1.背景
本文接【时序预测SARIMAX模型】 一文,采用LSTM模型进行平均温度数据预测。具体的背景和数据分析就不做重复说明,感兴趣可以去看上文即可。
2.LSTM模型
RNN(Recurrent Neural Network,循环神经网络)是一种特殊的神经网络,被广泛应用于序列数据的建模和预测,如自然语言处理、语音识别、时间序列预测等领域。RNN对时间序列的数据有着强大的提取能力,也被称作记忆能力。相对于传统的前馈神经网络,RNN具有循环连接,可以将前一时刻的输出作为当前时刻的输入,从而使得网络可以处理任意长度的序列数据,捕捉序列数据中的长期依赖关系。
图 1
LSTM(Long Short-Term Memory,长短时记忆网络)是一种特殊的 RNN,它通过引入门控机制和记忆单元等结构来增强 RNN 的记忆能力和表达能力。
LSTM 的基本结构包括一个循环单元和三个门控单元:输入门、遗忘门和输出门。循环单元接受当前时刻的输入和前一时刻的输出作为输入,并输出当前时刻的输出和传递到下一时刻的状态。输入门控制当前时刻的输入对状态的影响,遗忘门控制前一时刻的状态对当前状态的影响,输出门控制当前状态对输出的影响。记忆单元则用于存储和传递长期的信息。基于此,使得LSTM具有长期的记忆能力,并且具有防止梯度消失的特点,故我们选择LSTM作为库存预估模型的训练基础。
图 2
3.模型训练
3.1 查看数据信息
python
df.info()
<class 'pandas.core.frame.DataFrame'>
DatetimeIndex: 1462 entries, 2013-01-01 to 2017-01-01
Data columns (total 9 columns):
Column Non-Null Count Dtype
->-- ------ -------------- -----
0 meantemp 1462 non-null float64
1 humidity 1462 non-null float64
2 wind_speed 1462 non-null float64
3 meanpressure 1462 non-null float64
4 year 1462 non-null int32
5 month 1462 non-null int32
6 day 1462 non-null int32
7 dayofweek 1462 non-null int32
8 date 1462 non-null object
dtypes: float64(4), int32(4), object(1)
memory usage: 91.4+ KB
3.2 数据分析
从【时序预测SARIMAX模型】 中可以看到温度数据具有明显的周期特征,因此需要考虑做归一化。
python
from sklearn.preprocessing import RobustScaler, MinMaxScaler
robust_scaler = RobustScaler() # scaler for wind_speed
minmax_scaler = MinMaxScaler() # scaler for humidity
target_transformer = MinMaxScaler()
dl_train['wind_speed'] = robust_scaler.fit_transform(dl_train[['wind_speed']]) # robust for wind_speed
dl_train['humidity'] = minmax_scaler.fit_transform(dl_train[['humidity']]) # minmax for humidity
dl_train['meantemp'] = target_transformer.fit_transform(dl_train[['meantemp']]) # target
dl_test['wind_speed'] = robust_scaler.transform(dl_test[['wind_speed']])
dl_test['humidity'] = minmax_scaler.transform(dl_test[['humidity']])
dl_test['meantemp'] = target_transformer.transform(dl_test[['meantemp']])
display(df.head())
display(dl_train.head())
图 3
3.3 数据准备
py
from tensorflow.keras.models import Sequential
from tensorflow.keras.callbacks import EarlyStopping
def create_dataset(X, y, time_steps=1):
Xs, ys = [], []
for i in range(len(X) - time_steps):
v = X.iloc[i:(i + time_steps)].values
Xs.append(v)
ys.append(y.iloc[i + time_steps])
return np.array(Xs), np.array(ys)
sequence_length = 3 # adjust based on your dataset and experimentation
X_train, y_train = create_dataset(dl_train, dl_train['meantemp'], sequence_length)
X_test, y_test = create_dataset(dl_test, dl_test['meantemp'], sequence_length)
3.4 构建模型及评估
py
from tensorflow.keras.layers import LSTM,Dropout,Dense
# Build the LSTM model
lstm_model = Sequential()
lstm_model.add(LSTM(100, activation='tanh', input_shape=(sequence_length, X_train.shape[2])))
# lstm_model.add(LSTM(128, activation='softsign', input_shape=(sequence_length, X_train.shape[2])))
lstm_model.add(Dropout(0.5))
lstm_model.add(Dense(1))
lstm_model.compile(optimizer='adam', loss='mse')
# Define early stopping callback
early_stopping = EarlyStopping(monitor='val_loss', patience=10, restore_best_weights=True)
# Train the model
history = lstm_model.fit(X_train, y_train, epochs=30, validation_data=(X_test, y_test), batch_size=1, callbacks=[early_stopping])
# Evaluate the model
loss = lstm_model.evaluate(X_test, y_test)
print(f'Validation Loss: {loss}')
lstm_model.summary()
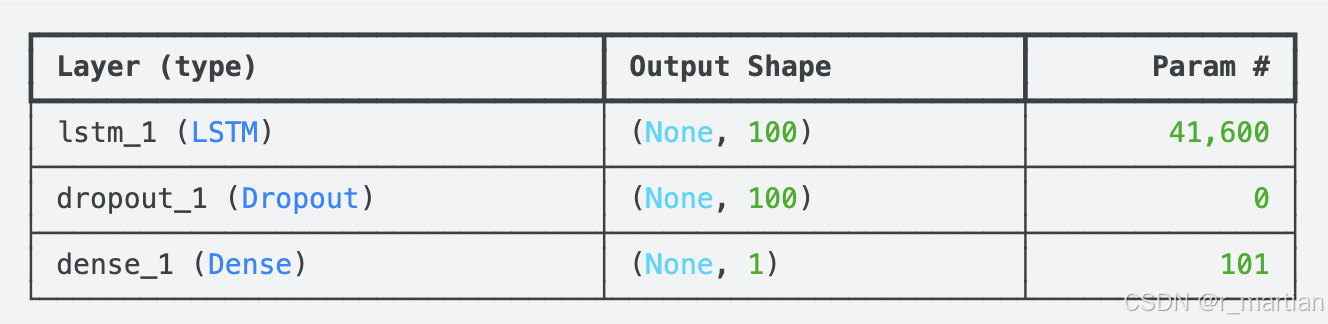
图 4
3.5 模型预测
py
# Make predictions
lstm_pred = lstm_model.predict(X_test)
lstm_pred = target_transformer.inverse_transform(lstm_pred) # Inverse transform to original scale
# Inverse transform the true values for comparison
y_test = y_test.reshape(-1, 1)
y_test = target_transformer.inverse_transform(y_test)
3.6 模型评估
py
# Calculate RMSE and R2 scores
from sklearn.metrics import mean_squared_error, r2_score, mean_absolute_error
rmse = np.sqrt(mean_squared_error(y_test, lstm_pred))
r2 = r2_score(y_test, lstm_pred)
print(f'RMSE: {rmse}')
print(f'R2 Score: {r2}')
# Plot the results
plt.figure(figsize=(14, 7))
plt.plot(df.index[-len(y_test):], y_test, label='True Values')
plt.plot(df.index[-len(y_test):], lstm_pred, label='Predictions', linestyle='dashed')
plt.xlabel('Date')
plt.ylabel('Mean Temperature')
plt.title('Mean Temperature Predictions vs True Values')
plt.legend()
plt.show()
# Get training and validation losses from history
training_loss = history.history['loss']
validation_loss = history.history['val_loss']
# Plot loss values over epochs
plt.plot(training_loss, label='Training Loss')
plt.plot(validation_loss, label='Validation Loss')
plt.xlabel('Epoch')
plt.ylabel('Loss (MSE)')
plt.title('Training and Validation Loss')
plt.legend()
plt.show()
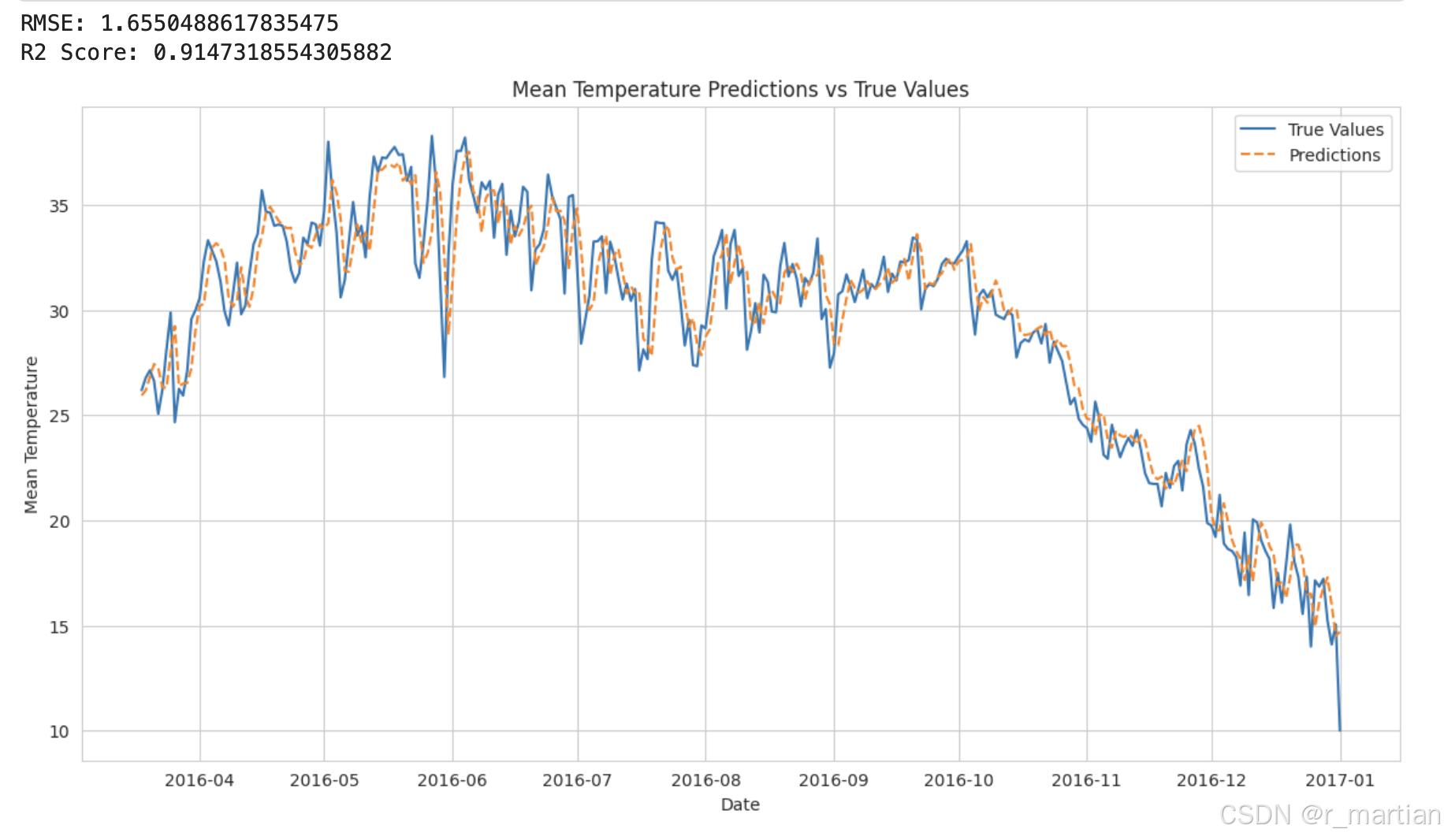
图 5
图 6
从结果看,模型拟合的效果还可以,相比于模型默认参数,做了一些参数调教,比如网络层数、激活函数以及Dropout等,还有一些参数尝试过调整,效果都一般,主要是对比不同参数下RMSE和R2两个值。后续出一些关于模型调参的文章,本文完。