目录
前言
摄像头OCR指的是利用摄像头捕捉图像中的文字信息,并通过光学字符识别(OCR)技术将其转换为可编辑的文本。
一、三个函数
1.显示图像
python
def cv_show(name, img):
cv2.imshow(name, img)
cv2.waitKey(60)
2.点排序
接收传入的坐标(为轮廓的四个顶点),
- 对每一行进行求和,
- 最小值是该轮廓的左上角,
- 最大值是右下角,
- 对每一行进行求差,
- 最小的是右上角,
- 最大的是右下角,
- 按照左上,右上,右下,左下的顺序填入rect矩阵
python
def order_points(pts):
# 共4个坐标点
rect = np.zeros((4, 2), dtype="float32") # 用来存储排序之后的坐标位置
# 按顺序找到对应坐标 0 1 2 3 分别是左上,右上,右下,左下
s = pts.sum(axis=1) # 对pts矩阵的每一行进行求和操作。 (x+y)
rect[0] = pts[np.argmin(s)]
rect[2] = pts[np.argmax(s)]
diff = np.diff(pts, axis=1) # 对pts矩阵的每一行进行求差操作。(y-x)
rect[1] = pts[np.argmin(diff)]
rect[3] = pts[np.argmax(diff)]
return rect
3.透视变换
- 获取排序之后的点坐标
- 计算该轮廓的宽和高的较大值,当做变换之后的图像宽高
- 通过cv2.getPerspectiveTransform方法计算透视变换矩阵
- 再通过cv2.warpPerspective方法获取透视变换之后的图像
python
def four_point_transform(image, pts):
# 获取输入坐标点
rect = order_points(pts)
(tl, tr, br, bl) = rect
# 计算输入的w和h的值 欧式距离公式
widthA = np.sqrt(((br[0] - bl[0]) ** 2) + ((br[1] - bl[1]) ** 2))
widthB = np.sqrt(((tr[0] - tl[0]) ** 2) + ((tr[1] - tl[1]) ** 2))
maxWidth = max(int(widthA), int(widthB))
heightA = np.sqrt(((tr[0] - br[0]) ** 2) + ((tr[1] - br[1]) ** 2))
heightB = np.sqrt(((tl[0] - bl[0]) ** 2) + ((tl[1] - bl[1]) ** 2))
maxHeight = max(int(heightA), int(heightB))
# 变换后对应坐标位置
dst = np.array([[0, 0], [maxWidth - 1, 0],
[maxWidth - 1, maxHeight - 1], [0, maxHeight - 1]], dtype="float32")
# 计算透视变换矩阵
M = cv2.getPerspectiveTransform(rect, dst)
# 应用透视变换
warped = cv2.warpPerspective(image, M, (maxWidth, maxHeight)) # 返回变换后结果
return warped
二、代码实例
1.打开摄像头
- 参数为0 则用电脑自带摄像头
- 参数为1 则用外接摄像头
- 若摄像头未被打开则输出Cannot open camera
python
cap = cv2.VideoCapture(0) # 确保摄像头是可以启动的状态 电脑自带摄像头用0 外接的用1
if not cap.isOpened():
print("Cannot open camera")
exit()
2.图像预处理
- 打开摄像头之后,读取每一帧的画面并显示
- 转换成灰度图,进行高斯滤波处理,
- 然后使用Canny算子进行边缘检测并显示,
- 再对边缘检测之后的图像进行轮廓检测,
- 只取轮廓大小前十的轮廓将其画出来,并显示
python
while True:
flag = 0 # 标识符 当前是否检测到文档
ret, image = cap.read()
orig = image.copy()
if not ret:
print('不能读取摄像头')
break
cv_show('image', image)
gray = cv2.cvtColor(image, cv2.COLOR_BGR2GRAY)
gray = cv2.GaussianBlur(gray, (5, 5), 0)
edged = cv2.Canny(gray, 75, 200)
cv_show('1', edged)
cnts = cv2.findContours(edged.copy(), cv2.RETR_EXTERNAL, cv2.CHAIN_APPROX_SIMPLE)[1]
cnts = sorted(cnts, key=cv2.contourArea, reverse=True)[:10]
image_contours = cv2.drawContours(image, cnts, -1, (0, 255, 0), 2)
cv_show('image_contours', image_contours)
输出:
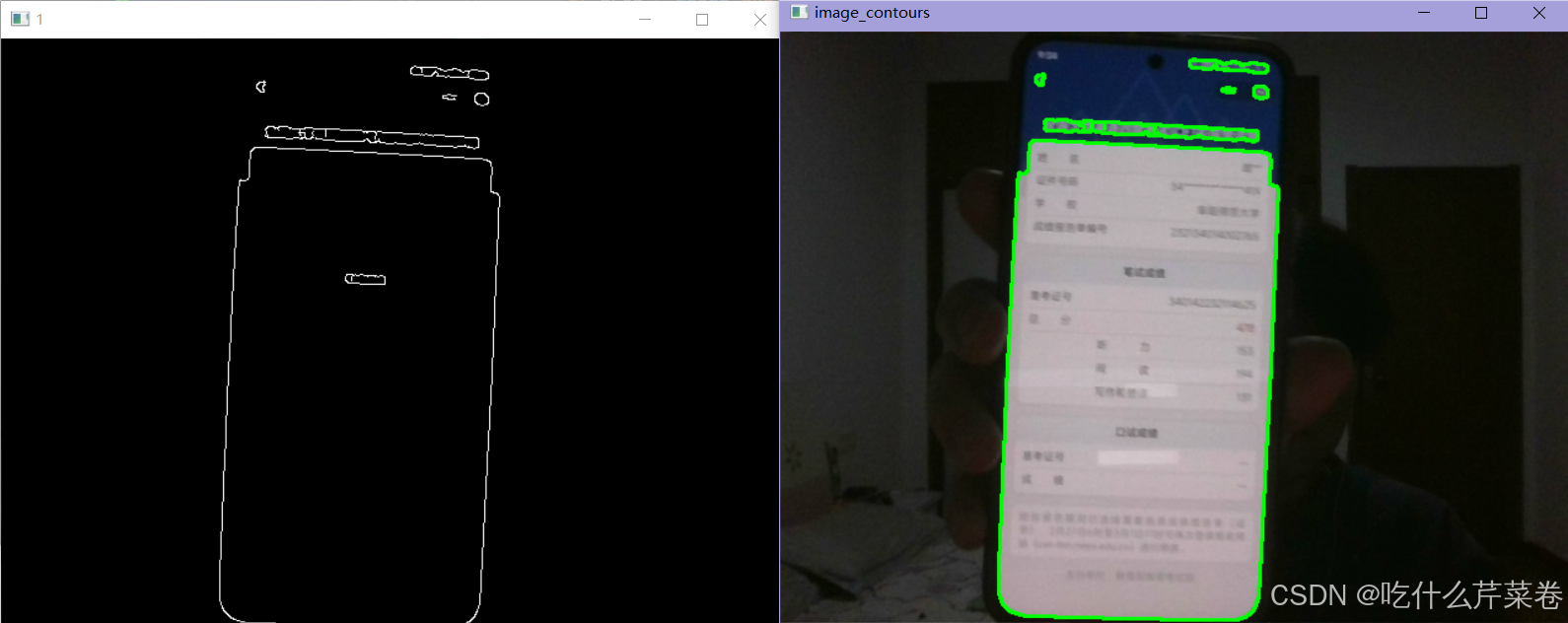
3.检测特定轮廓
- 遍历上述获取的轮廓
- 对轮廓进行近似处理,并获取其特征点集
- 判断轮廓面积大于20000 并且特征点集只有4个
python
for c in cnts:
peri = cv2.arcLength(c, True) # 计算轮廓的周长
# True表示是否选择封闭轮廓
approx = cv2.approxPolyDP(c, 0.05 * peri, True) # 返回轮廓点集
area = cv2.contourArea(approx)
if area > 20000 and len(approx) == 4:
screenCnt = approx
flag = 1
print(peri, area)
print('检测到文档')
break
4.对轮廓进行处理
- 如果在画面中获取到了符合条件的轮廓
- 就在原图上画出该轮廓
- 并将该轮廓图像进行透视变换并显示
- 最后对其进行二值化处理并显示
python
if flag == 1:
image_contours = cv2.drawContours(image, [screenCnt], 0, (0, 255, 0), 2)
cv_show('image', image_contours)
warped = four_point_transform(orig, screenCnt.reshape(4, 2))
cv_show('warped', warped)
warped = cv2.cvtColor(warped, cv2.COLOR_BGR2GRAY)
ref = cv2.threshold(warped, 0, 255, cv2.THRESH_BINARY | cv2.THRESH_OTSU)[1]
cv2.imshow('ref', ref)
cv2.waitKey(0)
输出:
5.释放资源
- 最后循环结束之后记得释放资源
python
cap.release() # 释放捕获器
cv2.destroyAllWindows() # 关闭图像窗口