前言
Opentelemetry
分布式链路跟踪( Distributed Tracing
)的概念最早是由 Google 提出来的,发展至今技术已经比较成熟,也是有一些协议标准可以参考。目前在 Tracing
技术这块比较有影响力的是两大开源技术框架:Netflix 公司开源的 OpenTracing
和 Google 开源的 OpenCensus
。两大框架都拥有比较高的开发者群体。为形成统一的技术标准,两大框架最终磨合成立了 OpenTelemetry
项目,简称 otel
。otel 有链路追踪和监控告警两大块,关于监控告警,可以查看另一篇文章:Go 链路追踪入门 Opentelemetry
Prometheus
Prometheus
源自 SoundCloud,拥有一整套开源系统监控和警报工具包,是支持 OpenTelemetry
的系统之一,是 CNCF 的第二个项目。
Grafana
Grafana 是一个开源的分析和可视化平台,它允许你查询、可视化和警报来自各种数据源的数据。它提供了一个用户友好的界面,用于创建和共享仪表板、图表和警报。Grafana 支持广泛的数据源,其中就包括 Prometheus
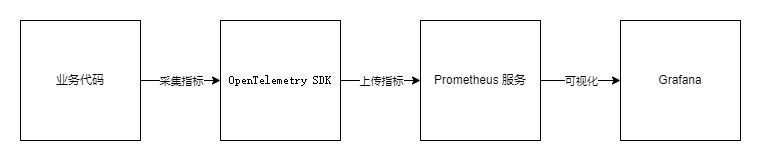
基础概念
这里为了简单入门,尽量简单的介绍一些抽象概念,结合着代码理解,如果不能理解也没关系,代码写着写着自然就明白了:
Meter Provider
用于接口化管理全局的 Meter
创建,相当于全局的监控指标管理工厂。
Meter
用于接口化创建并管理全局的 Instrument
,不同的 Meter
可以看做是不同的程序组件。
Instrument
用于管理不同组件下的各个不同类型的指标,例如 http.server.request.total
Measurements
对应指标上报的具体的 DataPoint
指标数据,是一系列的数值项。
Metric Reader
用于实现对指标的数据流读取,内部定义了具体操作指标的数据结构。OpenTelemetry
官方社区提供了多种灵活的 Reader
实现,例如 PeridRader、ManualReader
等。
Metric Exporter
Exporter
用于暴露本地指标到对应的第三方厂商,例如:Promtheus、Zipkin
等。
指标类型
OpenTelemetry metrics
有许多不同指标类型,可以把它想象成类似于 int, float
这种的变量类型:
Counter: 只增不减的指标,比如 http
请求总数,字节大小;
**Asynchronous Counter:**异步 Counter;
UpDownCounter: 可增可减的指标,比如 http
活动连接数;
**Asynchronous UpDownCounter:**异步 Counter;
Gauge: 可增可减的指标,瞬时计量的值,比如 CPU
使用,它是异步的;
Histogram :分组聚合指标,这个较为难以理解一些,可以移步此处查看,当然,后文也会有一个详细的例子来使用它。
实战:采集指标
废话了一堆,终于可以实战了。我们先以 http
请求总数为例来走一遍整个采集指标流程。安装扩展:
go get github.com/prometheus/client_golang
go get go.opentelemetry.io/otel/exporters/prometheus
go get go.opentelemetry.io/otel/metric
go get go.opentelemetry.io/otel/sdk/metric
打开 main.go
,编写以下代码:
package main
import (
"context"
"fmt"
"log"
"net/http"
"os"
"os/signal"
"github.com/prometheus/client_golang/prometheus/promhttp"
"go.opentelemetry.io/otel/exporters/prometheus"
api "go.opentelemetry.io/otel/metric"
"go.opentelemetry.io/otel/sdk/metric"
)
const meterName = "oldme_prometheus_testing"
var (
requestHelloCounter api.Int64Counter
)
func main() {
ctx := context.Background()
// 创建 prometheus 导出器
exporter, err := prometheus.New()
if err != nil {
log.Fatal(err)
}
// 创建 meter
provider := metric.NewMeterProvider(metric.WithReader(exporter))
meter := provider.Meter(meterName)
// 创建 counter 指标类型
requestHelloCounter, err = meter.Int64Counter("requests_hello_total")
if err != nil {
log.Fatal(err)
}
go serveMetrics()
ctx, _ = signal.NotifyContext(ctx, os.Interrupt)
<-ctx.Done()
}
func serveMetrics() {
log.Printf("serving metrics at localhost:2223/metrics")
http.Handle("/metrics", promhttp.Handler())
http.Handle("/index", http.HandlerFunc(func(w http.ResponseWriter, r *http.Request) {
// 记录 counter 指标
requestHelloCounter.Add(r.Context(), 1)
_, _ = w.Write([]byte("Hello, Otel!"))
}))
err := http.ListenAndServe(":2223", nil) //nolint:gosec // Ignoring G114: Use of net/http serve function that has no support for setting timeouts.
if err != nil {
fmt.Printf("error serving http: %v", err)
return
}
}
在我们的代码中,我们定义一个名字为 requests_hello_total
的 Int64Counter
指标类型,Int64Counter
代表这是一个只增不减的 int64
数值,用作记录请求总数正好合适。运行我们的程序,如果不出错的话,访问 http://localhost:2223/index 可以看到 Hello, Otel!
。并且我们访问 http://localhost:2223/metrics 可以看到指标数据:
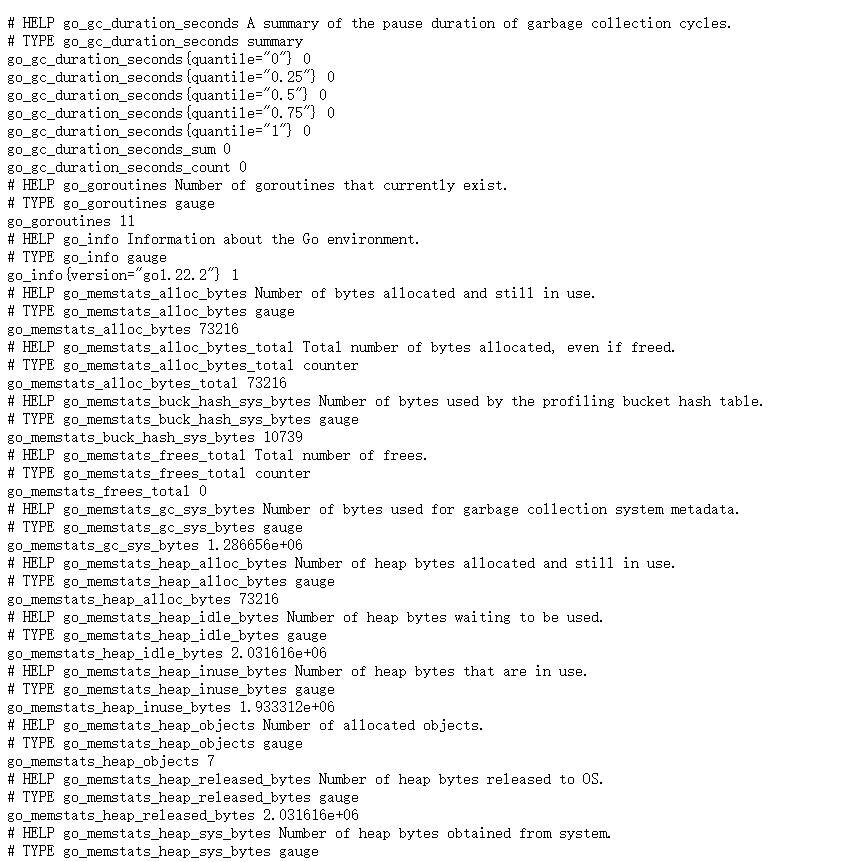
这里数据还没有进行可视化,我们先把流程走通,多访问几次 http://localhost:2223/index 可以看到 requests_hello_total
会增加:

Histogram
接下来我们采集一下 Histogram
指标,统计在 0.1, 0.2, 0.5, 1, 2, 5
秒以内的 http
请求数,在 main.go
中加上相关代码,可以直接复制过去:
package main
import (
"context"
"fmt"
"log"
"math/rand"
"net/http"
"os"
"os/signal"
"time"
"github.com/prometheus/client_golang/prometheus/promhttp"
"go.opentelemetry.io/otel/exporters/prometheus"
api "go.opentelemetry.io/otel/metric"
"go.opentelemetry.io/otel/sdk/metric"
)
const meterName = "oldme_prometheus_testing"
var (
requestHelloCounter api.Int64Counter
requestDurationHistogram api.Float64Histogram
)
func main() {
ctx := context.Background()
// 创建 prometheus 导出器
exporter, err := prometheus.New()
if err != nil {
log.Fatal(err)
}
// 创建 meter
provider := metric.NewMeterProvider(metric.WithReader(exporter))
meter := provider.Meter(meterName)
// 创建 counter 指标类型
requestHelloCounter, err = meter.Int64Counter("requests_hello_total")
if err != nil {
log.Fatal(err)
}
// 创建 Histogram 指标类型
requestDurationHistogram, err = meter.Float64Histogram(
"request_hello_duration_seconds",
api.WithDescription("记录 Hello 请求的耗时统计"),
api.WithExplicitBucketBoundaries(0.1, 0.2, 0.5, 1, 2, 5),
)
if err != nil {
log.Fatal(err)
}
go serveMetrics()
go goroutineMock()
ctx, _ = signal.NotifyContext(ctx, os.Interrupt)
<-ctx.Done()
}
func serveMetrics() {
log.Printf("serving metrics at localhost:2223/metrics")
http.Handle("/metrics", promhttp.Handler())
http.Handle("/index", http.HandlerFunc(func(w http.ResponseWriter, r *http.Request) {
// 记录 counter 指标
requestHelloCounter.Add(r.Context(), 1)
// 计算请求处理时间
startTime := time.Now()
// 模拟请求处理时间
time.Sleep(time.Duration(rand.Intn(3)) * time.Second)
defer func() {
duration := time.Since(startTime).Seconds()
requestDurationHistogram.Record(r.Context(), duration)
}()
_, _ = w.Write([]byte("Hello, Otel!"))
}))
err := http.ListenAndServe(":2223", nil) //nolint:gosec // Ignoring G114: Use of net/http serve function that has no support for setting timeouts.
if err != nil {
fmt.Printf("error serving http: %v", err)
return
}
}
// 随机模拟若干个协程
func goroutineMock() {
for {
go func() {
// 等待若干秒
var s = time.Duration(rand.Intn(10))
time.Sleep(s * time.Second)
}()
time.Sleep(1 * time.Millisecond)
}
}
走到这里,代码层面结束了,已经成功一半了,代码开源在 Github。之后我们就可以安装 Prometheus
服务端和 Grafana
来进行数据可视化。
安装 Prometheus
Prometheus
有多种安装方式,我这里依旧采用 Docker
安装,当然,你也可以使用其他方式安装,具体安装方式可以参考其他文章,后续 Grafana
同理,不在赘述,在 Prometheus.yml
中填写 targets
我们的地址:
scrape_configs:
- job_name: "prometheus"
static_configs:
- targets: ["localhost:2223"]
Prometheus
会自动去 {{target}}/metrics
中拉取我们的指标。之后在浏览器打开 Promethues
的地址,例如我的是:http://localhost:9090,如果全部正常的话可以在 status:targets
中看见我们的指标:
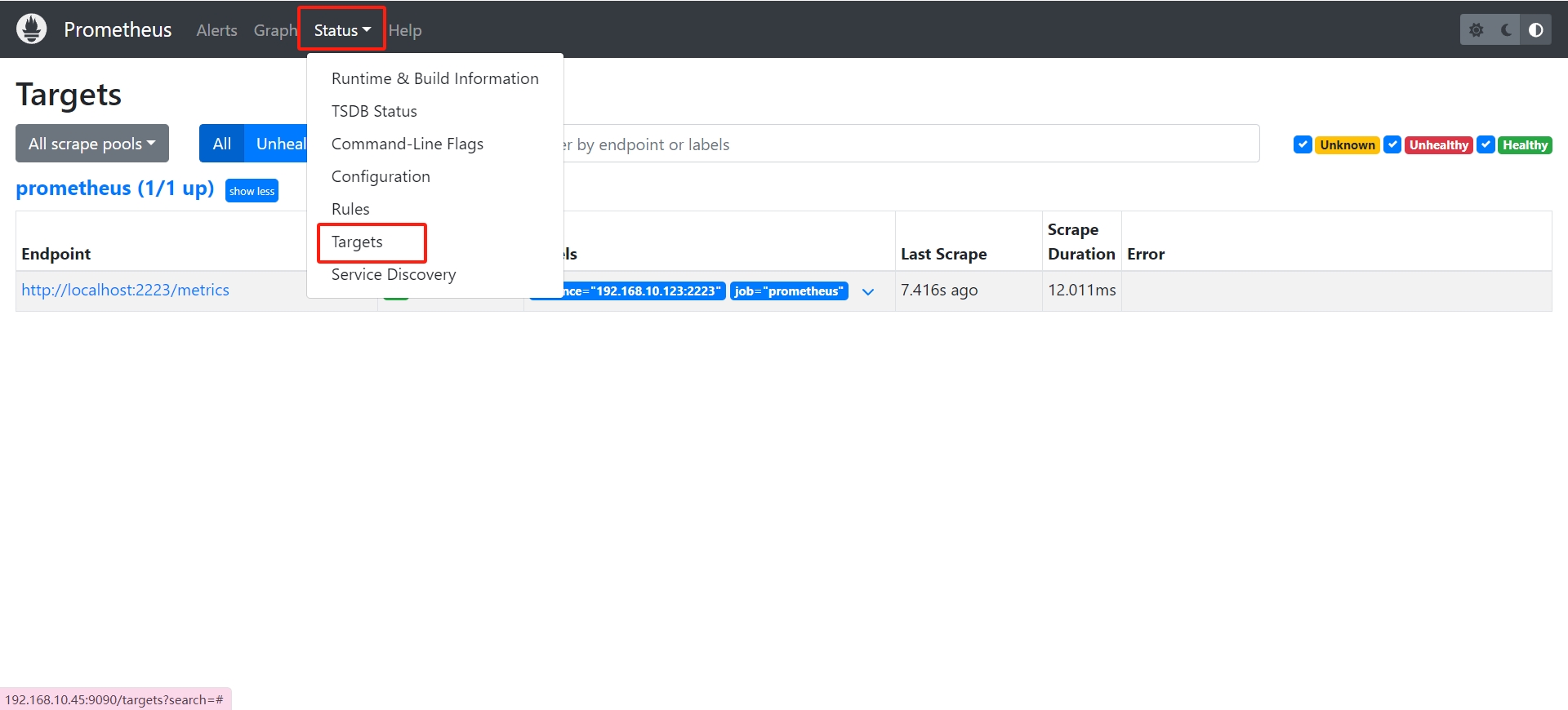
在 Promethues
的首页查询 requests_hello_total
指标可以看到可视化的图表:
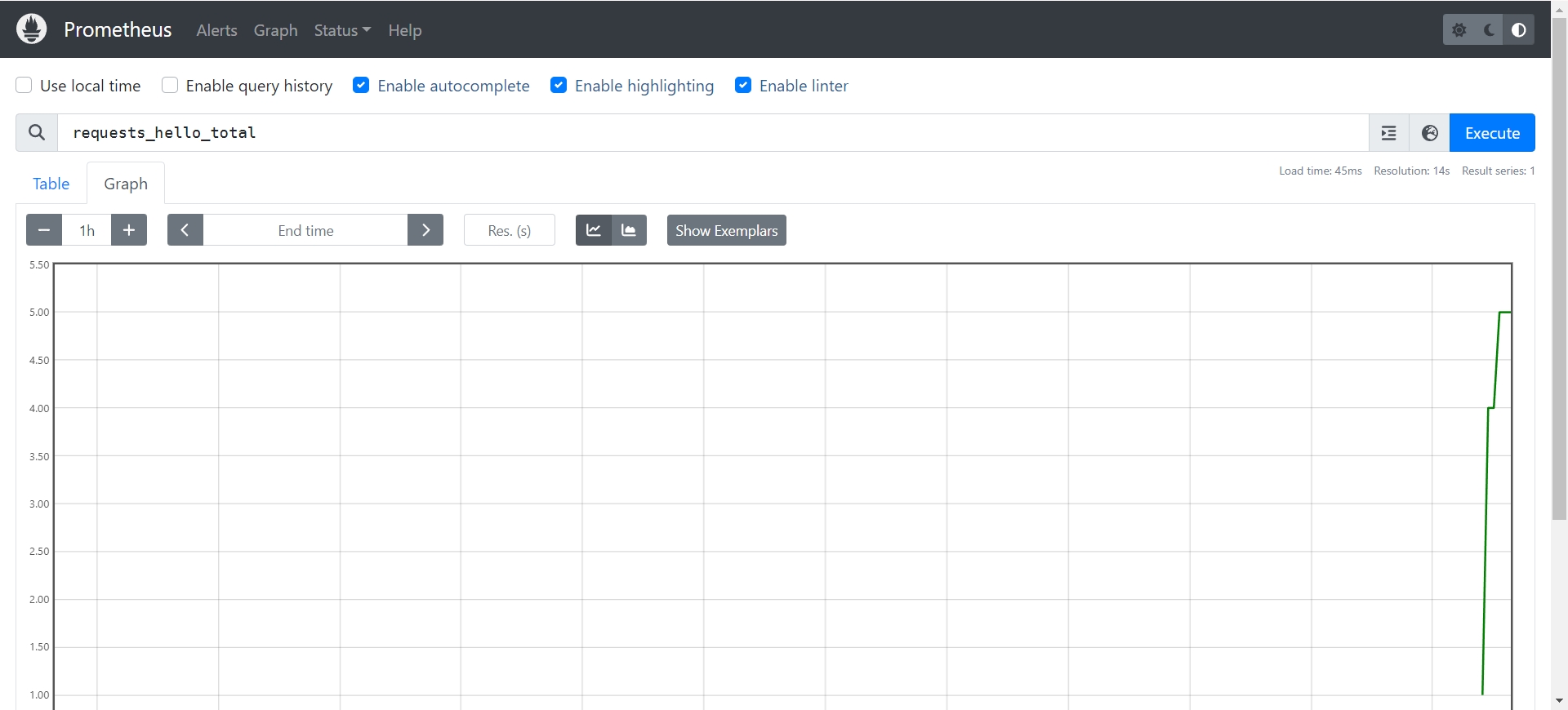
安装 Grafana
我的 Grafana
安装好了,登录进去后是这样的(我更改后默认颜色):
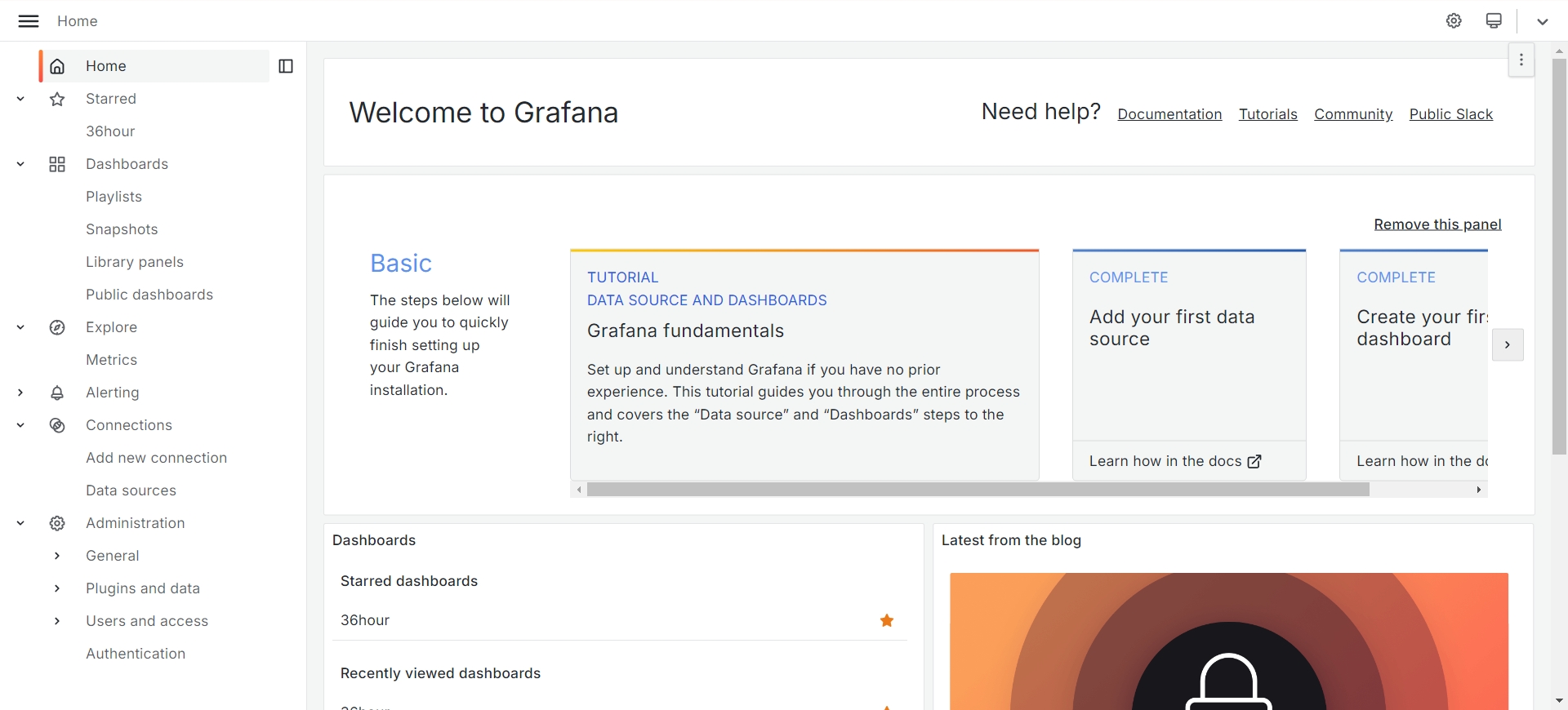
在 Data source
中添加 Prometheus
服务器,然后在 Dashboard
中添加我们想要监控的指标,即可看到更美观的图表: