在信息检索方法的发展历程中,我们见证了从传统的统计关键词匹配到如 BERT 这样的深度学习模型的转变。虽然传统方法提供了坚实的基础,但往往难以精准捕捉文本的语义关系。如 BERT 这样的稠密检索方法通过利用高维向量捕获文本的上下文语义,为搜索技术带来了显著进步。然而,由于这些方法依赖于特定领域的知识,它们在处理领域外(out-of-domain)问题时可能会遇到困难。
Learned 稀疏 Embedding 提供了一套独特的解决方案,结合了稀疏表示的可解释性(interpretability)和深度学习模型的语境理解能力。在本篇博客中,我们将探讨 Learned 稀疏向量的底层机制、优势以及它们在实际应用中的潜力。特别是与 Milvus 向量数据库结合时,稀疏向量能够改进信息检索系统,通过提高检索效率,提供富含上下文的答案,最终优化系统性能。
信息检索方式演变:从关键词匹配到上下文理解
早期信息检索系统主要依靠基于统计的关键词匹配方法,如 TF-IDF 和 BM25 等词袋(Bag of Words)算法。这些方法通过分析术语的出现频率和在整个文档库中的分布来评估文档的相关性。这些简单而又丰富的传统方法成为了互联网快速增长时期的流行工具。
2013 年,Google 推出了 Word2Vec,这是首次尝试使用高维向量来表示单词并捕捉它们细微的语义差异。这一方法标志着信息检索方法逐渐转向由机器学习驱动。
随着 BERT 的出现------一种基于 Transformer 的革命性预训练语言模型,彻底改变了信息检索的方式。BERT 利用强大的 Transformer 中的注意力机制,捕捉文档的复杂语境语义,并将其压缩成稠密向量,为查询提供强大且语义准确的检索,从根本上优化了信息检索过程。
BERT 推出三个月后,Nogueira 和 Cho 将其应用于 MS MARCO 段落排名任务。与之前最佳的 IRNet 方法相比,BERT 的效果提升了 30%,是 BM25 baseline 的 2 倍。到了 2024 年 3 月,文本排名分数更是高达 0.450 (eval)和 0.463(dev)。
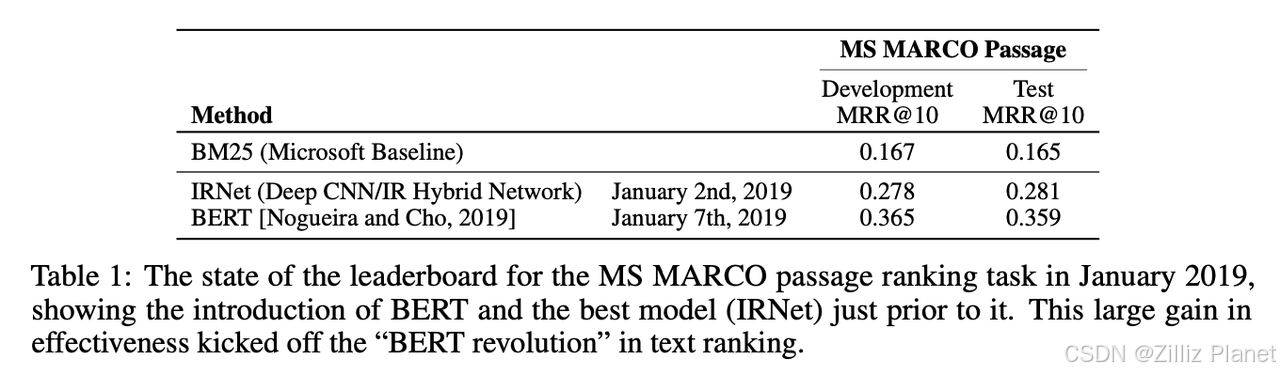
这些突破凸显了信息检索方法的不断演变。我们自此步入了自然语言处理(NLP)的纪元。
领域外信息检索挑战
稠密向量技术,如 BERT,与传统的词袋模型相比有着其独有的优势------能够精确把握文本中的复杂语境。这一特性极大地提升了信息检索系统在处理熟悉领域查询时的性能。
然而,当 BERT 进入非熟悉的领域进行 Out-of-Domain(OOD)检索时,会面临挑战。其训练过程本质上偏向于训练数据的特点,这使得它在为 OOD 数据集中未见过的文本片段生成有意义的 embeddings 时表现不佳,特别是在含有丰富特定领域术语的数据集中,这一限制尤为突出。
解决这一问题的一种可能方法是微调。然而,这种解决方案有时行不通或者成本较高。微调需要访问一个中到大型的标注数据集,包括与目标领域相关的查询、正面和负面文档。此外,创建这样的数据集需要领域专家的专业知识,以确保数据质量,这一过程十分耗时且成本高昂。
另一方面,尽管传统的词袋算法在处理 OOD 信息检索时面临词汇不匹配问题(查询与相关文档之间通常缺乏术语重叠),但它们在性能上仍然优于 BERT。这是因为在词袋算法中,未被识别的术语不是被"学习",而是被"精确匹配"的。
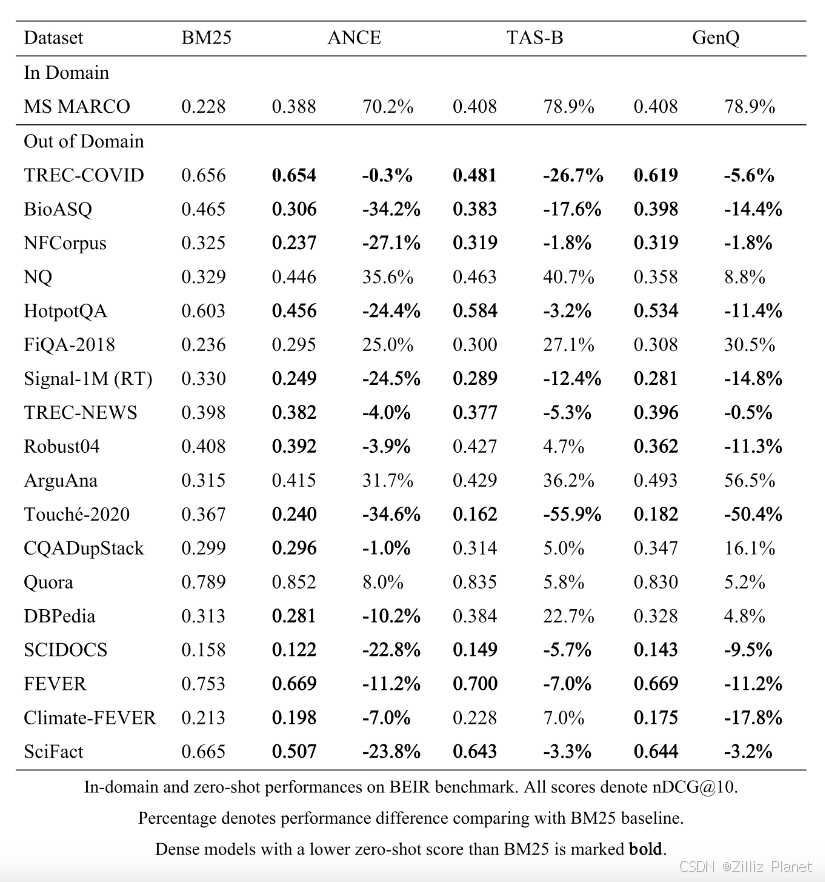
学习得到的稀疏向量:将传统稀疏向量表示与上下文信息相结合
结合 Out-of-Domain 检索的精确词匹配技术,如词袋模型和 BERT 等稠密向量检索方法进行语义检索,长期以来一直是信息检索领域的一项主要任务。幸运的是,出现了新的解决方法:学习得到的稀疏 embedding。
那么,到底什么是学习得到的稀疏 embedding 向量呢?
学习得到的稀疏 embedding 指的是通过复杂的 ML 模型(如 SPLADE 和 BGE-M3 等)生成的稀疏向量表示。与仅依赖于统计方法(如 BM25)生成的传统稀疏向量不同,学习得到的稀疏 embedding 在保留关键词搜索能力的同时,丰富了稀疏表示的上下文信息。它们能够辨识相邻或相关词语的重要性,即使这些词语在文本中没有明确出现。最终生成一种擅长捕捉相关关键词和类别的"学习得到的"稀疏表示。
以 SPLADE 为例。在编码给定文本时,SPLADE 生成的稀疏 embedding 形式为 token-to-weight 映射,例如:
{"hello": 0.33, "world": 0.72}
乍看之下,这些 embedding 与由统计方法生成的传统稀疏 embedding 类似。然而,它们的组成有一个关键区别:维度(词汇)和权重。带有上下文化信息的机器学习模型决定了学习型稀疏 embedding 的维度(词汇)和权重。这种稀疏表示与学习得到的 上下文的结合为信息检索任务提供了一种强大的工具,无缝弥合了精确词匹配和语义理解之间的鸿沟。
与稠密向量不同,学习得到的稀疏 embedding 采取更为简洁的方式,只保留文本信息中的关键内容。这种简化有助于防止模型过拟合(over-fitting)训练数据,提高模型在遇到新的数据模式时的泛化能力,尤其是在 Out-Of-Domain 信息检索场景中。
通过优先处理关键文本元素,同时舍弃不必要的细节,学习得到的稀疏 embedding 完美平衡了捕获相关信息与避免过拟合两个方面,从而增强了它们在各种检索任务中的应用价值。
学习得到的稀疏 Embedding与稠密检索方法相结合,在领域内检索任务中展现了强大的性能。来自 BGE-M3 的研究显示,学习得到的稀疏向量在多语言或跨语言检索任务中优于稠密向量,在准确性方面表现更佳。结合使用这两种 embeddings 时,检索准确性得到了进一步提升,展现了两种 Embedding 相辅相成的效果。
此外,这些 embedding 固有的稀疏性大大简化了向量相似性搜索,减少了需要消耗的计算资源。此外,学习得到的稀疏向量通过匹配增强上下文理解,可以快速检查匹配的文档,以确定相应的匹配词。此功能为深入了解检索过程提供了更精确的见解,提高了系统的透明度和可用性
代码示例
现在让我们来看看在密集检索效果不佳的情况下,学习得到的稀疏向量时如何表现的。
数据集 :MIRACL。
查询 :What years did Zhu Xi live?
备注:
-
朱熹是宋朝时期的中国书法家、历史学家、哲学家、诗人和政治家。
-
MIRACL 数据集是多语言的,本展示中我们仅使用英文部分的"训练"切分。它包含 26746 篇文章,其中七篇与朱熹相关。 我们分别使用密集和稀疏检索方法检索了这七个与查询相关的故事。最初,我们分别将所有故事编码为密集或稀疏 embeddings,并将它们存储在 Milvus 向量数据库中。随后,我们对查询进行编码,并使用 KNN 搜索识别与查询 embedding 最接近的前 10 个故事。
Sparse Search Results(IP distance, larger is closer): Score: 26.221 Id: 244468#1 Text: Zhu Xi, whose family originated in Wuyuan County, Huizhou ... Score: 26.041 Id: 244468#3 Text: In 1208, eight years after his death, Emperor Ningzong of Song rehabilitated Zhu Xi and honored him ... Score: 25.772 Id: 244468#2 Text: In 1179, after not serving in an official capacity since 1156, Zhu Xi was appointed Prefect of Nankang Military District (南康軍) ... Score: 23.905 Id: 5823#39 Text: During the Song dynasty, the scholar Zhu Xi (AD 1130--1200) added ideas from Daoism and Buddhism into Confucianism ... Score: 23.639 Id: 337115#2 Text: ... During the Song Dynasty, the scholar Zhu Xi (AD 1130--1200) added ideas from Taoism and Buddhism into Confucianism ... Score: 23.061 Id: 10443808#22 Text: Zhu Xi was one of many critics who argued that ... Score: 22.577 Id: 55800148#11 Text: In February 1930, Zhu decided to live the life of a devoted revolutionary after leaving his family at home ... Score: 20.779 Id: 12662060#3 Text: Holding to Confucius and Mencius' conception of humanity as innately good, Zhu Xi articulated an understanding of "li" as the basic pattern of the universe ... Score: 20.061 Id: 244468#28 Text: Tao Chung Yi (around 1329~1412) of the Ming dynasty: Whilst Master Zhu inherited the orthodox teaching and propagated it to the realm of sages ... Score: 19.991 Id: 13658250#10 Text: Zhu Shugui was 66 years old (by Chinese reckoning; 65 by Western standards) at the time of his suicide ... Dense Search Results(L2 distance, smaller is closer): Score: 0.600 Id: 244468#1 Text: Zhu Xi, whose family originated in Wuyuan County, Huizhou ... Score: 0.706 Id: 244468#3 Text: In 1208, eight years after his death, Emperor Ningzong of Song rehabilitated Zhu Xi and honored him ... Score: 0.759 Id: 13658250#10 Text: Zhu Shugui was 66 years old (by Chinese reckoning; 65 by Western standards) at the time of his suicide ... Score: 0.804 Id: 6667852#0 Text: King Zhaoxiang of Qin (; 325--251 BC), or King Zhao of Qin (秦昭王), born Ying Ji ... Score: 0.818 Id: 259901#4 Text: According to the 3rd-century historical text "Records of the Three Kingdoms", Liu Bei was born in Zhuo County ... Score: 0.868 Id: 343207#0 Text: Ruzi Ying (; 5 -- 25), also known as Emperor Ruzi of Han and the personal name of Liu Ying (劉嬰), was the last emperor ... Score: 0.876 Id: 31071887#1 Text: The founder of the Ming dynasty was the Hongwu Emperor (21 October 1328 -- 24 June 1398), who is also known variably by his personal name "Zhu Yuanzhang" ... Score: 0.882 Id: 2945225#0 Text: Emperor Ai of Tang (27 October 89226 March 908), also known as Emperor Zhaoxuan (), born Li Zuo, later known as Li Chu ... Score: 0.890 Id: 33230345#0 Text: Li Xi or Li Qi (李谿 per the "Zizhi Tongjian" and the "History of the Five Dynasties" or 李磎 per the "Old Book of Tang" ... Score: 0.893 Id: 172890#1 Text: The Wusun originally lived between the Qilian Mountains and Dunhuang (Gansu) near the Yuezhi ...
仔细查看结果:在稀疏检索中,7 个与朱熹相关的故事都排在前 10 名。而在稠密检索中,只有 2 个故事位于前 10。虽然稀疏和稠密检索方法均正确识别了编号为
244468#1
和244468#3
的段落,但稠密检索未能捕捉到其他相关故事。相反,稠密检索返回的其他 8 个故事与中国的其他历史故事相关,这些内容虽然模型认为与朱熹有关,但实际上无直接关联。如果您对背后的原理感兴趣,请继续阅读,我们将详细介绍如何使用 Milvus 进行向量搜索。
如何使用 Milvus 进行向量搜索
Milvus 是一款高度可扩展、性能出色的开源向量数据库。在最新的 2.4 版本中,Milvus 支持了稀疏和稠密向量(公测中)。本文将利用 Milvus 2.4 来存储数据集并执行向量搜索。
接下来,我们将演示如何利用 Milvus 在 MIRACL 数据集上执行查询"朱熹生活在哪个年代?"
。
我们使用 SPLADE 和 MiniLM-L6-v2 模型,将查询内容及 MIRACL 源数据集转化为稀疏和稠密向量。
首先,我们需要创建一个目录,并配置环境与 Milvus 服务,请确保您的系统中已安装 python(>=3.8)
、virtualenv
、docker
以及 docker-compose
。
mkdir milvus_sparse_demo && cd milvus_sparse_demo
# spin up a milvus local cluster
wget https://github.com/milvus-io/milvus/releases/download/v2.4.0-rc.1/milvus-standalone-docker-compose.yml -O docker-compose.yml
docker-compose up -d
# create a virtual environment
virtualenv -p python3.8 .venv && source .venv/bin/activate
touch milvus_sparse_demo.py
从 2.4 版本开始,pymilvus(Milvus 的 Python SDK)引入了一个可选的 model
模型模块。这个模块简化了使用模型将文本编码成稀疏或稠密向量的流程。此外,我们使用 pip
来安装 pymilvus model ,以便访问 HuggingFace 上的数据集。
pip install "pymilvus[model]" datasets tqdm
首先,使用 HuggingFace 的 Datasets
库下载数据集,收集所有的段落。
from datasets import load_dataset
miracl = load_dataset('miracl/miracl', 'en')['train']
# collect all passages in the dataset
docs = list({doc['docid']: doc for entry in miracl for doc in entry['positive_passages'] + entry['negative_passages']}.values())
print(docs[0])
# {'docid': '2078963#10', 'text': 'The third thread in the development of quantum field theory...'}
将查询编码。
from pymilvus import model
sparse_ef = model.sparse.SpladeEmbeddingFunction(
model_name="naver/splade-cocondenser-selfdistil",
device="cpu",
)
dense_ef = model.dense.SentenceTransformerEmbeddingFunction(
model_name='all-MiniLM-L6-v2',
device='cpu',
)
query = "What years did Zhu Xi live?"
query_sparse_emb = sparse_ef([query])
query_dense_emb = dense_ef([query])
查看生成的稀疏向量并找到权重最高的 Token。
sparse_token_weights = sorted([(sparse_ef.model.tokenizer.decode(col), query_sparse_emb[0, col])
for col in query_sparse_emb.indices[query_sparse_emb.indptr[0]:query_sparse_emb.indptr[1]]], key=lambda item: item[1], reverse=True)
print(sparse_token_weights[:7])
[('zhu', 3.0623178), ('xi', 2.4944391), ('zhang', 1.4790473), ('date', 1.4589322), ('years', 1.4154341), ('live', 1.3365831), ('china', 1.2351396)]
将所有文档进行编码。
from tqdm import tqdm
doc_sparse_embs = [sparse_ef([doc['text']]) for doc in tqdm(docs, desc='Encoding Sparse')]
doc_dense_embs = [dense_ef([doc['text']])[0] for doc in tqdm(docs, desc='Encoding Dense')]
接着,在 Milvus 中创建 Collection 以存储文档 id
、文本、稠密和稀疏向量等。然后插入数据。
from pymilvus import (
MilvusClient, FieldSchema, CollectionSchema, DataType
)
milvus_client = MilvusClient("http://localhost:19530")
collection_name = 'miracl_demo'
fields = [
FieldSchema(name="doc_id", dtype=DataType.VARCHAR, is_primary=True, auto_id=False, max_length=100),
FieldSchema(name="text", dtype=DataType.VARCHAR, max_length=65530),
FieldSchema(name="sparse_vector", dtype=DataType.SPARSE_FLOAT_VECTOR),
FieldSchema(name="dense_vector", dtype=DataType.FLOAT_VECTOR, dim=384),
]
milvus_client.create_collection(collection_name, schema=CollectionSchema(fields, "miracl demo"), timeout=5, consistency_level="Strong")
batch_size = 30
n_docs = len(docs)
for i in tqdm(range(0, n_docs, batch_size), desc='Inserting documents'):
milvus_client.insert(collection_name, [
{
"doc_id": docs[idx]['docid'],
"text": docs[idx]['text'],
"sparse_vector": doc_sparse_embs[idx],
"dense_vector": doc_dense_embs[idx]
}
for idx in range(i, min(i+batch_size, n_docs))
])
为加速搜索,对向量字段创建索引。
index_params = milvus_client.prepare_index_params()
index_params.add_index(
field_name="sparse_vector",
index_type="SPARSE_INVERTED_INDEX",
metric_type="IP",
)
index_params.add_index(
field_name="dense_vector",
index_type="FLAT",
metric_type="L2",
)
milvus_client.create_index(collection_name, index_params=index_params)
milvus_client.load_collection(collection_name)
进行搜索并查看结果。
k = 10
sparse_results = milvus_client.search(collection_name, query_sparse_emb, anns_field="sparse_vector", limit=k, search_params={"metric_type": "IP"}, output_fields=['doc_id', 'text'])[0]
dense_results = milvus_client.search(collection_name, query_dense_emb, anns_field="dense_vector", limit=k, search_params={"metric_type": "L2"}, output_fields=['doc_id', 'text'])[0]
print(f'Sparse Search Results:')
for result in sparse_results:
print(f"\tScore: {result['distance']} Id: {result['entity']['doc_id']} Text: {result['entity']['text']}")
print(f'Dense Search Results:')
for result in dense_results:
print(f"\tScore: {result['distance']} Id: {result['entity']['doc_id']} Text: {result['entity']['text']}")
以下为输出。稠密向量搜索结果中,仅前两个结果为和朱熹有关的故事。示例中我们精简了文本以提升可读性。
Sparse Search Results:
Score: 26.221 Id: 244468#1 Text: Zhu Xi, whose family originated in Wuyuan County, Huizhou ...
Score: 26.041 Id: 244468#3 Text: In 1208, eight years after his death, Emperor Ningzong of Song rehabilitated Zhu Xi and honored him ...
Score: 25.772 Id: 244468#2 Text: In 1179, after not serving in an official capacity since 1156, Zhu Xi was appointed Prefect of Nankang Military District (南康軍) ...
Score: 23.905 Id: 5823#39 Text: During the Song dynasty, the scholar Zhu Xi (AD 1130--1200) added ideas from Daoism and Buddhism into Confucianism ...
Score: 23.639 Id: 337115#2 Text: ... During the Song Dynasty, the scholar Zhu Xi (AD 1130--1200) added ideas from Taoism and Buddhism into Confucianism ...
Score: 23.061 Id: 10443808#22 Text: Zhu Xi was one of many critics who argued that ...
Score: 22.577 Id: 55800148#11 Text: In February 1930, Zhu decided to live the life of a devoted revolutionary after leaving his family at home ...
Score: 20.779 Id: 12662060#3 Text: Holding to Confucius and Mencius' conception of humanity as innately good, Zhu Xi articulated an understanding of "li" as the basic pattern of the universe ...
Score: 20.061 Id: 244468#28 Text: Tao Chung Yi (around 1329~1412) of the Ming dynasty: Whilst Master Zhu inherited the orthodox teaching and propagated it to the realm of sages ...
Score: 19.991 Id: 13658250#10 Text: Zhu Shugui was 66 years old (by Chinese reckoning; 65 by Western standards) at the time of his suicide ...
Dense Search Results:
Score: 0.600 Id: 244468#1 Text: Zhu Xi, whose family originated in Wuyuan County, Huizhou ...
Score: 0.706 Id: 244468#3 Text: In 1208, eight years after his death, Emperor Ningzong of Song rehabilitated Zhu Xi and honored him ...
Score: 0.759 Id: 13658250#10 Text: Zhu Shugui was 66 years old (by Chinese reckoning; 65 by Western standards) at the time of his suicide ...
Score: 0.804 Id: 6667852#0 Text: King Zhaoxiang of Qin (; 325--251 BC), or King Zhao of Qin (秦昭王), born Ying Ji ...
Score: 0.818 Id: 259901#4 Text: According to the 3rd-century historical text "Records of the Three Kingdoms", Liu Bei was born in Zhuo County ...
Score: 0.868 Id: 343207#0 Text: Ruzi Ying (; 5 -- 25), also known as Emperor Ruzi of Han and the personal name of Liu Ying (劉嬰), was the last emperor ...
Score: 0.876 Id: 31071887#1 Text: The founder of the Ming dynasty was the Hongwu Emperor (21 October 1328 -- 24 June 1398), who is also known variably by his personal name "Zhu Yuanzhang" ...
Score: 0.882 Id: 2945225#0 Text: Emperor Ai of Tang (27 October 89226 March 908), also known as Emperor Zhaoxuan (), born Li Zuo, later known as Li Chu ...
Score: 0.890 Id: 33230345#0 Text: Li Xi or Li Qi (李谿 per the "Zizhi Tongjian" and the "History of the Five Dynasties" or 李磎 per the "Old Book of Tang" ...
Score: 0.893 Id: 172890#1 Text: The Wusun originally lived between the Qilian Mountains and Dunhuang (Gansu) near the Yuezhi ...
至此,示例已完成,如果无需再使用,可以通过以下代码删除相关内容。
docker-compose down
cd .. && rm -rf milvus_sparse_demo
总结
本文探索了复杂的 Embedding 向量空间,展现了信息检索方法如何从传统的稀疏向量检索和稠密向量检索演变为创新型的 Learned 稀疏向量检索。我们还探究了两种机器学习模型------ BGE-M3 和 SPLADE,介绍了它们是如何生成 Learned 稀疏向量的。
利用这些先进的 Embedding 技术,我们实现了搜索和检索系统优化,为开发直观且响应迅速的平台注入了更多新的可能性。
敬请期待我们后续发布的文章!我们将展示如何在实际应用中利用这些技术,帮助您直观了解它们是如何重新定义信息检索的标准的。
注:
MIRACL链接:
https://huggingface.co/datasets/miracl/miracl