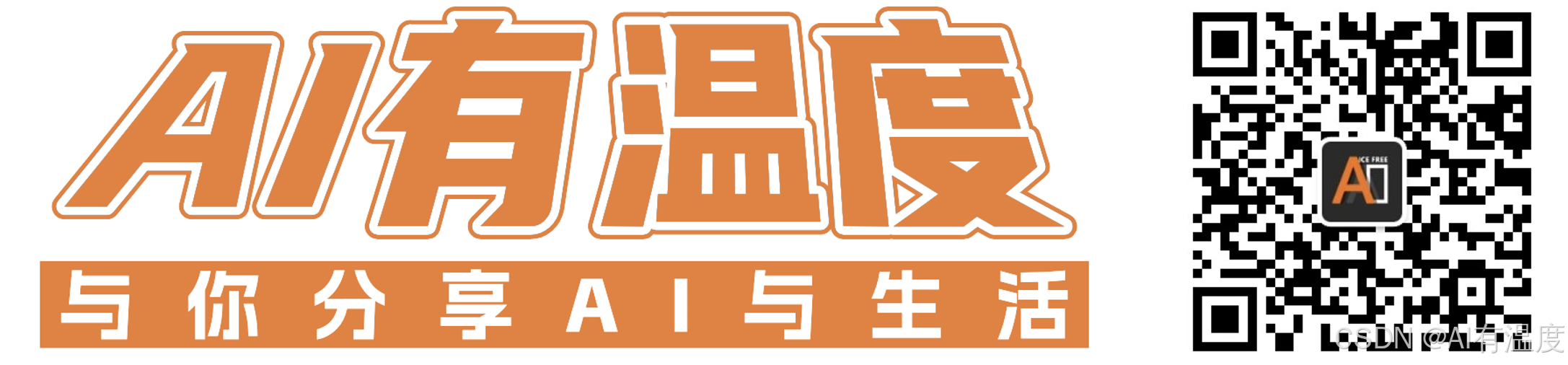
0 导读
在我们不知道什么是深度学习计算框架时,我们可以把PyTorch
看做是Python
的第三方库,在PyTorch
中定义了适用于深度学习的张量Tensor
,以及张量的各类计算。就相当于NumPy
中定义的Array
和对应的科学计算方法,正是这些基本数据类型和对应的方法函数,为我们进一步在PyTorch
上进行深度学习建模提供了基本对象和基本工具。
因此,我们需要熟练掌握PyTorch
中张量的基本操作方法。torch.Tensor
是一种包含单一数据类型元素的多维矩阵。
Python
import torch
torch.__version__
# '1.7.0'
1 张量的创建
张量的最基本创建方法和Numpy
中创建Array
的格式一致,都是创建函数的格式。
1.1 通过列表创建
Python
t = torch.tensor([1, 2])
print(t)
# tensor([1, 2])
1.2 通过元组创建
Python
t = torch.tensor((1, 2))
print(t)
# tensor([1, 2])
1.3 通过Numpy
创建
Python
import numpy as np
n = np.array([1, 2])
t = torch.tensor(n)
print(t)
# tensor([1, 2])
2 张量的数据类型
在Python
中,我们可以使用type()
方法查看一个变量的数据类型。
2.1 type()
Python
t = torch.tensor([1, 2])
print(type(t))
# <class 'torch.Tensor'>
在Python
环境中直接使用type()
方法打印变量t
的类型torch.Tensor
。那么Tensor
下有什么类型呢?我们需要使用dtype
方法进行查看。
2.2 dtype
Python
t = torch.tensor([1, 2])
print(t.dtype)
# torch.int64
我们可以看到t
的大类是Tensor
,更具体的说,它是torch.int64
类型的变量。
2.3 type()
与dtype
的不同
Python
i = torch.tensor([1, 2])
f = torch.tensor([1.0, 2.0])
print(type(i), i.dtype, sep = ' , ')
print(type(f), f.dtype, sep = ' , ')
# <class 'torch.Tensor'> , torch.int64
# <class 'torch.Tensor'> , torch.float32
我们可以看到,type()
不能识别出Tensor
内部的数据类型,只能识别出变量的基本类型是Tensor
,而dtype
方法可以识别出变量具体为哪种类型的Tensor
。
2.4 PyTorch
中Tensor
的数据类型
在PyTorch
中我们常用Tensor
的数据类型有整数型、浮点型和布尔型。具体如下:
数据类型 | dtype |
---|---|
32bit浮点数 | torch.float32或torch.float |
64bit浮点数 | torch.float64或torch.double |
16bit浮点数 | torch.half |
8bit无符号整数 | torch.unit8 |
8bit有符号整数 | torch.int8 |
16bit有符号整数 | torch.int16或torch.short |
32bit有符号整数 | torch.int32或torch.int |
64bit有符号整数 | torch.int64 |
布尔型 | torch.bool |
复数型 | torch.complex64 |
此外,我们可以在创建张量时通过dtype
参数直接定义它的类型。
Python
t = torch.tensor([1, 2], dtype = torch.float64)
print(t.dtype)
# torch.float64
3 张量类型的转化
3.1 张量类型的隐式转化
和Numpy
中Array
相同,当张量各元素属于不同类型时,系统会自动进行隐式转化。
Python
t = torch.tensor([1.1, 2])
print(t)
# tensor([1.1000, 2.0000])
Python
t = torch.tensor([True, 2])
print(t)
# tensor([1, 2])
3.2 张量类型的转化方法
可以使用.float()
、.int()
等方法对张量类型进行转化。
Python
t = torch.tensor([1, 2])
f = t.float()
print(f)
print(t)
# tensor([1., 2.])
# tensor([1, 2])
需要注意的是,这里并不会改变原来t
的数据类型。
Pytorch张量操作大全:
Pytorch使用教学1-Tensor的创建
Pytorch使用教学2-Tensor的维度
Pytorch使用教学3-特殊张量的创建与类型转化
Pytorch使用教学4-张量的索引
Pytorch使用教学5-视图view与reshape的区别
Pytorch使用教学6-张量的分割与合并
Pytorch使用教学7-张量的广播
Pytorch使用教学8-张量的科学运算
Pytorch使用教学9-张量的线性代数运算
Pytorch使用教学10-张量操作方法大总结