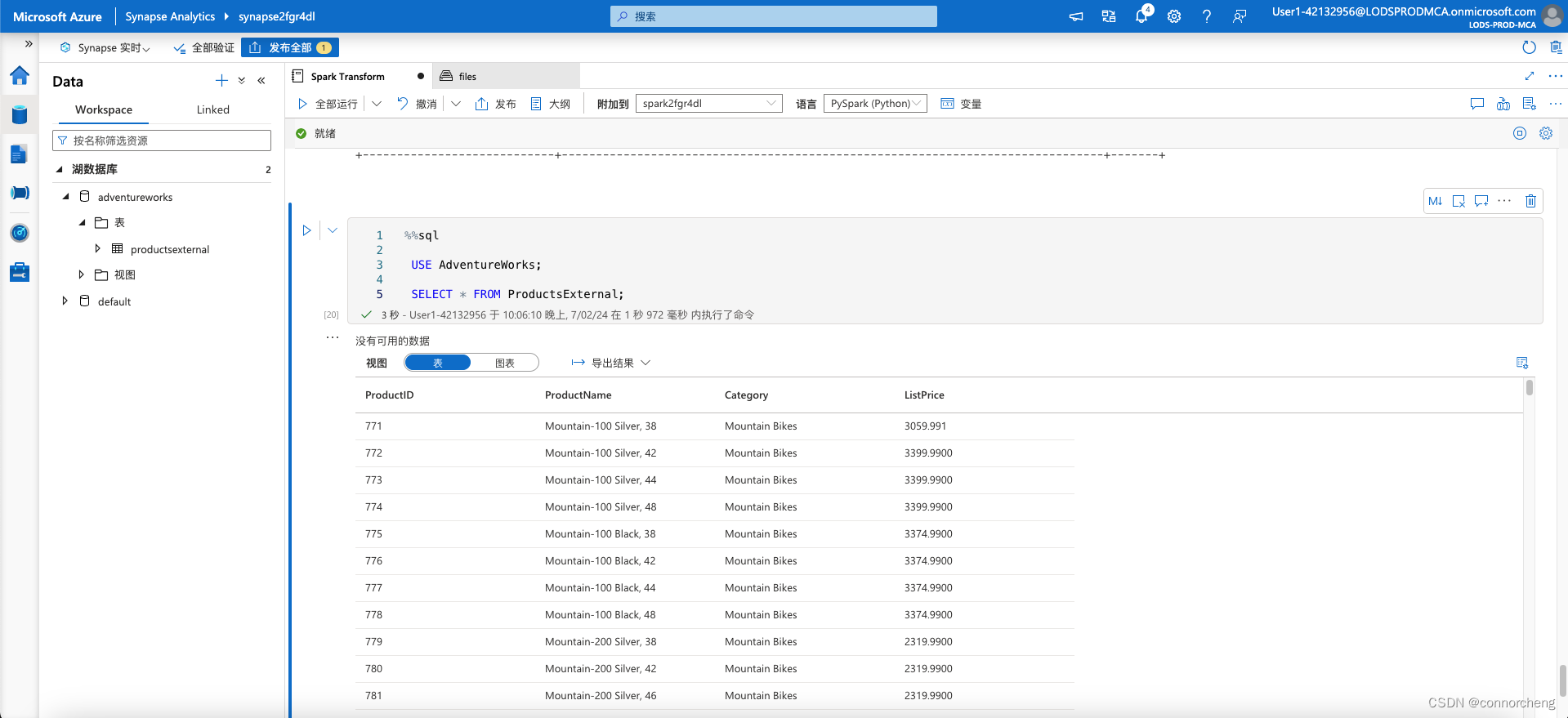
python
df.write.format("delta").saveAsTable("AdventureWorks.ProductsManaged")
spark.sql("DESCRIBE EXTENDED AdventureWorks.ProductsManaged").show(truncate=False)
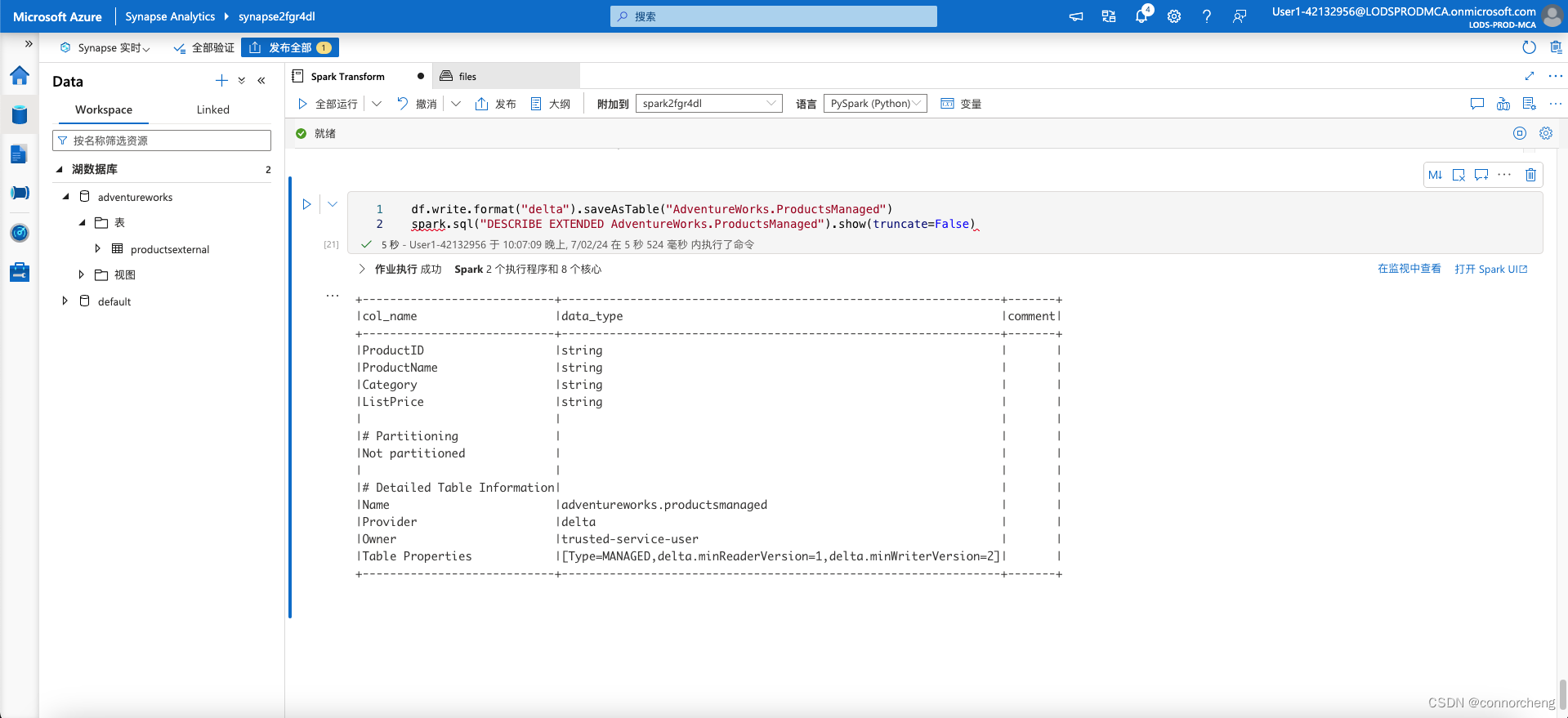
This code creates a managed tabled named ProductsManaged based on the DataFrame you originally loaded from the products.csv file (before you updated the price of product 771). You do not specify a path for the parquet files used by the table - this is managed for you in the Hive metastore, and shown in the Location property in the table description (in the files/synapse/workspaces/synapsexxxxxxx/warehouse path).
sql
%%sql
USE AdventureWorks;
SELECT * FROM ProductsManaged;
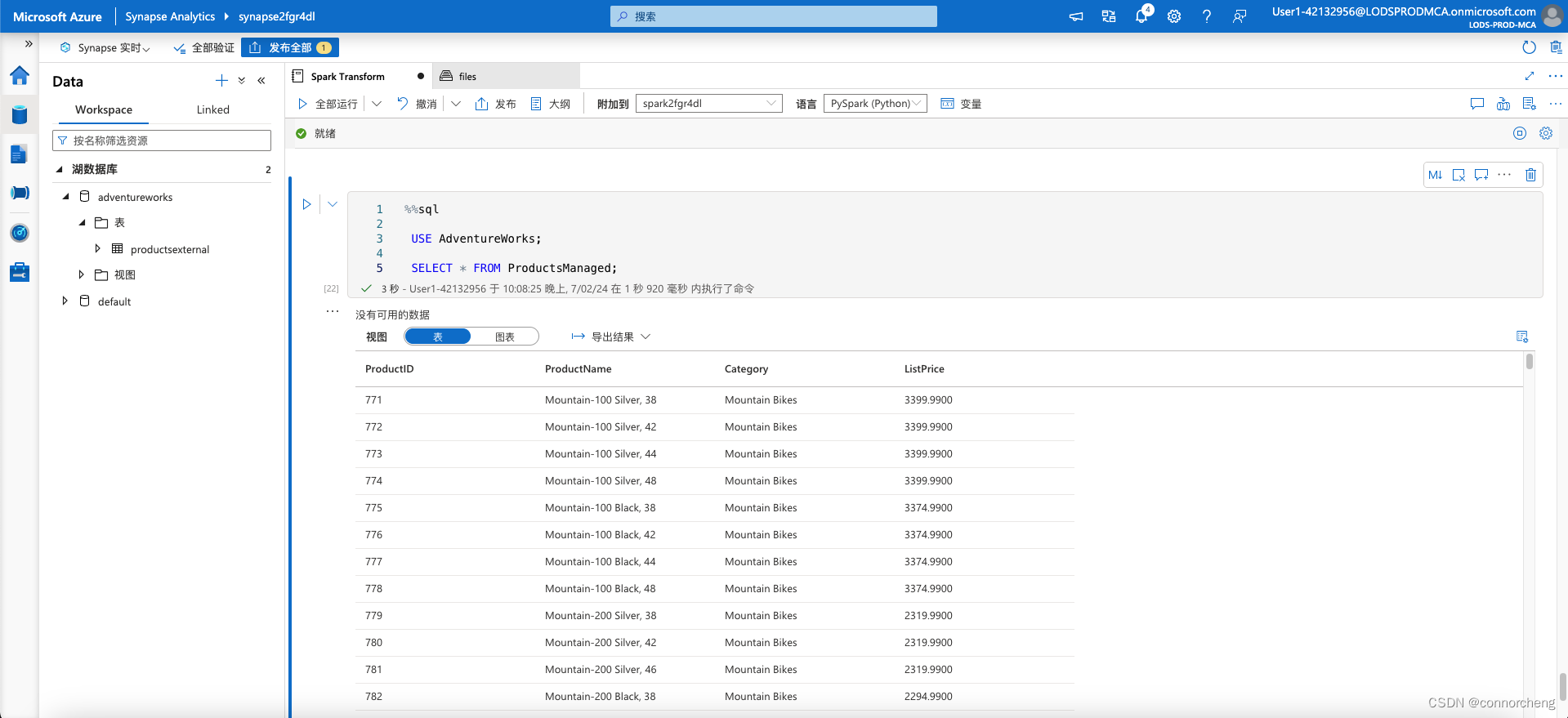
sql
%%sql
USE AdventureWorks;
SHOW TABLES;
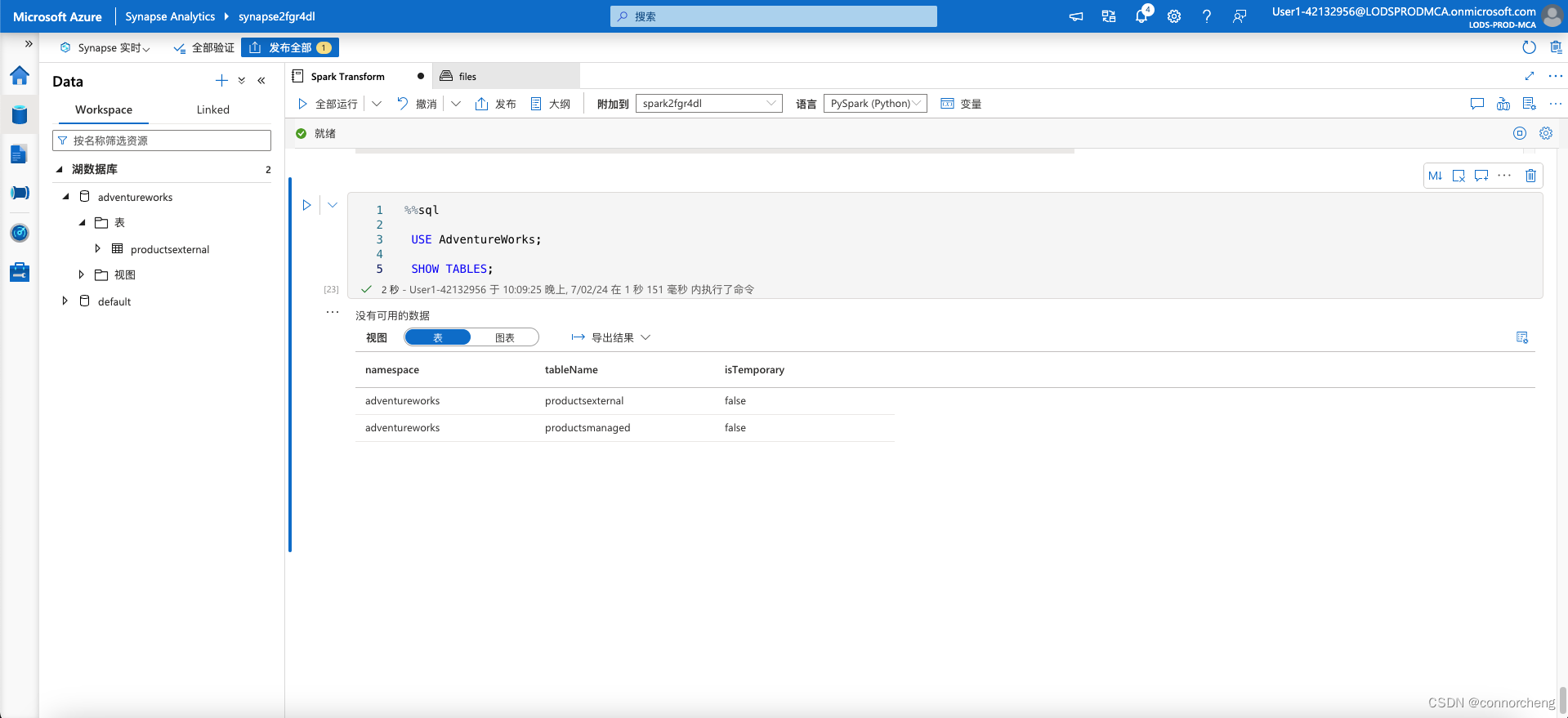
sql
%%sql
USE AdventureWorks;
DROP TABLE IF EXISTS ProductsExternal;
DROP TABLE IF EXISTS ProductsManaged;
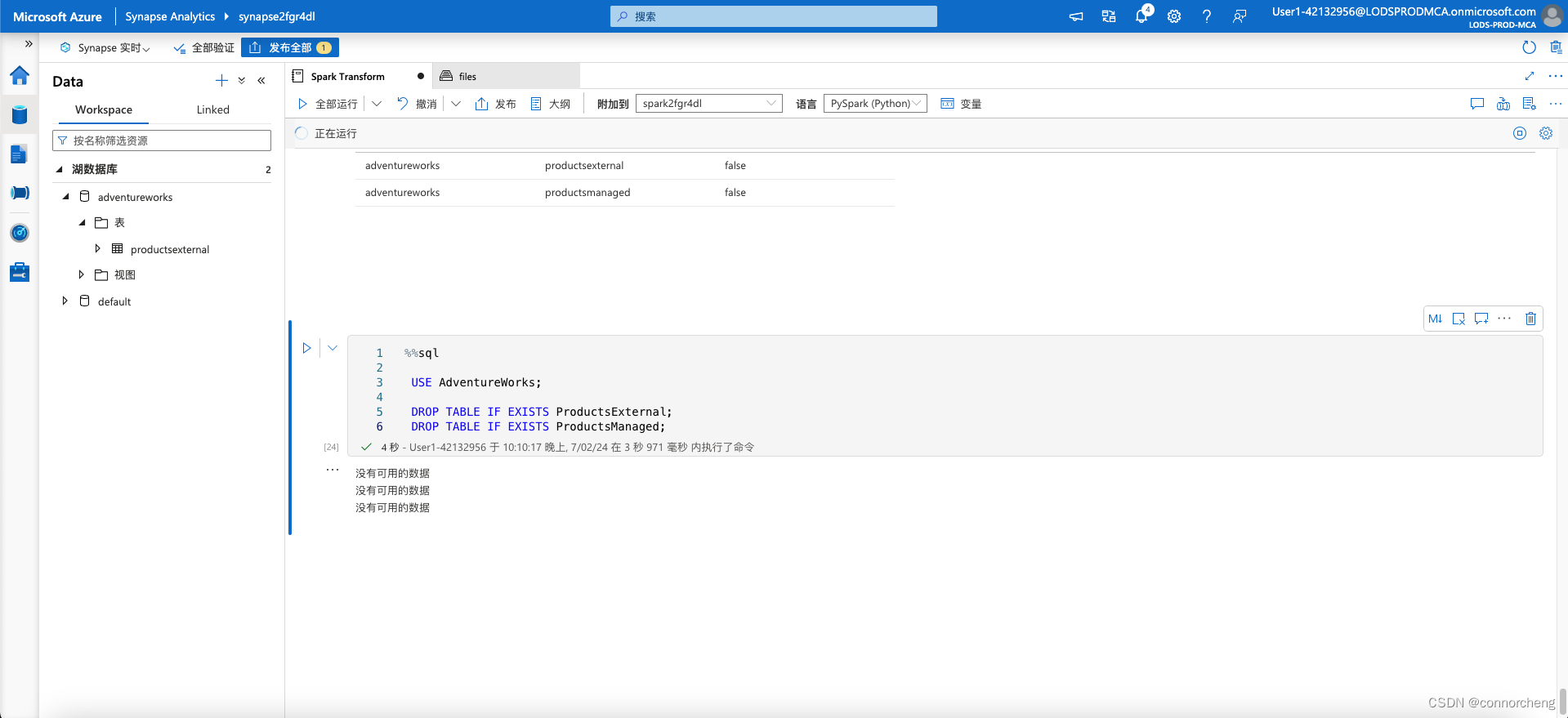
- Return to the files tab and view the files/delta/products-delta folder. Note that the data files still exist in this location. Dropping the external table has removed the table from the metastore, but left the data files intact.
- View the files/synapse/workspaces/synapsexxxxxxx/warehouse folder, and note that there is no folder for the ProductsManaged table data. Dropping a managed table removes the table from the metastore and also deletes the table's data files.
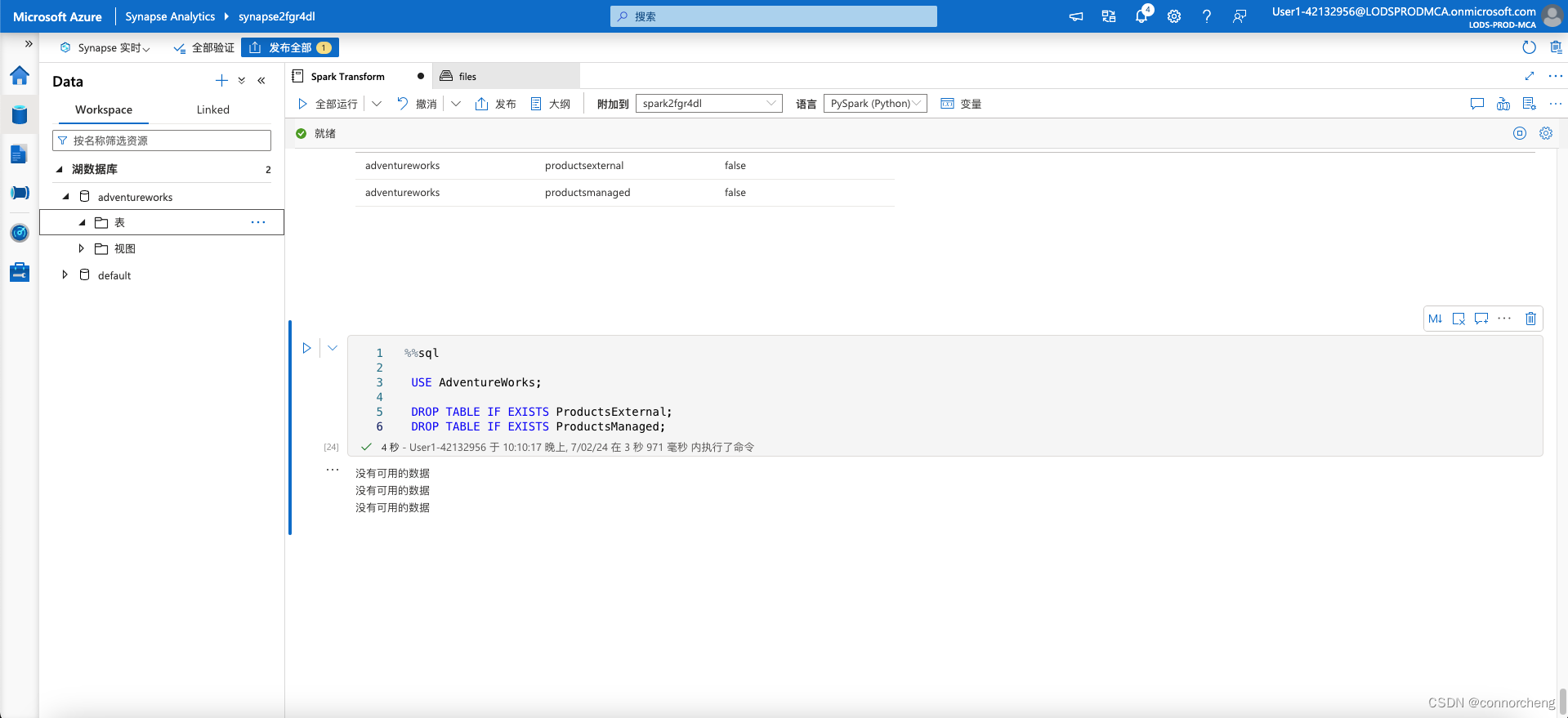
sql
%%sql
USE AdventureWorks;
CREATE TABLE Products
USING DELTA
LOCATION '/delta/products-delta';
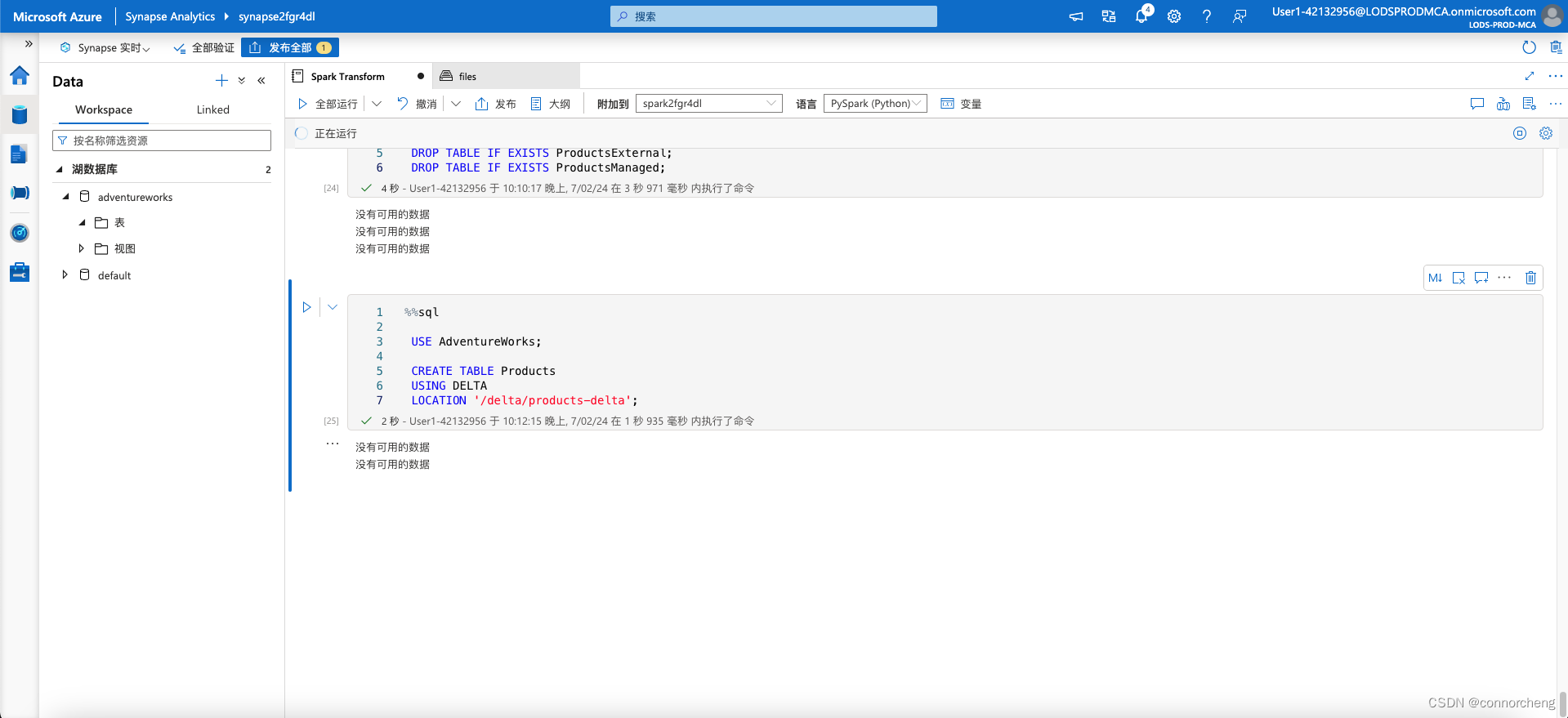
sql
%%sql
USE AdventureWorks;
SELECT * FROM Products;
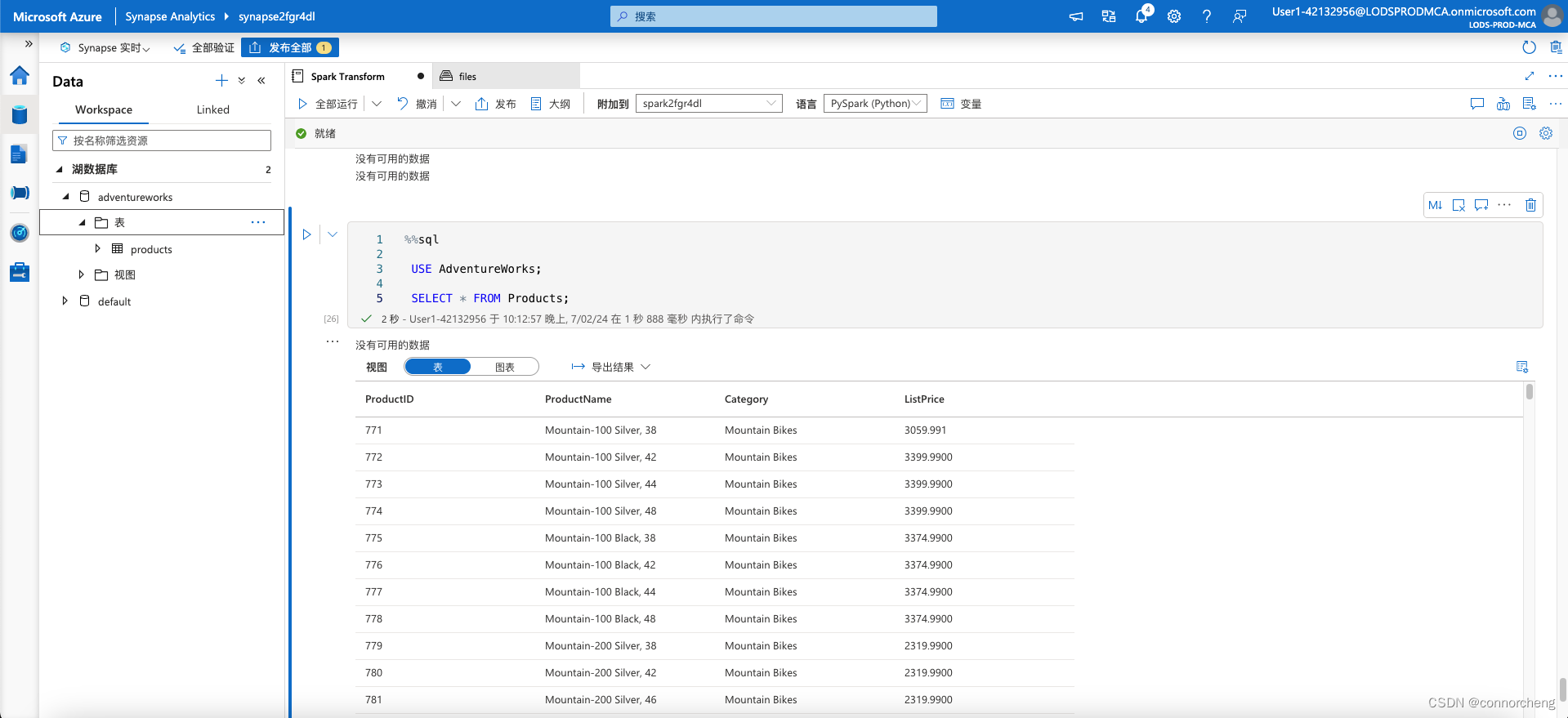
python
from notebookutils import mssparkutils
from pyspark.sql.types import *
from pyspark.sql.functions import *
# Create a folder
inputPath = '/data/'
mssparkutils.fs.mkdirs(inputPath)
# Create a stream that reads data from the folder, using a JSON schema
jsonSchema = StructType([
StructField("device", StringType(), False),
StructField("status", StringType(), False)
])
iotstream = spark.readStream.schema(jsonSchema).option("maxFilesPerTrigger", 1).json(inputPath)
# Write some event data to the folder
device_data = '''{"device":"Dev1","status":"ok"}
{"device":"Dev1","status":"ok"}
{"device":"Dev1","status":"ok"}
{"device":"Dev2","status":"error"}
{"device":"Dev1","status":"ok"}
{"device":"Dev1","status":"error"}
{"device":"Dev2","status":"ok"}
{"device":"Dev2","status":"error"}
{"device":"Dev1","status":"ok"}'''
mssparkutils.fs.put(inputPath + "data.txt", device_data, True)
print("Source stream created...")
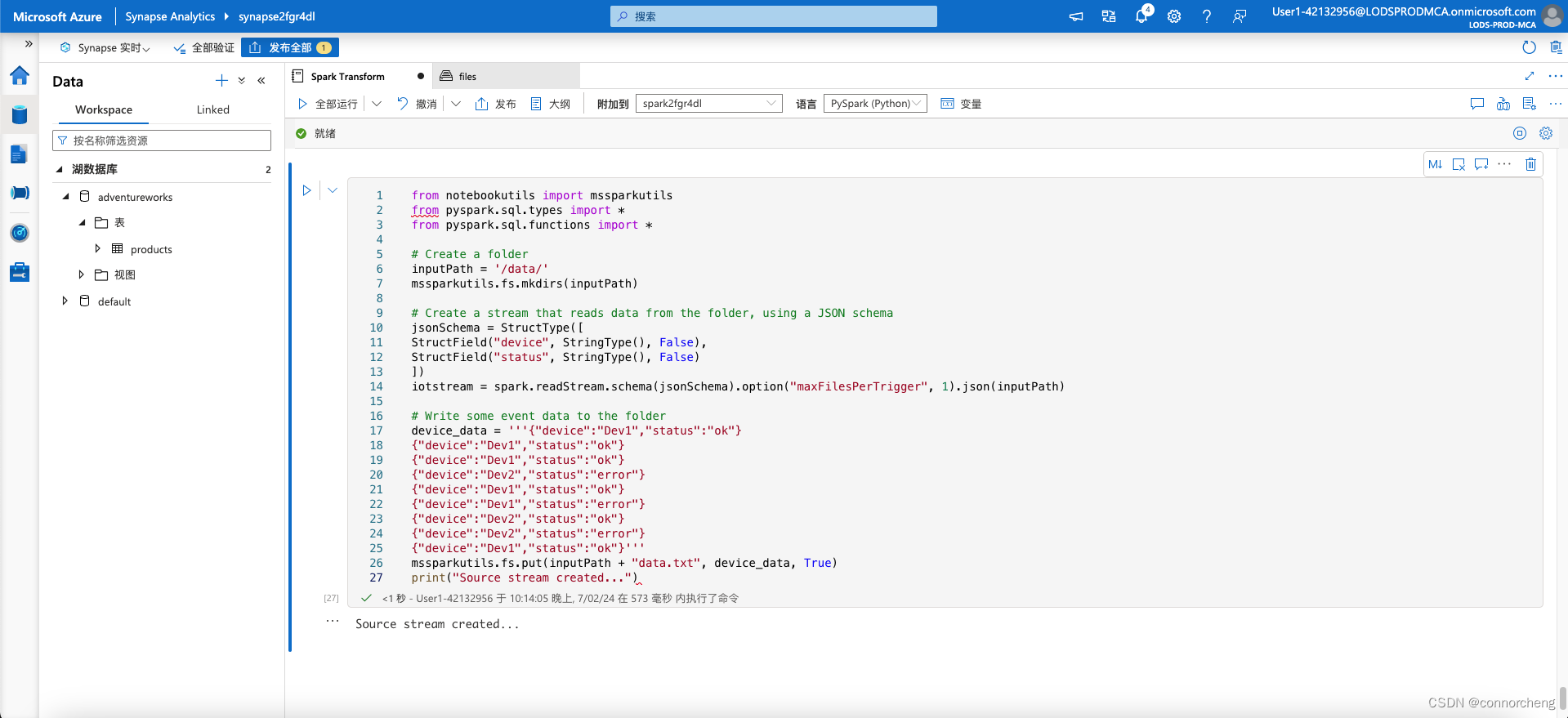
Ensure the message Source stream created... is printed. The code you just ran has created a streaming data source based on a folder to which some data has been saved, representing readings from hypothetical IoT devices.
python
# Write the stream to a delta table
delta_stream_table_path = '/delta/iotdevicedata'
checkpointpath = '/delta/checkpoint'
deltastream = iotstream.writeStream.format("delta").option("checkpointLocation", checkpointpath).start(delta_stream_table_path)
print("Streaming to delta sink...")
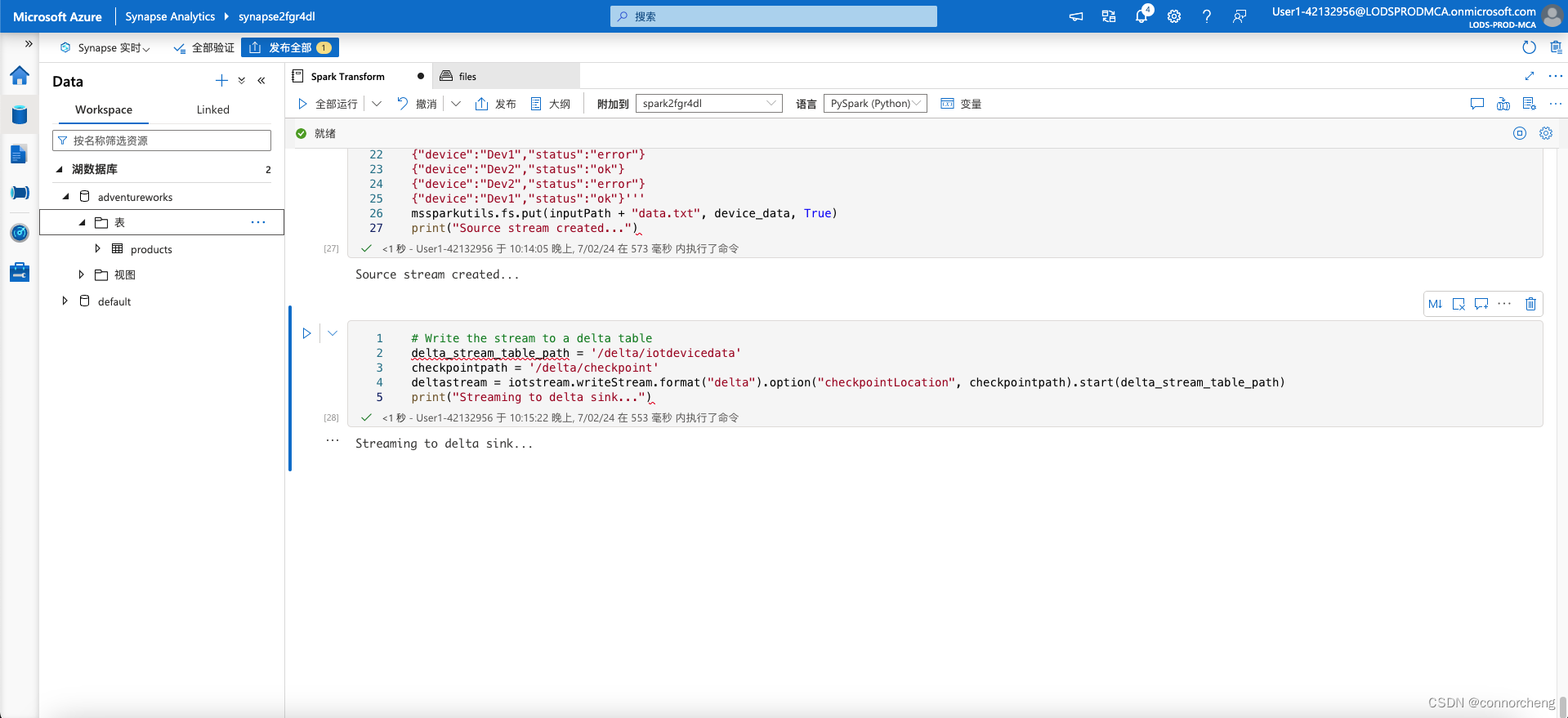
python
# Read the data in delta format into a dataframe
df = spark.read.format("delta").load(delta_stream_table_path)
display(df)
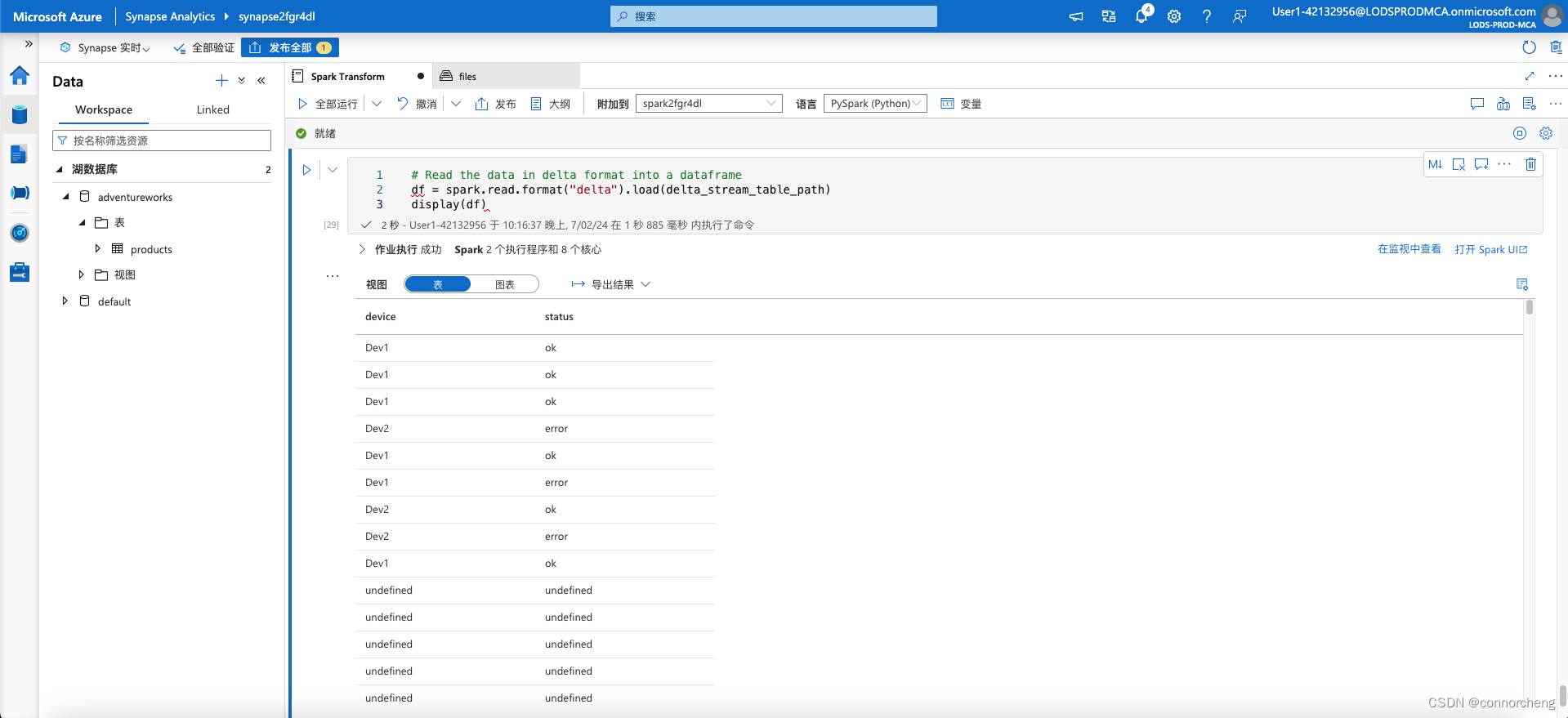
python
# create a catalog table based on the streaming sink
spark.sql("CREATE TABLE IotDeviceData USING DELTA LOCATION '{0}'".format(delta_stream_table_path))
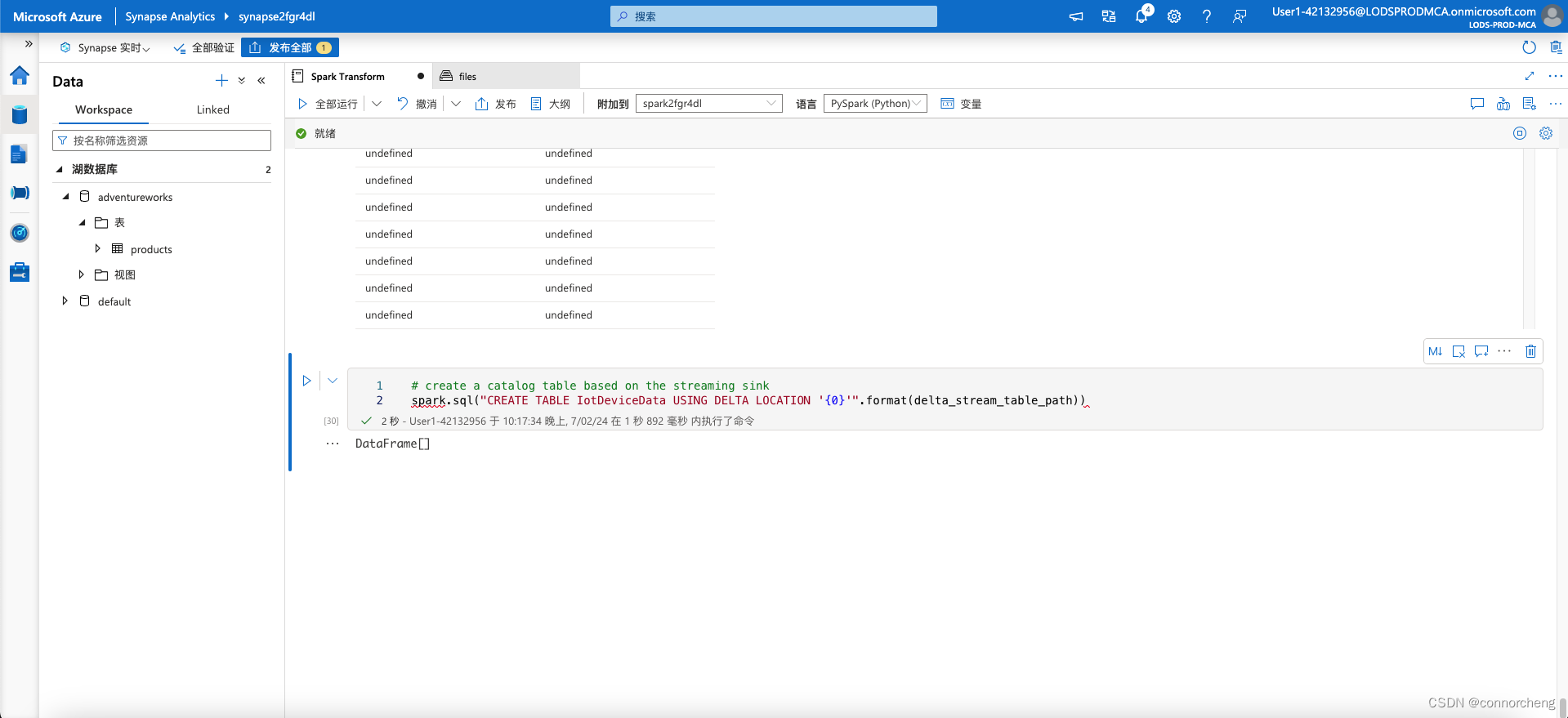
sql
%%sql
SELECT * FROM IotDeviceData;
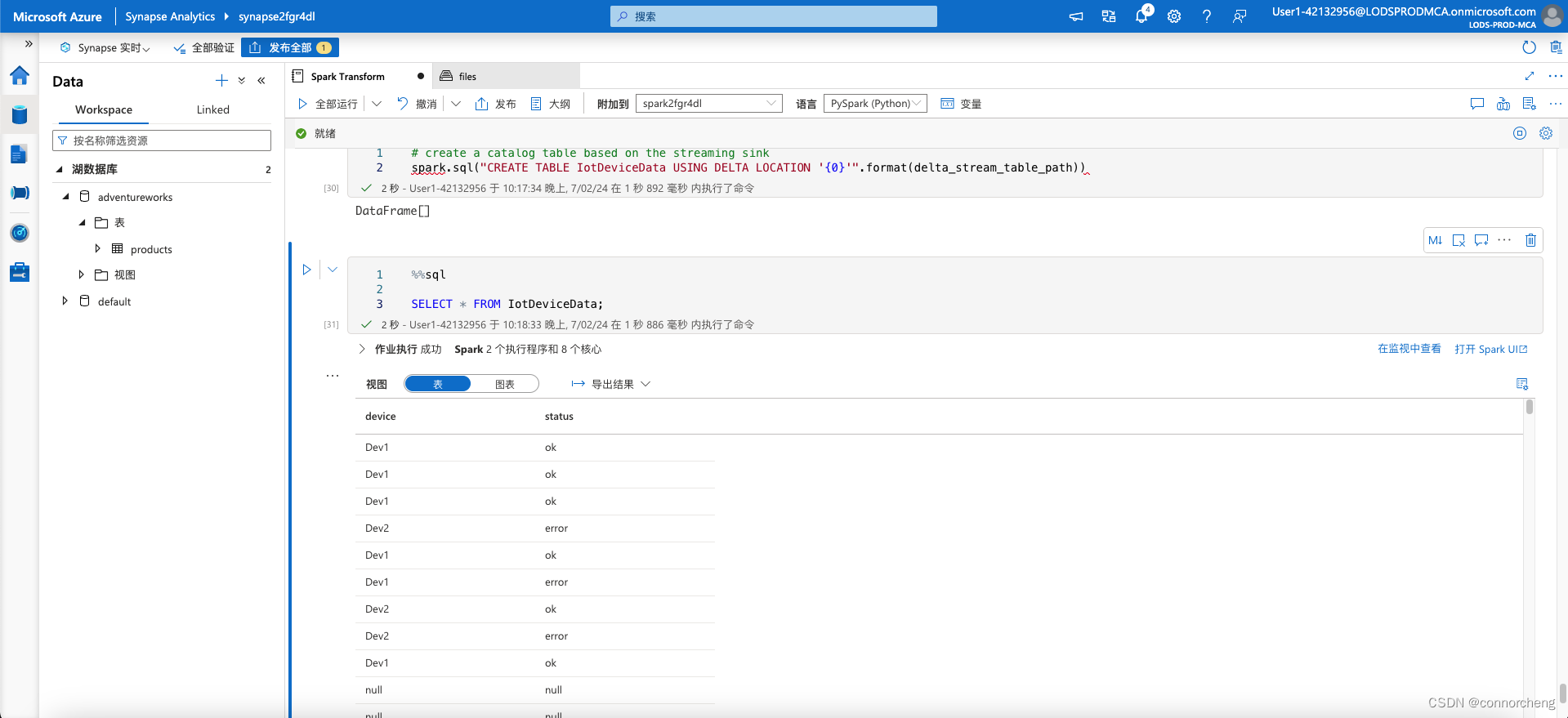
python
# Add more data to the source stream
more_data = '''{"device":"Dev1","status":"ok"}
{"device":"Dev1","status":"ok"}
{"device":"Dev1","status":"ok"}
{"device":"Dev1","status":"ok"}
{"device":"Dev1","status":"error"}
{"device":"Dev2","status":"error"}
{"device":"Dev1","status":"ok"}'''
mssparkutils.fs.put(inputPath + "more-data.txt", more_data, True)
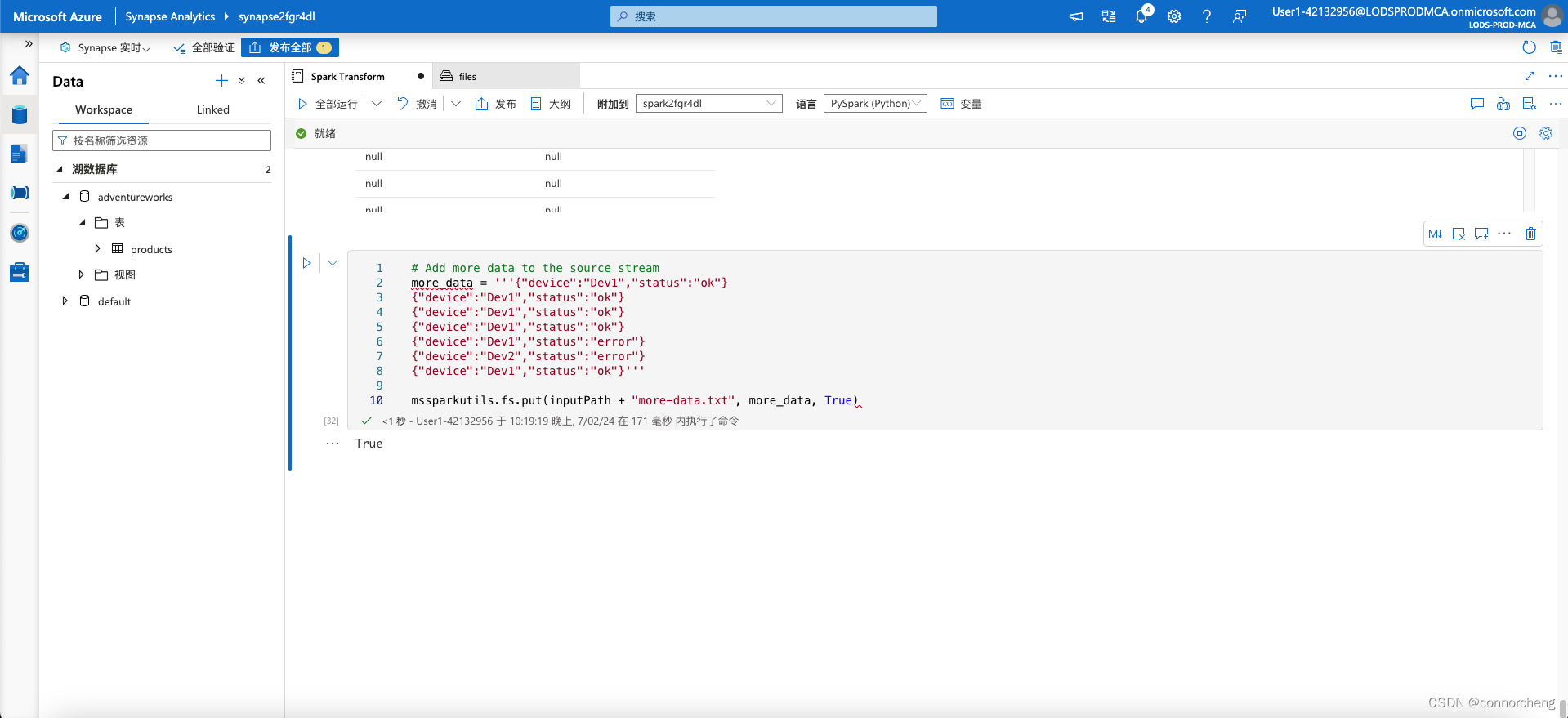
sql
%%sql
SELECT * FROM IotDeviceData;
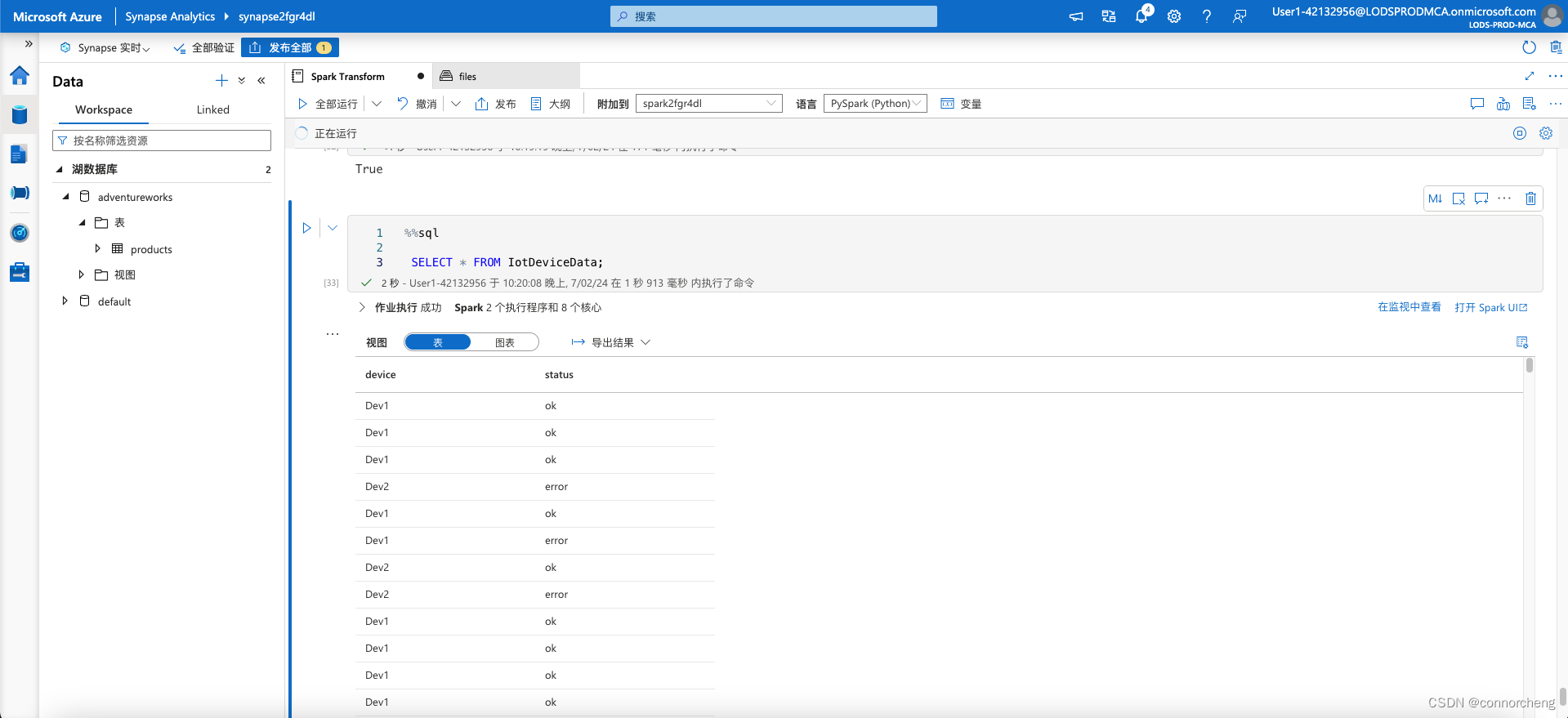
python
deltastream.stop()
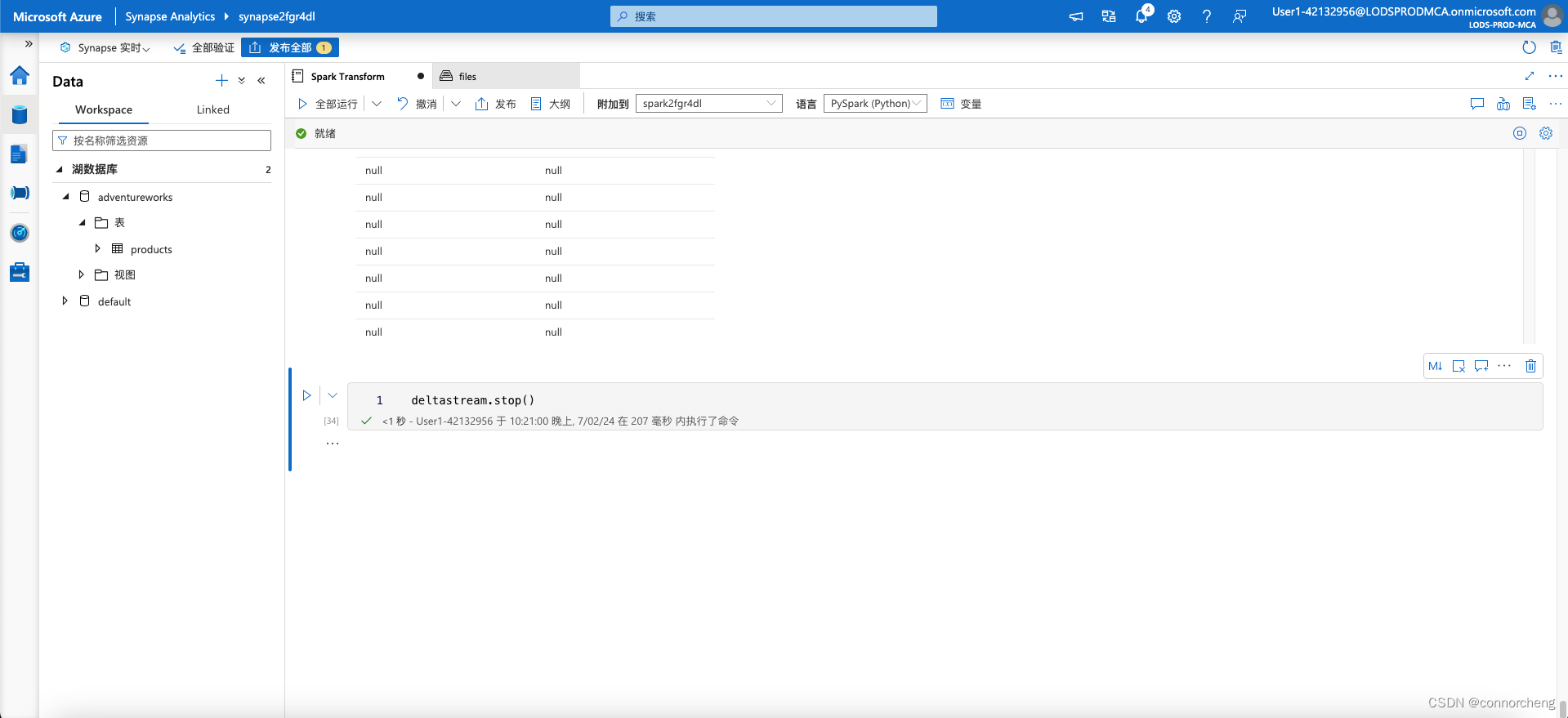
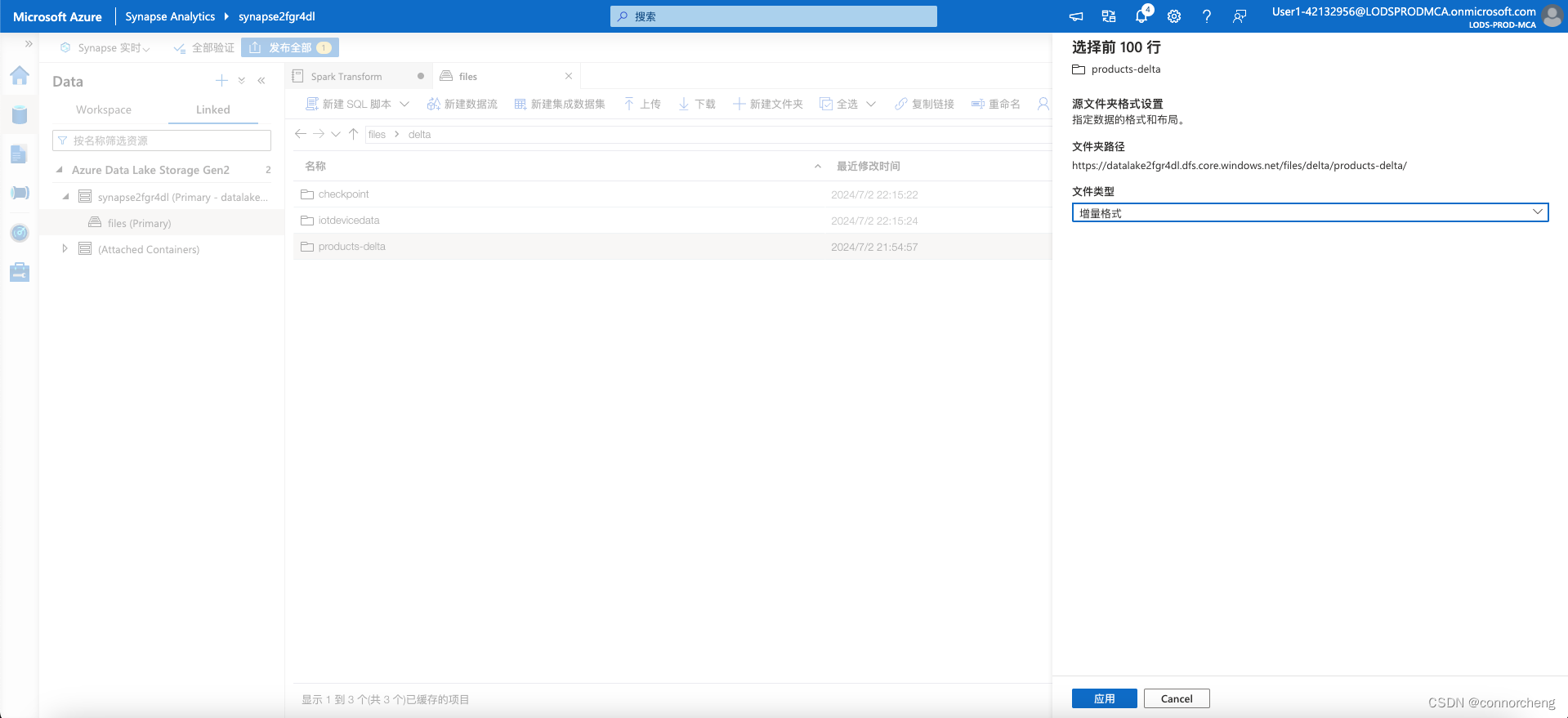
sql
-- This is auto-generated code
SELECT
TOP 100 *
FROM
OPENROWSET(
BULK 'https://datalakexxxxxxx.dfs.core.windows.net/files/delta/products-delta/',
FORMAT = 'DELTA'
) AS [result]
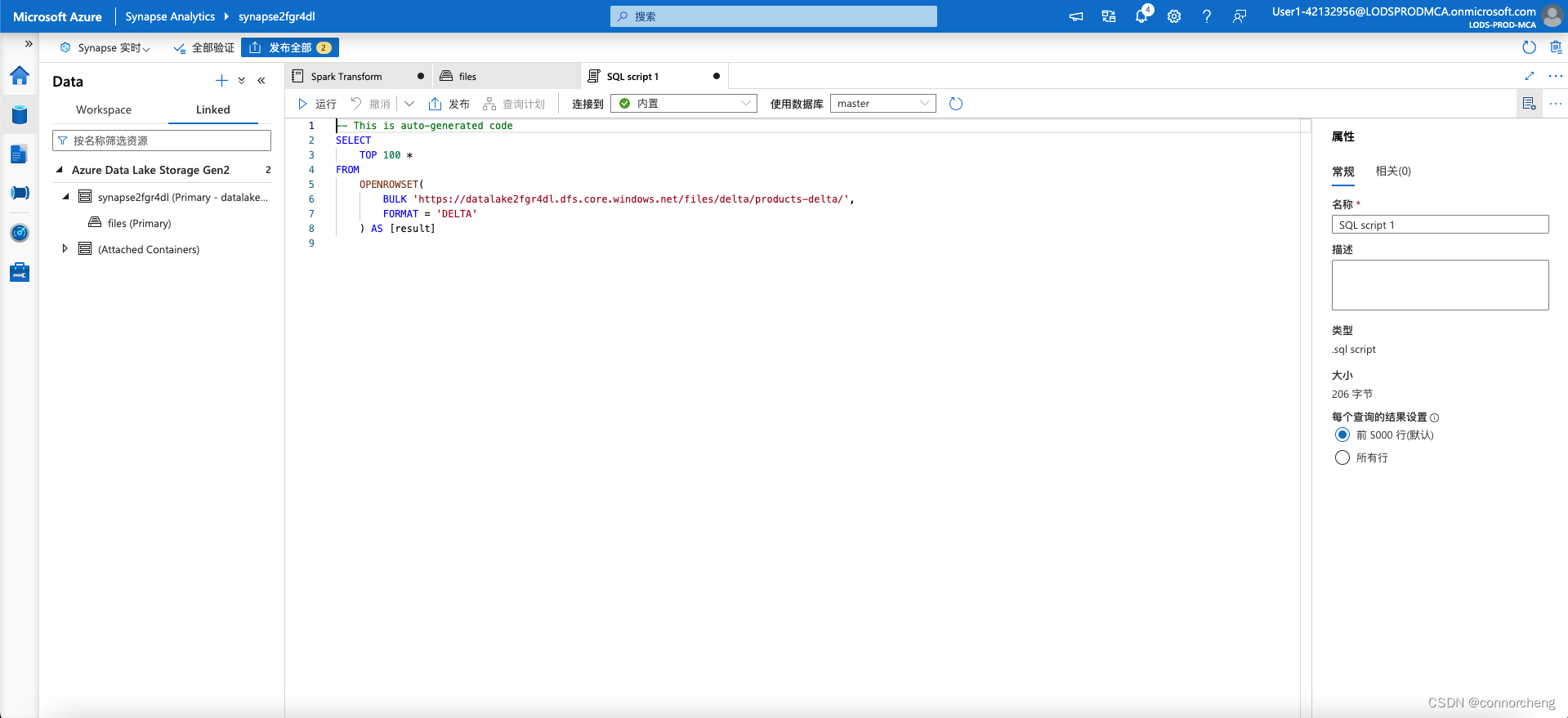
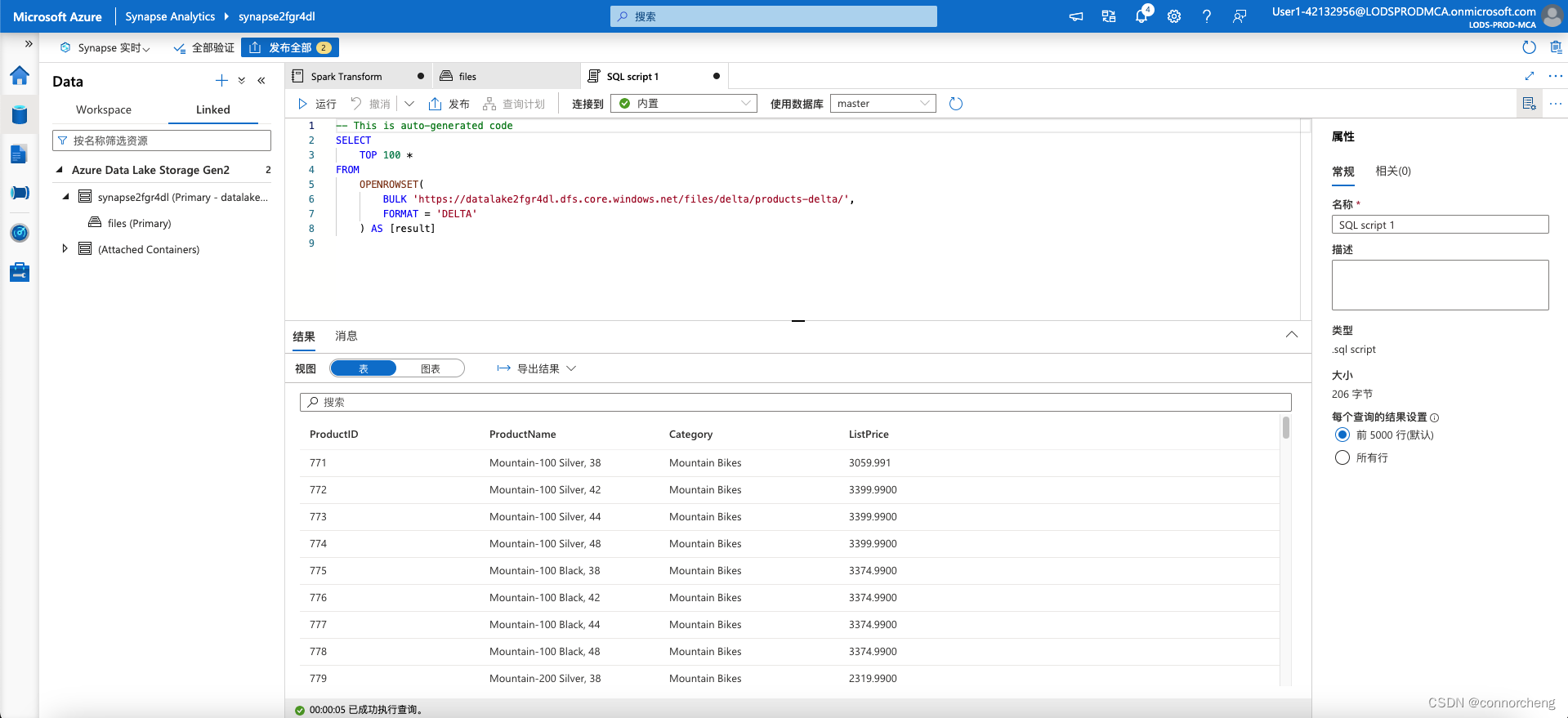
sql
USE AdventureWorks;
SELECT * FROM Products;
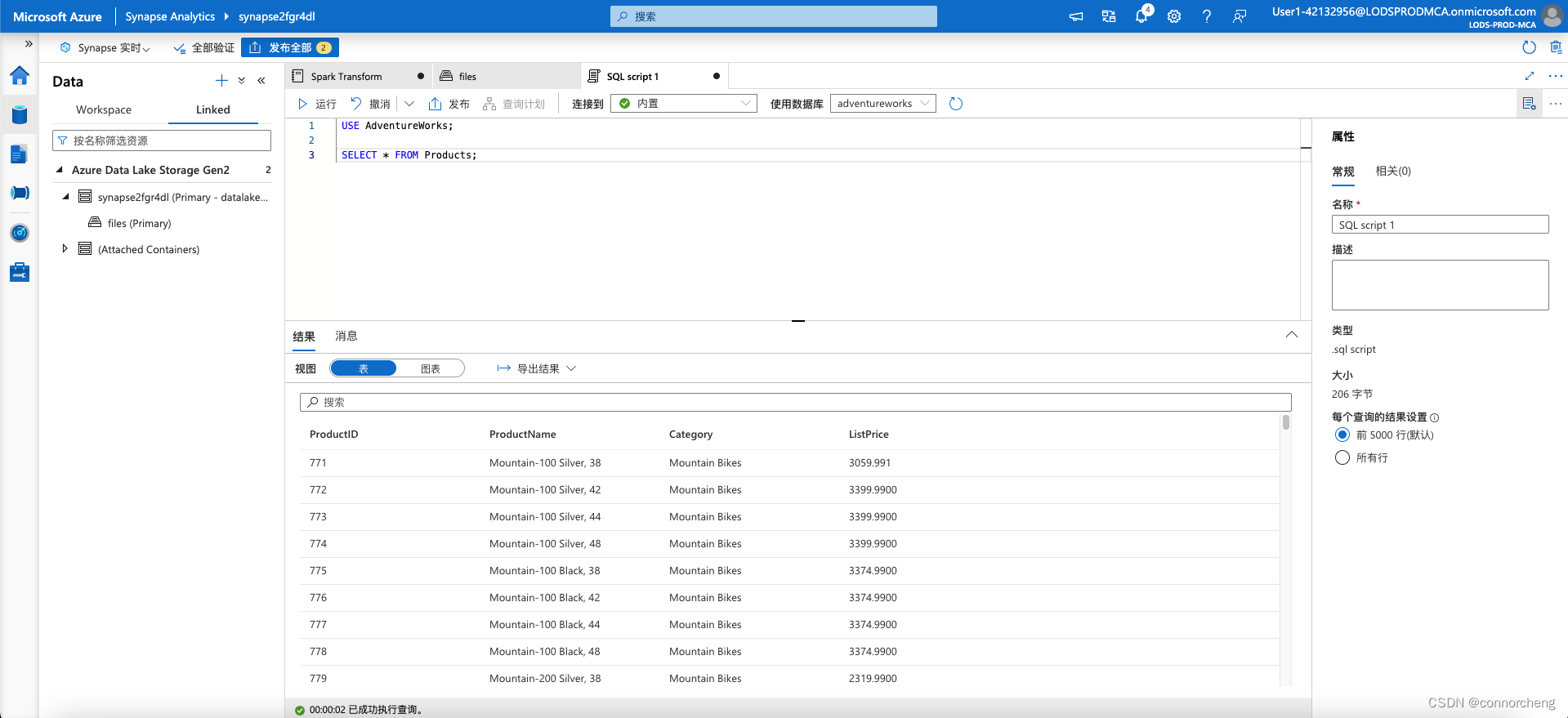
Run the code and observe that you can also use the serverless SQL pool to query Delta Lake data in catalog tables that are defined the Spark metastore.