一、引言
令牌桶算法(Token Bucket Algorithm, TBA)是一种流行于网络通信领域的流量控制和速率限制算法。它允许一定程度的突发传输,同时限制长时间内的传输速率。令牌桶算法广泛应用于网络流量管理、API请求限流等场景。其基本原理是通过一个"桶"来控制数据的发送速率。桶内存储一定数量的"令牌",每个令牌代表一个数据包的发送权限。令牌以固定的速率生成,数据包的发送需要消耗令牌。若桶内没有令牌,数据包则需要等待,直到有令牌可用。
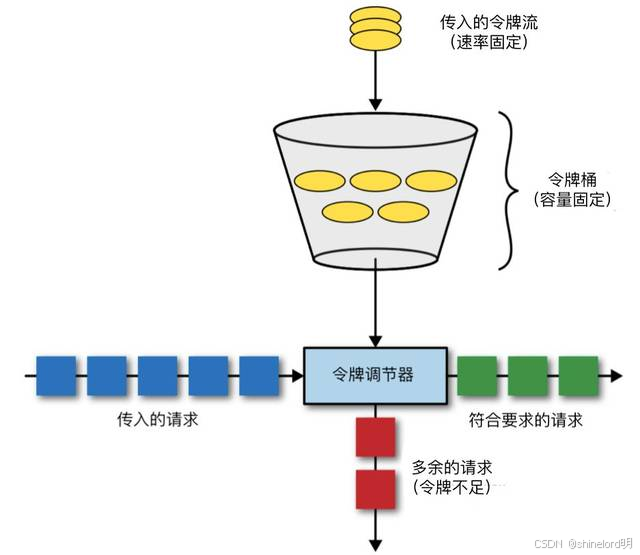
二、算法原理
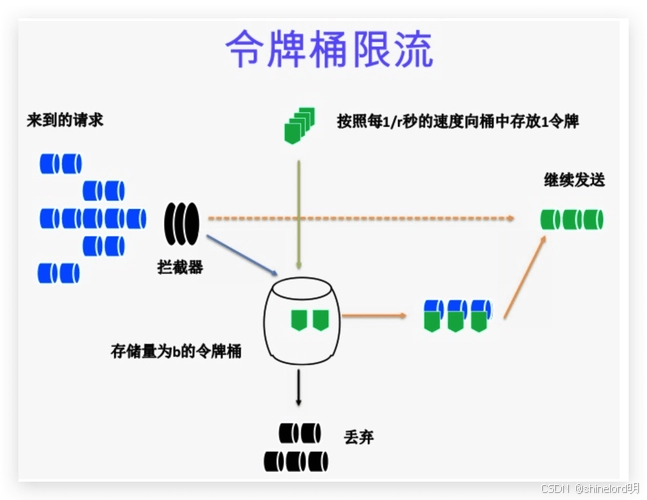
令牌桶算法基于以下核心概念:
- 令牌桶:一个虚拟的容器,用来存放固定数量的令牌。
- 令牌填充速率:系统以固定的速率向桶中添加令牌。
- 令牌消耗:每当一个数据包发送时,就从桶中移除一个k如果桶中没有令牌,数据包将被延迟发送或丢弃,直到桶中有足够的令牌。
三、数据结构
令牌桶算法需要以下数据结构:
- 令牌桶:存储令牌的容器。
- 时间戳:记录上一次填充令牌的时间。
四、算法使用场景
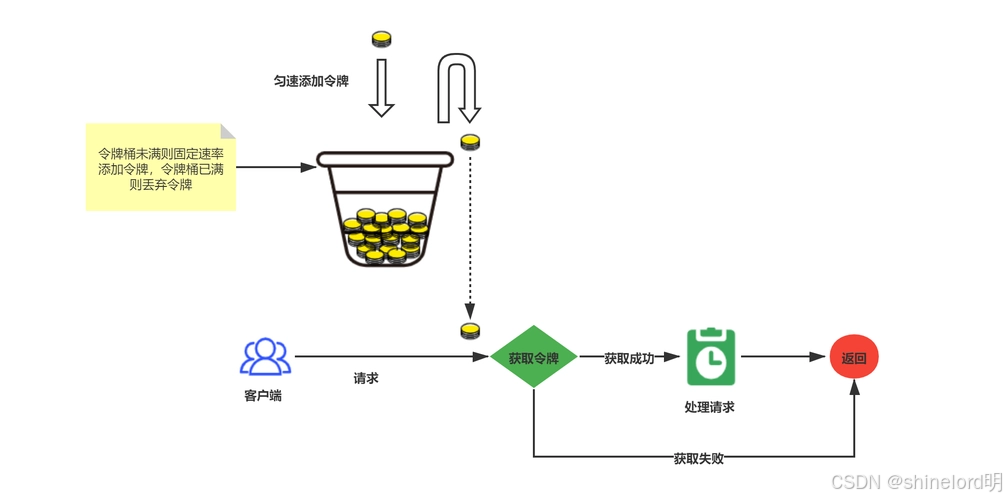
令牌桶算法适用于:
- 网络带宽管理:控制用户的网络流量,防止滥用。
- API速率限制:限制API调用频率,保护后端服务。
- 云服务提供商:为不同级别的用户提供不同速率的服务。
- 任务调度:限制任务执行的速率,避免资源争用。
五、算法实现
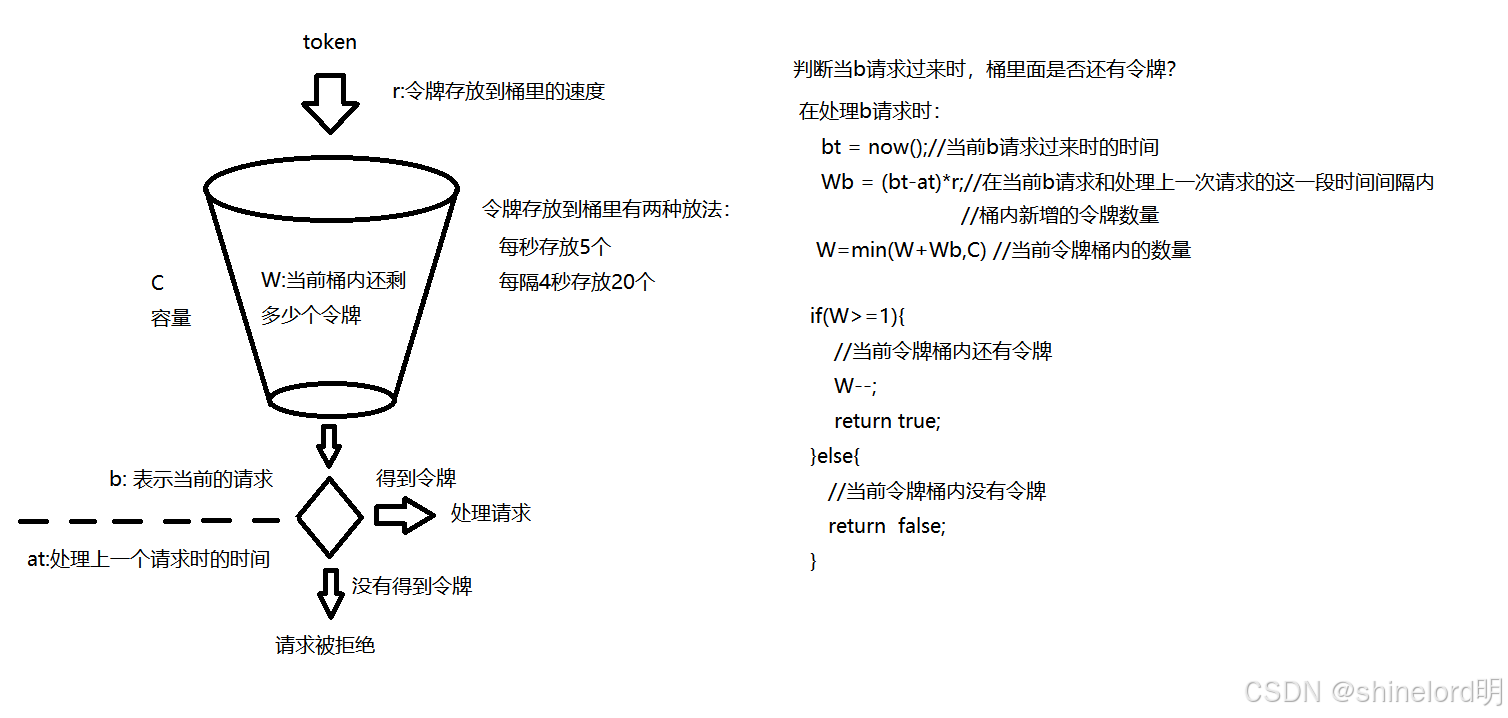
初始化:设置令牌桶的容量和生成令牌的速率。
生成令牌:以固定的时间间隔向桶中添加令牌,直到达到桶的最大容量。
请求处理:检查桶中是否有令牌。如果有,消耗一个令牌并处理请求。如果没有,根据策略拒绝请求或等待。
伪代码:
cpp
function acquireToken(tokensRequested):
currentTime = getCurrentTime()
elapsedTime = currentTime - lastRefillTimestamp
newTokens = elapsedTime * refillRate
tokens = min(capacity, tokens + newTokens)
lastRefillTimestamp = currentTime
if tokens >= tokensRequested:
tokens -= tokensRequested
return true
else:
return false
六、其他同类算法对比
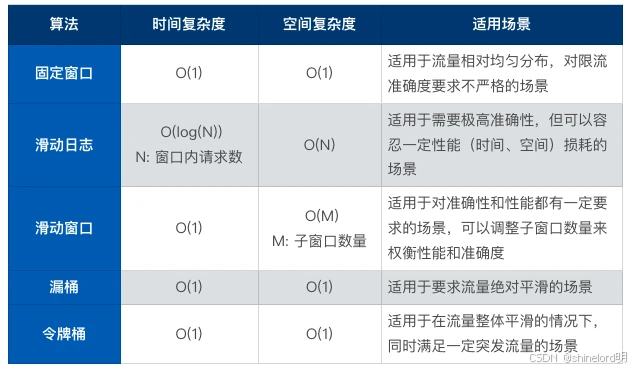
**漏桶算法 (Leaky Bucket):**漏桶算法具有恒定的输出速率,处理数据的速度不会因为突发流量而变化。适合于需要恒定速率输出的场景。
**固定窗口计数算法 (Fixed Window Counter):**统计在固定时间窗口内的请求数量,适合简单的请求限制,但对突发流量不够友好。
**滑动窗口计数算法 (Sliding Window Counter):**在固定时间窗口中,使用滑动窗口来统计请求数量,能够更平滑地控制流量。
**计数器算法(Counter Algorithm):**计数器算法通过计数器来限制一段时间内的请求次数,适用于简单的速率限制场景。
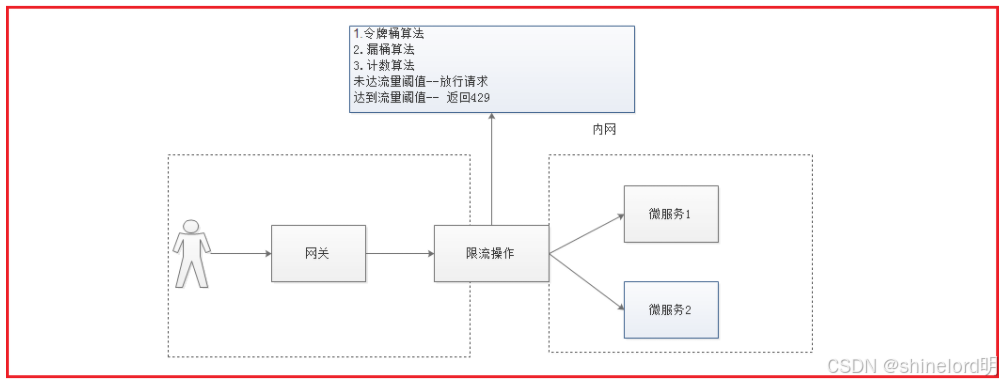
七、多语言实现
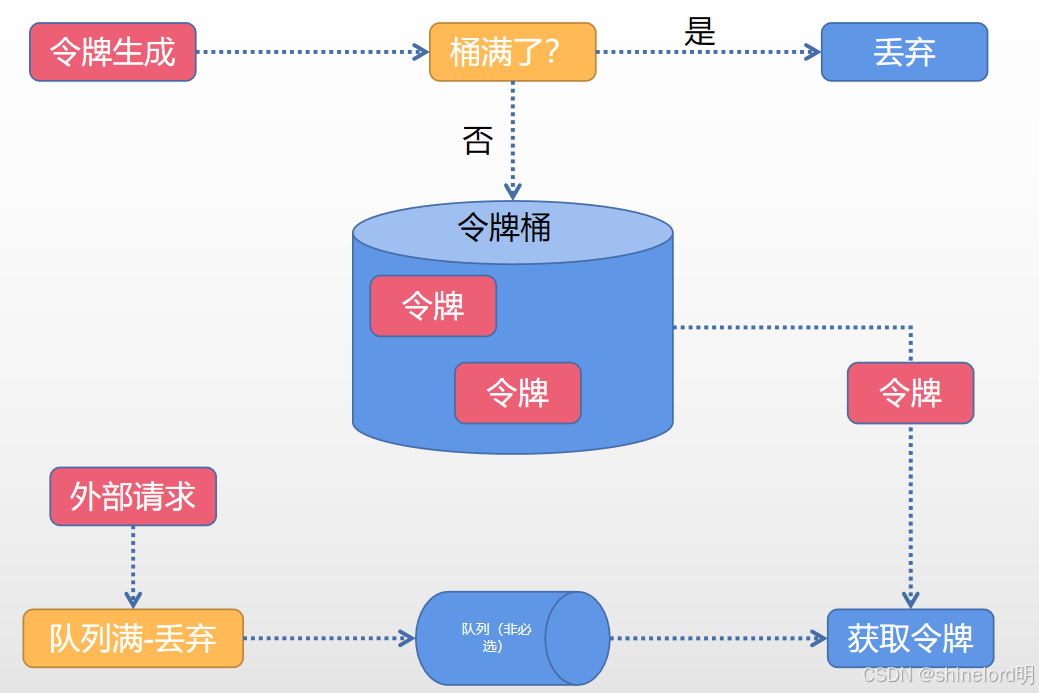
Java
java
import java.util.concurrent.TimeUnit;
public class TokenBucket {
private final long capacity;
private long tokens;
private final long refillRate;
private long lastRefillTime;
public TokenBucket(long capacity, long refillRate) {
this.capacity = capacity;
this.tokens = capacity;
this.refillRate = refillRate;
this.lastRefillTime = System.currentTimeMillis();
}
private void refill() {
long now = System.currentTimeMillis();
long elapsed = now - lastRefillTime;
long newTokens = elapsed * refillRate / 1000;
tokens = Math.min(capacity, tokens + newTokens);
lastRefillTime = now;
}
public synchronized boolean consume(long tokensToConsume) {
refill();
if (tokens >= tokensToConsume) {
tokens -= tokensToConsume;
return true;
}
return false;
}
}
Python
python
import time
from threading import Lock
class TokenBucket:
def __init__(self, capacity, refill_rate):
self.capacity = capacity
self.tokens = capacity
self.refill_rate = refill_rate
self.last_refill_time = time.time()
self.lock = Lock()
def refill(self):
current_time = time.time()
elapsed = current_time - self.last_refill_time
new_tokens = elapsed * self.refill_rate
self.tokens = min(self.capacity, self.tokens + new_tokens)
self.last_refill_time = current_time
def consume(self, tokens_to_consume):
with self.lock:
self.refill()
if self.tokens >= tokens_to_consume:
self.tokens -= tokens_to_consume
return True
return False
C++
cpp
#include <chrono>
#include <mutex>
class TokenBucket {
public:
TokenBucket(long capacity, long refillRate)
: capacity(capacity), tokens(capacity), refillRate(refillRate),
lastRefillTime(std::chrono::steady_clock::now()) {}
bool consume(long tokensToConsume) {
std::lock_guard<std::mutex> lock(mtx);
refill();
if (tokens >= tokensToConsume) {
tokens -= tokensToConsume;
return true;
}
return false;
}
private:
long capacity;
long tokens;
long refillRate;
std::chrono::steady_clock::time_point lastRefillTime;
std::mutex mtx;
void refill() {
auto now = std::chrono::steady_clock::now();
auto elapsed = std::chrono::duration_cast<std::chrono::seconds>(now - lastRefillTime).count();
long newTokens = elapsed * refillRate;
tokens = std::min(capacity, tokens + newTokens);
lastRefillTime = now;
}
};
Go
Go
package main
import (
"sync"
"time"
)
type TokenBucket struct {
capacity int64
tokens int64
refillRate int64
lastRefillTime time.Time
mu sync.Mutex
}
func NewTokenBucket(capacity int64, refillRate int64) *TokenBucket {
return &TokenBucket{
capacity: capacity,
tokens: capacity,
refillRate: refillRate,
lastRefillTime: time.Now(),
}
}
func (tb *TokenBucket) refill() {
now := time.Now()
elapsed := now.Sub(tb.lastRefillTime).Seconds()
newTokens := int64(elapsed) * tb.refillRate
tb.tokens = min(tb.capacity, tb.tokens+newTokens)
tb.lastRefillTime = now
}
func (tb *TokenBucket) Consume(tokensToConsume int64) bool {
tb.mu.Lock()
defer tb.mu.Unlock()
tb.refill()
if tb.tokens >= tokensToConsume {
tb.tokens -= tokensToConsume
return true
}
return false
}
func min(a, b int64) int64 {
if a < b {
return a
}
return b
}
八. 实际的服务应用场景代码框架
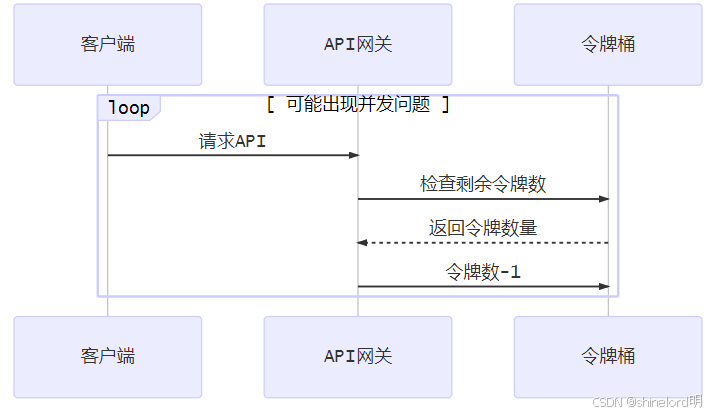
场景描述
假设我们正在开发一个API服务,需要限制每个用户的请求频率,以防止滥用。我们可以使用令牌桶算法来实现这一功能。
代码框架
简单的Java Spring Boot应用程序的代码框架,展示如何使用令牌桶算法进行API请求限流。
项目结构
src
└── main
├── java
│ └── com
│ └── example
│ ├── TokenBucket.java
│ └── ApiController.java
└── resources
└── application.properties
算法逻辑
TokenBucket.java
java
import org.springframework.stereotype.Component;
import java.util.concurrent.TimeUnit;
@Component
public class TokenBucket {
private final long capacity = 10; // 最大令牌数
private long tokens = capacity;
private final long refillRate = 1; // 每秒生成1个令牌
private long lastRefillTime = System.currentTimeMillis();
private synchronized void refill() {
long now = System.currentTimeMillis();
long elapsed = now - lastRefillTime;
long newTokens = TimeUnit.MILLISECONDS.toSeconds(elapsed) * refillRate;
tokens = Math.min(capacity, tokens + newTokens);
lastRefillTime = now;
}
public synchronized boolean consume(long tokensToConsume) {
refill();
if (tokens >= tokensToConsume) {
tokens -= tokensToConsume;
return true;
}
return false;
}
}
控制器
ApiController.java
java
import org.springframework.beans.factory.annotation.Autowired;
import org.springframework.web.bind.annotation.GetMapping;
import org.springframework.web.bind.annotation.RequestParam;
import org.springframework.web.bind.annotation.RestController;
@RestController
public class ApiController {
@Autowired
private TokenBucket tokenBucket;
@GetMapping("/api/resource")
public String getResource(@RequestParam String userId) {
if (tokenBucket.consume(1)) {
return "Resource accessed successfully.";
} else {
return "Too many requests. Please try again later.";
}
}
}
配置
application.properties
bash
server.port=8080
启动服务
在项目根目录下,使用以下命令启动Spring Boot应用:
bash
./mvnw spring-boot:run
测试
使用Postman或cURL测试API:
bash
curl "http://localhost:8080/api/resource?userId=123"
令牌桶算法是一种有效的流量控制技术,能够平滑流量并限制突发请求。通过在桶中动态生成和管理令牌来限制数据发送速率。算法的核心原理是设置桶的容量和令牌生成速率,从而控制请求处理的速率,适用于网络流量控制和API限流等场景。相比其他算法(如漏桶算法、固定窗口计数等),令牌桶能更灵活地应对突发流量。