模块出处
[CVPR 22] [link] [code] Pyramid Grafting Network for One-Stage High Resolution Saliency Detection
模块名称
Cross-Model Grafting Module (CMGM)
模块作用
Transformer与CNN之间的特征融合
模块结构
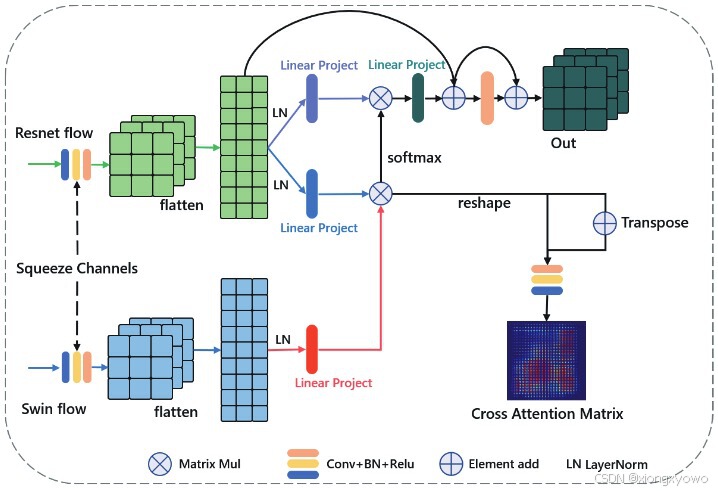
模块思想
Transformer在全局特征上更优,CNN在局部特征上更优,对这两者进行进行融合的最简单做法是直接相加或相乘。但是,相加或相乘本质上属于"局部"操作,如果某片区域两个特征的不确定性都较高,则会带来许多噪声。为此,本文提出了CMGM模块,通过交叉注意力的形式引入更为广泛的信息来增强融合效果。
模块代码
python
import torch.nn.functional as F
import torch.nn as nn
import torch
class CMGM(nn.Module):
def __init__(self, dim, num_heads=8, qkv_bias=True, qk_scale=None):
super().__init__()
self.num_heads = num_heads
head_dim = dim // num_heads
self.scale = qk_scale or head_dim ** -0.5
self.k = nn.Linear(dim, dim , bias=qkv_bias)
self.qv = nn.Linear(dim, dim * 2, bias=qkv_bias)
self.proj = nn.Linear(dim, dim)
self.act = nn.ReLU(inplace=True)
self.conv = nn.Conv2d(8,8,kernel_size=3, stride=1, padding=1)
self.lnx = nn.LayerNorm(64)
self.lny = nn.LayerNorm(64)
self.bn = nn.BatchNorm2d(8)
self.conv2 = nn.Sequential(
nn.Conv2d(64,64,kernel_size=3, stride=1, padding=1),
nn.BatchNorm2d(64),
nn.ReLU(inplace=True),
nn.Conv2d(64,64,kernel_size=3, stride=1, padding=1),
nn.BatchNorm2d(64),
nn.ReLU(inplace=True)
)
def forward(self, x, y):
batch_size = x.shape[0]
chanel = x.shape[1]
sc = x
x = x.view(batch_size, chanel, -1).permute(0, 2, 1)
sc1 = x
x = self.lnx(x)
y = y.view(batch_size, chanel, -1).permute(0, 2, 1)
y = self.lny(y)
B, N, C = x.shape
y_k = self.k(y).reshape(B, N, 1, self.num_heads, C // self.num_heads).permute(2, 0, 3, 1, 4)
x_qv= self.qv(x).reshape(B,N,2,self.num_heads, C // self.num_heads).permute(2, 0, 3, 1, 4)
x_q, x_v = x_qv[0], x_qv[1]
y_k = y_k[0]
attn = (x_q @ y_k.transpose(-2, -1)) * self.scale
attn = attn.softmax(dim=-1)
x = (attn @ x_v).transpose(1, 2).reshape(B, N, C)
x = self.proj(x)
x = (x+sc1)
x = x.permute(0,2,1)
x = x.view(batch_size,chanel,*sc.size()[2:])
x = self.conv2(x)+x
return x, self.act(self.bn(self.conv(attn+attn.transpose(-1,-2))))
if __name__ == '__main__':
x = torch.randn([1, 64, 11, 11])
y = torch.randn([1, 64, 11, 11])
cmgm = CMGM(dim=64)
out1, out2 = cmgm(x, y)
print(out1.shape) # out feature 1, 64, 11, 11
print(out2.shape) # cross attention matrix 1, 8, 121, 121