💎 我的主页:************************************************************************************************************************************************************************************************************************************************************2的n次方_****************************************************************************************************************************************************************************************************************************************************************

💎1. 引言
随着物联网设备数量的爆炸性增长和对实时处理需求的增加,边缘计算与人工智能(Edge AI)成为一个热门话题。Edge AI 通过在本地设备上运行 AI 算法,减少对云计算的依赖,实现低延迟、高效能的智能应用。这在自动驾驶、智能家居、工业自动化等领域有着广泛的应用前景。
💎2. 什么是边缘计算与 AI?
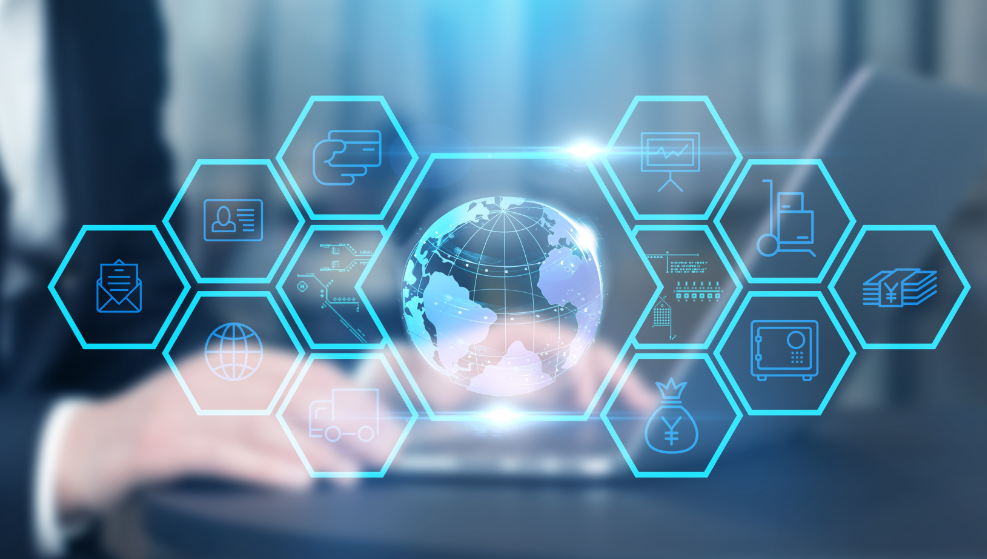
边缘计算是一种分布式计算范式,旨在将计算和数据存储资源移动到数据生成的边缘设备上,而不是依赖于集中式的数据中心。Edge AI 则是在边缘计算架构中部署 AI 模型,使其能够在本地设备上进行实时数据处理和决策。
💎3. 边缘计算与 AI 的优势
低延迟:由于数据处理在本地进行,边缘计算显著减少了数据传输的延迟,满足实时应用的需求。
节省带宽:通过在本地处理数据,只传输必要的信息到云端,大大减少了带宽消耗。
增强隐私:本地数据处理有助于保护用户隐私,减少数据泄露的风险。
高可靠性:即使没有网络连接,本地设备仍然可以继续工作,提高系统的可靠性和稳定性。
💎4. 边缘计算与 AI 的应用场景
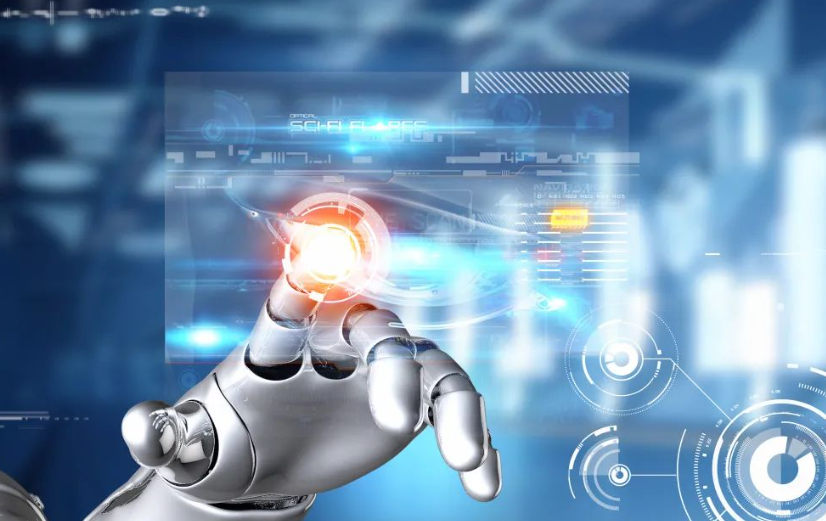
1. 自动驾驶:自动驾驶车辆需要实时处理大量传感器数据,如摄像头、雷达和激光雷达。Edge AI 能够在本地进行快速决策,确保行车安全。
2. 智能家居:智能音箱、安防摄像头等设备通过 Edge AI 实现本地语音识别、图像处理等功能,提升用户体验。
3. 工业自动化:在制造业中,Edge AI 可以在生产线本地进行质量检测、设备监控和故障预测,提升生产效率和产品质量。
💎5. 边缘计算与 AI 的技术实现
💎5.1 边缘设备
边缘设备可以是各种类型的硬件,包括单板计算机(如 Raspberry Pi)、嵌入式系统、智能摄像头和工业控制器等。这些设备通常具有有限的计算资源和电源约束,因此需要高效的 AI 模型。
💎5.2 边缘 AI 框架
有多种框架和工具可以帮助开发和部署 Edge AI 模型:
- TensorFlow Lite:适用于移动设备和嵌入式设备的轻量级深度学习框架。
- OpenVINO:由英特尔开发的工具包,用于优化和部署深度学习推理。
- NVIDIA Jetson:适用于机器人、无人机和智能摄像头的 AI 计算平台。
💎6. 实战:使用 TensorFlow Lite 实现图像分类
以下是一个使用 TensorFlow Lite 在 Raspberry Pi 上实现图像分类的示例。
💎6.1 安装依赖
首先,确保你的 Raspberry Pi 已经安装了 Python 和 TensorFlow Lite:
bash
sudo apt-get update
sudo apt-get install -y python3-pip
pip3 install tflite-runtime
pip3 install numpy Pillow
💎6.2 下载模型
下载预训练的 MobileNet V2 模型,并将其转换为 TensorFlow Lite 格式:
python
import tensorflow as tf
model = tf.keras.applications.MobileNetV2(weights="imagenet", input_shape=(224, 224, 3))
converter = tf.lite.TFLiteConverter.from_keras_model(model)
tflite_model = converter.convert()
with open("mobilenet_v2.tflite", "wb") as f:
f.write(tflite_model)
💎6.3 运行推理
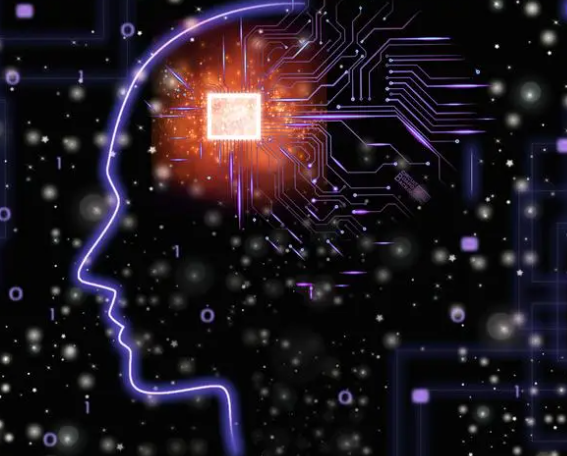
使用 TensorFlow Lite 在 Raspberry Pi 上运行图像分类:
python
import numpy as np
import tflite_runtime.interpreter as tflite
from PIL import Image
# 加载模型
interpreter = tflite.Interpreter(model_path="mobilenet_v2.tflite")
interpreter.allocate_tensors()
# 获取输入和输出张量
input_details = interpreter.get_input_details()
output_details = interpreter.get_output_details()
# 预处理图像
def preprocess_image(image_path):
img = Image.open(image_path).resize((224, 224))
img = np.array(img, dtype=np.float32)
img = np.expand_dims(img, axis=0)
img /= 255.0
return img
# 运行推理
def classify_image(image_path):
img = preprocess_image(image_path)
interpreter.set_tensor(input_details[0]['index'], img)
interpreter.invoke()
output_data = interpreter.get_tensor(output_details[0]['index'])
predictions = np.squeeze(output_data)
return predictions
# 测试图像分类
image_path = "test_image.jpg"
predictions = classify_image(image_path)
top_k = predictions.argsort()[-5:][::-1]
print(f"Top predictions: {top_k}")
💎7. 结论
边缘计算与 AI 的结合为实时智能应用带来了巨大的潜力。通过在本地设备上运行 AI 模型,可以实现低延迟、高效能的实时数据处理和决策。在未来,随着硬件性能的不断提升和 AI 技术的进一步发展,Edge AI 将在更多领域发挥重要作用。
