1. 项目介绍
杂草是农业中的主要问题之一,对作物生长和产量造成严重威胁。传统的手动识别和管理方式效率低下且不够精确,因此需要借助先进的计算机视觉技术来提升农业生产的效率和质量。ResNet作为一种深度学习模型,在处理复杂的图像分类任务中表现出色,不仅可以有效解决农田中杂草多样化、形态复杂的问题,还能够推动农业智能化发展,减少对化学农药的依赖,实现农业可持续发展的目标。通过利用ResNet进行杂草分类,可以为农民提供更智能、精确的农业管理方案,促进农业生产效率的提升和农业产业的现代化进程。因此,本项目使用国产化框架PaddleClas+Swanlab+Gradio+Swanhub进行杂草分类实验。
PaddlePaddle(飞桨)是百度开发的企业级深度学习平台,旨在支持从模型开发到部署的全流程深度学习应用。它提供了丰富的工具和库,支持多种深度学习任务,包括图像处理、自然语言处理、语音识别等。PaddlePaddle
PaddleClas是Paddle框架中专门用于图像分类任务的工具库。它提供了一整套端到端的解决方案,包括数据处理、模型定义、训练、评估和部署,旨在帮助开发者快速构建和部署高效的图像分类模型。PaddleClas
SwanLab是一款开源、轻量级的AI实验跟踪工具,通过提供友好的API,结合超参数跟踪、指标记录、在线协作等功能,提高ML实验跟踪和协作体验。欢迎使用SwanLab | SwanLab官方文档
Swanhub是由极客工作室开发的一个开源模型协作分享社区。它为AI开发者提供了AI模型托管、训练记录、模型结果展示、API快速部署等功能。欢迎使用Swanhub
Gradio是一个开源的Python库,旨在帮助数据科学家、研究人员和从事机器学习领域的开发人员快速创建和共享用于机器学习模型的用户界面。Gradio
2. 准备部分
2.1 环境安装
安装以下3个库:
paddle
swanlab
gradio
安装命令:
pip install paddle swanlab gradio
2.2 下载数据集
杂草分类数据集:DeepWeeds
DeepWeeds
--images
----1.jpg
----2.jpg
--train.txt
--val.txt
--test.txt
--classnames.txt
它们各自的作用与意义:
-
DeepWeeds文件夹:该文件夹用于存储图片文件夹images、训练集测试集验证集文件和标签文件
-
images文件夹:该文件夹用于保存训练、测试、验证图片文件夹。
-
train.txt、val.txt、test.txt文件:该文件用于保存训练、测试、验证集图片路径与类别。
-
classnames文件:用于保存类别标签
2.3 下载PaddleClas框架
模型链接:PaddleClas模型
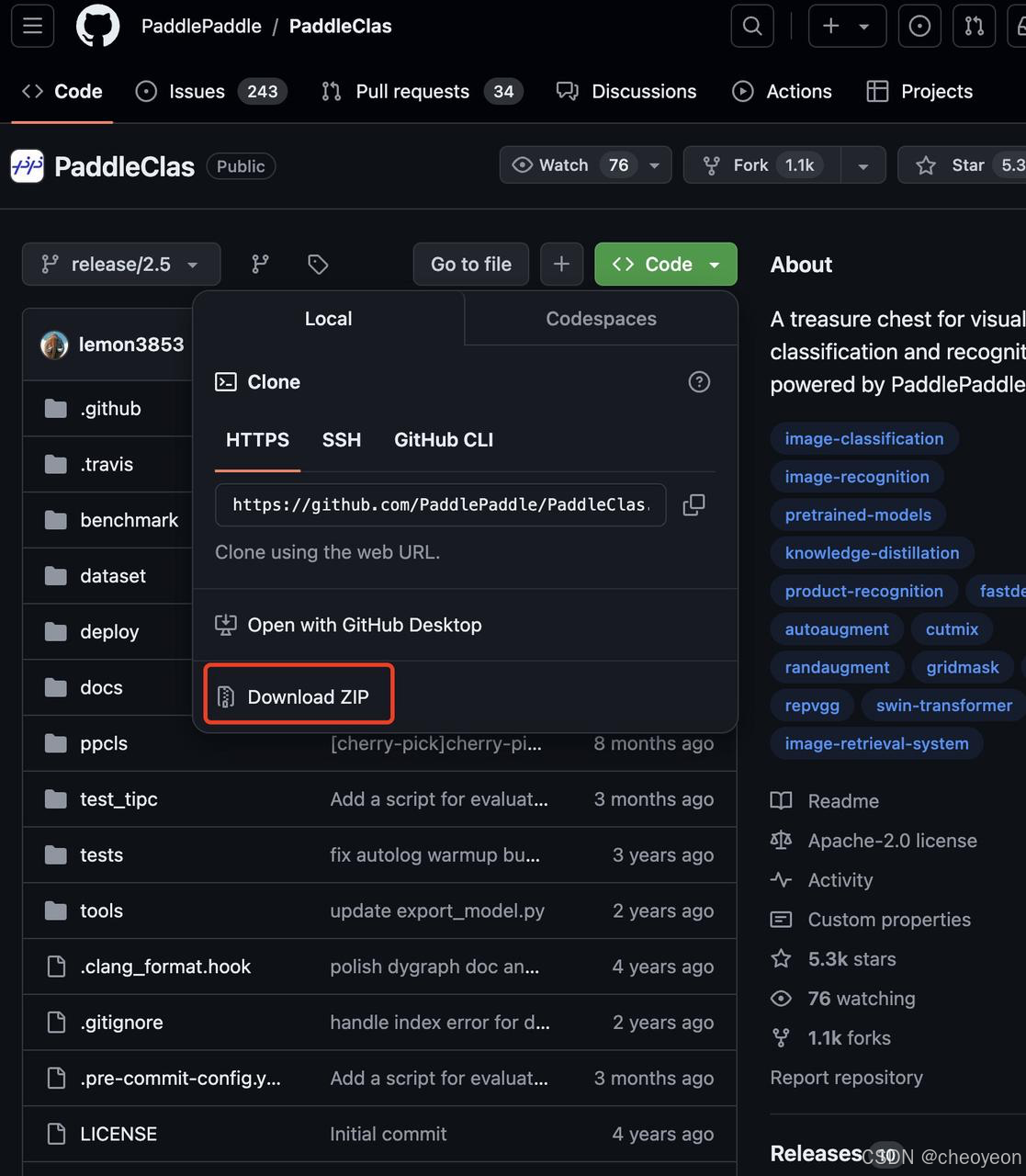
解压后得到PaddleClas文件夹。
2.4 创建文件目录
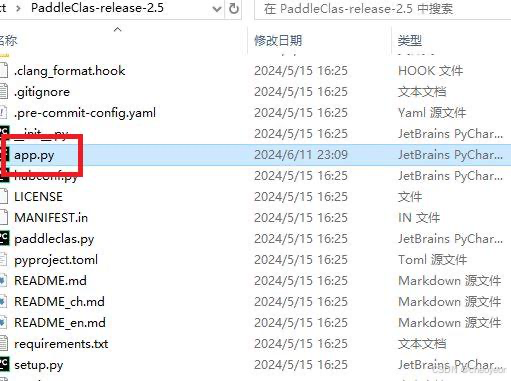
它的作用是:运行运行Gradio Demo的脚本
3. ResNet模型训练
3.1 修改配置
首先在PaddleClas文件夹中找到ppcls-->configs-->ImageNet-->Res2Net-->Res2Net50_14w_8s.yaml。
分别修改epochs为100,类别class_num为9,训练图片路径,验证图片路径和标签文件。一共修改7处。
# global configs
Global:
checkpoints: null
pretrained_model: null
output_dir: ./output/
device: gpu
save_interval: 1
eval_during_train: True
eval_interval: 1
epochs: 100###########################1##############################
print_batch_step: 10
use_visualdl: False
# used for static mode and model export
image_shape: [3, 224, 224]
save_inference_dir: ./inference
# model architecture
Arch:
name: Res2Net50_14w_8s
class_num: 9############################2##############################
# loss function config for traing/eval process
Loss:
Train:
- CELoss:
weight: 1.0
epsilon: 0.1
Eval:
- CELoss:
weight: 1.0
Optimizer:
name: Momentum
momentum: 0.9
lr:
name: Cosine
learning_rate: 0.1
regularizer:
name: 'L2'
coeff: 0.0001
# data loader for train and eval
DataLoader:
Train:
dataset:
name: ImageNetDataset
image_root: ./weeds/images/#################3#######################
cls_label_path: ./weeds/train.txt###########4########################
transform_ops:
- DecodeImage:
to_rgb: True
channel_first: False
- RandCropImage:
size: 224
- RandFlipImage:
flip_code: 1
- NormalizeImage:
scale: 1.0/255.0
mean: [0.485, 0.456, 0.406]
std: [0.229, 0.224, 0.225]
order: ''
batch_transform_ops:
- MixupOperator:
alpha: 0.2
sampler:
name: DistributedBatchSampler
batch_size: 64
drop_last: False
shuffle: True
loader:
num_workers: 4
use_shared_memory: True
Eval:
dataset:
name: ImageNetDataset
image_root: ./DeepWeeds/images/###############5#######################
cls_label_path: ./DeepWeeds/val.txt###########6########################
transform_ops:
- DecodeImage:
to_rgb: True
channel_first: False
- ResizeImage:
resize_short: 256
- CropImage:
size: 224
- NormalizeImage:
scale: 1.0/255.0
mean: [0.485, 0.456, 0.406]
std: [0.229, 0.224, 0.225]
order: ''
sampler:
name: DistributedBatchSampler
batch_size: 64
drop_last: False
shuffle: False
loader:
num_workers: 4
use_shared_memory: True
Infer:
infer_imgs: docs/images/inference_deployment/whl_demo.jpg
batch_size: 10
transforms:
- DecodeImage:
to_rgb: True
channel_first: False
- ResizeImage:
resize_short: 256
- CropImage:
size: 224
- NormalizeImage:
scale: 1.0/255.0
mean: [0.485, 0.456, 0.406]
std: [0.229, 0.224, 0.225]
order: ''
- ToCHWImage:
PostProcess:
name: Topk
topk: 5
class_id_map_file: ./DeepWeeds/classnaems.txt###########7##################
Metric:
Train:
Eval:
- TopkAcc:
topk: [1, 5]
3.2 使用Swanlab
在PaddleClas文件夹中找tools-->train.py。初始化swanlab
# Copyright (c) 2021 PaddlePaddle Authors. All Rights Reserved.
#
# Licensed under the Apache License, Version 2.0 (the "License");
# you may not use this file except in compliance with the License.
# You may obtain a copy of the License at
#
# http://www.apache.org/licenses/LICENSE-2.0
#
# Unless required by applicable law or agreed to in writing, software
# distributed under the License is distributed on an "AS IS" BASIS,
# WITHOUT WARRANTIES OR CONDITIONS OF ANY KIND, either express or implied.
# See the License for the specific language governing permissions and
# limitations under the License.
from __future__ import absolute_import
from __future__ import division
from __future__ import print_function
import os
import sys
__dir__ = os.path.dirname(os.path.abspath(__file__))
sys.path.append(os.path.abspath(os.path.join(__dir__, '../')))
from ppcls.utils import config
from ppcls.engine.engine import Engine
import swanlab
# -*- coding: utf-8 -*-
if __name__ == "__main__":
args = config.parse_args()
config = config.get_config(
args.config, overrides=args.override, show=False)
config.profiler_options = args.profiler_options
engine = Engine(config, mode="train")
## 初始化swanlab
swanlab.init(
experiment_name="Swanlab_ResNet50_PaddleClas",
description="Train ResNet50 for weeds classification.",
project="Swanhub_Weeds_Classification",
config={
"model": "ResNet50",
"optim": "Adam",
"lr": 0.001,
"batch_size": 64,
"num_epochs": 100,
"num_class": 9,
}
)
engine.train()
在PaddleClas中找ppcls-->engine-->train-->utils.py中添加以下代码:
swanlab.log({"train_lr_msg": lr_msg.split(": ")[1]}) #
swanlab.log({"train_CELoss": metric_msg.split(",")[0].split(': ')[1]}) ##
swanlab.log({'train_loss': metric_msg.split(",")[1].split(': ')[1]})
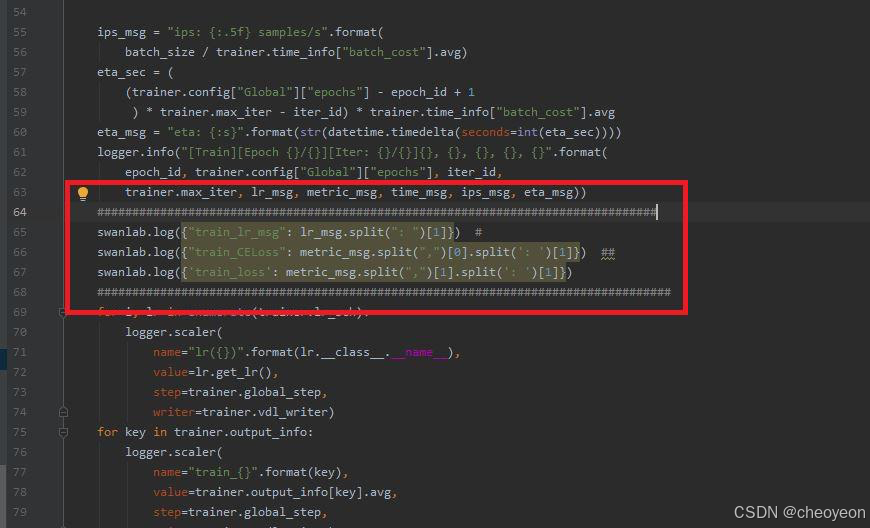
在PaddleClas文件夹中找ppcls-->engine-->engine.py,添加以下代码:
swanlab.log({'best_metric': best_metric.get('metric')})
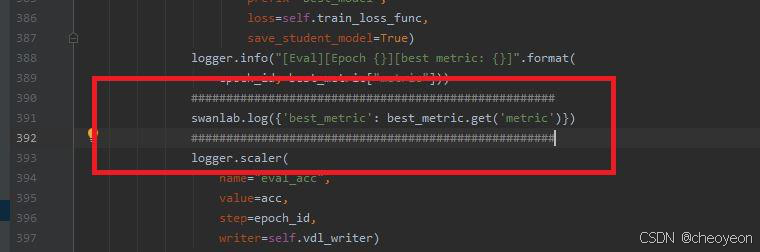
3.3 模型训练
在控制台输入以下命令:
python -m paddle.distributed.launch tools/train.py -c ./ppcls/configs/ImageNet/Res2Net/Res2Net50_14w_8s.yaml
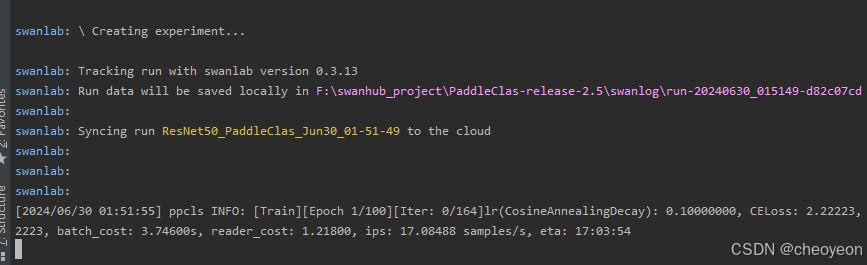
在swanlab查看实验详细信息
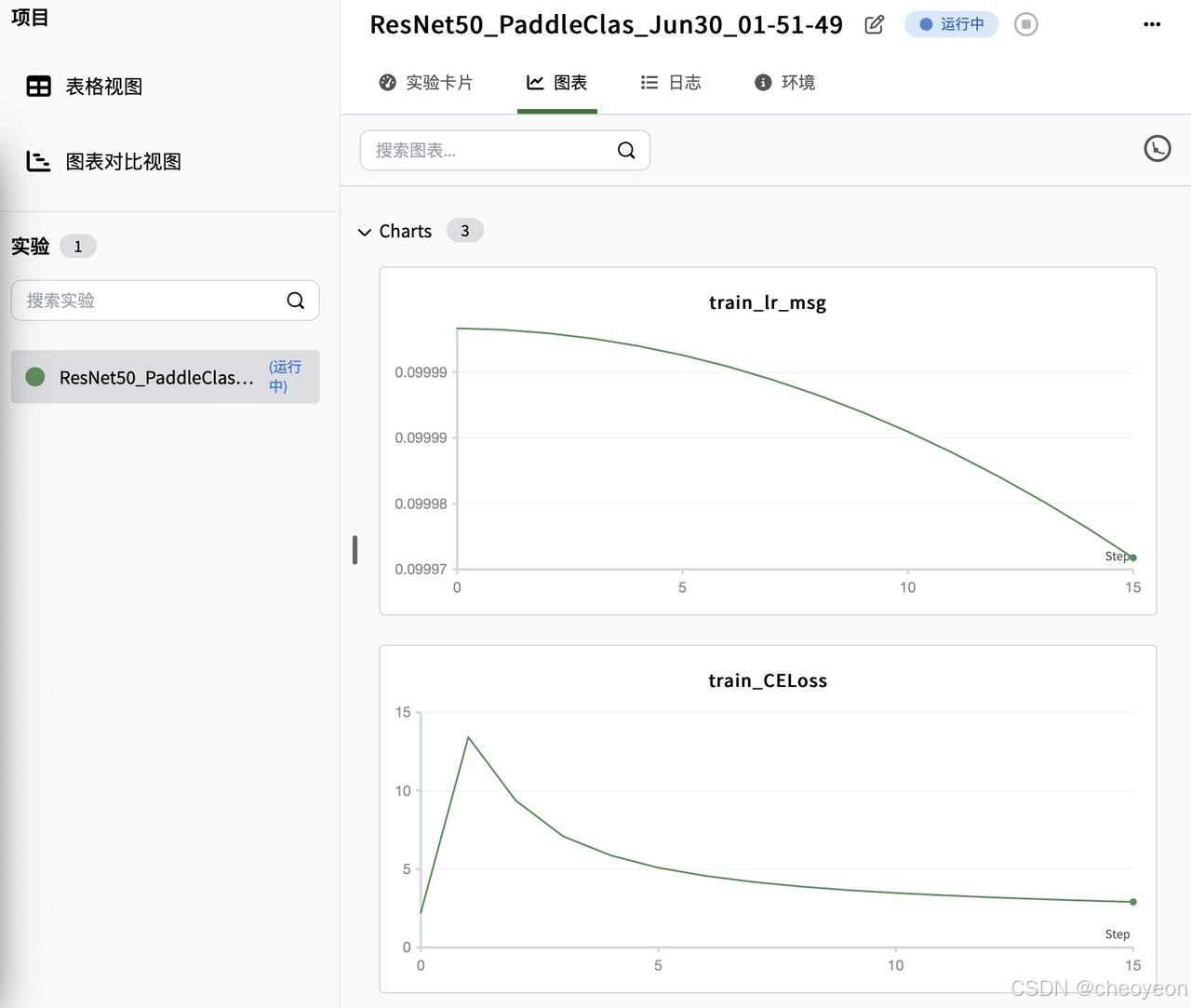
实验结果如下:
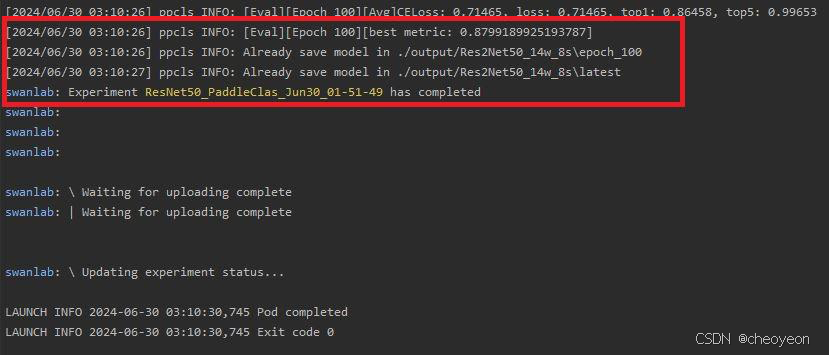
swanlab查看实验结果
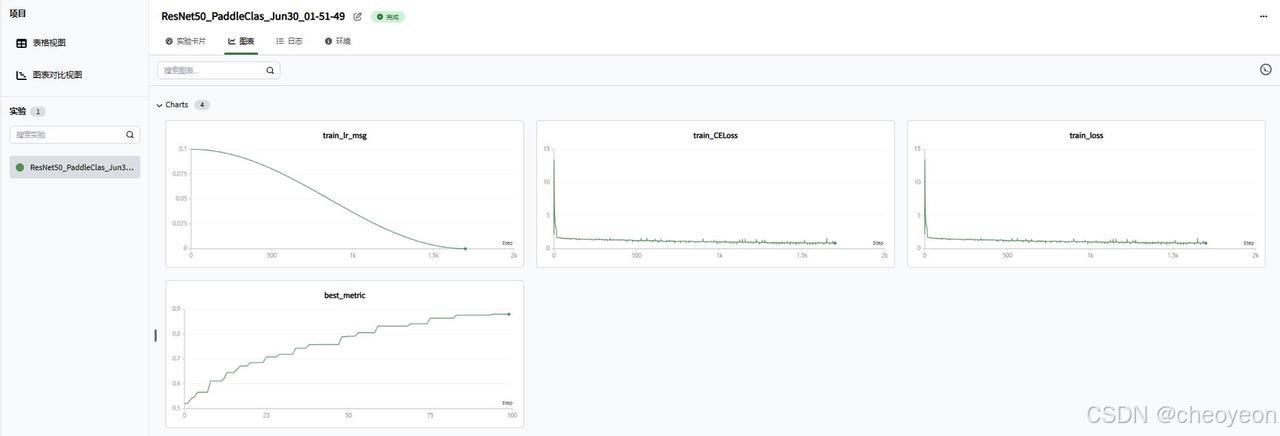
3.4 模型推理
将以下代码输入控制台:
python tools/infer.py -c ./ppcls/configs/ImageNet/Res2Net/Res2Net50_14w_8s.yaml -o Infer.infer_imgs=./DeepWeeds/infer/01.jpg -o Global.pretrained_model=./output/Res2Net50_14w_8s/best_model
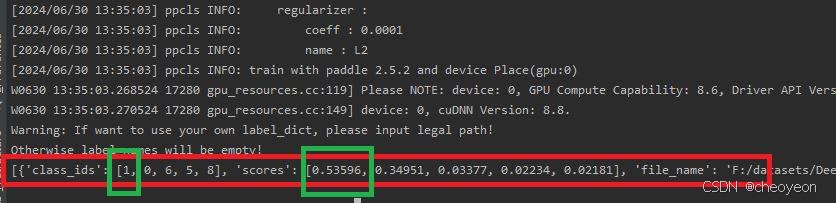
4. DarkNet53模型训练
4.1 修改配置
首先在PaddleClas文件夹中找到ppcls-->configs-->ImageNet-->DarkNet-->DarkNet53.yaml。
分别修改epochs为100,类别class_num为9,训练图片路径,验证图片路径和标签文件。一共修改7处。
# global configs
Global:
checkpoints: null
pretrained_model: null
output_dir: ./output/
device: gpu
save_interval: 1
eval_during_train: True
eval_interval: 1
epochs: 100
print_batch_step: 10
use_visualdl: False
# used for static mode and model export
image_shape: [3, 256, 256]
save_inference_dir: ./inference
# model architecture
Arch:
name: DarkNet53
class_num: 9
# loss function config for traing/eval process
Loss:
Train:
- CELoss:
weight: 1.0
epsilon: 0.1
Eval:
- CELoss:
weight: 1.0
Optimizer:
name: Momentum
momentum: 0.9
lr:
name: Cosine
learning_rate: 0.1
regularizer:
name: 'L2'
coeff: 0.0001
# data loader for train and eval
DataLoader:
Train:
dataset:
name: ImageNetDataset
image_root: F:/datasets/DeepWeeds/images
cls_label_path: F:/datasets/DeepWeeds/train.txt
transform_ops:
- DecodeImage:
to_rgb: True
channel_first: False
- RandCropImage:
size: 256
- RandFlipImage:
flip_code: 1
- NormalizeImage:
scale: 1.0/255.0
mean: [0.485, 0.456, 0.406]
std: [0.229, 0.224, 0.225]
order: ''
batch_transform_ops:
- MixupOperator:
alpha: 0.2
sampler:
name: DistributedBatchSampler
batch_size: 64
drop_last: False
shuffle: True
loader:
num_workers: 4
use_shared_memory: True
Eval:
dataset:
name: ImageNetDataset
image_root: F:/datasets/DeepWeeds/images
cls_label_path: F:/datasets/DeepWeeds/val.txt
transform_ops:
- DecodeImage:
to_rgb: True
channel_first: False
- ResizeImage:
resize_short: 292
- CropImage:
size: 256
- NormalizeImage:
scale: 1.0/255.0
mean: [0.485, 0.456, 0.406]
std: [0.229, 0.224, 0.225]
order: ''
sampler:
name: DistributedBatchSampler
batch_size: 64
drop_last: False
shuffle: False
loader:
num_workers: 4
use_shared_memory: True
Infer:
infer_imgs: docs/images/inference_deployment/whl_demo.jpg
batch_size: 10
transforms:
- DecodeImage:
to_rgb: True
channel_first: False
- ResizeImage:
resize_short: 292
- CropImage:
size: 256
- NormalizeImage:
scale: 1.0/255.0
mean: [0.485, 0.456, 0.406]
std: [0.229, 0.224, 0.225]
order: ''
- ToCHWImage:
PostProcess:
name: Topk
topk: 5
class_id_map_file: F:/datasets/DeepWeeds/classnames
Metric:
Train:
Eval:
- TopkAcc:
topk: [1, 5]
4.2 使用Swanlab
在PaddleClas文件夹中找tools-->train.py。修改初始化swanlab
# Copyright (c) 2021 PaddlePaddle Authors. All Rights Reserved.
#
# Licensed under the Apache License, Version 2.0 (the "License");
# you may not use this file except in compliance with the License.
# You may obtain a copy of the License at
#
# http://www.apache.org/licenses/LICENSE-2.0
#
# Unless required by applicable law or agreed to in writing, software
# distributed under the License is distributed on an "AS IS" BASIS,
# WITHOUT WARRANTIES OR CONDITIONS OF ANY KIND, either express or implied.
# See the License for the specific language governing permissions and
# limitations under the License.
from __future__ import absolute_import
from __future__ import division
from __future__ import print_function
import os
import sys
__dir__ = os.path.dirname(os.path.abspath(__file__))
sys.path.append(os.path.abspath(os.path.join(__dir__, '../')))
from ppcls.utils import config
from ppcls.engine.engine import Engine
import swanlab
# -*- coding: utf-8 -*-
if __name__ == "__main__":
args = config.parse_args()
config = config.get_config(
args.config, overrides=args.override, show=False)
config.profiler_options = args.profiler_options
engine = Engine(config, mode="train")
## 初始化swanlab
swanlab.init(
experiment_name="Swanlab_DrakNet53_PaddleClas",
description="Train DarkNet53 for weeds classification.",
project="Swanhub_Weeds_Classification",
config={
"model": "DarkNet53",
"optim": "Adam",
"lr": 0.001,
"batch_size": 64,
"num_epochs": 100,
"num_class": 9,
}
)
engine.train()
4.3 模型训练
在控制台输入以下命令:
python -m paddle.distributed.launch tools/train.py -c ./ppcls/configs/ImageNet/DarkNet/DarknetNet53.yaml
在swanlab查看实验详细信息

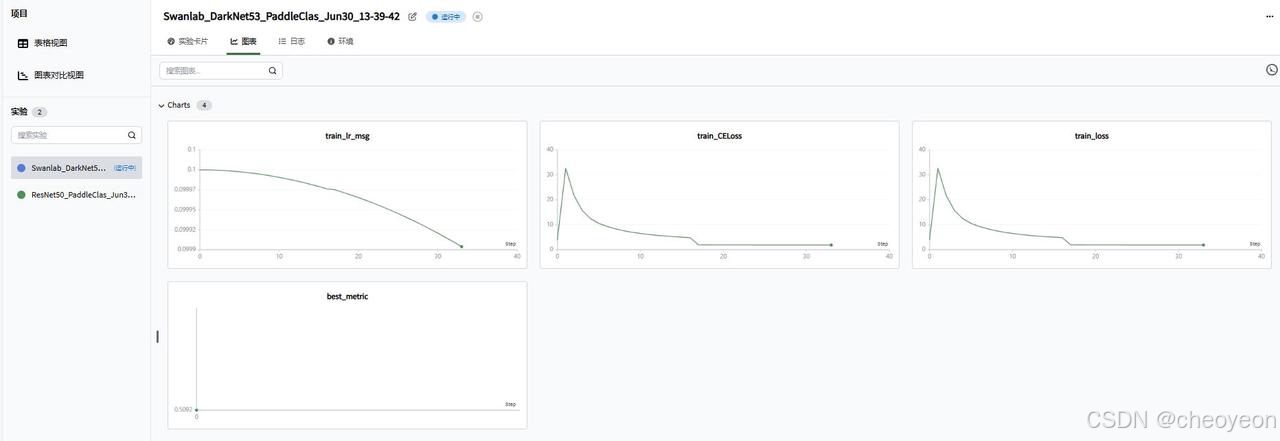
实验结果如下:
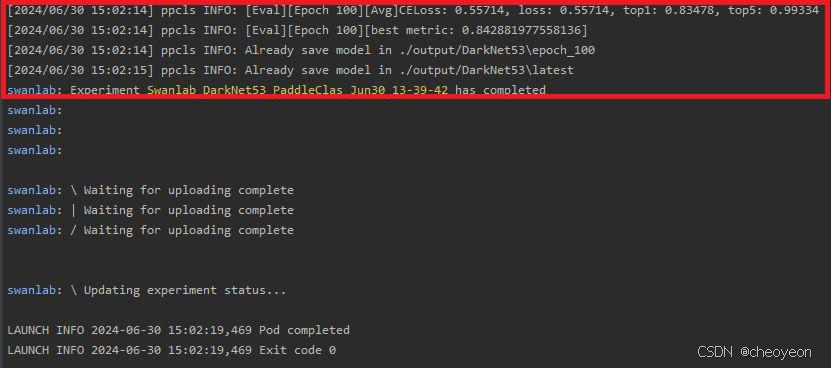
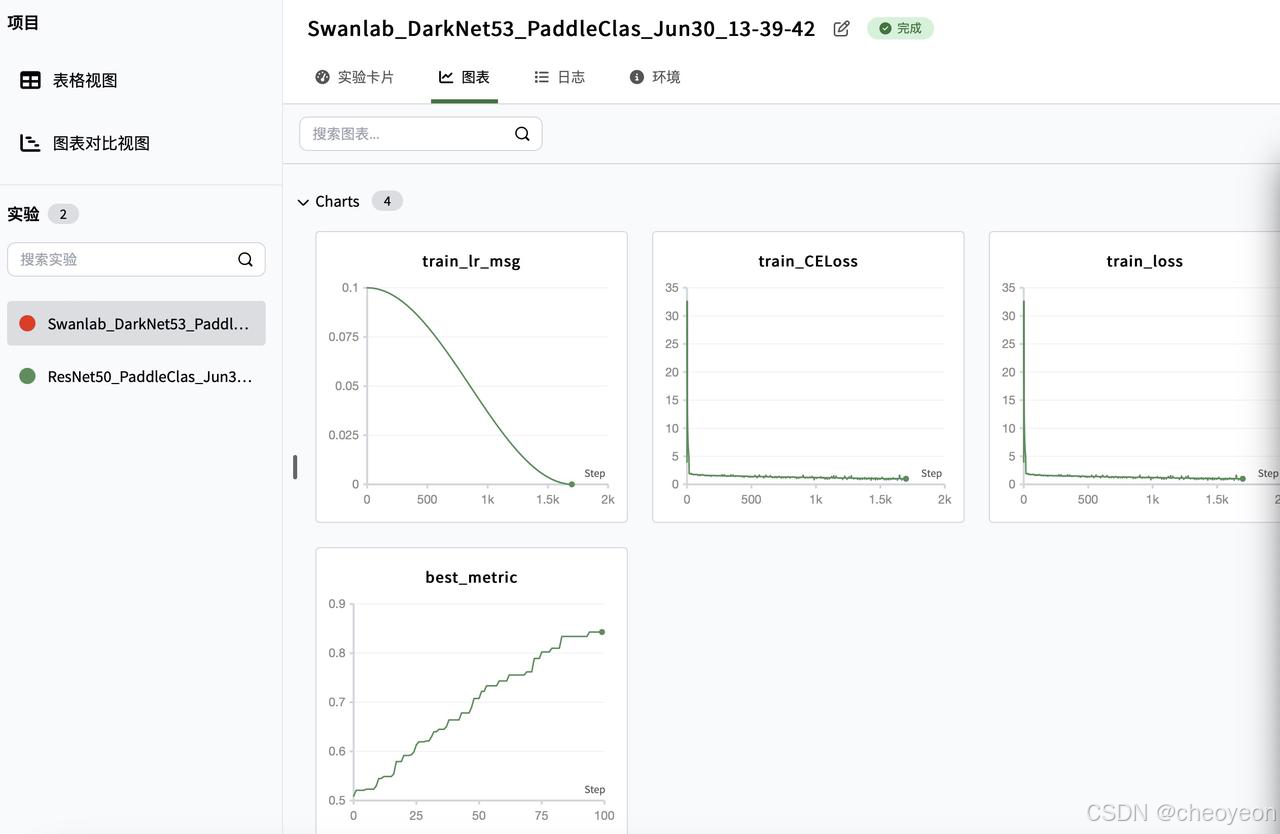
4.4 模型推理
将以下代码输入控制台:
python tools/infer.py -c ./ppcls/configs/ImageNet/DarkNet/DarkNet53.yaml -o Infer.infer_imgs=./DeepWeeds/infer/01.jpg -o Global.pretrained_model=./output/DarkNet53/best_model
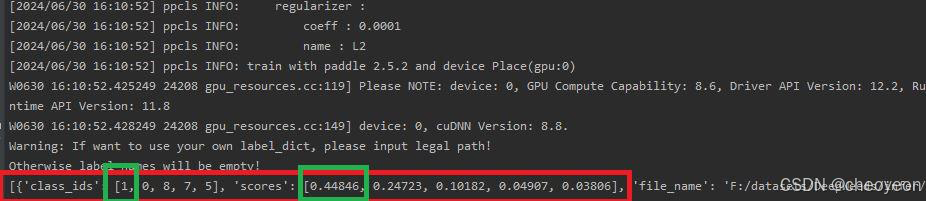
4.5 Swanlab结果展示
从图中可以看出,ResNet50模型的效果要比DarkNet53模型好,swanlab提供了便利的对比图表功能。
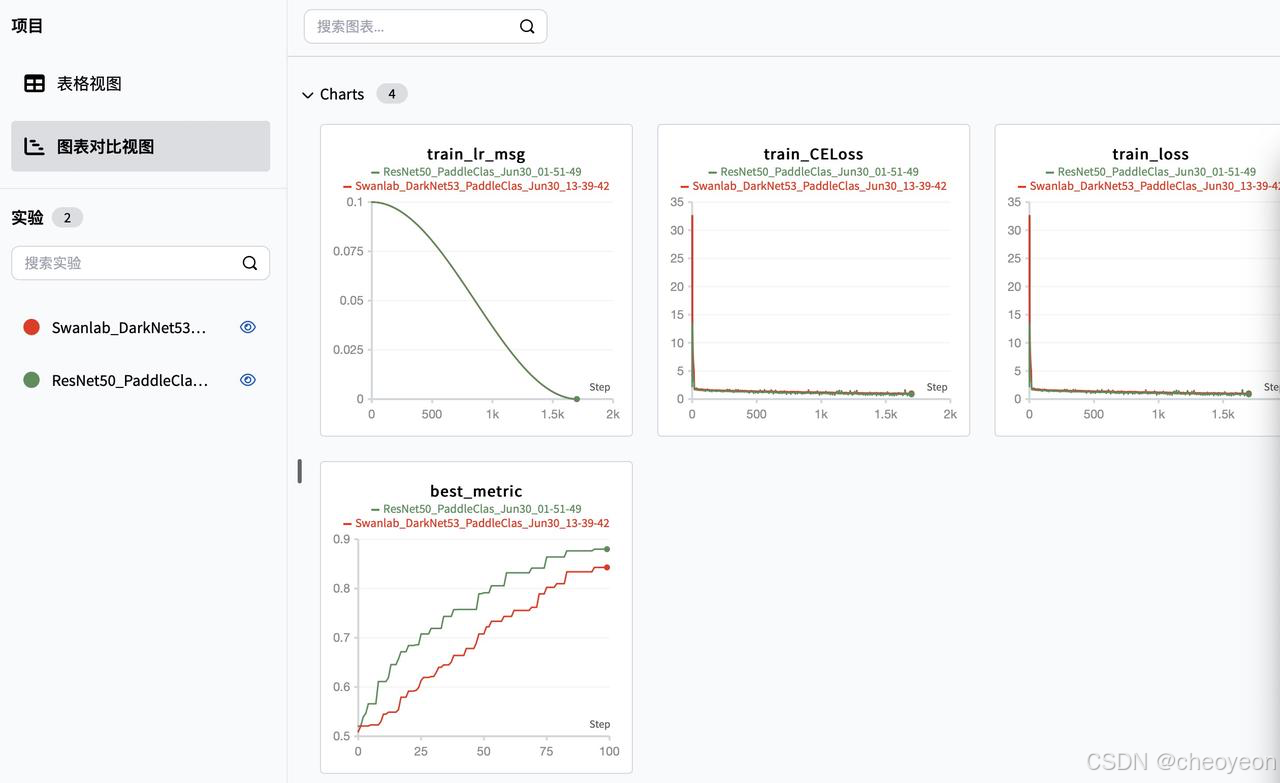
5. Gradio演示
未完待续。。。
6. Swanhub上传并演示demo
未完待续。。。